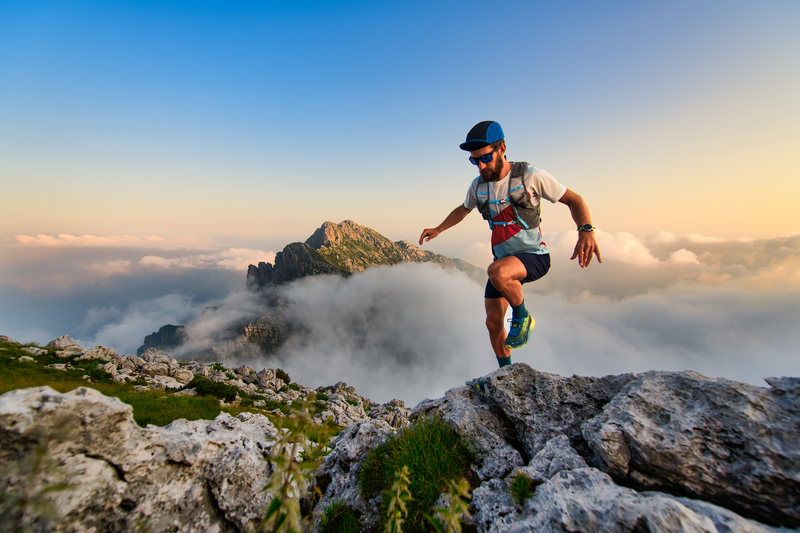
94% of researchers rate our articles as excellent or good
Learn more about the work of our research integrity team to safeguard the quality of each article we publish.
Find out more
ORIGINAL RESEARCH article
Front. Neurorobot.
Volume 19 - 2025 | doi: 10.3389/fnbot.2025.1544694
This article is part of the Research Topic Advancements in Neural Learning Control for Enhanced Multi-Robot Coordination View all 3 articles
The final, formatted version of the article will be published soon.
You have multiple emails registered with Frontiers:
Please enter your email address:
If you already have an account, please login
You don't have a Frontiers account ? You can register here
Foundation pit deformation prediction is a critical aspect of underground engineering safety assessment, influencing construction quality and personnel safety. However, due to complex geological conditions and numerous environmental interference factors, traditional prediction methods struggle to achieve precise modeling. Conventional approaches, including numerical simulations, empirical formulas, and machine learning models, suffer from limitations such as high computational costs, poor generalization, or excessive dependence on specific data distributions.Recently, deep learning models, particularly cross-modal architectures, have demonstrated great potential in engineering applications. However, effectively integrating multi-modal data for improved prediction accuracy remains a significant challenge. This study proposes a Multi-Scale CLIP (Contrastive Language-Image Pretraining) framework, ModuCLIP, designed for foundation pit deformation prediction in multi-modal robotic systems. The framework leverages a self-supervised contrastive learning mechanism to integrate multi-source information, including images, textual descriptions, and sensor data, while employing a multi-scale feature learning approach to enhance adaptability to complex conditions. Experiments conducted on multiple foundation pit engineering datasets demonstrate that ModuCLIP outperforms existing methods in terms of prediction accuracy, generalization, and robustness. The findings suggest that this framework provides an efficient and precise solution for foundation pit deformation prediction while offering new insights into multi-modal robotic perception and engineering monitoring applications.
Keywords: Foundation Pit Deformation Prediction, Multi-Modal Robotics, Contrastive learning, Multi-scale features, deep learning
Received: 13 Dec 2024; Accepted: 17 Feb 2025.
Copyright: © 2025 Bo. This is an open-access article distributed under the terms of the Creative Commons Attribution License (CC BY). The use, distribution or reproduction in other forums is permitted, provided the original author(s) or licensor are credited and that the original publication in this journal is cited, in accordance with accepted academic practice. No use, distribution or reproduction is permitted which does not comply with these terms.
* Correspondence:
Linwen Bo, Gansu Industrial Vocational and Technical College, Tianshui, China
Disclaimer: All claims expressed in this article are solely those of the authors and do not necessarily represent those of their affiliated organizations, or those of the publisher, the editors and the reviewers. Any product that may be evaluated in this article or claim that may be made by its manufacturer is not guaranteed or endorsed by the publisher.
Research integrity at Frontiers
Learn more about the work of our research integrity team to safeguard the quality of each article we publish.