- 1Neurological Intensive Care Unit, Deyang People's Hospital, Deyang, China
- 2Department of Nursing, Deyang People's Hospital, Deyang, China
- 3Department of Nursing, Affiliated Hospital of Southwest Medical University, Luzhou, China
Background: This study collects and analyzes clinical data on enteral nutrition therapy in neurocritical patients, develops and validates a feeding intolerance (FI) risk prediction model, and provides a theoretical basis for screening patients with high risk of feeding intolerance (FI) and delivering personalized care.
Methods: A convenience sampling method was employed to select 300 patients who were admitted to a tertiary hospital in China for early enteral nutrition therapy in the neurointensive care unit between April 2022 and December 2022. Independent risk factors for FI were identified using univariate and logistic regression analyses. A prediction model was established, and the goodness of fit and discriminant validity of the model were evaluated.
Results: The incidence of FI in neurocritical patients receiving enteral nutrition was 71%. Logistic regression analysis identified age, Glasgow Coma Scale (GCS) scores, Acute Physiology and Chronic Health Evaluation II (APACHE II) scores, mechanical ventilation, feeding via the nasogastric tube route, hyperglycemia, and low serum albumin as independent risk factors for the development of FI (p < 0.05). The predictive formula for FI risk was established as follows: Logit p = −14.737 + 1.184 × mechanical ventilation +2.309 × feeding route +1.650 × age + 1.336 × GCS tertile (6–8 points) + 1.696 × GCS tertile (3–5 points) + 1.753 × APACHE II score + 1.683 × blood glucose value +1.954 × serum albumin concentration. The Hosmer–Lemeshow test showed χ2 = 9.622, p = 0.293, and the area under the ROC curve was 0.941 (95% confidence interval: 0.912–0.970, p < 0.001). The optimal critical value was 0.767, with a sensitivity of 85.9%, a specificity of 90.8%, and a Youden index of 0.715.
Conclusion: The early enteral nutrition FI risk prediction model developed in this study demonstrated good predictive ability. This model can serve as a valuable reference for effectively assessing the risk of FI in neurocritical patients, thereby enhancing clinical outcomes.
1 Introduction
Enteral nutrition is the preferred method of providing nutrition to critically ill patients. Both the Society of Critical Care Medicine and the American Society for Parenteral and Enteral Nutrition recommend that enteral nutrition should be initiated as early as possible within 24–48 h of admission to the care unit when there are no contraindications to enteral nutrition (1). Early enteral nutrition helps nourish the gastrointestinal mucosa and enhance neuroendocrine function, thereby protecting the intestinal mucosal barrier and immune function (2–5). Enteral nutrition has been found to be associated with reduced infection rates, accelerated wound healing, reduced duration of invasive mechanical ventilation, shorter length of stay in the care unit, and reduced mortality rates (6, 7). However, during clinical practice, acute gastrointestinal dysfunction often occurs in critically ill patients under intense stress, with feeding intolerance (FI) being the most common clinical manifestation (8). According to some studies, the incidence of FI in critically ill patients ranges from 30.5 to 75% (9–12).
The neurointensive care unit (NCU) is a specific facility that mainly admits and treats critically ill patients with neurological diseases and concurrent or potential organ dysfunction, such as severe ischemic stroke, cerebral hemorrhage, severe traumatic brain injury (TBI), and increased intracranial pressure, among others (13). These patients often experience a higher incidence of FI due to impaired consciousness, abnormal brain-gut axis regulation, vagal inhibition, and so on (14, 15). The occurrence of FI in NCU patients poses a substantial risk for poor prognosis, including increased duration of invasive mechanical ventilation, prolonged hospitalization, increased infection rate, and mortality rate, among others (16–18).
However, the awareness level of FI among healthcare professionals may vary due to the absence of a standardized definition. In a 2014 meta-analysis (19), up to 43 clinical manifestations of FI were observed, such as vomiting, regurgitation, diarrhea, constipation, increased abdominal girth, subjective discomfort, and aspiration. The commonly used definition of FI in clinical practice is based on the 2012 European Society of Critical Care Medicine definition of FI (20), which includes the following three aspects: the presence of gastrointestinal discomfort symptoms, such as vomiting, abdominal distension, diarrhea, constipation, loss of bowel sounds, and a high gastric residual volume (GRV) >500 mL/d; the discontinuation of enteral nutrition due to gastrointestinal bleeding or other factors; and the failure to achieve an energy intake of 20 kcal/kg/d within 72 h of the start of enteral nutrition. FI is considered to have occurred if one or more of the above criteria are met.
Current preventive measures for FI mainly include early identification of risk factors and early intervention. Numerous factors for FI have been identified, including mechanical ventilation (19), hyperglycemia (8), and hypoproteinemia (21). For early detection, methods such as ultrasound (22, 23), intra-abdominal pressure monitoring (24), and biomarkers (25) can be employed; however, their specificity remains to be tested. In addition, although some studies have proposed risk factors for FI and established risk prediction models, the majority of these studies target critically ill patients or patients with severe pancreatitis and fewer for NCU patients. Therefore, this study aims to identify the risk factors and develop a prediction model for FI during early EN in NCU patients in order to recognize the high-risk group at an early stage, thereby providing a valuable clinical reference for timely intervention and improvement of patient outcomes.
2 Materials and methods
2.1 Study design
This prospective cohort study was conducted in a 24-bed NCU at a tertiary general hospital in China, which also serves as a teaching hospital. The study took place between April 2022 and December 2022. No additional interventions were conducted other than the necessary assessments. Therefore, data collection was not burdensome for patients, and data were collected and analyzed anonymously. The study was also approved by the hospital’s Ethics Committee under the ethical code 2022-04-042-K01.
2.2 Enrollment
A convenience sampling method was adopted to collect clinical data on 300 patients admitted to the NCU for early enteral nutrition. The inclusion criteria for this study are as follows: (1) critically ill patients with neurological disease and existing or potential organ dysfunction; (2) aged ≥18 years; (3) first placement of nasogastric/nasoenteric tubes; (4) enteral feeding was initiated within 48 h of admission; (5) nasal feeding for ≥7 days; and (6) patients/family members signed an informed consent form and were willing to cooperate with the investigator.
The exclusion criteria are as follows: (1) severe nutritional disorders, digestive insufficiency, and cirrhosis; (2) previous intestinal obstruction; (3) bleeding from the esophagus, stomach, or intestines; and (4) incomplete data.
2.3 Definition of feeding intolerance
According to the FI definition of the European Society of Critical Care Medicine (20) and considering the disease characteristics of NCU patients, the judgment criteria for FI in this study were as follows: ① Vomiting/reflux: It refers to the passage of gastric contents through the esophagus and out of the body through the mouth. The criterion for judging vomiting/reflux was the presence of nutrient fluid in the mouth or spillage of nutrient fluid from a nasogastric or nasoenteric tube when the tube is opened. ② Diarrhea: Patients with >3 bowel movements per day, feces with a water content of 80% or more, and unformed consistency were considered to have diarrhea. Using the HART diarrhea scoring method (26), each bowel movement was scored, and diarrhea was considered to be present if the 24-h cumulative total score was ≥12. ③ Constipation: This is defined as no bowel movement for 3 consecutive days or one bowel movement in 2–3 days, with dry and hard stool is dry and hard amounting to <50 g. ④ Aspiration: This refers to the aspiration of gastric contents via the airway. ⑤ High gastric residual volume (GRV): A volume of ≥500 mL per day was considered high; ⑥ Gastrointestinal Bleeding: This is determined after the patient vomited, regurgitated fluid, or had visible bloody fluid in the stool along with a positive occult blood test and/or after the diagnosis has been confirmed by a physician, such as through gastroscopy.
2.4 Method of nutritional support
Nurses in NCU followed the doctor’s orders to initiate enteral nutrition therapy for patients. The nursing team in the department developed an enteral nutrition therapy program, drawing upon several key resources for guidance. These resources included “Expert Consensus on Enteral Feeding Nursing for Patients with Severe Neurocritical Diseases (2022 Edition)” (27), “Nursing Practice Guideline For Enteral Nutrition In Patients With Stroke (2021 Edition)” (28), and the group standard “Intubation And Maintenance Of Nasointestinal Tube In Adult Patients” (29) issued by the Chinese Nursing Association in January 2022. The program includes several practices as follows: (1) utilizing a special pump to administer the EN solution at a temperature of approximately 37–40°C; (2) placing special signs for EN beside the patient’s bed to distinguish it from intravenous infusion; (3) flushing the tubes with 20–30 mL of warm water before and after each feeding, as well as every 4 h during the infusion of EN. Every shift, the exposed portion of the feeding tube should be inspected, and the nasal cavity should be checked. Moreover, the airbag pressure should be maintained at 25–30 cmH2O for patients with artificial airways, and subglottic suction should be performed simultaneously.
2.5 Candidate predictors
After analyzing the relevant literature on FI, integrating this information with clinical data, and engaging in discussions with experts, we identified risk factors that may affect the occurrence of FI in neurocritical patients. We identified 27 potential predictors categorized into four main groups: (1) individual patient factors: age, sex, body mass index (BMI) (30), smoking history, drinking history; (2) disease characteristics: primary diagnosis, past history, Glasgow Coma Scale (GCS) scores (31), nutritional risk screening (NRS) 2002 scores (32), Acute Physiology and Chronic Health Evaluation II (APACHE II) scores (33); (3) therapeutic factors: whether surgery was performed, use of mechanical ventilation, target temperature management, use of two or more antibiotics, use of vasoactive drugs, sedatives, analgesics, potassium preparations, acid inhibitors, feeding routes, and nasal feeding preparations; (4) monitoring data: body temperature, level of blood glucose, serum albumin, prealbumin, serum lactate, and potassium.
2.6 Data collection
The data for this study were obtained from electronic medical records and critical care information systems. Data collection for this study began when enteral nutrition was initiated in NCU patients. The collector conducted a 7-day data collection of patients’ FI at 03:00, 09:00, 15:00, and 21:00 daily. Two investigators collected the data with mutual verification of completeness, authenticity, and accuracy. Additionally, a dedicated individual was tasked with maintaining complete data records.
2.7 Sample size
According to the sample size calculation criteria based on the logistic regression analysis proposed by Gao and Zhang (34), the sample size should be 5–10 times the number of independent variables divided by the incidence of disease. A total of 19 independent variables were included in this study. With the incidence of FI at approximately 68.3%, as determined by a small pre-survey in the hospital, and assuming a 10% loss to follow-up, the minimum sample size required for this study is calculated as follows: 27*5*(1 + 10%)/68.3% = 217.
2.8 Statistical analysis
Patients were divided into FI and non-FI groups based on the diagnostic criteria. Excel 2013 software was used to create a double-entry database for double entry for data validation, and SPSS Statistics version 25.0 software was used for data analysis. Count data were expressed as frequency and percentage (%), and the chi-Squared (χ2) test was performed between the two groups. Normal measure data were expressed as mean ± standard deviation (SD), and two independent samples t-test was used for comparison between groups; non-normal measure data were expressed as median and quartile, and Wilcoxon rank-sum test in non-parametric test was used for comparison between groups. A risk prediction model was developed using the binary logistic regression analysis, and the model’s distinction and calibration were evaluated using the receiver operating characteristic (ROC) area under the curve (AUC) and Hosmer–Lemeshow (H-L) goodness-of-fit test. The application efficacy of the model was verified by its accuracy, with a significance level of p < 0.05 was used in this study.
3 Results
3.1 Patient characteristics
Ultimately, 300 patients were included in this study based on the inclusion and exclusion criteria (Figure 1). Among them, 213 were classified into the FI group, resulting in an overall incidence rate of 71%. Within the FI group, constipation was identified in 105 cases (49.3%), diarrhea in 80 cases (37.6%), reflux/vomiting in 20 cases (9.38%), aspiration in 5 cases (2.34%), high gastric remnants in 2 cases (0.93%), and gastrointestinal bleeding in 1 case (0.5%).
3.2 Univariate analysis of FI
The univariate analysis showed statistically significant differences between the two groups in several factors such as age, APACHE II scores, GCS scores, blood glucose, serum albumin, serum lactate, blood potassium, mechanical ventilation, sedation, route of feeding, and temperature ≥ 38.5°C(p < 0.05) (Table 1).
3.3 Logistic regression analysis of FI
Logistic regression analyses were conducted with the incidence of FI as the dependent variable and the variables with significant differences in the univariate analysis as the independent variables. The assignments of variables are shown in Table 2. Logistic regression analyses showed that seven factors were associated with FI, including age, GCS scores, APACHE II scores, route of feeding, mechanical ventilation, hyperglycemia, and serum albumin. The visualization results of the logistic regression analyses are shown in Table 3.
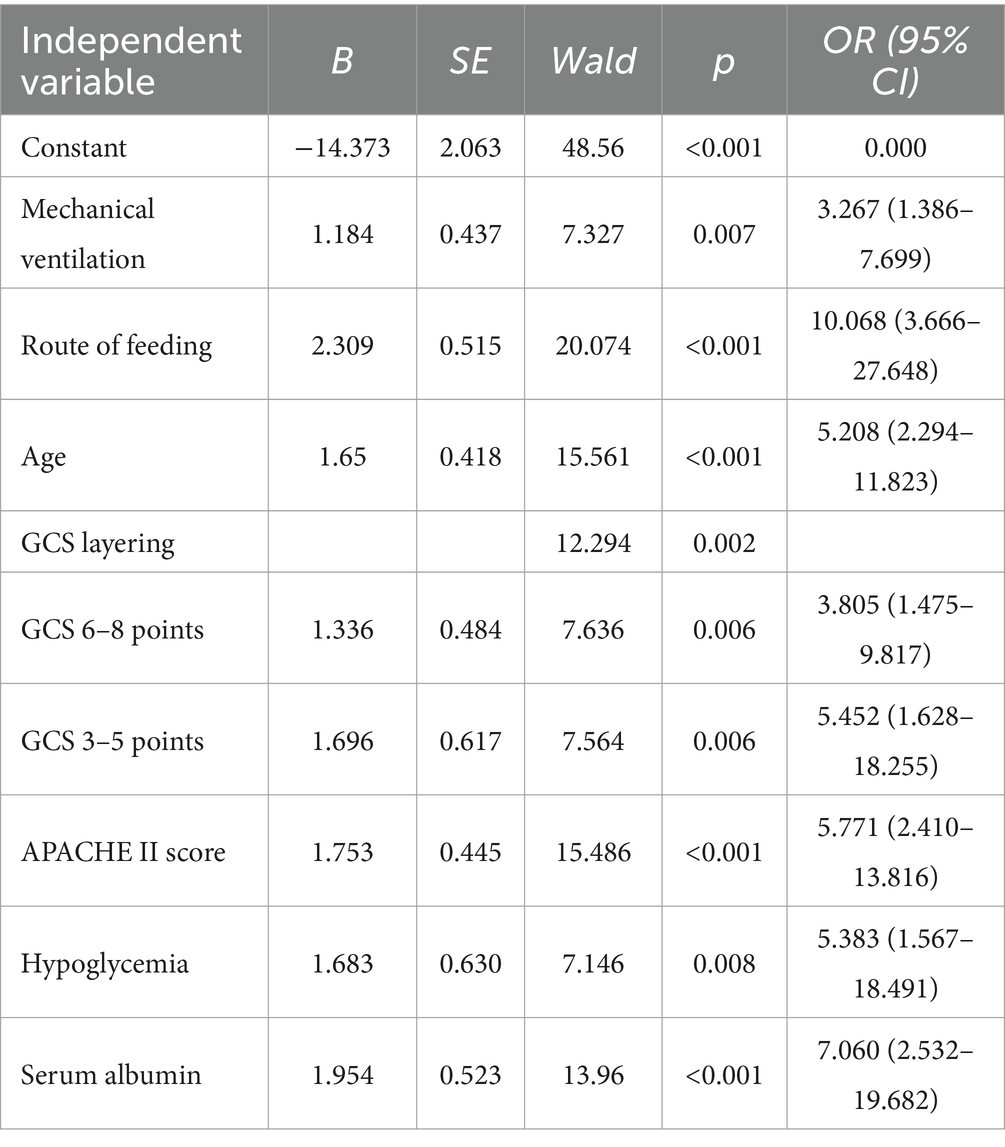
Table 3. Logistic regression analysis of risk factors for developing FI in neurocritical care patients.
3.4 Development of model
The partial regression coefficients of the FI independent predictors determined based on the binary logistic regression analysis were used to develop the model. The fitted regression equation of the FI risk prediction model for neurocritical patients is as follows: Logit p = = − 14.737 + 1.184 × mechanical ventilation+2.309 × route of feeding +1.650 × age+1.336 × GCS tertile (6–8 points) + 1.696 × GCS tertile (3–5 points) + 1.753 × APACHE II scores+1.683 × blood glucose value+1.954 × serum albumin.
3.5 Validation of model
The goodness of fit of the model was tested using the H-L test, yielding a χ2 value of 9.622 with P = 0.293(> 0.05). This indicates that the predictive ability of the FI risk model closely aligns with the actual incidence rate, demonstrating good compliance. The ROC curve was plotted to calculate the AUC, resulting in an AUC of 0.941 with a 95% CI of 0.912–0.970, p < 0.001. When the optimal risk cutoff value was set at 0.767%, the sensitivity of the model was 85.9% and the specificity of the model was 90.8%. At this point, the Youden index reached its maximum at 0.715, signifying that the constructed FI prediction model had a better ability to discriminate whether FI occurs or not. See Figure 2 for further details.
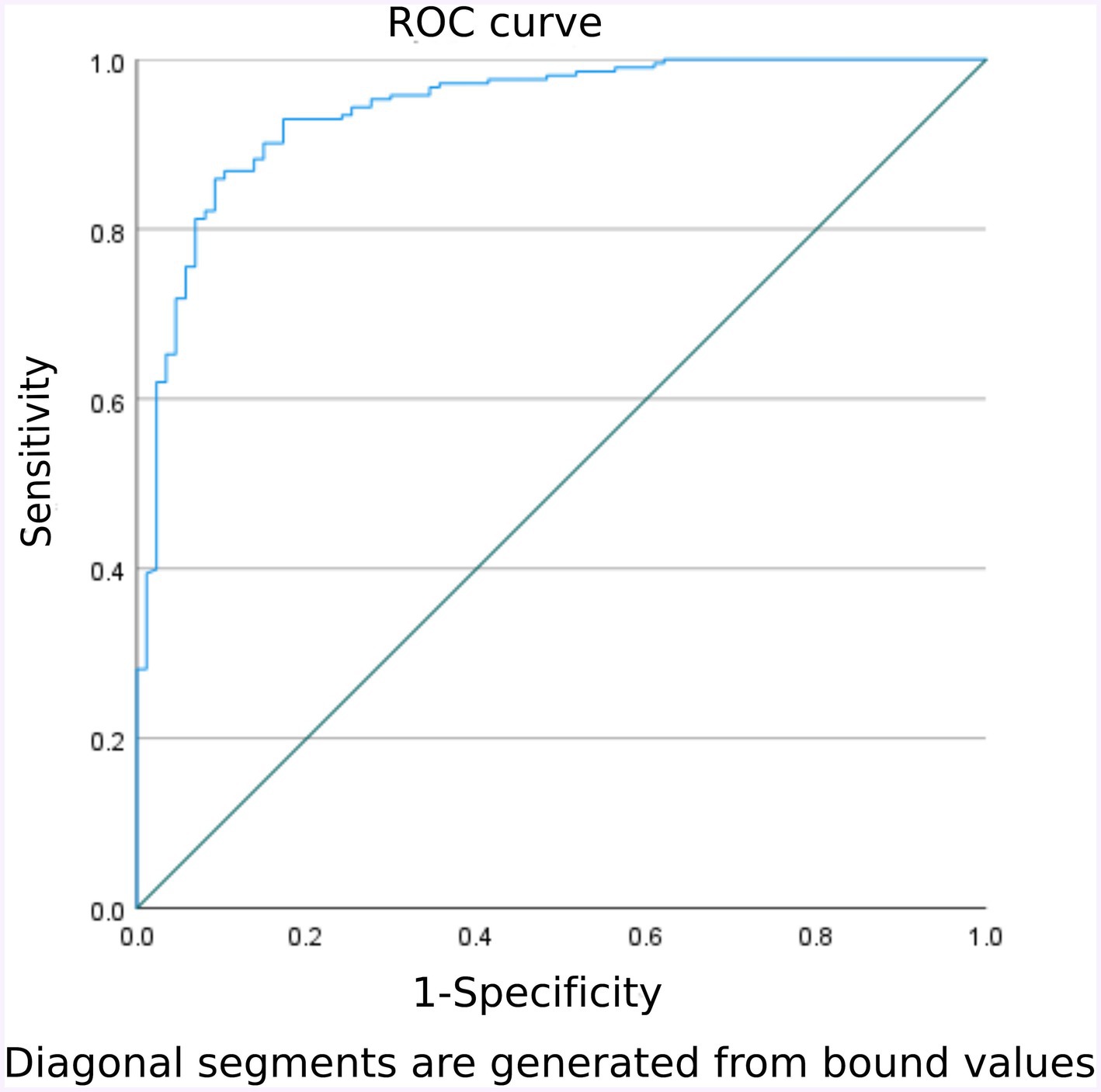
Figure 2. Validation group model predicts ROC curves for early enteral nutrition FI in NCU patients.
4 Discussion
In this study, the incidence of FI was found to be 71%, which is a high rate and aligns with previous research conducted by Pinto et al. (15) and Zhu et al. (12). After brain injuries in NCU patients, under the interactive influence of brain–gut axis, the inhibited vagus nerve weakens the patients’ gastrointestinal motility, which is often manifested by symptoms such as abdominal distension, constipation, and high GRV clinically (35, 36). Simultaneously, under the stress response of the body, there is a heightened risk of acute damage to the intestinal mucosal barrier (37, 38), and this condition facilitates the entry of bacteria, endotoxin, and intestinal cytokines into the bloodstream, further aggravating gastrointestinal dysfunction (39). Additionally, due to insufficient organ perfusion, gastrointestinal edema, and a significant decrease in intestinal absorptive capacity (40, 41), patients become more susceptible to clinical manifestations of FI, such as vomiting and diarrhea. Therefore, from a pathophysiological perspective, NCU patients are more prone to FI compared to other patients. Clinical nurses should pay attention to FI and take proactive measures to reduce its incidence.
The current study revealed that age ≥ 60 years is an independent risk factor for FI during early enteral nutrition in NCU patients, which is in agreement with the findings of Tao et al. (42). It may be related to the pathophysiological mechanism of the human body. As age advances, intestinal function tends to decline and undergo atrophy, accompanied by a reduction in digestive function and absorption capacity. Consequently, this phenomenon increases the risk of FI.
According to the definition associated with the GCS score, when the GCS score is less than 8, the patient is considered to be in a comatose state (i.e., severely impaired in consciousness), of which a score of 3–5 indicates a deep coma, while a score of 6–8 indicates a light coma. The study found that a lower GCS score and a higher APACHE II score are associated with an increased risk of FI, which is consistent with the findings of Li et al. (43) and Yang et al. (44). As early as 2011, Chen et al. (45) pointed out the heightened risk of FI in patients with neurological disorders. Lower GCS scores signal more severe brain injuries, resulting in increased intestinal ischemia and edema (46). Conversely, higher APACHE II scores indicate a greater severity of the patient’s condition. At such levels, the body’s stress response is increased, and the systemic inflammatory response leads to increased protein consumption, intestinal dysbiosis, intestinal mucosal barrier damage, and reduced gastrointestinal tolerance, especially for patients with an APACHE II score of >20. Their monitoring should be strengthened (47), and healthcare providers should also be vigilant regarding the potential occurrence of FI in these patients.
The risk of FI in this study was greater in NCU patients who were mechanically ventilated during early enteral nutrition, which is in line with the results of several studies (21, 48). The findings from studies by Blaser et al. (19) and Reintam et al. (49) indicated that the risk of FI in mechanically ventilated patients was as high as 80.2 to 85%. Mechanical ventilation can impair the function of the lower esophageal sphincter and cause gastroesophageal reflux. The endotracheal tube cuff compresses the esophagus, which can result in pharyngeal muscle-wasting atrophy (49). Mechanical ventilation also increases patients’ intrathoracic and intra-abdominal pressures, particularly with prolonged use of high levels of positive end-expiratory pressure (PEEP). This can lead to a decrease in cardiac output and peripheral organ perfusion, resulting in a reduced blood supply to the gastrointestinal tract, and ultimately increasing the risk of FI. Therefore, it is essential to closely monitor mechanically ventilated patients, especially those who use high levels of PEEP, strengthen the assessment of the patient’s respiratory status, and stop the mechanical ventilation therapy as soon as possible.
This study showed that the route of enteral feeding was also one of the independent risk factors for FI in NCU patients. Patients with neurological impairment were at a high risk of aspiration, often accompanied by increased intracranial pressure, and prone to choking and vomiting (50). For NCU patients, tube feeding is often required due to diminished state of consciousness and the use of mechanical ventilation (1). Guidelines recommend that patients requiring tube feeding should opt for nasogastric tube feeding. For those at a high risk of aspiration or with elevated gastric residual volume, post-pyloric feeding, preferably through the nasoenteric tube, is recommended (51). In 2021, a meta-analysis revealed that (52) post-pyloric feeding can significantly reduce the incidence of gastrointestinal complications such as reflux, vomiting, diarrhea, bloating, gastric remnant, and constipation. Subsequently, in 2022, the Chinese Nursing Association (CNA) issued an expert consensus on enteral nutrition for NCU patients (27). It is recommended that for NCU patients, if the GRV is >100 mL and does not improve after 48 h of adjusting the infusion rate or using gastric prokinetic drugs, the placement of a nasoenteric tube is advised.
The results of this study showed that hyperglycemia before feeding would increase the risk of FI. Hyperglycemia reflexively reduces gastric tone and exacerbates gastric retention, but it also accelerates the pyloric activity, contributing to uncoordinated gastroduodenal contractions and impaired gastric emptying (8), which are also consistent with the results of Nguyen et al.’s study (53). For NCU patients, blood glucose levels are also an important factor affecting disease prognosis (54). According to critical patient blood glucose management guidelines (55), controlling blood glucose levels at approximately 7.8–10.0 mmol/L is relatively safe. However, there are various factors affecting patients’ blood glucose levels. Therefore, personalized blood glucose management according to patients’ different disease conditions, including the selection of medication and enteral nutritional preparations, is essential in clinical practice. It will be the focus of the blood glucose management of NCU patients in the future.
In this study, patients with low levels of serum albumin were also identified as being at risk for FI. Serum albumin serves many vital physiological functions, such as maintaining cellular osmotic pressure, scavenging oxygen-free radicals, and transporting fatty acids and cholesterol (56). When hypoproteinemia occurs, osmotic pressure changes and gastrointestinal mucosal edema increases the incidence of FI (21, 57). Dynamic monitoring in clinical settings should be strengthened, and timely supplementation is necessary when hypoalbuminemia occurs to reduce the incidence of FI.
In this study, the univariate analysis of sedation showed statistical significance. However, the results of the multivariate analysis showed that sedation was not an independent risk factor for FI in patients. Sedative drugs inhibit the release of excitatory neurotransmitters, leading to slower bowel movements. Previous studies (58) have proven that higher doses of sedatives are associated with more severe gastrointestinal dyskinesia. It is possible that there may be a difference between the results derived from unifactorial and multifactorial analyses in the prediction model, and the reasons for this difference could be analyzed in relation to the specific type of drug used, the dose used, and the duration of its use. Future studies can further focus on the effects of the above drugs on patients’ FI. In addition, some predictors, such as specific gastrointestinal biomarkers, or more granular clinical factors (e.g., inflammation markers), were not included in the model in the present study, considering their clinical utility as well as measurement accuracy. These aspects can be further explored in future studies.
The area under the ROC curve of this model was 0.941, with a 95% CI of 0.912–0.970, and the H-L goodness-of-fit resulted in a p-value of 0.293 (>0.05), indicating that the model had a good ability to predict whether FI occurred in practice. In clinical application, the area under the ROC curve was 0.924, with a 95% CI of 0.878–0.970 (p < 0.001); when the optimal cut-off value was set at 46.3%, the sensitivity of the model was 96.0%, the specificity was 74.4%, and the Youden index was 0.704, indicating that the model facilitates healthcare professionals to efficiently and accurately identify the high-risk group of FI among enteral nutrition patients in NCU. Thus, positive and effective measures can be implemented for this high-risk group. These measures include, but are not limited to, the rational selection of feeding routes for enteral nutrition, the establishment of a scientific, standardized implementation plan for enteral nutrition, the appropriate use of pro-gastric motivational drugs, the use of probiotics, the addition of soluble dietary fiber, early rehabilitation of critically ill patients, and traditional Chinese medicine therapies such as massage. In conclusion, early identification and early intervention are crucial steps in reducing the risk of FI.
5 Limitations
This study had some limitations. First, the data used to develop the prediction model were drawn exclusively from patients in a single tertiary hospital, which may limit the representativeness of the sample and suggest that the results require further validation. Second, the study did not explore important clinical outcomes such as ICU length of stay or mortality, which could be areas for future research. Finally, while we conducted internal validation of the model, external validation was not performed. Thus, the predictive effectiveness of the model should be continuously validated in future studies to enable broader generalization.
6 Conclusion
The incidence of FI in NCU patients was notably high, with independent risk factors identified as age, GCS scores, APACHE II scores, feeding route, mechanical ventilation, hypoglycemia, and serum albumin levels. Therefore, early screening for FI risk in these patients may be particularly important to reduce its occurrence. This study developed a predictive model for FI risk in NCU patients, demonstrating strong predictive performance and clinical utility. The model may be used as an objective and convenient screening tool for the early identification of high-risk patients, enabling the implementation of appropriate interventions to mitigate FI occurrence and improve patient outcomes.
Data availability statement
The original contributions presented in the study are included in the article/supplementary material, further inquiries can be directed to the corresponding authors.
Ethics statement
The studies involving humans were approved by the “Apricot Grove Scholars” Discipline Talent Research Enhancement Program (YYZX2022061). The studies were conducted in accordance with the local legislation and institutional requirements. The participants provided their written informed consent to participate in this study.
Author contributions
RY: Writing – review & editing, Writing – original draft, Methodology, Data curation, Conceptualization. LL: Writing – review & editing, Writing – original draft, Methodology, Data curation. JM: Writing – review & editing, Writing – original draft, Data curation. XL: Writing – review & editing, Writing – original draft, Formal analysis. FY: Writing – review & editing, Writing – original draft, Formal analysis. S-FM: Writing – review & editing, Writing – original draft, Formal analysis.
Funding
The author(s) declare that financial support was received for the research, authorship, and/or publication of this article. This study was supported by the “Apricot Grove Scholars” Discipline Talent Research Enhancement Program (YYZX2022061).
Conflict of interest
The authors declare that the research was conducted in the absence of any commercial or financial relationships that could be construed as a potential conflict of interest.
Publisher’s note
All claims expressed in this article are solely those of the authors and do not necessarily represent those of their affiliated organizations, or those of the publisher, the editors and the reviewers. Any product that may be evaluated in this article, or claim that may be made by its manufacturer, is not guaranteed or endorsed by the publisher.
References
1. McClave, SA, Martindale, RG, Vanek, VW, McCarthy, M, Roberts, P, Taylor, B, et al. Guidelines for the provision and assessment of nutrition support therapy in the adult critically ill patient: Society of Critical Care Medicine (SCCM) and American Society for Parenteral and Enteral Nutrition (a.S.P.E.N.). JPEN J Parenter Enteral Nutr. (2009) 33:277–316. doi: 10.1177/0148607109335234
2. Martindale, RG, and Warren, M. Should enteral nutrition be started in the first week of critical illness? Curr Opin Clin Nutr Metab Care. (2015) 18:202–6. doi: 10.1097/mco.0000000000000146
3. Mandell, SP, and Gibran, NS. Early enteral nutrition for burn injury. Adv Wound Care (New Rochelle). (2014) 3:64–70. doi: 10.1089/wound.2012.0382
4. Berger, MM . The 2013 Arvid Wretlind lecture: evolving concepts in parenteral nutrition. Clin Nutr. (2014) 33:563–70. doi: 10.1016/j.clnu.2014.03.005
5. Li, J . The rationality of preferred enteral nutrition. Parenteral and enteral nutrition. (2013) 20:321–3. doi: 10.16151/j.1007-810x.2013.06.002
6. Wang, Z, Ding, W, Fang, Q, Zhang, L, Liu, X, and Tang, Z. Effects of not monitoring gastric residual volume in intensive care patients: a meta-analysis. Int J Nurs Stud. (2019) 91:86–93. doi: 10.1016/j.ijnurstu.2018.11.005
7. Singer, P, Blaser, AR, Berger, MM, Alhazzani, W, Calder, PC, Casaer, MP, et al. ESPEN guideline on clinical nutrition in the intensive care unit. Clin Nutr. (2019) 38:48–79. doi: 10.1016/j.clnu.2018.08.037
8. Liu, H, Mi, Y, and Huang, P. Research progress on feeding intolerance of enteral nutrition in critically ill patients. J Adv Nurs. (2021) 36:333–8. doi: 10.16821/j.cnki.hsjx.2021.04.006
9. Qiu, C, Chen, C, Zhang, W, Kou, Q, Wu, S, Zhou, L, et al. Fat-modified enteral formula improves feeding tolerance in critically ill patients: a multicenter, single-blind, randomized controlled trial. JPEN J Parenter Enteral Nutr. (2017) 41:785–95. doi: 10.1177/0148607115601858
10. Wang, K, McIlroy, K, Plank, LD, Petrov, MS, and Windsor, JA. Prevalence, outcomes, and Management of Enteral Tube Feeding Intolerance: a retrospective cohort study in a tertiary center. JPEN J Parenter Enteral Nutr. (2017) 41:959–67. doi: 10.1177/0148607115627142
11. Cheng, W, Meishan, L, and Guo, H. Research progress of early enteral nutrition feeding intolerance in critically ill patients. Chin J Nurs. (2017) 52:98–102. doi: 10.3969/j.issn.1672-1756.2018.03.017
12. Yuanxia Zhu, YZ, and Yancai,. Correlation between intra-abdominal pressure and enteral nutritional feeding intolerance in patients with mechanical ventilation in ICU. Nurs Prac Res. (2021) 18:175–8. doi: 10.3969/j.issn.1672-9676.2021.02.005
13. Moheet, AM, Livesay, SL, Abdelhak, T, Bleck, TP, Human, T, Karanjia, N, et al. Standards for neurologic critical care units: a statement for healthcare professionals from the Neurocritical care society. Neurocrit Care. (2018) 29:145–60. doi: 10.1007/s12028-018-0601-1
14. Peng, J, Liu, GW, Li, F, Yuan, M, Xiang, Y, and Qin, D. The correlation between feeding intolerance and poor prognosis of patients with severe neurological conditions: a case-control study. Expert Rev Neurother. (2019) 19:1265–70. doi: 10.1080/14737175.2019.1679627
15. Pinto, TF, Rocha, R, Paula, CA, and de Jesus, RP. Tolerance to enteral nutrition therapy in traumatic brain injury patients. Brain Inj. (2012) 26:1113–7. doi: 10.3109/02699052.2012.666369
16. Lew, CCH, Yandell, R, Fraser, RJL, Chua, AP, Chong, MFF, and Miller, M. Association between malnutrition and clinical outcomes in the intensive care unit: a systematic review [formula: see text]. JPEN J Parenter Enteral Nutr. (2017) 41:744–58. doi: 10.1177/0148607115625638
17. Deane, AM, Chapman, MJ, Reintam Blaser, A, McClave, SA, and Emmanuel, A. Pathophysiology and treatment of gastrointestinal motility disorders in the acutely ill. Nutr Clin Pract. (2019) 34:23–36. doi: 10.1002/ncp.10199
18. Mentec, H, Dupont, H, Bocchetti, M, Cani, P, Ponche, F, and Bleichner, G. Upper digestive intolerance during enteral nutrition in critically ill patients: frequency, risk factors, and complications. Crit Care Med. (2001) 29:1955–61. doi: 10.1097/00003246-200110000-00018
19. Blaser, AR, Starkopf, J, Kirsimägi, Ü, and Deane, AM. Definition, prevalence, and outcome of feeding intolerance in intensive care: a systematic review and meta-analysis. Acta Anaesthesiol Scand. (2014) 58:914–22. doi: 10.1111/aas.12302
20. Reintam Blaser, A, Malbrain, ML, Starkopf, J, Fruhwald, S, Jakob, SM, De Waele, J, et al. Gastrointestinal function in intensive care patients: terminology, definitions and management. Recommendations of the ESICM working group on abdominal problems. Intensive Care Med. (2012) 38:384–94. doi: 10.1007/s00134-011-2459-y
21. Qingnan Zhang, XQ, and Chen, J. Influencing factors and countermeasures of enteral feeding intolerance in critically ill patients. Chin J Gerontol. (2019) 39:4516–8. doi: 10.3969/j.issn.1005-9202.2019.18.047
22. Chuang Chen, JX, and Liu, S. A. Study on the monitor of gastric residual volume by ultrasound its prediction on feeding intolerance during enteral nutrition in critically ill patients in intensive care unit. Chinese J Emergency Med. (2020) 29:1291–5. doi: 10.3760/cma.j.issn.1671-0282.2020.10.005
23. Jin Hou, AG . Advances in bedside ultrasound assessment of gastric residual volume in critically ill, enterally fed patients. J Nurs Sci. (2021) 36:101–4. doi: 10.3870/j.issn.1001-4152.2021.02.101
24. Bejarano, N, Navarro, S, Rebasa, P, García-Esquirol, O, and Hermoso, J. Intra-abdominal pressure as a prognostic factor for tolerance of enteral nutrition in critical patients. JPEN J Parenter Enteral Nutr. (2013) 37:352–60. doi: 10.1177/0148607112464238
25. Sekino, M, Funaoka, H, Sato, S, Okada, K, Inoue, H, Yano, R, et al. Intestinal fatty acid-binding protein level as a predictor of 28-day mortality and bowel ischemia in patients with septic shock: a preliminary study. J Crit Care. (2017) 42:92–100. doi: 10.1016/j.jcrc.2017.07.012
26. Mi, Y, Huang, H, Shang, Y, Shao, X, Huang, P, Xiang, C, et al. Expert consensus on prevention and management of enteral nutrition therapy complications for critically ill patients in China (2021 edition). Zhonghua Wei Zhong Bing Ji Jiu Yi Xue. (2021) 33:903–17. doi: 10.3760/cma.j.cn121430-20210310-00357
27. Liu, F, Gao, L, and Wang, X. Expert consensus on enteral feeding nursing for patients with severe neurological diseases. Chin J Nurs. (2022) 57:261–4. doi: 10.3761/j.issn.0254-1769.2022.03.001
28. West China Evidence-based Nursing Center SU, Nursing Management Committee of Chinese Nursing Association, Neurosurgery Branch of Chinese Medical Association . Nursing practice guideline for enteral nutrition in patients with stroke. Chin J Evid Based Med. (2021) 21:628–41. doi: 10.7507/1672-2531.202101115
29. Shi, HY, Liu, AH, Ma, X, Wang, X, Zhang, LJ, and Ma, YL. Interpretation of the group standard “Retention and maintenance of nasoenteric tubes in adults. Chin J Acute Crit Care Nurs. (2023) 4:1011–5. doi: 10.3761/j.issn.2096-7446.2023.11.009
30. Weir, CB, and Jan, A. BMI classification percentile and cut off points In: StatPearls. Treasure Island (FL): StatPearls Publishing (2024)
31. Teasdale, G, and Jennett, B. Assessment of coma and impaired consciousness. A practical scale. Lancet. (1974) 304:81–4. doi: 10.1016/s0140-6736(74)91639-0
32. Kondrup, J, Rasmussen, HH, Hamberg, O, and Stanga, Z. Nutritional risk screening (NRS 2002): a new method based on an analysis of controlled clinical trials. Clin Nutr. (2003) 22:321–36. doi: 10.1016/s0261-5614(02)00214-5
33. Knaus, WA, Draper, EA, Wagner, DP, and Zimmerman, JE. APACHE II: a severity of disease classification system. Crit Care Med. (1985) 13:818–29. doi: 10.1097/00003246-198510000-00009
34. Gao, YX, and Zhang, JX. The sample size of logistic regression analysis was determined. Evid Based Med. (2018) 18:122–4. doi: 10.12019/j.issn.1671-5144.2018.02.015
35. Durgan, DJ, Lee, J, McCullough, LD, and Bryan, RM Jr. Examining the role of the microbiota-gut-brain Axis in stroke. Stroke. (2019) 50:2270–7. doi: 10.1161/strokeaha.119.025140
36. Liu, Y, Dong, J, Zhang, Z, Liu, Y, and Wang, Y. How brain infarction links with the microbiota-gut-brain Axis: hints from studies focusing on the risk factors for ischemic stroke. Front Neurosci. (2022) 16:877937. doi: 10.3389/fnins.2022.877937
37. Gwak, MG, and Chang, SY. Gut-brain connection: microbiome, gut barrier, and environmental sensors. Immune Netw. (2021) 21:e20. doi: 10.4110/in.2021.21.e20
38. Zhu, KJ, Huang, H, Chu, H, Yu, H, and Zhang, SM. Alterations in enterocyte mitochondrial respiratory function and enzyme activities in gastrointestinal dysfunction following brain injury. World J Gastroenterol. (2014) 20:9585–91. doi: 10.3748/wjg.v20.i28.9585
39. Wang, T. Development of risk assessment scale for enteral feeding intolerance in critically Ill patients. Third Military Medical University. (2016).
40. Btaiche, IF, Chan, LN, Pleva, M, and Kraft, MD. Critical illness, gastrointestinal complications, and medication therapy during enteral feeding in critically ill adult patients. Nutr Clin Pract. (2010) 25:32–49. doi: 10.1177/0884533609357565
41. Yu, XY, Yin, HH, and Zhu, JC. Increased gut absorptive capacity in rats with severe head injury after feeding with probiotics. Nutrition. (2011) 27:100–7. doi: 10.1016/j.nut.2010.01.010
42. Tao, W . Clinical characteristics and risk factors of feeding intolerance in neurosurgery patients with nasal feeding. J Adv Nurs. (2018) 33:910–2. doi: 10.16821/j.cnki.hsjx.2018.10.014
43. Lei, L, Shen, MS, Ling, F, Xu, Y, and Mao, YQ. Multivariate analysis of early enteral nutritional intolerance in patients with severe craniocerebral trauma. J Adv Nurs. (2012) 27:1832–5. doi: 10.16821/j.cnki.hsjx.2012.20.004
44. Yang, BY, Li, LT, Li, YL, and Wang, Y. Investigation of enteral nutrition feeding intolerance in ICU patients and analysis of influencing factors. J Hubei Medical University. (2019) 38:595–8. doi: 10.13819/j.issn.2096-708x.2019.06.019
45. Chen, YD, Li, SJ, Sun, FH, Liu, YY, and Hu, WL. Monitoring of medical complications after acute ischemic stroke in a neurological intensive care unit. Eur Neurol. (2011) 66:204–9. doi: 10.1159/000330551
46. Duan, H, Hao, C, Fan, Y, Wang, H, Liu, Y, Hao, J, et al. The role of neuropeptide Y and aquaporin 4 in the pathogenesis of intestinal dysfunction caused by traumatic brain injury. J Surg Res. (2013) 184:1006–12. doi: 10.1016/j.jss.2013.03.096
47. Wei, Z, Jiang, HJ, Jiang, XG, Li, KK, Wu, YD, and Zhang, LY. Meta-analysis of risk factors of enteral feeding intolerance in critically ill patients. Parenteral and enteral nutrition. (2020) 27:313–20. doi: 10.16151/j.1007-810x.2020.05.013
48. Ren, S, He, C, and Li, Y. Analysis of influencing factors of enteral nutritional intolerance in patients with sepsis. Parenteral and enteral nutrition. (2018) 25:355–8. doi: 10.16151/j.1007-810x.2018.11.008
49. Reintam Blaser, A, Parm, P, Kitus, R, and Starkopf, J. Intra-abdominal hypertension and gastrointestinal symptoms in mechanically ventilated patients. Crit Care Res Pract. (2011) 2011:982507. doi: 10.1155/2011/982507
50. Neurotrauma Specialty Group of the Chinese Medical Association Branch of Traumatology . Chinese expert consensus on enteral nutrition management process for Craniocerebral trauma patients. Chinese J Trauma. (2019) 35:193–8. doi: 10.3760/cma.j.issn.1001-8050.2019.03.001
51. Wang, D, Zheng, SQ, Chen, XC, Jiang, SW, and Chen, HB. Comparisons between small intestinal and gastric feeding in severe traumatic brain injury: a systematic review and meta-analysis of randomized controlled trials. J Neurosurg. (2015) 123:1194–201. doi: 10.3171/2014.11.Jns141109
52. Liu, Y, Wang, Y, Zhang, B, Wang, J, Sun, L, and Xiao, Q. Gastric-tube versus post-pyloric feeding in critical patients: a systematic review and meta-analysis of pulmonary aspiration- and nutrition-related outcomes. Eur J Clin Nutr. (2021) 75:1337–48. doi: 10.1038/s41430-021-00860-2
53. Nguyen, N, Ching, K, Fraser, R, Chapman, M, and Holloway, R. The relationship between blood glucose control and intolerance to enteral feeding during critical illness. Intensive Care Med. (2007) 33:2085–92. doi: 10.1007/s00134-007-0869-7
54. Yuan, T, He, H, Liu, Y, Wang, J, Kang, X, Fu, G, et al. Association between blood glucose levels and Glasgow outcome score in patients with traumatic brain injury: secondary analysis of a randomized trial. Trials. (2022) 23:38. doi: 10.1186/s13063-022-06005-5
55. Song, XM, Cao, M, Xiang, LJ, Wang, Y, and Zhang, XM. Blood glucose management in critically ill patients. Clinical meta-analysis. (2022) 37:66–71. doi: 10.3969/j.issn.1004-583X.2022.01.013
56. Kim, S, McClave, SA, Martindale, RG, Miller, KR, and Hurt, RT. Hypoalbuminemia and clinical outcomes: what is the mechanism behind the relationship? Am Surg. (2017) 83:1220–7. doi: 10.1177/000313481708301123
57. Cai, ZF, Gu, J, and Zhao, P. Analysis of risk factors of enteral feeding intolerance in patients with severe acute pancreatitis. Modern Prevent Med. (2018) 45:4403–7.
Keywords: neurocritical, feeding intolerance, risk factors, prediction model, enteral nutrition
Citation: Yuan R, Liu L, Mi J, Li X, Yang F and Mao S (2024) Development and validation of a risk prediction model for feeding intolerance in neurocritical patients with enteral nutrition. Front. Nutr. 11:1481279. doi: 10.3389/fnut.2024.1481279
Edited by:
Arved Weimann, St. Georg Hospital, GermanyReviewed by:
Jae Gil Lee, Ewha Womans Medical Center, Republic of KoreaZhang Haoling, University of Science Malaysia (USM), Malaysia
Gagandeep Dhillon, University of Maryland, Baltimore, United States
Copyright © 2024 Yuan, Liu, Mi, Li, Yang and Mao. This is an open-access article distributed under the terms of the Creative Commons Attribution License (CC BY). The use, distribution or reproduction in other forums is permitted, provided the original author(s) and the copyright owner(s) are credited and that the original publication in this journal is cited, in accordance with accepted academic practice. No use, distribution or reproduction is permitted which does not comply with these terms.
*Correspondence: Shifang Mao, MTE3MjA1ODUzNEBxcS5jb20=; Fang Yang, MzI5ODcxNTgwQHFxLmNvbQ==