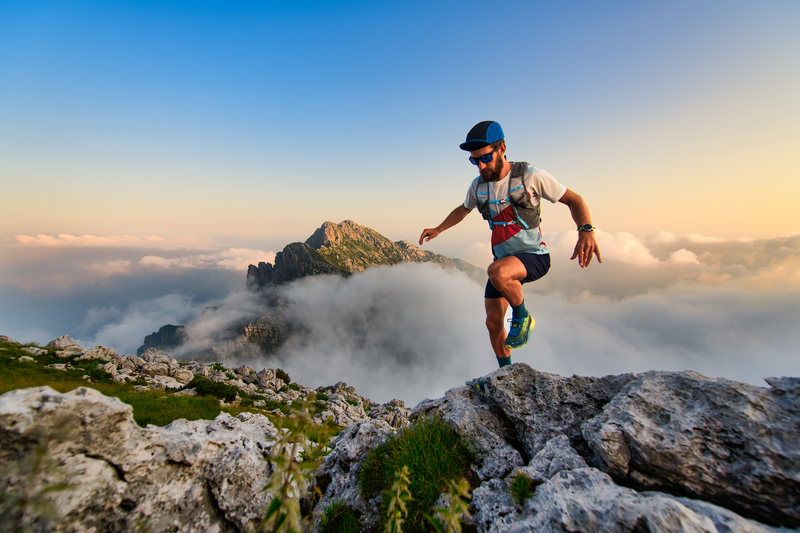
94% of researchers rate our articles as excellent or good
Learn more about the work of our research integrity team to safeguard the quality of each article we publish.
Find out more
ORIGINAL RESEARCH article
Front. Nutr.
Sec. Nutrition and Metabolism
Volume 12 - 2025 | doi: 10.3389/fnut.2025.1557280
The final, formatted version of the article will be published soon.
You have multiple emails registered with Frontiers:
Please enter your email address:
If you already have an account, please login
You don't have a Frontiers account ? You can register here
Background: Gut microbiota is reported to be related to the onset of insulin resistance (IR) and type 2 diabetes mellitus (T2DM). The dietary index for gut microbiota (DI-GM) is a novel index for reflecting gut microbiota diversity. We aimed to evaluate the association of DI-GM with T2DM and IR. Methods: This cross-sectional research comprised 10 600 participants aged ≥20 from the National Health and Nutrition Examination Survey (NHANES) 2007-2018. We employed weighted multivariable linear and logistic regression models to examine the correlation of DI-GM with T2DM and IR. Linear or nonlinear relationships were examined by restricted cubic spline (RCS) regression. Additionally, subgroup and sensitivity analyses were performed to ensure the reliability of the results. Mediation analysis explored the roles of body mass index (BMI) and inflammatory factors in these associations. Results: Higher DI-GM were inversely associated with T2DM (OR=0.93, 95%CI: 0.89-0.98) and IR (OR=0.95, 95%CI: 0.91-0.99) after adjusting for confounders. DI-GM≥6 group showed significantly lower risks of T2DM (OR=0.74, 95%CI: 0.60-0.91) and IR (OR=0.77, 95%CI: 0.62-0.95). RCS demonstrated a linear relationship between DI-GM and T2DM, as well as IR. DI-GM was also inversely correlated with the risk markers of T2DM. Mediation analysis showed that BMI and the systemic inflammation response index partly mediated the association of DI-GM with T2DM and IR, while the systemic immune-inflammation index mediated only the association with T2DM. Conclusions: DI-GM is inversely associated with T2DM and IR, with BMI and inflammatory markers partly mediating this association.
Keywords: Gut Microbiota, type 2 diabetes mellitus, Insulin Resistance, BMI, Inflammatory marker, NHANES
Received: 08 Jan 2025; Accepted: 07 Mar 2025.
Copyright: © 2025 Qu, Yang, Xie, Ye and Shao. This is an open-access article distributed under the terms of the Creative Commons Attribution License (CC BY). The use, distribution or reproduction in other forums is permitted, provided the original author(s) or licensor are credited and that the original publication in this journal is cited, in accordance with accepted academic practice. No use, distribution or reproduction is permitted which does not comply with these terms.
* Correspondence:
Liu Ye, The First Branch, The First Affiliated Hospital of Chongqing Medical University, Chongqing, China
Yue Shao, First Affiliated Hospital of Chongqing Medical University, Chongqing, China
Disclaimer: All claims expressed in this article are solely those of the authors and do not necessarily represent those of their affiliated organizations, or those of the publisher, the editors and the reviewers. Any product that may be evaluated in this article or claim that may be made by its manufacturer is not guaranteed or endorsed by the publisher.
Research integrity at Frontiers
Learn more about the work of our research integrity team to safeguard the quality of each article we publish.