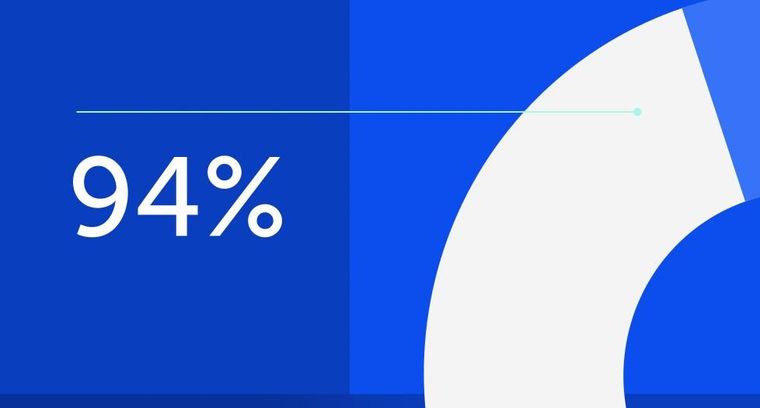
94% of researchers rate our articles as excellent or good
Learn more about the work of our research integrity team to safeguard the quality of each article we publish.
Find out more
ORIGINAL RESEARCH article
Front. Earth Sci.
Sec. Geohazards and Georisks
Volume 13 - 2025 | doi: 10.3389/feart.2025.1543271
This article is part of the Research TopicRecent Advances in Image Fusion and Quality Improvement for Cyber-Physical Systems, Volume IIIView all 9 articles
The final, formatted version of the article will be published soon.
Select one of your emails
You have multiple emails registered with Frontiers:
Notify me on publication
Please enter your email address:
If you already have an account, please login
You don't have a Frontiers account ? You can register here
Karst collapse monitoring is challenging due to data sparsity, complex underground dynamics, and the need for real-time adaptation. Traditional methods often struggle to provide accurate and timely risk assessments. To address these issues, we propose the Integrated Karst Collapse Prediction Network (IKCPNet), a framework that integrates multi-source imaging, geophysical modeling, and machine learning techniques. IKCPNet processes seismic imaging, hydrological patterns, and environmental factors to predict collapse risks with high precision.The model leverages an advanced data encoding mechanism to fuse diverse inputs and a physics-informed module to capture subsurface changes. Additionally, a dynamic risk assessment strategy enhances decision-making by incorporating real-time feedback and probabilistic mapping.Experimental results show that IKCPNet significantly improves prediction accuracy and risk mitigation. On the OpenSARShip dataset, it achieves an accuracy of 94.34±0.02 and an IoU of 90.23±0.02, outperforming the previous best model by 1.22 and 0.89 points, respectively. These findings highlight the potential of our approach for enhancing geological hazard monitoring through multi-source data integration.
Keywords: Karst collapse, Multi-source image, Segmentation techniques, Cyber-physical Systems, risk prediction
Received: 11 Dec 2024; Accepted: 14 Mar 2025.
Copyright: © 2025 Bo. This is an open-access article distributed under the terms of the Creative Commons Attribution License (CC BY). The use, distribution or reproduction in other forums is permitted, provided the original author(s) or licensor are credited and that the original publication in this journal is cited, in accordance with accepted academic practice. No use, distribution or reproduction is permitted which does not comply with these terms.
* Correspondence: Linwen Bo, Gansu Industrial Vocational and Technical College, Tianshui, China
Disclaimer: All claims expressed in this article are solely those of the authors and do not necessarily represent those of their affiliated organizations, or those of the publisher, the editors and the reviewers. Any product that may be evaluated in this article or claim that may be made by its manufacturer is not guaranteed or endorsed by the publisher.
Supplementary Material
Research integrity at Frontiers
Learn more about the work of our research integrity team to safeguard the quality of each article we publish.