- Neuro-Robotics Laboratory, Department of Robotics, Graduate School of Engineering, Tohoku University, Sendai, Japan
Introduction: When humans grasp an object, they are capable of recognizing its characteristics, such as its stiffness and shape, through the sensation of their hands. They can also determine their level of confidence in the estimated object properties. In this study, we developed a method for multi-fingered hands to estimate both physical and geometric properties, such as the stiffness and shape of an object. Their confidence levels were measured using proprioceptive signals, such as joint angles and velocity.
Method: We have developed a learning framework based on probabilistic inference that does not necessitate hyperparameters to maintain equilibrium between the estimation of diverse types of properties. Using this framework, we have implemented recurrent neural networks that estimate the stiffness and shape of grasped objects with their uncertainty in real time.
Results: We demonstrated that the trained neural networks are capable of representing the confidence level of estimation that includes the degree of uncertainty and task difficulty in the form of variance and entropy.
Discussion: We believe that this approach will contribute to reliable state estimation. Our approach would also be able to combine with flexible object manipulation and probabilistic inference-based decision making.
1 Introduction
Human beings possess a remarkable capacity to discern physical characteristics of grasped objects, such as stiffness and shape, from the sensory signals of the hand with a high degree of freedom. Furthermore, but they are also able to recognize their confidence in the estimated object properties, which they use for manipulation and further exploration. For example, humans are able to recognize the shape of an object by touching it. When the shape is unclear at the first touch, they recognize it and touch the object again to make sure where to grasp. When the grasped object is likely to be soft and fragile, humans would grasp it conservatively based on the degree of uncertainty in the rigidity. These abilities are essential to realize robotic hands that are capable of performing stable grasping and manipulation.
Object properties can be classified into two distinct types of categories: geometric properties and physical properties (Wang et al., 2020). The geometric properties include the position, pose, shape, etc., whereas the physical properties include the mass, stiffness, texture, etc. Estimating both types of properties is essential for proper object manipulation. For example, the geometric properties provide the necessary posture of the hand necessary to grasp the object and maintain its form closure, which is crucial for stable grasping, while the physical properties determine the maximum amount of grasping force the robot can exert without breaking the object. Robots need to know those properties from sensory information unless those properties were given in advance. However, putting multiple various sensors on the hand can increase the cost. Thus, it is preferable to estimate the object properties from proprioceptive information, such as finger joint angles, which can be measured in most cases for joint control.
There are studies regarding the estimation of geometric and physical properties with robotic hands (Wang et al., 2020; Spiers et al., 2016; Gao et al., 2016). However, in contrast to estimation of geometric properties. It has been widely researched that the estimation of physical properties or combining them with geometric-property estimation is not fully researched. There exist numerous methods for estimating physical-property. The estimation of stiffness necessitates the utilization of additional sensors for force measurements (Kicki et al., 2019; Spiers et al., 2016), resulting in an increase in costs and mechanical complexity. Furthermore, it has been observed that stiffness estimation is typically performed solely once at the terminal time step (Bednarek et al., 2021). For adaptive grasping and dexterous manipulation, robotic hands need to estimate both types of object properties in real time in order to adjust grasping force.
When a robotic hand estimates object properties from proprioception in real time, it is important to handle uncertainty as this task requires to estimate from limited information. For example, it is impossible to estimate the objects' properties of objects before contact, which results in the epistemic uncertainty, which is caused by lack of knowledge. Furthermore, observation noises in sensory signals degrade the quality of estimation, resulting in the aleatoric uncertainty, which is due to randomness. Without estimating those uncertainties, robots would make incorrect decisions based on unreliable estimates that could have been avoided with further exploration and conservative actions. Another issue that arises when estimating object is the necessity of weight parameters in the loss function to balance the scales of the multiple properties, which that is a time-consuming process.
To address the above issues, this study introduces a method to estimate both geometric and physical properties using confidence factors, which measure uncertainty. We develop design a learning framework based on probabilistic inference and apply the method to neural networks with a robotic hand without tactile sensors in simulation. In the framework, we utilized a loss function without hyper-parameters and a time-series chunking technique that could improve learning stability. Neural networks are implemented to generate the variance of the estimated stiffness, which value can be regarded as the confidence level of estimation. Although neural networks outputting variance are not novel (Nix and Weigend, 1994), we apply this approach to object-property estimation with a multi-fingered robotic hand and demonstrate its effectiveness to this task. Contributions from of this study are listed below.
1. We have developed a framework that enables for robotic hands to assess stiffness and shape of an object, incorporating their uncertainty.
2. We designed a loss function without hyperparameters in order to balance the scales between different properties.
3. We demonstrate that trained neural networks are capable of estimating the stiffness and the shape by utilizing proprioceptive signals, while also estimating the confidence level of estimation, taking into account and task difficulty, such as variance and entropy.
2 Materials and methods
2.1 Overview
We consider a situation where a robotic hand grasps an object with pre-defined control commands and estimates the object's properties, particularly its stiffness and the shape. The robot hand is capable of measuring joint angles and joint angular velocity, but it does not have visual sensors, tactile sensors, or force sensors. We finally develop neural networks that generate estimates of the object's properties sequentially.
We define mathematical symbols as follows. Let the stiffness be expressed as a scalar value k>0, and let the shape be expressed as a discrete label , where C denotes the number of classes. Furthermore, it is recommended that the joint angles, joint angular velocities, and joint angle commands be designated as q∈ℝD, , and qcmd∈ℝD, respectively, where D denoting the degrees of freedom (DoF). We occasionally refer to observations as for simplicity.
2.2 Training strategy
In many studies, the root mean square errors (RMSEs) are used to estimate continuous values. However, there are a few that make the object property estimation difficult:
1. It is impossible in principle to estimate the properties of an object properties before contact with the object, resulting in the epistemic uncertainty. RMSEs are unable to handle such kind of uncertainty.
2. As neural networks are capable of estimating multiple types of properties with varying units and different representations (e.g., continuous values or discrete labels), such as weight constants are typically required to balance the estimation errors among various types of properties. The cost of designing weight constants increases with the increase in the number of properties.
To address the above issues, we designed the estimation task as a probabilistic inference. Probabilistic inference can naturally express the uncertainty of the object's properties that happens before touch. Furthermore, by considering the task as a likelihood maximization problem, it can be transformed into probabilistic inference of a single joint distribution of multiple properties, which does not have weight constants.
Concretely, the object property estimation task is formulated as the following optimization problem:
Here, N and n indicate the dataset size and the index of samples in the dataset. and indicate the parameters of the probabilistic distributions of the stiffness and the shape, respectively. and can be regarded as estimation results of kn and sn in a statistic way. We then consider its negative log-likelihood as follows:
Thus, the optimization problem can be equivalently converted as follows:
We assume each negative log-likelihood term can be decomposed as follows:
We also model the probabilistic distribution of the stiffness as a Gaussian distribution as follows:
Here, the parameter is expressed as , where μn and indicate the mean and the variance. On the other hand, the probabilistic distribution of the shape is modeled as a discrete distribution obtained through the softmax function as follows:
Here, the parameter is expressed as , where for all c = 1, …, C. The above equation corresponds to the cross-entropy. Hereinafter, we use and .
Finally, the optimization problem is converted as follows:
This can be computed by minimizing the following learning loss:
It is noteworthy that the learning loss L lacks any hyper-parameters, such as weight constants. Instead, σn behaves as a weight balancing the RMSE of the stiffness and the classification errors of the shape CE(sn, zn). Unlike a constant weight, σn itself is to be optimized by the term of logσn, resulting in a statistically optimal value.
2.3 Estimation with neural networks
We develop a neural network architecture that estimates parameters such as and from a time series of observations. A simple approach is to use recurrent neural networks that receive observations y for each time step. However, it may lead to too deep layers in time, which may result in unstable learning, high computational cost, and slow inference. Therefore, we treat a time series of raw observations with a high sampling rate as a time series of chunks with a low sampling rate (Kutsuzawa et al., 2017, 2018). Similar techniques have also been employed in a Transformer-based model for robotic imitation learning (Zhao et al., 2023). Concretely, we transformed a time series (y[0], y[1], …, y[t], …, y[T−1]) into (Υ[0], Υ[1], …, Υ[λ], …, Υ[Λ−1]), where
Here, K∈ℕ indicates the chunk size. Finally, the time-series length of T can be reduced to a shorter length , where ⌈•⌉ indicates the ceiling function. This technique can reduce the time-series length approximately K times shorter, making learning more stable with a lower computational cost. Although it also reduces the estimation frequency, it does not matter in many cases as estimation usually does not require a high update frequency. Although chunking may limit the representation ability of the model, it would be better than down-sampling, which is similar to chunking but decimates the data samples. Thus, it is anticipated that this chunking technique yields more advantages than disadvantages. This technique is graphically shown in Figure 1.
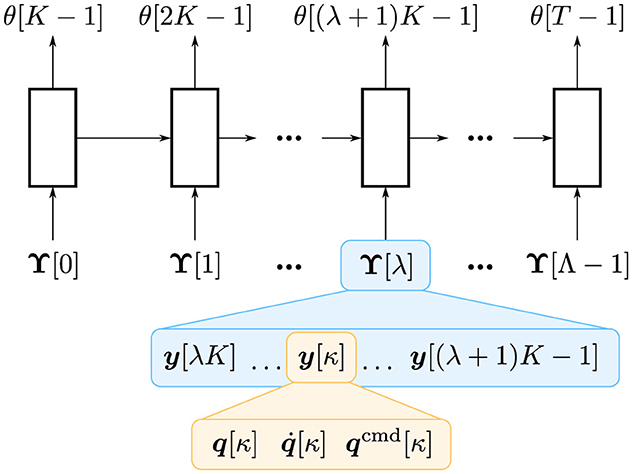
Figure 1. Overview of time-series chunking. A recurrent neural network, illustrated as blocks, receives Υ[λ] that consists of K observations: (y[λK], y[λK+1], …, y[(λ+1)K−1]). θ[t] indicates the estimated parameters of the stiffness and the shape at the t-th time step, i.e., θ[t] = (θk[t], θs[t]).
A neural network generates the values of, μ, logσ2, and z. Here, we use logσ2 instead of σ or σ2 as a primitive term because logσ2 can take −∞ to ∞, making it more manageable for a linear-combination layer. Therefore, in practice, the learning loss defined in Equation 8 is transformed as follows:
2.4 Evaluation setup
We used a MuJoCo (Todorov et al., 2012) implementation of Allegro Hand, implemented by Zakka et al. (2022), as shown in Figure 2. We controlled flexion motion at the joints; 11 degrees of freedom were obtained. The entire process is controlled.
We employed three distinct categories of target objects: cylinder-shaped objects, box-shaped objects, namely sphere-shaped objects, as shown in Figure 3. An object was modeled as a composite of small capsule elements connected by springs each other. The object stiffness was determined by specifying the spring stiffness connecting the elements. Objects were fixed to the space to avoid falling out of the hand. Note that this study focuses on the object property estimation.
For data collection, we controlled the robotic hand with a PD positional controller for 2.2 s with predefined joint angle commands as described in Table 1. For each episode, we recorded a time series of 33-dimensional data that consists of joint angles, joint velocity, and joint angle commands. The observation was measured. The delay is set to 1 ms. Examples of data are shown in Figure 4.
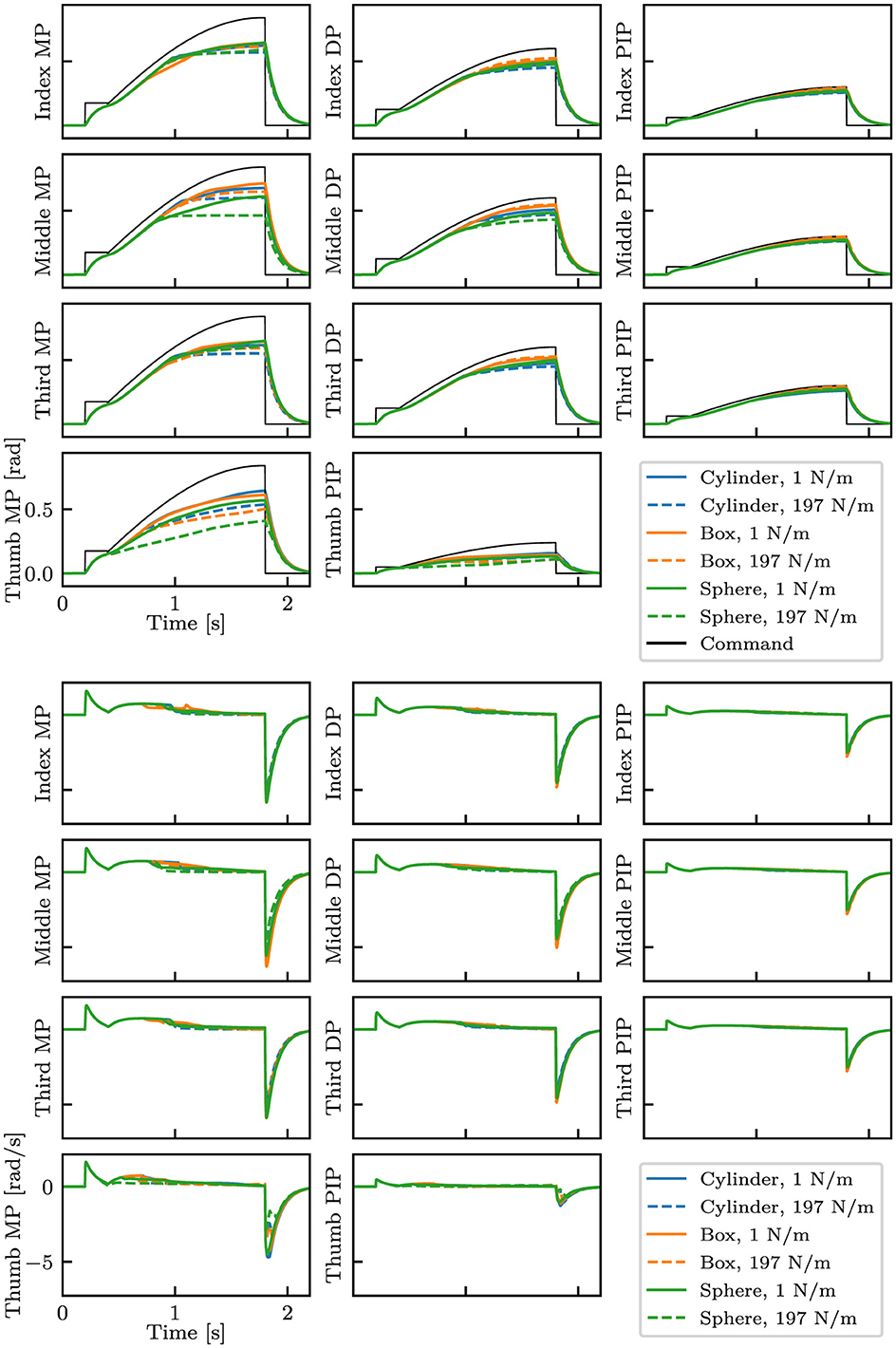
Figure 4. Examples of collected data with different shapes and stiffness. The top eleven plots show the finger joint angles and the commands, whereas the bottom eleven plots show the angular velocity of the joints. Three types of objects with the smallest (1 N/m) and highest (197 N/m) stiffness are shown.
We collected data with the three types of objects, while varying the stiffness with the range from 1 to 197 N/m in 4 N/m increments and varying the position and the orientation in 10 random values in the range of ±5 mm and ±5 deg, respectively; 1,500 data were collected in total. We call this dataset the standard dataset; we use this dataset for training unless otherwise specified. Seventy percentage of the dataset was used for training, and the remaining 30% were used for validation. During the course of training, we introduced Gaussian noises to q and , while varying σ as for each mini-batch; here, denotes a uniform distribution of x within the range of a ≤ x<b.
In order to evaluate the model's ability to handle diverse data, we prepared another training dataset with varying object sizes and positions/orientations. In addition to the configuration of the standard dataset, objects of varying sizes are incorporated. This dataset comprises of 4,500 data points (1,500 data points each for the normal, bigger, and smaller sizes). We refer to it as a full dataset.
We used a neural network architecture as illustrated in Figure 5. It consists of a recurrent layer with long short-term memories (LSTMs) with 256 units, followed by a dropout layer with a dropout rate of 0.5 and a full-connection layer. For learning stability, we calculate μ as follows:
where denotes the output of a neural-network output. It should be noted that the above scaling is different from the scaling of loss function terms that keep the balance between the gradient of each term; the latter one is difficult to design. The hyperparameters utilized for training are listed in Table 2.
3 Results
3.1 Estimation results with i.i.d. data
We first evaluated the proposed method with objects with stiffness that were unlearned but within the range of the training dataset. Precisely, the range of stiffness varied with the range from 3 to 195 N/m in 32 N/m increments. Additionally, the position/orientation was varied in 10 random values, resulting in a total of 210 data points. As these test data are almost independent and identically distributed (so-called i.i.d.) to the standard dataset, we will call them the i.i.d. dataset.
Figure 6 shows the estimation results. Before touching the object (approximately before 0.5 s), the estimated mean stiffness was around 100 N/m with a large standard deviation in all cases. Additionally, the shape estimation was almost even (≈33%) for all classes. After touching the object, the estimated values converged close to near the true values. The standard deviations were also reduced, as the mean values converged to the true values. The entropy of shape estimation was also decreased a similar manner. At this juncture, the entropy H was calculated from the estimated probabilities of the shape as follows:
where p(s = Sc) indicates the estimated probability that the grasped object belongs to the class Sc.
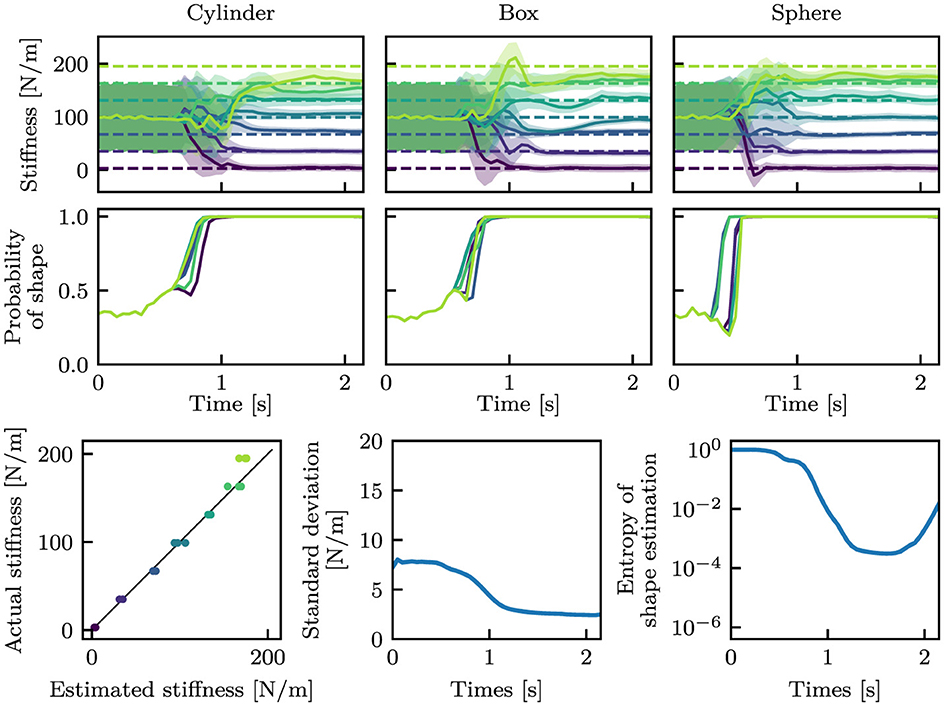
Figure 6. Estimation results with the i.i.d. dataset. The stiffness and shape estimation results are shown at the top and middle rows, respectively. For the stiffness estimation results, dashed lines indicate the true stiffness, and solid curves and filled areas indicate μ and σ. For the shape estimation results, only the estimated probabilities of the true shape classes are shown. The bottom-left graph shows the correspondence between the estimated mean stiffness at the final time step and the true stiffness, where the black line is y = x. The bottom-middle and bottom-right graphs show the mean standard deviations in stiffness estimation and the mean entropy in shape estimation, respectively.
Figure 7 picks up the case of k = 99 N/m of the box-shaped object among Figure 6. The mean values were nearly constant throughout the episode, whereas the standard deviation drastically changed before and after the hand touched the object.
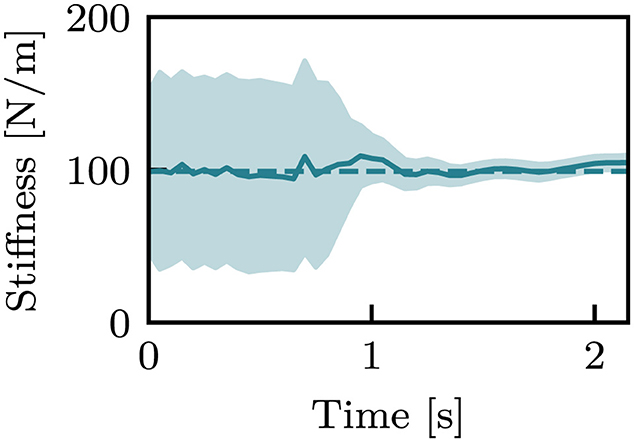
Figure 7. An estimation result with k = 99 N/m of the box-shaped object from the i.i.d. dataset. Dashed lines indicate the true stiffness, and solid curves and filled areas indicate μ and σ.
We also compared the proposed model trained with the standard dataset with baseline models that did not generate the stiffness variance. The baseline models were trained using the identical training dataset and identical hyper-parameters, with the exception of the following loss function:
where α indicates the weight coefficient of the stiffness errors. Figure 8 shows the performance comparison between the proposed model and baseline models with varying performance of α. In the baseline models, a low α resulted in large errors in stiffness estimation, whereas a high α resulted in low performance in shape estimation. The proposed model achieved the highest estimate estimation performance compared to the baseline models.
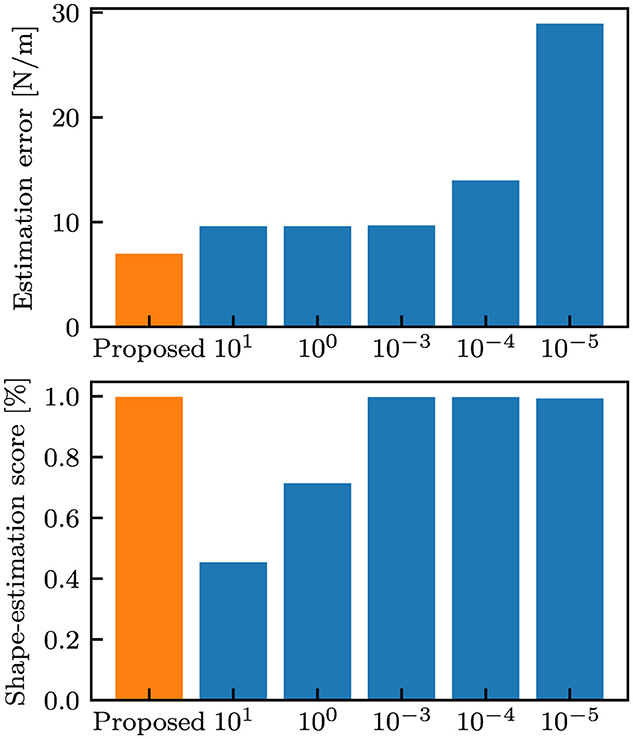
Figure 8. Comparison with baseline models. Each bar indicates the mean of the values at the final time step when evaluated on the i.i.d. dataset. The orange bars correspond to the proposed model, and the blue bars correspond to the baseline models, where the label values indicate α.
3.2 Estimation results with bigger objects
To evaluate how neural networks respond to novel objects, we evaluated the proposed method with objects with larger sizes than those in the training dataset. We shall refer to them as the bigger dataset.
Figure 9 shows the estimation results. It can be observed that the estimation errors were larger than those in Figure 6, i.e., the i.i.d. dataset. Also, the estimated values were largely fluctuated at the beginning of grasping (around 1.0 s).
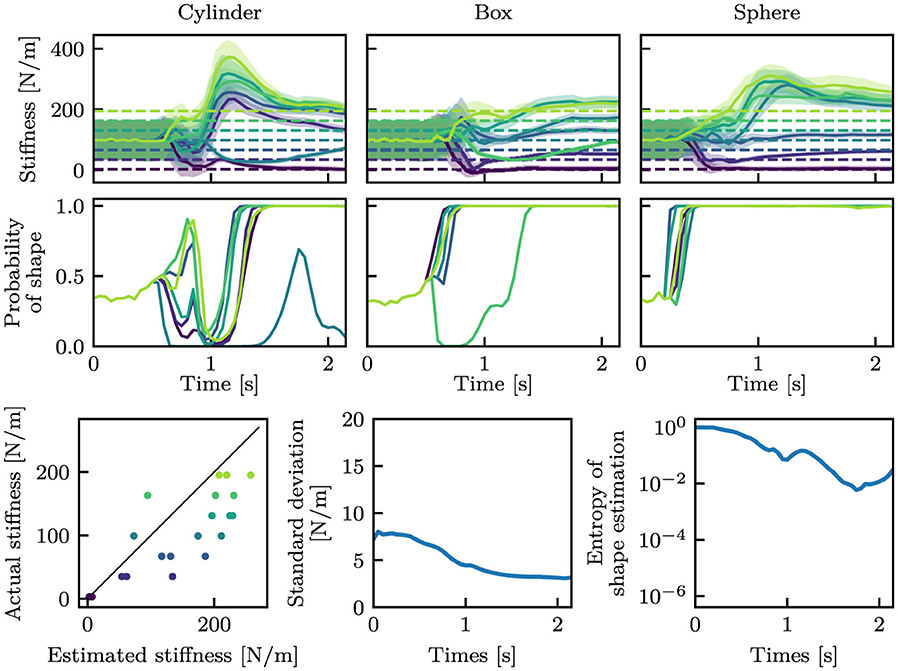
Figure 9. Estimation results with bigger objects. The stiffness and shape estimation results are shown at the top and middle rows, respectively. For the stiffness estimation results, dashed lines indicate the true stiffness, and solid curves and filled areas indicate μ and σ. For the shape estimation results, only estimates of the true shape classes are shown. The bottom-left graph shows the correlation between the estimated mean stiffness at the final time step and the actual stiffness, wherein the black line is y = x. The bottom-middle and bottom-right graphs show the mean standard deviations for stiffness estimation and the mean entropy for shape estimation, respectively.
In order to demonstrate the capability of our method for more diverse data, we also trained the same architecture using the full dataset, which contains objects with varying sizes. Please note that training on a full dataset brings the bigger dataset within the learned range. Figure 10 shows the estimation outcomes. Compared to Figure 9, the model trained with the full dataset correctly estimated the object's properties.
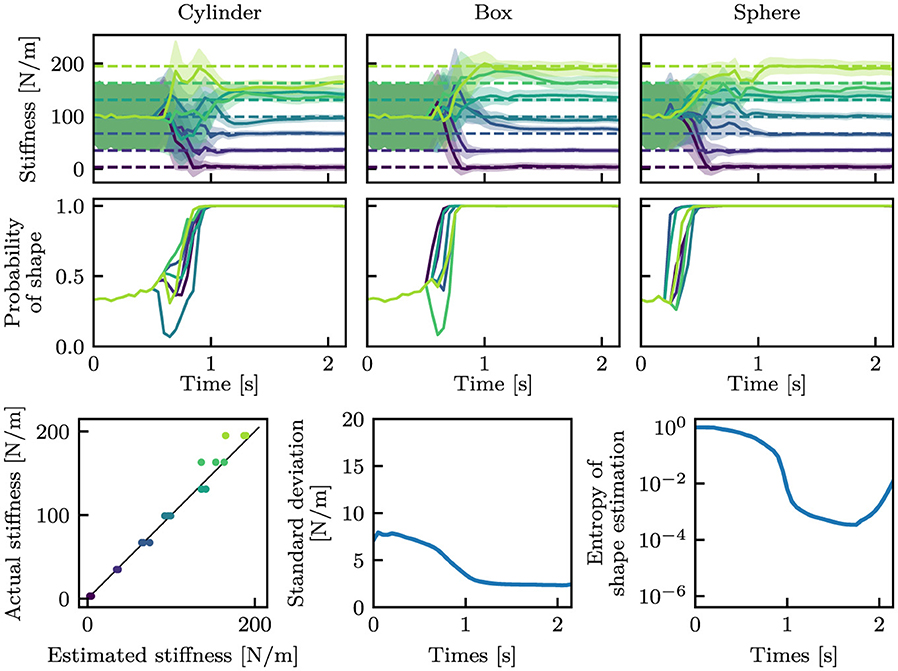
Figure 10. Estimation results with bigger objects by the proposed model trained with the full dataset. The stiffness and shape estimation results are shown at the top and middle rows, respectively. For the stiffness estimation results, dashed lines indicates the true stiffness, and solid curves and filled areas indicate μ and σ. For the shape estimation outcomes, solely the estimated probabilities of the true shape classes are shown. The bottom-left graph shows the correspondence between the estimated mean stiffness at the final time step and the true stiffness, where the black line is y = x. The bottom-middle and bottom-right graphs show the mean standard deviations in stiffness estimation and the mean entropy in shape estimation, respectively.
3.3 Estimation results under observation noises
Subsequently, we assessed the efficacy of the proposed methodology in estimating object properties in the presence of observation noise. In this case, the setup other than observation noises was the same as in Section 3.1. We added two datasets with different observation noises to each dataset. One is achieved with the following noise applied:
This dataset is often referred to as the small noise dataset. Here, εq and denote the noise applied to the joint angles q and the joint angular velocity , respectively. Noises were incorporated for each time step and each degree of freedom autonomously. Another one has the following noise applied:
This dataset is often referred to as the large noise dataset.
Figure 11 shows the estimation outcomes. In contrast to Figure 6, the estimated values showed significant variation. Additionally, it can be observed that the estimated values fluctuated even before touch (before around 0.5 s).
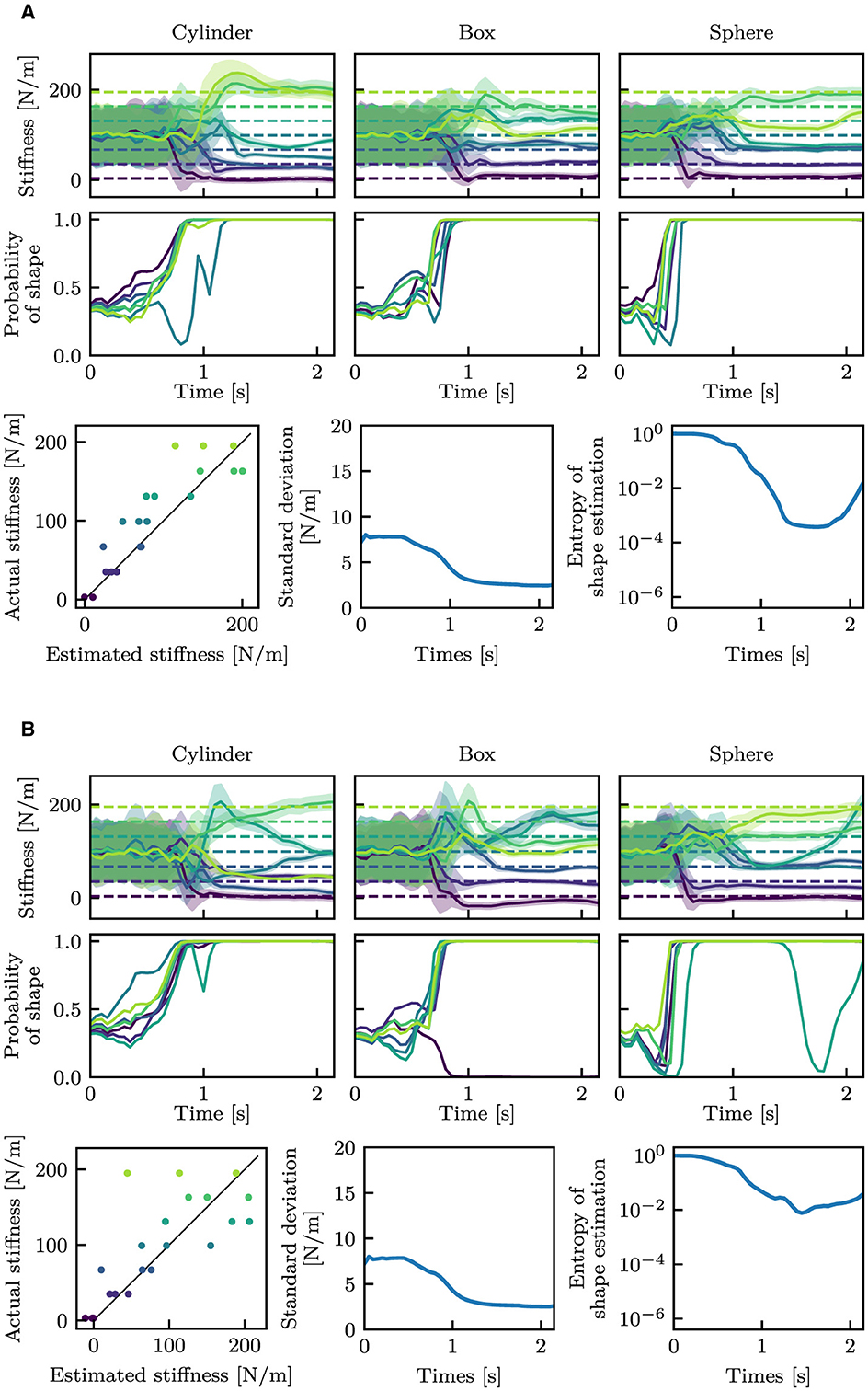
Figure 11. Estimation results with observation noises. The stiffness and shape estimation results are shown at the top and middle rows, respectively. For the stiffness estimation outcomes, dashed lines indicate the true stiffness, and solid curves and filled areas indicate μ and σ. For the shape estimation results, only the estimated probabilities of the true shape classes are shown. The bottom-left graph shows the correlation between the estimated mean stiffness at the final time step and the true stiffness, where the black line is y = x. The bottom-middle and bottom-right graphs show the mean standard deviations for stiffness estimation and the mean entropy for shape estimation, respectively. (A) Small noise. (B) Large noise.
3.4 Comparison of estimated variance
In the previous subsections, we tested trained neural networks with four test datasets: the i.i.d., bigger, smaller, and large noise datasets. In this section, we further analyze the estimated variance (standard deviation).
Figure 12 shows the relationship between the estimated standard deviation and the estimation errors for stiffness. It can be observed that the model trained on the standard dataset predicted significant high standard deviations when significant errors were detected in the i.i.d. dataset. In contrast, the model trained on the full dataset exhibited similar behavior on the bigger dataset in addition to the i.i.d. dataset. The correlation coefficients between the standard deviation and the errors are described in Table 3.
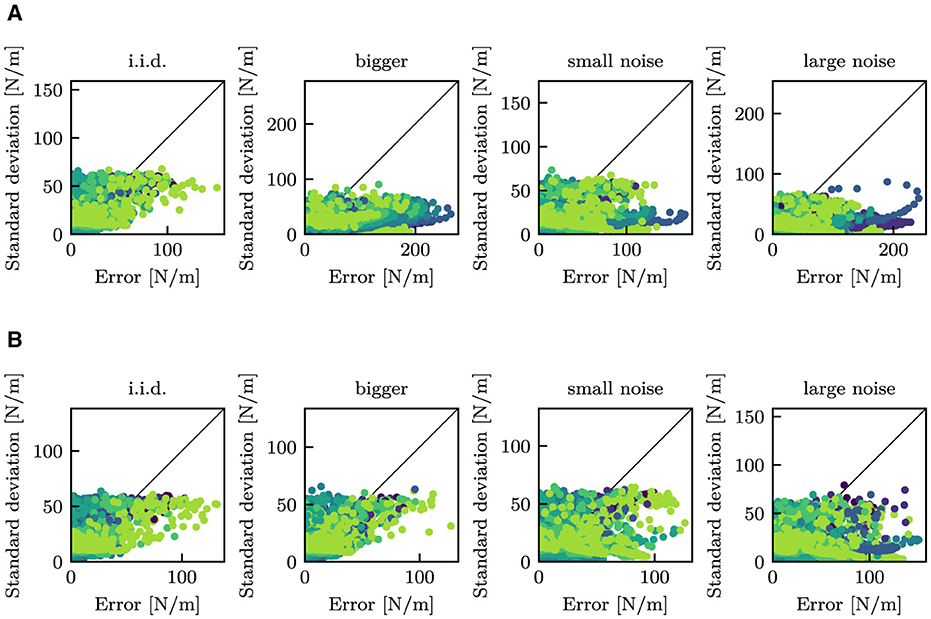
Figure 12. Comparison between errors and standard deviations. Here, the errors are the mean deviations between the true stiffness k and the estimated mean stiffness μ. Each point indicates an estimated value at each time step. Note that values after 0.75 s were shown to exclude values before touch. Colors correspond to the true stiffness; same as those in Figures 6–11. The black lines indicate that y = x. (A) The proposed model trained with the standard dataset. (B) The proposed model trained with the full dataset.
Figure 13 shows variations in the estimated standard deviation of stiffness estimation and the entropy of shape estimation in relation to the authentic stiffness for the test datasets. The standard deviations generally increased with increasing stiffness, whereas the entropy decreased with increasing stiffness. For the bigger dataset, the proposed model trained with the standard dataset produced higher standard deviations and entropy compared to other datasets. In contrast, the proposed model was trained with the full dataset, which contains data with bigger objects, and resulted in compatible entropy and even lower standard deviations than the i.i.d. dataset.
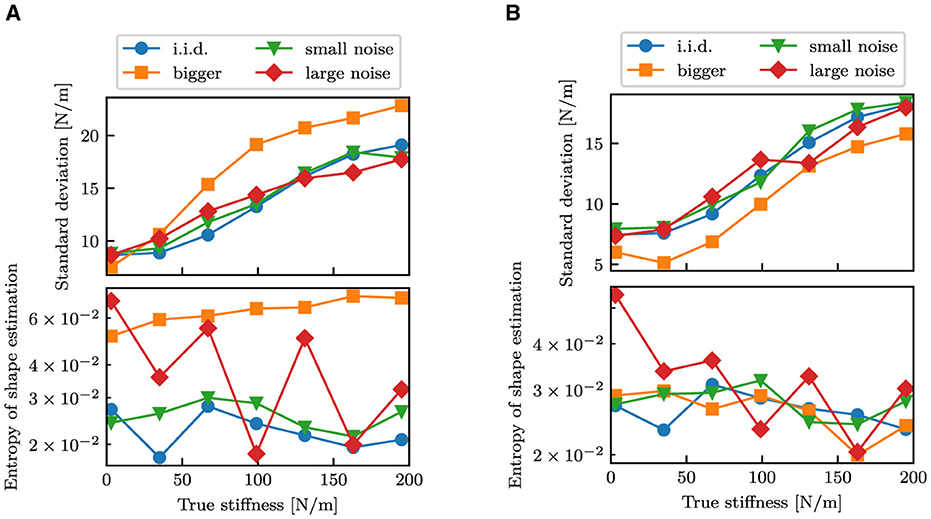
Figure 13. Comparison of the magnitude of estimation uncertainty between different test datasets. Each point indicates the mean standard deviation or the mean entropy after 0.75 s. (A) The proposed model trained with the standard dataset. (B) The proposed model trained with the full dataset.
4 Discussion
4.1 Discussions and conclusions
The proposed model was trained on the standard dataset and was able to estimate the stiffness and the shape from proprioception signals, i.e., joint angle and joint angular velocity. As shown in Figure 6, the trained neural network was capable of accurately estimating object properties from the i.i.d. dataset. In the other datasets, although the accuracy decreased, the stiffness was generally estimated using the correct large and small relationships. Furthermore, the shape was accurately estimated in the majority of instances. The capability of the model can be improved by using a more diverse dataset, as shown in Figure 9. The proposed model that was trained with the full dataset was able to perform well in the bigger dataset.
One of the primary objectives of this study is to generate the variance of stiffness estimation. The estimated variance can be regarded as the confidence level of estimation, including aleatoric and epistemic uncertainty. Variance as epistemic uncertainty can be clearly observed from Figure 7. In that result, the mean stiffness μ was almost constant throughout the episode. However, the standard deviation remarkably changed before and after touching the object. Before touch, the robot cannot estimate the stiffness, as it lacks information about the stiffness. This results in a high variance, which means high epistemic uncertainty. After touch, a wealth of information is provided. The robot is provided with information about the physical properties of the object is provided to the robot through proprioception signals, which allow the robot to decrease the variance. This fundamental change can be found in all cases in Figures 6–11. Moreover, as observed in Figure 13A, the variance was estimated to be larger for the bigger, small noise, and large noise datasets in comparison to the i.i.d. dataset. In contrast, in the model trained on the full dataset, the variance in the bigger dataset decreased (Figure 13B). This implies that the variance that could be reduced by incorporating additional training data appears to be a reflection of the degree of epistemic uncertainty. Based on these factors, it we can be inferred that the proposed models can explicitly indicate their level of confidence in the estimated values in the form of variance. This ability is very helpful when the robot will use the estimated values for motion planning and decision making.
The entropy of shape estimation displayed a resemblance to that observed in the standard deviations of stiffness. The entropy was high prior to contact, indicating a high degree of epistemic uncertainty. Also, the large noise dataset resulted in higher entropy than those in the small noise dataset, which would mean aleatoric uncertainty. Similar to stiffness estimation, the disparity in the bigger dataset between the two models can also be observed in shape estimation. The entropy of the bigger dataset was higher in the model trained on the standard dataset, whereas it was similar to the other test datasets in the model trained on the full dataset. Therefore, the models provide a confidence level for shape estimation based on uncertainty as the entropy, which would also help subsequent decision making.
A comparison between the proposed and baseline models suggests the superiority of the proposed methodology. As shown in Figure 8, the baseline models resulted in varying performance based on the weight coefficient, α. A low α resulted in a low performance in stiffness estimation, whereas a high α resulted in low performance in shape estimation. Therefore, the baseline model necessitates the appropriate value of α to attain satisfactory performance. It will be more difficult as the number of variables to be estimated increases since the number of weight coefficients to be adjusted also increases. In contrast, the proposed model performed the best both in stiffness and shape estimation without any weight coefficient to be adjusted. The balance between the two estimation tasks is automatically taken by the estimation of stiffness variance.
As shown in Figure 13, the estimated variance increased and the entropy slightly decreased with the increasing stiffness. The first reason would be due to the fact that the robot estimates the stiffness based on the deformation of the object through proprioception. According to Hooke's law, the stiffness k can be computed from the displacement δx and contact force f in the following manner:
From the above relationship, it is clear that the amount of displacement is inversely proportional to the increase in stiffness. Therefore, in a high-stiffness object, it is imperative for the robot needs to assess its stiffness based on minimal displacements. This task is comparatively more challenging than estimating an object with low-stiffness. The reduction in variance for the bigger dataset in Figure 13B is explained by the fact that larger objects deform more significantly for the same stiffness, resulting in an easier task. On the other hand, the diminution in entropy may be attributed to the ease with which soft objects can deform. The large deformation will it difficult for the robotic hand to determine the original shape from finger joint angles. In the rigid objects, in contrast, the fingers can bend to follow the object's shape, resulting in an easier shape estimation. Results in Figure 13 may reflect those facts, suggesting that the neural networks could also estimate the confidence level according to the difficulty of the task.
Future work is to combine this strategy with control. Our method, neural networks can represent the confidence level of estimation, including uncertainty and task difficulty, as well as variance and entropy. This aids a task planner in determining the balance between exploration and exploitation. Recent studies have focused on approaches for modeling decision making through probabilistic inference, such as control as inference (Levine, 2018) and active inference (Friston et al., 2016). Our method, which is based on probabilistic inference, can be combined with other methods into a single probabilistic inference.
4.2 Related works
The measurement and estimation of stiffness holds significant importance in engineering. Thus, stiffness measurements have been made in some area. For example, Wang et al. (2016) summarized stiffness measurement methods for train areas. Marter et al. (2018) measured the stiffness of polymer foams using dot markers on the objects. Hattori and Serpa (2015) estimated the normal stiffness of the objects using a neural network. For robotic grasping and manipulation, Kicki et al. (2019) estimated object stiffness by measuring the contact force and the finger distance with varying grasping force. Spiers et al. (2016) combined tactile and actuator signals to assess the stiffness and the posture of the objects. For stiffness measurement, those methods use both contact force and displacement information. This is intuitive, since the Hooke's law argues that the stiffness k is a ratio of the displacement δx and contact force f, i.e., . On the other hand, in fact, it is also possible to estimate stiffness without directly measuring of force. For example, a reaction force observer (Murakami et al., 1993; Ohnishi et al., 1996) facilitates the estimation of external force in the absence of force sensors. Coutinho and Cortesão (2014) proposed a method for stiffness estimation method based on two force observers with different stiffness candidates. Also, Bednarek et al. (2021) estimated the stiffness of the grasped objects using a soft gripper with internal measurement units (IMUs) through learning. These studies support the possibility of stiffness estimation from proprioception signals. However, this is yet to be fully evaluated in the context of multi-finger robotic hands.
The estimate of object shape by contact has been investigated. Tsujimura and Yabuta (1989) proposed a method for object-shape detection by using a probe with a six-axis force/torque sensor. Mimura and Funahashi (1994) classified tools based on their contact state, which can be identified by force/torque signals. Also, the force signal-based object-shape estimation is often realized using particle filters (von Drigalski et al., 2020; Kutsuzawa et al., 2020; Bimbo et al., 2022). Drimus et al. (2014) developed a novel approach. The tactile array sensor utilizes piezoresistive rubber and thread electrodes for object classification, coupled with a gripper. Gao et al. (2016) used convolutional neural networks to associate haptic and visual measurements with haptic adjectives, such as rough, hairy, and soft. There are also methods to estimate the object shape by using a particle filter (Behbahani et al., 2015) and a Gaussian process (Khadivar et al., 2023), both of which are based on probabilistic inference. According to those methods, probabilistic inference is effective for geometric-property estimation. Based on this fact, we also evaluate geometric properties using a probabilistic way unified with stiffness estimation in this study.
There are numerous robotic hands with tactile sensors (Dahiya et al., 2010; Narita et al., 2020; Cirillo et al., 2022; Spiers et al., 2016). Although tactile sensors and their applications are undergoing development, they are still in their infancy. First, high-precision tactile sensors are expensive; this is a problem when considering mass production and industrial applications. Second, sensors are often sensitive to external disturbances such as temperature, light, or electromagnetic fields. Given their cost and robustness against the external environment, the primary use of proprioception sensors is effective. Robotic hands can have the ability to handle contact even without tactile sensors. The robotic hand developed by Ajoudani et al. (2013) does not have force sensors, but instead estimates contact force by using interaction torque observers. Xu et al. (2021) developed a soft gripper with a grasping force estimation method using a neural network based on the amount of deformation captured by a camera. Nagabandi et al. (2020) and Andrychowicz et al. (2020) used a robotic hand with a 24 degrees-of-freedom robotic hand for dexterous manipulation with reinforcement learning.
Handling uncertainty is an important topic in machine learning field. Uncertainties are sometimes classified into aleatoric and epistemic uncertainty with the former referring to uncertainty due to randomness, and the latter referring to uncertainty caused by lack of knowledge (Hora, 1996; Hüllermeier and Waegeman, 2021). A common approach to uncertainty is probabilistic inference, such as the Gaussian process, Kalman filter, and Monte Carlo methods, but these methods have limited representation ability or are often computationally expensive in high-dimensional signal processing. For methods based on neural networks, Bayesian neural networks can naturally perform Bayesian inference (Denker and LeCun, 1990), but the naive way can also be computationally expensive. Nix and Weigend (1994) first proposed a neural network that could generate the mean and variance of the target-data distribution. This method is often used because it is simple to implement and calculate. As per recent well-known models, variational auto-encoders (Kingma and Welling, 2013) utilize neural networks to generate the mean and variance to represent a Gaussian distribution of latent variables. Deep reinforcement learning methods also incorporate policy neural networks that generate variance of actions (Haarnoja et al., 2018). More details can be figured out in Gawlikowski et al. (2023). As robotic applications of variance-output neural networks, Ding et al. (2021) developed neural networks model for soft actuators that predict the actuator's position, contact location, and contact force, including their variance. Also, Takahashi et al. (2021) used neural networks treats estimate estimation errors and treat the estimated estimation errors as the actual amount of epistemic uncertainty. We adopted the approach of Nix and Weigend (1994) for stiffness estimation due to its simplicity, while addressing the shape classification task together. We will demonstrate that this approach can not only can quantify estimation uncertainty, but also eliminate hyper-parameters from the training loss function.
Data availability statement
The datasets presented in this study can be found in online repositories. The names of the repository/repositories and accession number(s) can be found below: https://github.com/kyo-kutsuzawa/object_property_estimation_in_robotic_hand.
Author contributions
KK: Conceptualization, Data curation, Formal analysis, Funding acquisition, Investigation, Methodology, Project administration, Resources, Software, Supervision, Validation, Visualization, Writing – original draft, Writing – review & editing. MM: Conceptualization, Data curation, Investigation, Methodology, Resources, Software, Validation, Visualization, Writing – original draft, Writing – review & editing, Formal analysis. DO: Supervision, Writing – review & editing, Conceptualization. MH: Conceptualization, Project administration, Supervision, Writing – review & editing.
Funding
The author(s) declare financial support was received for the research, authorship, and/or publication of this article. This work was supported by JSPS KAKENHI (Grant Number 22K14212) and the Innovation and Technology Commission of the HKSAR Government under the InnoHK initiative, Hong Kong.
Conflict of interest
The authors declare that the research was conducted in the absence of any commercial or financial relationships that could be construed as a potential conflict of interest.
Publisher's note
All claims expressed in this article are solely those of the authors and do not necessarily represent those of their affiliated organizations, or those of the publisher, the editors and the reviewers. Any product that may be evaluated in this article, or claim that may be made by its manufacturer, is not guaranteed or endorsed by the publisher.
Supplementary material
The Supplementary Material for this article can be found online at: https://www.frontiersin.org/articles/10.3389/fnbot.2024.1466630/full#supplementary-material
References
Ajoudani, A., Godfrey, S. B., Catalano, M., Grioli, G., Tsagarakis, N. G., and Bicchi, A. (2013). “Teleimpedance control of a synergy-driven anthropomorphic hand,” in 2013 IEEE/RSJ International Conference on Intelligent Robots and Systems (Tokyo), 1985–1991.
Andrychowicz, O. A. M., Baker, B., Chociej, M., Józefowicz, R., McGrew, B., Pachocki, J., et al. (2020). Learning dexterous in-hand manipulation. Int. J. Rob. Res. 39, 3–20. doi: 10.1177/0278364919887447
Bednarek, M., Kicki, P., Bednarek, J., and Walas, K. (2021). Gaining a sense of touch object stiffness estimation using a soft gripper and neural networks. Electronics 10:96. doi: 10.3390/electronics10010096
Behbahani, F. M. P., Taunton, R., Thomik, A. A. C., and Faisal, A. A. (2015). “Haptic slam for context-aware robotic hand prosthetics - simultaneous inference of hand pose and object shape using particle filters,” in 2015 7th International IEEE/EMBS Conference on Neural Engineering (NER) (Montpellier: IEEE), 719–722. doi: 10.1109/NER.2015.7146724
Bimbo, J., Morgan, A. S., and Dollar, A. M. (2022). Force-based simultaneous mapping and object reconstruction for robotic manipulation. IEEE Robot. Automat. Lett. 7, 4749–4756. doi: 10.1109/LRA.2022.3152244
Cirillo, A., Laudante, G., and Pirozzi, S. (2022). “Wire grasping by using proximity and tactile sensors,” in 2022 IEEE 5th International Conference on Industrial Cyber-Physical Systems (ICPS) (Coventry: IEEE), 1–6.
Coutinho, F., and Cortesão, R. (2014). Online stiffness estimation for robotic tasks with force observers. Control Eng. Pract. 24, 92–105. doi: 10.1016/j.conengprac.2013.11.002
Dahiya, R., Metta, G., Valle, M., and Sandini, G. (2010). Tactile sensing—from humans to humanoids. IEEE Transact. Robot. 26, 1–20. doi: 10.1109/TRO.2009.2033627
Denker, J., and LeCun, Y. (1990). Transforming neural-net output levels to probability distributions. Adv. Neur. Inf. Process. Syst. 3, 853–859.
Ding, Z. Y., Loo, J. Y., Baskaran, V. M., Nurzaman, S. G., and Tan, C. P. (2021). Predictive uncertainty estimation using deep learning for soft robot multimodal sensing. IEEE Robot. Automat. Lett. 6, 951–957. doi: 10.1109/LRA.2021.3056066
Drimus, A., Kootstra, G., Bilberg, A., and Kragic, D. (2014). Design of a flexible tactile sensor for classification of rigid and deformable objects. Robot. Auton. Syst. 62, 3–15. doi: 10.1016/j.robot.2012.07.021
Friston, K., FitzGerald, T., Rigoli, F., Schwartenbeck, P., O'Doherty, J., and Pezzulo, G. (2016). Active inference and learning. Neurosci. Biobehav. Rev. 68, 862–879. doi: 10.1016/j.neubiorev.2016.06.022
Gao, Y., Hendricks, L. A., Kuchenbecker, K. J., and Darrell, T. (2016). “Deep learning for tactile understanding from visual and haptic data,” in 2016 IEEE International Conference on Robotics and Automation (ICRA) (Stockholm: IEEE), 536–543.
Gawlikowski, J., Tassi, C. R. N., Ali, M., Lee, J., Humt, M., Feng, J., et al. (2023). A survey of uncertainty in deep neural networks. Artif. Intell. Rev. 56, 1513–1589. doi: 10.1007/s10462-023-10562-9
Haarnoja, T., Zhou, A., Abbeel, P., and Levine, S. (2018). “Soft actor-critic: off-policy maximum entropy deep reinforcement learning with a stochastic actor,” in Proceedings of the 35th International Conference on Machine Learning (PMLR), Vol. 80 (Stockholm: PMLR), 1861–1870.
Hattori, G., and Serpa, A. L. (2015). Contact stiffness estimation in ANSYS using simplified models and artificial neural networks. Finite Elements Anal. Des. 97, 43–53. doi: 10.1016/j.finel.2015.01.003
Hora, S. C. (1996). Aleatory and epistemic uncertainty in probability elicitation with an example from hazardous waste management. Reliabil. Eng. Syst. Saf. 54, 217–223. doi: 10.1016/S0951-8320(96)00077-4
Hüllermeier, E., and Waegeman, W. (2021). Aleatoric and epistemic uncertainty in machine learning: an introduction to concepts and methods. Mach. Learn. 110, 457–506. doi: 10.1007/s10994-021-05946-3
Khadivar, F., Yao, K., Gao, X., and Billard, A. (2023). Online active and dynamic object shape exploration with a multi-fingered robotic hand. Rob. Auton. Syst. 166:104461. doi: 10.1016/j.robot.2023.104461
Kicki, P., Bednarek, M., and Walas, K. (2019). “Robotic manipulation of elongated and elastic objects,” in 2019 Signal Processing: Algorithms, Architectures, Arrangements, and Applications (SPA) (Poznan: IEEE), 23–27.
Kingma, D. P., and Welling, M. (2013). Auto-encoding variational bayes. arXiv [preprint]. doi: 10.48550/arXiv.1312.6114
Kutsuzawa, K., Sakaino, S., and Tsuji, T. (2017). “Sequence-to-sequence models for trajectory deformation of dynamic manipulation,” in IECON 2017 - 43rd Annual Conference of the IEEE Industrial Electronics Society (Beijing), 5227–5232.
Kutsuzawa, K., Sakaino, S., and Tsuji, T. (2018). Sequence-to-sequence model for trajectory planning of nonprehensile manipulation including contact model. IEEE Robot. Automat. Lett. 3, 3606–3613. doi: 10.1109/LRA.2018.2854958
Kutsuzawa, K., Sakaino, S., and Tsuji, T. (2020). Simultaneous estimation of contact position and tool shape using an unscented particle filter. IEEJ J. Ind. Appl. 9, 505–514. doi: 10.1541/ieejjia.9.505
Levine, S. (2018). Reinforcement learning and control as probabilistic inference: tutorial and review. arXiv [preprint]. doi: 10.48550/arXiv.1805.00909
Marter, A., Dickinson, A., Pierron, F., and Browne, M. (2018). A practical procedure for measuring the stiffness of foam like materials. Exp. Tech. 42, 439–452. doi: 10.1007/s40799-018-0247-0
Mimura, N., and Funahashi, Y. (1994). “Parameter identification of contact conditions by active force sensing,” in Proceedings of the 1994 IEEE International Conference on Robotics and Automation (San Diego, CA: IEEE Comput. Soc. Press), 2645–2650.
Murakami, T., Yu, F., and Ohnishi, K. (1993). Torque sensorless control in multidegree-of-freedom manipulator. IEEE Transact. Ind. Electron. 40, 259–265. doi: 10.1109/41.222648
Nagabandi, A., Konoglie, K., Levine, S., and Kumar, V. (2020). “Deep dynamics models for learning dexterous manipulation,” in 3rd Conference on Robot Learning (CoRL 2019) (Osaka: PMLR), 100, 1101–1112.
Narita, T., Nagakari, S., Conus, W., Tsuboi, T., and Nagasaka, K. (2020). “Theoretical derivation and realization of adaptive grasping based on rotational incipient slip detection,” in 2020 IEEE International Conference on Robotics and Automation (ICRA) (Paris: IEEE), 531–537.
Nix, D., and Weigend, A. (1994). “Estimating the mean and variance of the target probability distribution,” in Proceedings of 1994 IEEE International Conference on Neural Networks (ICNN'94), Vol. 1 (Orlando, FL: IEEE), 55–60.
Ohnishi, K., Shibata, M., and Murakami, T. (1996). Motion control for advanced mechatronics. IEEE/ASME Transact. Mechatron. 1, 56–67. doi: 10.1109/3516.491410
Spiers, A. J., Liarokapis, M. V., Calli, B., and Dollar, A. M. (2016). Single-grasp object classification and feature extraction with simple robot hands and tactile sensors. IEEE Trans. Haptics 9, 207–220. doi: 10.1109/TOH.2016.2521378
Takahashi, K., Ko, W., Ummadisingu, A., and Maeda, S.-i. (2021). “Uncertainty-aware self-supervised target-mass grasping of granular foods,” in 2021 IEEE International Conference on Robotics and Automation (ICRA) (Xi'an: IEEE), 2620–2626.
Todorov, E., Erez, T., and Tassa, Y. (2012). “MuJoCo: a physics engine for model-based control,” in 2012 IEEE/RSJ International Conference on Intelligent Robots and Systems (Vilamoura), 5026–5033.
Tsujimura, T., and Yabuta, T. (1989). Object detection by tactile sensing method employing force/torque information. IEEE Transact. Robot. Automat. 5, 444–450. doi: 10.1109/70.88059
von Drigalski, F., Taniguchi, S., Lee, R., Matsubara, T., Hamaya, M., Tanaka, K., et al. (2020). “Contact-based in-hand pose estimation using bayesian state estimation and particle filtering.” in 2020 IEEE International Conference on Robotics and Automation (ICRA) (Paris: IEEE), 7294–7299.
Wang, C., Zhang, X., Zang, X., Liu, Y., Ding, G., Yin, W., et al. (2020). Feature sensing and robotic grasping of objects with uncertain information: a review. Sensors 20:3707. doi: 10.3390/s20133707
Wang, P., Wang, L., Chen, R., Xu, J., Xu, J., and Gao, M. (2016). Overview and outlook on railway track stiffness measurement. J. Mod. Transport. 24, 89–102. doi: 10.1007/s40534-016-0104-8
Xu, W., Zhang, H., Yuan, H., and Liang, B. (2021). A compliant adaptive gripper and its intrinsic force sensing method. IEEE Transact. Robot. 37, 1584–1603. doi: 10.1109/TRO.2021.3060971
Zakka, K., Tassa, Y., and MuJoCo Menagerie Contributors (2022). MuJoCo Menagerie: A Collection of High-Quality Simulation Models for MuJoCo.
Keywords: robotic hand, grasping, stiffness estimation, shape estimation, probabilistic inference, deep learning, proprioception
Citation: Kutsuzawa K, Matsumoto M, Owaki D and Hayashibe M (2024) Learning-based object's stiffness and shape estimation with confidence level in multi-fingered hand grasping. Front. Neurorobot. 18:1466630. doi: 10.3389/fnbot.2024.1466630
Received: 18 July 2024; Accepted: 11 October 2024;
Published: 19 November 2024.
Edited by:
Ying Mao, Fordham University, United StatesReviewed by:
Zefan Du, Fordham University, United StatesShuwen Kan, Fordham University, United States
Copyright © 2024 Kutsuzawa, Matsumoto, Owaki and Hayashibe. This is an open-access article distributed under the terms of the Creative Commons Attribution License (CC BY). The use, distribution or reproduction in other forums is permitted, provided the original author(s) and the copyright owner(s) are credited and that the original publication in this journal is cited, in accordance with accepted academic practice. No use, distribution or reproduction is permitted which does not comply with these terms.
*Correspondence: Kyo Kutsuzawa, a3V0c3V6YXdhQHRvaG9rdS5hYy5qcA==