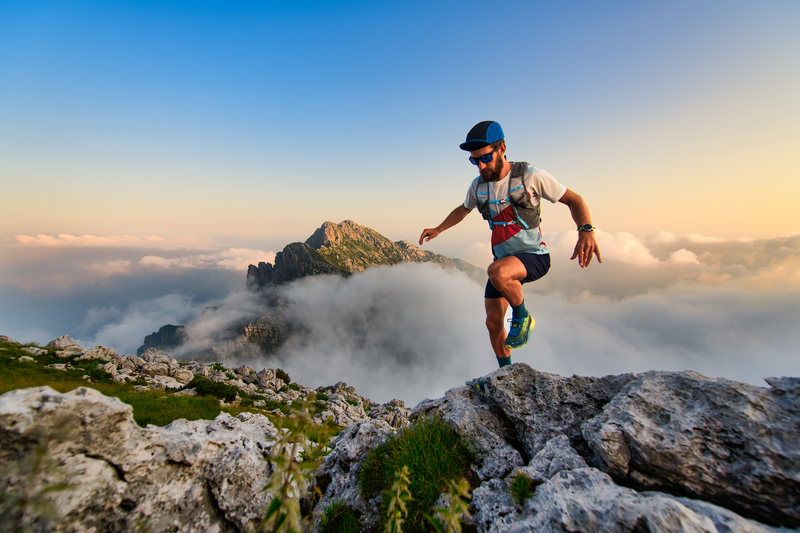
95% of researchers rate our articles as excellent or good
Learn more about the work of our research integrity team to safeguard the quality of each article we publish.
Find out more
ORIGINAL RESEARCH article
Front. Oncol. , 13 May 2022
Sec. Thoracic Oncology
Volume 12 - 2022 | https://doi.org/10.3389/fonc.2022.817372
This article is part of the Research Topic Epidemiology, Screening and Diagnosis of Lung Cancer View all 24 articles
Background: This study aimed to develop an artificial neural network (ANN) model for predicting synchronous organ-specific metastasis in lung cancer (LC) patients.
Methods: A total of 62,151 patients who diagnosed as LC without data missing between 2010 and 2015 were identified from Surveillance, Epidemiology, and End Results (SEER) program. The ANN model was trained and tested on an 75/25 split of the dataset. The receiver operating characteristic (ROC) curves, area under the curve (AUC) and sensitivity were used to evaluate and compare the ANN model with the random forest model.
Results: For distant metastasis in the whole cohort, the ANN model had metrics AUC = 0.759, accuracy = 0.669, sensitivity = 0.906, and specificity = 0.613, which was better than the random forest model. For organ-specific metastasis in the cohort with distant metastasis, the sensitivity in bone metastasis, brain metastasis and liver metastasis were 0.913, 0.906 and 0.925, respectively. The most important variable was separate tumor nodules with 100% importance. The second important variable was visceral pleural invasion for distant metastasis, while histology for organ-specific metastasis.
Conclusions: Our study developed a “two-step” ANN model for predicting synchronous organ-specific metastasis in LC patients. This ANN model may provide clinicians with more personalized clinical decisions, contribute to rationalize metastasis screening, and reduce the burden on patients and the health care system.
Lung cancer (LC) is one of the most commonly diagnosed malignancy as well as the leading cause of cancer-related death both in males and females worldwide (1, 2). Approximately 30-40% of LC patients present with distant metastasis (DM) at the time of diagnosis (3–5). And distant metastasis is responsible for a large morbidity and mortality burden among LC patients (6, 7). The most common metastatic site is bone, followed by liver, brain and adrenal gland (8, 9). Distant metastasis is closely related to treatment decisions and clinical outcomes. Therefore, it is important to identify and diagnose distant metastasis in the early period.
Computed tomography (CT), magnetic resonance imaging (MRI), single-photon emission computed tomography (SPECT) and positron emission tomography/computed tomography (PET/CT) are the common techniques to screen the distant metastasis in LC patients. However, routine DM screening to all LC patients is controversial because of low detection rate of asymptomatic patients, invasive operation, potential risk of adverse reactions, complex process and high cost (10–14). Therefore, there are strong requirements for the identification of a high-risk group with distant metastasis and the rationalization of DM screening in LC patients.
The occurrence and development of lung cancer is very complicated, and most of the clinical characteristics exhibit a multidimensional and non-linear relationship. The artificial neural network (ANN) is a complex non-linear model inspired by the working of biological neural networks (15–17). In the face of huge and complex medical data, it has the ability to discover underlying patterns and constantly adjust the algorithm to adapt to new patient information (18–20). In recent years, the ANN has been applied successfully in clinical medicine, including diagnosis, image identification and outcome prediction (16, 21–24).
In this study, we aim to develop an ANN model to predict synchronous organ-specific metastasis in LC patients. This study may provide clinicians with more personalized clinical decisions, reduce the unnecessary financial burden of patients, and allocate medical resources more rationally.
We obtained the research participants from the Surveillance, Epidemiology, and End Results (SEER) Program. The SEER program is supported by the US National Cancer Institute, covers cases from 18 cancer registries, and represents approximately 28-30% of the population (25). Patient data were screened via the SEER*Stat software (version 8.3.6). Since the data was anonymized, no additional institutional review board approval or patient informed consent was required.
We included patients diagnosed with lung cancer between 2010 and 2015. Variables of interest included age, sex, race, marital status, insurance, primary site, histology, grade, tumor size, separate tumor nodules, visceral pleural invasion, T-stage, N-stage, and organ-specific metastases. We excluded the patients whose reporting sources were “Autopsy only” or “Death certification only”, as well as those who did not have complete information on all the above variables.
A multilayer perceptron ANN was created consisting of an input, an output, and one or more hidden layers (Figure 1). In this research, thirteen selected demographic or clinical variables were served as the input layers neurons, and one variable (metastasis or no metastasis) was served as the output layer neuron. The number of neurons in the hidden layer was set empirically. 75% of patients was used to develop the model (the training group), while the remaining 25% was used to evaluate the developed model (the testing group). A back propagation (BP) method was used to train the multilayer perceptron ANN, which modified the weight of the interneuron connections to reduce the total errors during the repeated development cycles. During the learning progresses, the errors between ANN model outputs and expected outputs were minimized (21). In this study, the number of epochs was selected from the set {10, 20, 30, 50, 100, 500}.
Figure 1 Schematic structure of the artificial neural network (ANN) model including one input layer with 13 nodes, nine hidden layers with 100 nodes, and one output layer with 1 node.
Kaplan-Meier analysis was used for comparison of survival among the subgroups classified by distant metastasis. Multivariate Cox proportional hazard analyses was conducted to estimate the hazard ratio (HR), and the corresponding 95% confidence interval (CI), for the potential risk factors. The model performance was evaluated with the receiver operating characteristic (ROC) curves and areas under the curve (AUC), which is a score ranging from 0.50 to 1.0. All statistical analyses were conducted using SPSS version 21.0 and RStudio Version 1.0.153. A two-tailed P value <0.05 was considered statistically significant.
From 2010 to 2015, 62,151 patients with lung cancer were consecutively included in this study. Patient characteristics were described in Table 1. The population with a median age of 68 (IQR, 61-75) years and White people (n=50589, 81.4%) predominated. The distribution of male and female was almost 1:1. The most common primary site was upper lobe (n=37284, 60%) and the most common histological subtype was adenocarcinoma (n=33036, 53.2%). Of these patients, 12,182 (19.6%) developed distant metastases, including 3,982 (6.4%) with bone metastases, 3,674 (5.9%) with brain metastases, and 1,307 (2.1%) with liver metastases.
A cohort of 29,296 patients was used to analyze cancer-specific survival (CSS). The median CSS for patients with none metastasis, bone metastasis, brain metastasis, liver metastasis and two or three metastases were 10 months, 4 months, 4 months, 4 months and 3 months, respectively (Table 2). Kaplan-Meier analysis showed the similar trend in Figure 2. In addition, multivariate Cox proportional hazard analyses revealed that bone metastasis (OR=1.630, p<0.001), brain metastasis (OR=1.698, p<0.001), liver metastasis (OR=1.673, p<0.001) and two or three metastases (OR=2.025, p<0.001) were associated with poor prognosis (Table 2).
Figure 2 Kaplan-Meier analysis of cancer-specific survival for patients with lung cancer stratified by organ-specific metastasis.
In the training of ANN model, we manually increased the number of hidden layers starting with 5 layers. The predictive sensitivity culminated with 9 layers and adding more layer did not improve the performance but increased time of computation (Table 3). In the end, the ANN model was constructed with 13 neurons in the input layer, 100 neurons in each of the 9 hidden layers and 1 neuron in the output layer (Figure 1). Meanwhile, we compared the RF model (ntree=500) with the ANN model, and the RF model showed obvious overfitting (Figure 3).
Table 3 Performance of the artificial neural network (ANN) model with increasing layers for predicting distant metastasis.
Figure 3 Receiver operating characteristic curve of (A) the artificial neural network (ANN) model and (B) the random forest (RF) model.
In this study, we first evaluated the model performance for predicting distant metastasis in the whole cohort (AUC: 0.759, accuracy: 0.669, sensitivity: 0.906, specificity: 0.613, false positive rate: 0.387, false negative rate: 0.094, likelihood ratio positive: 2.339, likelihood ratio negative: 0.154). Then we evaluated the model performance for predicting organ-specific metastasis in the cohort with distant metastasis (Figure 4; Table 4). The sensitivity in bone metastasis, brain metastasis and liver metastasis were 0.913, 0.906 and 0.925, respectively.
Figure 4 Receiver operating characteristic curve of the artificial neural network (ANN) model for predicting organ-specific metastasis.
Table 4 Performance of the artificial neural network (ANN) model for predicting organ-specific metastasis.
By applying ANN methods with variable importance measures, the importance of the 13 variables was standardized and the top 10 were showed in Figure 5. The most important variable was separate tumor nodules with 100% importance. The second important variable was visceral pleural invasion for distant metastasis, while histology for organ-specific metastasis. And the sex variable only appeared in bone metastases. Relatively, the race and insurance variable were less important in the whole cohort.
Figure 5 Variable importance from the artificial neural network (ANN) model for predicting (A) distant metastasis and (B) organ-specific metastasis [(1) bone, (2) brain, and (3) liver].
With the increasing incidence of distant metastasis of lung cancer, this field has gradually become one of the hot spots in clinical research (26–29). Our study suggested that distant metastasis was a risk factor for poor prognosis, and the median CSS for LC patients with bone metastasis, brain metastasis, liver metastasis and two or three metastases are 4 months, 4 months, 4 months and 3 months, respectively, which was similar to previous studies (28–32). Thus, early identification and diagnosis of distant metastasis is meaningful to improve prognosis and can assist clinicians in making therapeutic choices.
However, the cost of screening in an unselected population is considerable and the benefit is questionable, given the conflicting international screening guidelines and clinicians’ possible tendency to conduct investigations in excess of the recommended stage (14, 33–35). In this study, we developed a “two-step” ANN model for predicting synchronous organ-specific metastasis in LC patients. Our ANN model has high predictive power, with sensitivity of 0.906 for distant metastasis, 0.913 for bone metastasis, 0.925 for brain metastasis and 0.906 for liver metastasis. It can help predict the possibility of organ-specific metastasis in LC patients and alert high-risk patients for further investigation, which can provide clinicians with more accurate and personalized clinical decisions.
Previously, Zhou et al. used machine learning methods to analyze the distant metastasis possibility of lung cancer based on clinical and radiomic features (36). In this study, if only the features extracted from the CT image were used, the AUC was 72.84%. After combined with the patients’ clinical features, 89.09% could be achieved. The authors did not utilize ANN and included radiomic features, limiting direct comparison with our model. Recently, Liu et al. constructed a nomogram to predict bone metastasis of small cell lung cancer (SCL), which had a c-index of 0.745 in the internal validation set (30). Meanwhile, a multivariate model developed by Cacho-Díaz et al. was used to predict brain metastases of non-small cell lung cancer (NSCLC) and showed a predictive sensibility of 72% (27). Although the random forest classifier showed a good performance in predicting overall survival and the early response during radiotherapy in NSCLC, it performed unsatisfactorily in the predictions of our study (37, 38). Therefore, compared with traditional statistical models, our ANN model has superior performance.
In this study, we identified important features in the ANN model, with the top five including separate tumor nodules, visceral pleural invasion, histology, N-stage and tumor size, which were in line with the previous studies (27, 28, 30, 32, 36, 39, 40). Similar to our study, sex and N-stage were reported to be related to the occurrence of bone metastases (30, 32, 40). Interestingly, the correlation between larger tumor size and a higher risk of bone metastasis was uncertain (30, 39). And it was reported that age, sex, T-stage were independent predictors of brain metastasis (27, 28, 31, 41). Although the carcinoembryonic antigen (CEA) levels and epidermal growth factor receptor gene (EGFR) mutation status were associated with brain metastasis in patients with newly diagnosed NSCLC, we did not include these variables because they were not provided in the SEER database (27, 41).
This study should be considered in the context of several limitations. First, the study does not include an independent external cohort to validate the model, which is an important focus of future research. Nonetheless, we hope that the use of the SEER database, which accounts for about 28% of the United States population, will improve generalizability. Second, due to retrospective studies, the excluded missing data may lead to selection bias. Therefore, 25% of patients were randomly assigned to the testing group, which allowed for pseudo-prospective evaluation of our model and thus reduced bias.
In conclusion, despite the limitations, we developed and validated a novel ANN model for the prediction of synchronous organ-specific metastasis in patients with lung cancer. This ANN model may help clinicians to make individualized prediction and rational metastasis screening.
The original contributions presented in the study are included in the article/supplementary material. Further inquiries can be directed to the corresponding authors.
Conception and design: HG, Z-GW and LX. Administrative support: LX and XLD. Provision of study materials or patients: Z-YH and HG. Collection and assembly of data: Z-YH and HG. Data analysis and interpretation: Z-GW and HG. Manuscript writing: All authors. Final approval of manuscript: All authors.
This study was supported by the National Natural Science Foundation of China (grant number 71874058).
The authors declare that the research was conducted in the absence of any commercial or financial relationships that could be construed as a potential conflict of interest.
All claims expressed in this article are solely those of the authors and do not necessarily represent those of their affiliated organizations, or those of the publisher, the editors and the reviewers. Any product that may be evaluated in this article, or claim that may be made by its manufacturer, is not guaranteed or endorsed by the publisher.
The authors acknowledge Guanglian Xiong, Jia Song and Yinghui Li for their advice on the modification of the model parameters. The authors acknowledge the efforts of the National Cancer Institute.
1. Bray F, Ferlay J, Soerjomataram I, Siegel RL, Torre LA, Jemal A. Global Cancer Statistics 2018: GLOBOCAN Estimates of Incidence and Mortality Worldwide for 36 Cancers in 185 Countries. CA Cancer J Clin (2018) 68(6):394–424. doi: 10.3322/caac.21492
2. Siegel RL, Miller KD, Jemal A. Cancer Statistics, 2017. CA Cancer J Clin (2017) 67(1):7–30. doi: 10.3322/caac.21387
3. Rosti G, Bevilacqua G, Bidoli P, Portalone L, Santo A, Genestreti G. Small Cell Lung Cancer. Ann Oncol (2006) 17 Suppl 2:ii5–10. doi: 10.1093/annonc/mdj910
4. Quint LE. Staging Non-Small Cell Lung Cancer. Cancer Imaging (2007) 7(1):148–59. doi: 10.1102/1470-7330.2007.0026
5. He YY, Zhang XC, Yang JJ, Niu FY, Zeng Z, Yan HH, et al. Prognostic Significance of Genotype and Number of Metastatic Sites in Advanced Non-Small-Cell Lung Cancer. Clin Lung Cancer (2014) 15(6):441–7. doi: 10.1016/j.cllc.2014.06.006
6. Wang X, Adjei AA. Lung Cancer and Metastasis: New Opportunities and Challenges. Cancer Metastasis Rev (2015) 34(2):169–71. doi: 10.1007/s10555-015-9562-4
7. Altorki NK, Markowitz GJ, Gao D, Port JL, Saxena A, Stiles B, et al. The Lung Microenvironment: An Important Regulator of Tumour Growth and Metastasis. Nat Rev Cancer (2019) 19(1):9–31. doi: 10.1038/s41568-018-0081-9
8. Tamura T, Kurishima K, Nakazawa K, Kagohashi K, Ishikawa H, Satoh H, et al. Specific Organ Metastases and Survival in Metastatic Non-Small-Cell Lung Cancer. Mol Clin Oncol (2015) 3(1):217–21. doi: 10.3892/mco.2014.410
9. Nakazawa K, Kurishima K, Tamura T, Kagohashi K, Ishikawa H, Satoh H, et al. Specific Organ Metastases and Survival in Small Cell Lung Cancer. Oncol Lett (2012) 4(4):617–20. doi: 10.3892/ol.2012.792
10. Schoenmaekers JJAO, Dingemans AC, Hendriks LEL. Brain Imaging in Early Stage Non-Small Cell Lung Cancer: Still a Controversial Topic? J Thorac Dis (2018) 10(Suppl 18):S2168–71. doi: 10.21037/jtd.2018.06.68
11. Tang C, Liu Y, Qin H, Li X, Guo W, Li J, et al. Clinical Significance of Serum BAP, TRACP 5b and ICTP as Bone Metabolic Markers for Bone Metastasis Screening in Lung Cancer Patients. Clin Chim Acta (2013) 426:102–7. doi: 10.1016/j.cca.2013.09.011
12. Barak A, Neudorfer M, Heilweil G, Merimsky O, Lowenstein A, Inbar M, et al. Decreased Prevalence of Asymptomatic Choroidal Metastasis in Disseminated Breast and Lung Cancer: Argument Against Screening. Br J Ophthalmol (2007) 91(1):74–5. doi: 10.1136/bjo.2006.099416
13. Chai X, Yinwang E, Wang Z, Wang Z, Xue Y, Li B, et al. Predictive and Prognostic Biomarkers for Lung Cancer Bone Metastasis and Their Therapeutic Value. Front Oncol (2021) 11:692788. doi: 10.3389/fonc.2021.692788
14. Vinod SK. Should We Screen for Brain Metastases in Non-Small Cell Lung Cancer? J Med Imaging Radiat Oncol (2018) 62(3):380–2. doi: 10.1111/1754-9485.12743
15. Sargent DJ. Comparison of Artificial Neural Networks With Other Statistical Approaches: Results From Medical Data Sets. Cancer (2001) 91(8 Suppl):1636–42. doi: 10.1002/1097-0142(20010415)91:8+<1636::aid-cncr1176>3.0.co;2-d
16. Kononenko I. Machine Learning for Medical Diagnosis: History, State of the Art and Perspective. Artif Intell Med (2001) 23(1):89–109. doi: 10.1016/s0933-3657(01)00077-x
17. Dayhoff JE, DeLeo JM. Artificial Neural Networks: Opening the Black Box. Cancer (2001) 91(8 Suppl):1615–35. doi: 10.1002/1097-0142(20010415)91:8+<1615::aid-cncr1175>3.0.co;2-l
18. Patel JL, Goyal RK. Applications of Artificial Neural Networks in Medical Science. Curr Clin Pharmacol (2007) 2(3):217–26. doi: 10.2174/157488407781668811
19. Kourou K, Exarchos TP, Exarchos KP, Karamouzis MV, Fotiadis DI. Machine Learning Applications in Cancer Prognosis and Prediction. Comput Struct Biotechnol J (2014) 13:8–17. doi: 10.1016/j.csbj.2014.11.005
20. Cruz JA, Wishart DS. Applications of Machine Learning in Cancer Prediction and Prognosis. Cancer Inform (2007) 2:59–77. doi: 10.1177/117693510600200030
21. Hu X, Cammann H, Meyer HA, Miller K, Jung K, Stephan C. Artificial Neural Networks and Prostate Cancer–Tools for Diagnosis and Management. Nat Rev Urol (2013) 10(3):174–82. doi: 10.1038/nrurol.2013.9
22. Bhambhvani HP, Zamora A, Shkolyar E, Prado K, Greenberg DR, Kasman AM, et al. Development of Robust Artificial Neural Networks for Prediction of 5-Year Survival in Bladder Cancer. Urol Oncol (2021) 39(3):193.e7–193.e12. doi: 10.1016/j.urolonc.2020.05.009
23. Tran KA, Kondrashova O, Bradley A, Williams ED, Pearson JV, Waddell N. Deep Learning in Cancer Diagnosis, Prognosis and Treatment Selection. Genome Med (2021) 13(1):152. doi: 10.1186/s13073-021-00968-x
24. Khanagar SB, Naik S, Al Kheraif AA, Vishwanathaiah S, Maganur PC, Alhazmi Y, et al. Application and Performance of Artificial Intelligence Technology in Oral Cancer Diagnosis and Prediction of Prognosis: A Systematic Review. Diagn (Basel) (2021) 11(6):1004. doi: 10.3390/diagnostics11061004
25. Wang ZG, He ZY, Chen YY, Huan G, Du XL. Incidence and Survival Outcomes of Secondary Liver Cancer: A Surveillance Epidemiology and End Results Database Analysis. Transl Cancer Res (2021) 10(3):1273–83. doi: 10.21037/tcr-20-3319
26. Liu W, Wu J. Lung Cancer With Bone Metastases in the United States: An Analysis From the Surveillance, Epidemiologic, and End Results Database. Clin Exp Metastasis (2018) 35(8):753–61. doi: 10.1007/s10585-018-9943-5
27. Cacho-Díaz B, Cuapaténcatl LD, Rodríguez JA, Garcilazo-Reyes YJ, Reynoso-Noverón N, Arrieta O. Identification of a High-Risk Group for Brain Metastases in Non-Small Cell Lung Cancer Patients. J Neurooncol (2021) 155(1):101–6. doi: 10.1007/s11060-021-03849-w
28. Reddy SP, Dowell JE, Pan E. Predictors of Prognosis of Synchronous Brain Metastases in Small-Cell Lung Cancer Patients. Clin Exp Metastasis (2020) 37(4):531–9. doi: 10.1007/s10585-020-10040-4
29. Cai H, Wang H, Li Z, Lin J, Yu J. The Prognostic Analysis of Different Metastatic Patterns in Extensive-Stage Small-Cell Lung Cancer Patients: A Large Population-Based Study. Future Oncol (2018) 14(14):1397–407. doi: 10.2217/fon-2017-0706
30. Liu C, Yi J, Jia J. Diagnostic and Prognostic Nomograms for Bone Metastasis in Small Cell Lung Cancer. J Int Med Res (2021) 49(10):3000605211050735. doi: 10.1177/03000605211050735
31. Zhu H, Zhou L, Guo Y, Yang G, Dong Q, Zhang Z, et al. Factors for Incidence Risk and Prognosis in Non-Small-Cell Lung Cancer Patients With Synchronous Brain Metastasis: A Population-Based Study. Future Oncol (2021) 17(19):2461–73. doi: 10.2217/fon-2021-0103
32. Zhang C, Mao M, Guo X, Cui P, Zhang L, Xu Y, et al. Nomogram Based on Homogeneous and Heterogeneous Associated Factors for Predicting Bone Metastases in Patients With Different Histological Types of Lung Cancer. BMC Cancer (2019) 19(1):238. doi: 10.1186/s12885-019-5445-3
33. Diaz ME, Debowski M, Hukins C, Fielding D, Fong KM, Bettington CS. Non-Small Cell Lung Cancer Brain Metastasis Screening in the Era of Positron Emission Tomography-CT Staging: Current Practice and Outcomes. J Med Imaging Radiat Oncol (2018) 62(3):383–8. doi: 10.1111/1754-9485.12732
34. Hudson BJ, Crawford MB, Curtin JJ. Brain Imaging in Lung Cancer Patients Without Symptoms of Brain Metastases: A National Survey of Current Practice in England. Clin Radiol (2015) 70(6):610–3. doi: 10.1016/j.crad.2015.02.007
35. Vernon J, Andruszkiewicz N, Schneider L, Schieman C, Finley CJ, Shargall Y, et al. Comprehensive Clinical Staging for Resectable Lung Cancer: Clinicopathological Correlations and the Role of Brain MRI. J Thorac Oncol (2016) 11(11):1970–5. doi: 10.1016/j.jtho.2016.06.003
36. Zhou H, Dong D, Chen B, Fang M, Cheng Y, Gan Y, et al. Diagnosis of Distant Metastasis of Lung Cancer: Based on Clinical and Radiomic Features. Transl Oncol (2018) 11(1):31–6. doi: 10.1016/j.tranon.2017.10.010
37. D’Amico NC, Sicilia R, Cordelli E, Tronchin L, Greco C, Fiore M, et al. Radiomics-Based Prediction of Overall Survival in Lung Cancer Using Different Volumes-of-Interest. Appl Sci (2020) 10:6425. doi: 10.3390/app10186425
38. Ramella S, Fiore M, Greco C, Cordelli E, Sicilia R, Merone M, et al. A Radiomic Approach for Adaptive Radiotherapy in Non-Small Cell Lung Cancer Patients. PloS One (2018) 13(11):e0207455. doi: 10.1371/journal.pone.0207455
39. Li J, Liu F, Yu H, Zhao C, Li Z, Wang H. Different Distant Metastasis Patterns Based on Tumor Size Could be Found in Extensive-Stage Small Cell Lung Cancer Patients: A Large, Population-Based SEER Study. PeerJ (2019) 7:e8163. doi: 10.7717/peerj.8163
40. Song Q, Shang J, Zhang C, Zhang L, Wu X. Impact of the Homogeneous and Heterogeneous Risk Factors on the Incidence and Survival Outcome of Bone Metastasis in NSCLC Patients. J Cancer Res Clin Oncol (2019) 145(3):737–46. doi: 10.1007/s00432-018-02826-7
Keywords: machine learning, artificial neural network, SEER, metastasis, lung cancer
Citation: Gao H, He Z-y, Du X-l, Wang Z-g and Xiang L (2022) Machine Learning for the Prediction of Synchronous Organ-Specific Metastasis in Patients With Lung Cancer. Front. Oncol. 12:817372. doi: 10.3389/fonc.2022.817372
Received: 18 November 2021; Accepted: 11 April 2022;
Published: 13 May 2022.
Edited by:
Yutong He, Fourth Hospital of Hebei Medical University, ChinaReviewed by:
Carlo Greco, Policlinico Universitario Campus Bio-Medico, ItalyCopyright © 2022 Gao, He, Du, Wang and Xiang. This is an open-access article distributed under the terms of the Creative Commons Attribution License (CC BY). The use, distribution or reproduction in other forums is permitted, provided the original author(s) and the copyright owner(s) are credited and that the original publication in this journal is cited, in accordance with accepted academic practice. No use, distribution or reproduction is permitted which does not comply with these terms.
*Correspondence: Zheng-gang Wang, ODQ2NzM1NTY0QHFxLmNvbQ==; Li Xiang, eGxseWZAaHVzdC5lZHUuY24=
Disclaimer: All claims expressed in this article are solely those of the authors and do not necessarily represent those of their affiliated organizations, or those of the publisher, the editors and the reviewers. Any product that may be evaluated in this article or claim that may be made by its manufacturer is not guaranteed or endorsed by the publisher.
Research integrity at Frontiers
Learn more about the work of our research integrity team to safeguard the quality of each article we publish.