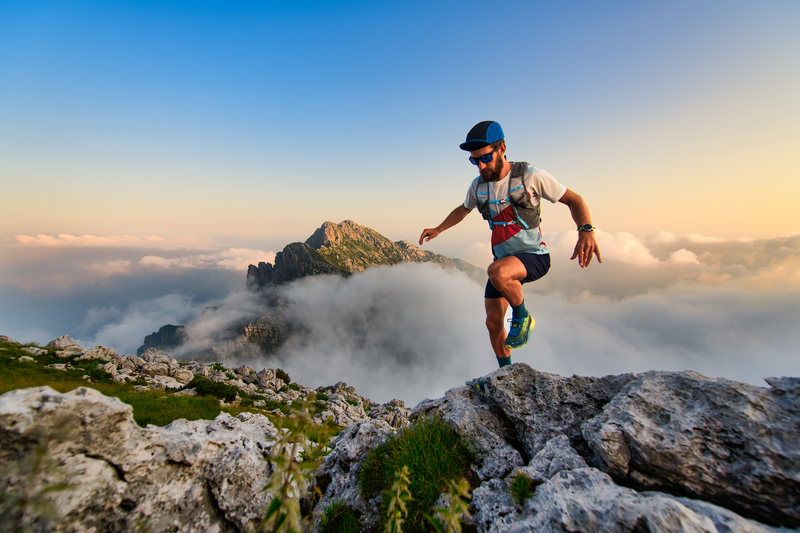
94% of researchers rate our articles as excellent or good
Learn more about the work of our research integrity team to safeguard the quality of each article we publish.
Find out more
ORIGINAL RESEARCH article
Front. Neurorobot. , 05 April 2024
Volume 18 - 2024 | https://doi.org/10.3389/fnbot.2024.1371385
This article is part of the Research Topic Machine Learning and Applied Neuroscience: Volume II View all 4 articles
In the realm of human motion recognition systems, the augmentation of 3D human pose data plays a pivotal role in enriching and enhancing the quality of original datasets through the generation of synthetic data. This augmentation is vital for addressing the current research gaps in diversity and complexity, particularly when dealing with rare or complex human movements. Our study introduces a groundbreaking approach employing Generative Adversarial Networks (GANs), coupled with Support Vector Machine (SVM) and DenseNet, further enhanced by robot-assisted technology to improve the precision and efficiency of data collection. The GANs in our model are responsible for generating highly realistic and diverse 3D human motion data, while SVM aids in the effective classification of this data. DenseNet is utilized for the extraction of key features, facilitating a comprehensive and integrated approach that significantly elevates both the data augmentation process and the model's ability to process and analyze complex human movements. The experimental outcomes underscore our model's exceptional performance in motion quality assessment, showcasing a substantial improvement over traditional methods in terms of classification accuracy and data processing efficiency. These results validate the effectiveness of our integrated network model, setting a solid foundation for future advancements in the field. Our research not only introduces innovative methodologies for 3D human pose data enhancement but also provides substantial technical support for practical applications across various domains, including sports science, rehabilitation medicine, and virtual reality. By combining advanced algorithmic strategies with robotic technologies, our work addresses key challenges in data augmentation and motion quality assessment, paving the way for new research and development opportunities in these critical areas.
3D human orientation data augmentation is an advanced research topic involving the fields of computer vision and machine learning, which aims at augmenting existing datasets by generating new, synthesized 3D human movement data. This process is crucial for improving the performance of human action recognition systems, especially in applications that require a high degree of accuracy and robustness (Xu et al., 2021), such as motion quality assessment, virtual reality, video surveillance, and health monitoring. The core aim of 3D human orientation data augmentation is to create new data that can extend and complement the original training dataset (Li X. et al., 2023). This is because the original dataset may be limiting, such as insufficient number of samples, insufficient sample diversity, or inability to cover all possible human movements. By augmenting the data, it is possible to generate new data samples that are synthesized but highly similar to the real data in terms of structure and motion characteristics.
In recent years, the application of Generative Adversarial Networks (GANs) in the field of 3D human pose data augmentation has become a focal point of contemporary research. GANs, with their unique architecture of generators and discriminators, generate new data instances while learning and mimicking the distribution of real data. In the context of 3D human movement data, GANs are capable of creating lifelike sequences of human movements, which is particularly valuable for training deep learning models. These synthetic data significantly increase the diversity and size of the training set, aiding the model in learning more complex and subtle human movement patterns (Nian, 2022). Moreover, GANs demonstrate great potential in addressing data imbalance issues by generating samples that are scarce or absent in the original training set, thus enhancing the model's ability to recognize rare or challenging movements (Ning et al., 2024). Overall, the application of GANs in 3D human pose data augmentation not only improves the performance and generalization capability of models but also opens new avenues for a deeper understanding of complex human movements.
Robotic-assisted technology, due to its high precision and repeatability, is particularly suited for assisting with Generative Adversarial Networks (GANs) in researching data augmentation methods for 3D human body orientation. This technology utilizes advanced sensors and precise control systems to simulate and record complex human actions, providing high-quality and diverse data for datasets (Cai et al., 2021). Specifically, robot-assisted technology is capable of capturing human motion data with extreme precision through the use of advanced sensors such as optical trackers and force sensors (Li J. et al., 2023). Additionally, by precisely controlling the robot's movements, specific human motions can be replicated under safe conditions, which is crucial for generating realistic and diverse datasets. This approach not only improves the quality of the data but also significantly enhances the efficiency and reliability of data collection. The application of robotic-assisted technology in the field of 3D human posture data augmentation opens new directions for research (Lee et al., 2021). It not only enhances the realism and diversity of the data generated by GANs but also provides more accurate and abundant data resources for research in fields like sports science and rehabilitation medicine. By further optimizing robotic technologies and data processing methods, there is potential for achieving more advanced data augmentation techniques in the future, bringing revolutionary progress to 3D human posture estimation and related application fields.
However, due to the inherent complexity and variability of 3D human body data, effectively enhancing such data remains a complex challenge. One of the foremost challenges lies in achieving realism and diversity in the generated data (Gao et al., 2023). Realism entails ensuring that the generated data closely resembles real-world 3D human body movements, ensuring optimal model performance in practical applications. Achieving this level of realism is intricate, as it necessitates consideration of factors such as body posture, movement smoothness, joint naturalness, and more. Diversity, on the other hand, involves generating 3D human body data of different types, backgrounds, and environments to enable the model to adapt to diverse scenarios and application requirements. Data diversity is crucial for enhancing the model's generalization capabilities but poses its own set of challenges. Another critical challenge is the cost associated with data collection and annotation. Collecting 3D human pose data typically requires specialized equipment like motion capture systems, significantly driving up the cost of data acquisition. Furthermore, annotating large-scale datasets demands substantial human and time resources. Consequently, researchers and practitioners face considerable difficulties in data collection and annotation. One potential solution is the exploration of unsupervised or weakly supervised learning approaches to reduce reliance on extensively annotated data, but this remains an active research area.
In addressing the challenges inherent in 3D human pose data augmentation, this work introduces an innovative GANs-SVM-DenseNet network model that significantly advances the field. Traditional methods in data augmentation and motion quality assessment often struggle with generating diverse and realistic datasets that accurately mimic the complex nature of human movements. This limitation hampers the development of robust machine learning models capable of precise motion recognition and quality assessment.
We introduce a pioneering approach that synergizes GAN-generated synthetic data with high-precision, robotics-assisted data collection techniques. This integration is novel, enhancing the quality and diversity of data available for 3D human pose analysis, a step beyond the current state-of-the-art practices that rely on either synthetic or real-world data in isolation. Our proposed model harnesses the generative power of GANs to produce varied and lifelike 3D human motion datasets, overcoming the challenge of limited data diversity. The SVM component of our model brings high precision in classifying the quality of these movements, addressing the challenge of accurately evaluating complex motion patterns. DenseNet further enhances our model by extracting intricate features from the augmented data, ensuring that the subtleties of human motion are captured and utilized effectively for quality assessment.
We provide a comprehensive evaluation of our proposed method against current state-of-the-art models in 3D data augmentation. Our analysis not only demonstrates the superiority of our approach in generating realistic and diverse human poses but also highlights the specific improvements our method offers over existing techniques in terms of data quality, usability, and application to motion quality assessment. The integration of these technologies enables our model to not only augment 3D human pose data with high realism and diversity but also to assess motion quality with unprecedented accuracy. This contribution is pivotal for applications requiring precise motion analysis, such as in healthcare for rehabilitation assessment, in sports science for performance evaluation, and in the development of interactive virtual reality environments. By elaborating on the specific challenges these technologies address, this work clearly delineates its contribution to the field, setting a new benchmark for future research in 3D human pose data augmentation and motion quality assessment.
The contribution points of this paper are as follows:
• We successfully applied the integration of GANs, SVM, and DenseNet to the study of 3D human orientation data enhancement. This integration not only improves the quality and diversity of data enhancement, but also enhances the performance of the model in processing complex 3D human movement data. Our research results help to address the limitations of current 3D human motion datasets in terms of size and diversity, and provide new directions for future related research.
• We integrated robot-assisted techniques in our study to improve the accuracy of 3D human orientation data acquisition and processing efficiency. By comparing with traditional methods, we show how robotics can achieve higher accuracy and consistency in the data acquisition process. This finding not only improves data quality, but also provides practical guidance and reference for future applications in similar fields.
• We comprehensively evaluated the potential of the model for application in the assessment of movement quality, and the experimental results confirmed that the model performs well in processing and analyzing complex human movements with high efficiency and accuracy. This result not only provides strong technical support for practical applications in the fields of sports science and rehabilitation medicine, but also offers new possibilities for technological innovation and development in these fields.
The structure of this article is organized as follows: Section 2 delves into the related work, encompassing existing studies on 3D human posture estimation and data augmentation techniques pertinent to our research. Section 3 introduces our proposed robotic-assisted data augmentation method, detailing the technical specifics and implementation process. Section 4 presents the experimental design, execution, and the results obtained using our proposed method, along with a comparative analysis against existing technologies. Section 5 discusses the experimental outcomes, explores potential areas for method improvement, and suggests directions for future research.
This research focuses on using deep learning techniques to enhance 3D human motion data. Specifically, it explores how Convolutional Neural Networks (CNNs) and Recurrent Neural Networks (RNNs) can be utilized to process and generate more diverse and rich human motion data (Pham et al., 2019). These techniques can learn from existing small datasets and generate new data samples to support more complex motion analysis and machine learning applications (Wang et al., 2018). CNNs are primarily used here to process and understand spatial features in 3D motion data, such as the positions and orientations of human joints. RNNs, on the other hand, are employed to handle time-series data, capturing the dynamic changes and temporal dependencies in movements (Zhang et al., 2022). Combining these two types of networks, researchers can generate 3D motion data with high realism and coherence. The key advantage of this method is its ability to significantly expand the size and diversity of existing datasets, especially in areas where collecting large amounts of high-quality data is costly (Liu et al., 2021). The data generated through deep learning can be used to train more complex machine learning models, improving their performance and accuracy in handling complex movements.
Deep learning-based data augmentation methods, while effective in expanding datasets, encounter certain limitations. Primarily, the quality of the data they generate is closely tied to the quality of the original dataset (Han et al., 2020). This means that limitations or flaws in the original dataset can lead to generated data that does not fully capture the real movements' diversity and complexity. Additionally, these deep learning models demand significant computational resources and extensive training time, particularly for large-scale or complex datasets, which could be a hindrance in environments with limited resources. Furthermore, the technical aspect of designing and optimizing these models requires specialized expertise (Wang and Zhang, 2022). Without this, there's a risk of incorrect model architecture or parameter settings, potentially resulting in low-quality data or issues such as overfitting.
In recent years, deep learning, especially Convolutional Neural Networks (CNNs), has made significant strides in the field of human pose estimation. These methods typically involve large-scale annotated datasets containing images of human bodies in various postures with their corresponding keypoint locations (Luvizon et al., 2020). CNN models are trained to accurately identify and locate key body parts, such as the head, arms, and legs, from these images. The essence of these models is their ability to learn complex feature representations from images (Le, 2023), achieved through layers of convolutions, activations, and pooling. During training, the network fine-tunes millions of parameters to gradually enhance its ability to recognize human poses (Ukita and Uematsu, 2018). As the model depth increases, the network recognizes more abstract and complex image features, leading to more precise pose estimation.
A significant direction of development is real-time pose estimation (Iqbal and Gall, 2016). This requires models to be not only accurate but also fast enough to process images in a video stream in real-time. Researchers have developed a series of optimization algorithms and lightweight network architectures to reduce computational burdens while maintaining high accuracy. Another important branch in this field is 3D pose estimation. Unlike 2D estimation, 3D pose estimation aims to reconstruct a three-dimensional model of the human posture from images (Szczuko, 2019). This demands more complex network architectures and algorithms to solve the mapping problem from two-dimensional images to three-dimensional space.
Despite the remarkable achievements of deep learning in human pose estimation, these methods have some limitations. Firstly, they largely depend on vast amounts of annotated data. Without sufficient, high-quality annotated data, the performance of the models significantly drops. This is particularly evident in recognizing special postures or rare movements. Secondly, although some models achieve real-time processing, they typically require substantial computational resources, limiting their application in mobile devices or edge computing environments (Wu et al., 2020). While 3D pose estimation provides more spatial information, accurately recovering 3D information from 2D images remains a highly challenging problem. The robustness of these models in handling occlusions, changes in viewpoints, or atypical postures still needs improvement.
This study explores the application of Random Forests in assessing movement quality (Zhu et al., 2019). Random Forest is a robust machine learning method that falls under ensemble learning. It improves overall prediction accuracy by constructing multiple decision trees and combining their predictions. This method excels in handling complex and non-linear data, making it particularly suited for movement data analysis (Zhou et al., 2020). In this research, data is collected from athletes performing various movements, including parameters like strength, speed, endurance, and accuracy of movement. The Random Forest model is trained to identify and analyze these data to assess the quality and efficiency of the movement.
A key advantage of Random Forest is its high tolerance for data and ability to handle a large number of input variables. This makes it especially suitable for analyzing movement data, which often contains multiple dimensions and complex relationships. Additionally, its efficacy in preventing overfitting is critical for maintaining the model's generalization capability (De Mello et al., 2020). In practice, this method can be used to help coaches and athletes better understand performance, guide training plans, and identify potential areas for improvement. For example, in sports like gymnastics or weightlifting, analyzing the quality of movements can provide specific guidance for technical improvements and adjustments.
Random Forest is effective with complex datasets, yet it is not without its limitations. A key challenge is its need for a substantial amount of training data to ensure accurate predictions, as each decision tree in the forest requires sufficient information (Zhang and Ma, 2019). With limited data, the model's performance may suffer. Additionally, the interpretability of the Random Forest's results can be less straightforward compared to other models. Given its reliance on an ensemble of decision trees, comprehending and articulating the exact prediction process can be intricate, posing challenges in scenarios where a precise understanding of the model's decision-making is crucial (Matloob et al., 2021). And while Random Forest is generally adaptable to various movement data types, its effectiveness can diminish in certain conditions, particularly when the data contains significant noise or outliers.
This research investigates the use of motion capture systems in a Virtual Reality (VR) environment to analyze and assess human movement. In this method, VR technology is used to create an immersive environment, while motion capture systems are employed to accurately record athletes' body movements and postures (Shi et al., 2019). This combination offers a unique platform for detailed analysis of various aspects of movement, including speed, strength, accuracy, and coordination. A major advantage of using a VR environment is that it allows researchers and athletes to experiment and train in a controlled and safe setting (Wedel et al., 2020). Additionally, VR technology can simulate various sporting scenarios and conditions, providing more diverse training and assessment opportunities. Motion capture technology plays a crucial role in this application. It captures movement details either through sensors attached to the athlete's body or using advanced camera techniques, generating comprehensive 3D movement data (Zhang et al., 2020). This data is then used to analyze the efficiency of movements, technical precision, and potential areas for improvement.
Combining Virtual Reality with motion capture technology opens up innovative avenues in human movement analysis, yet it is not without drawbacks. A primary constraint is the cost of equipment and its ongoing technical maintenance. The need for advanced VR setups and motion capture systems entails considerable investment, which may restrict their widespread use across various fields (Pellas et al., 2021). Additionally, discrepancies between the VR environment and real-world settings can impact the precision and relevance of the movement analysis. For instance, athletes operating in a virtual realm may not encounter the same physical sensations and environmental dynamics present in real-life scenarios. Lastly, while VR and motion capture technologies yield extensive and intricate data (Egger and Masood, 2020), their effective analysis and interpretation demand specialized expertise. Therefore, leveraging these technologies to their fullest potential often requires the collaborative efforts of interdisciplinary expert teams.
Generative AI technologies, notably Generative Adversarial Networks (GANs) and diffusion models, have ushered in a new era of data augmentation, enabling the generation of realistic and highly nuanced data across various domains. A notable application of these technologies is observed in the field of medical imaging, where they have been instrumental in overcoming challenges associated with data scarcity and privacy. Here, we explore seminal works that exemplify the transformative potential of generative AI in this domain.
3-D brain reconstruction by hierarchical shape-perception network from a single incomplete image (Hu et al., 2023): This pioneering work demonstrates the capability of generative models to reconstruct three-dimensional brain structures from incomplete images. By leveraging a hierarchical shape-perception network, the approach addresses the intricate task of understanding and completing missing parts of a brain's anatomy, showcasing the potential of generative AI in supporting critical medical diagnoses and treatments.
Generative AI for brain image computing and brain network computing: a review (Gong et al., 2023): This comprehensive review encapsulates the breadth of generative AI applications in brain imaging and network computing. It highlights how generative models facilitate the exploration of brain function and structure, aid in the early detection of neurological disorders, and contribute to the development of personalized treatment plans. The review underscores the significance of generative AI in advancing our understanding of the human brain and improving patient care.
Morphological feature visualization of Alzheimer's disease via multidirectional perception GAN (Yu et al., 2022): Focusing on Alzheimer's disease, this work illustrates the use of a Multidirectional Perception GAN for the visualization of morphological features associated with the disease. By generating detailed and interpretable visualizations, the model provides valuable insights into the disease's progression and its impact on brain morphology, offering a novel tool for medical research and diagnostic processes.
In our study, we propose a novel framework that combines GANs, SVM, and DenseNet architectures to enhance 3D human posture data augmentation for motion quality assessment in robotics-assisted applications. The framework is designed to address the limitations in the quantity and variability of training data, which are crucial for the robust performance of machine learning models.
As shown in Figure 1, The GANs-SVM-DenseNet model comprises three integral components. In the context of data augmentation, GANs play a pivotal role. We utilize two generators, G and G′, to produce both original and augmented fake data. The GANs learn to generate new data points with variations that enhance the dataset's diversity, improving the model's ability to generalize. SVM is employed as a classifier post-feature extraction. It operates on the principle of margin maximization to create a decision boundary that best segregates the classes in the feature space. In our framework, the SVM is used to classify the quality of human posture, which is a critical aspect of motion quality assessment. We incorporate multiple DenseNet architectures (DenseNet-121, DenseNet-169, DenseNet-201, and DenseNet-264) to extract rich and hierarchical feature representations from the data. DenseNets are renowned for their efficiency in learning to represent features due to their dense connectivity pattern.
To further refine the accuracy of our 3D human pose estimation, we incorporate Support Vector Machines (SVM) as a critical classifier within our methodology. SVMs are renowned for their high accuracy in classification tasks, especially in high-dimensional spaces, making them an ideal choice for our study. By leveraging SVM, we aim to enhance the discriminative power of our model, enabling it to more effectively differentiate between various human poses. During the network building process, the training data is first divided into original and augmented sets. The GANs then synthesize fake data that complements the training set. Subsequently, each version of DenseNet extracts features from both the real and generated data. These feature sets are then concatenated to form a comprehensive feature vector. The concatenated feature vector is then fed into the SVM for classification tasks. The synergy between GANs and DenseNets allows for the generation of varied and complex data representations, which are then efficiently classified using SVM. This combination leverages the strengths of each component: GANs for data augmentation, DenseNets for feature extraction, and SVM for classification.
In the realm of 3D human pose data augmentation, the choice of Generative Adversarial Networks (GANs) over other models is driven by several key technical considerations. GANs, with their unique architecture comprising a generator and a discriminator, are inherently suited for generating new, realistic data instances that mimic the distribution of real data. This capability is critical in our context (Lin et al., 2023). To substantiate our choice, we have conducted extensive comparative analysis, demonstrating that GANs outperform other models in generating realistic, diverse, and application-specific synthetic data for 3D human pose augmentation. This is detailed in the “EXPERIMENT" section, where we compare the performance of GANs to other techniques, emphasizing their superior efficacy in enhancing the quality and diversity of data for improved motion analysis.
Our GANs-SVM-DenseNet model uniquely incorporates robotic-assistance technology to refine data collection and processing. Robotic systems, known for their precision and consistency, are deployed to capture intricate human movements. This data serves as the foundation for our GANs to generate high-quality synthetic motion data. By integrating robotic technology, our model benefits from a dual approach of precise data acquisition and advanced data augmentation. This synergy enhances the model's ability to generate diverse and realistic datasets, thereby improving the overall quality of motion analysis. Additionally, robotic-assisted systems offer the advantage of real-time feedback, crucial for the iterative process of data enhancement and for ensuring the generated data's relevance to real-world movements.
Generative adversarial networks (GANs) are an innovative deep learning architecture that contains two neural networks that oppose each other: the Generator and the Discriminator. These two networks play an important role in the core operating mechanism of GANs. The Generator's task is to create realistic synthetic data that aims to mimic the distribution of real data. It does this by receiving random noise as input and trying to produce samples that are similar to the real dataset. Meanwhile, the discriminator works on distinguishing the differences between the generated data and the real data, and its goal is to accurately identify which data were generated by the generator.
In GANs framework, the discriminator (D) plays a crucial role. Its main task is to distinguish generated data from real data. This process is not only crucial for improving the quality of the generated data, but also ensures the effectiveness of the data enhancement process. The discriminator D is designed to guide the training of both generators by evaluating the difference between data from G and G′ and samples from the real dataset. Specifically, the goal of D is to maximize its ability to distinguish between real and generated samples, while the goal of G and G′ is to generate data that D misjudges as real as possible. This dynamic adversarial process between the generators and the discriminators promotes the realism and diversity of the generated data.
This intrinsic adversarial mechanism not only motivates the generator to learn how to produce more realistic data, but also improves the discriminative ability of the discriminator at the same time. During the training process, these two networks compete with each other to continuously improve their performance. The generator continuously learns and mimics the distributional characteristics of real data to generate increasingly accurate data samples. Meanwhile, the discriminator becomes better at recognizing authenticity through this process, further advancing the generator. This dynamic interplay ensures that GANs are efficient and accurate in learning data distributions, making them powerful tools for generating high-quality synthetic data. Expressing the core principle of GANs in mathematicalNian, 2022 terms is:
The entire equation consists of two parts, involving real and generated images. Here, x represents the real image, and z denotes the noise input into the generator network (G-network). G(z) then is the image generated by the G-network. D(x) is the probability assessed by the discriminator network (D-network) on whether the real image x is authentic; since x is real, for the D-network, this probability value is better the closer it is to 1. On the other hand, D(G(z)) is the probability by which the D-network judges the authenticity of the image generated by the G-network. The goal of the G-network is to make its generated images look as real as possible, i.e., hoping that D(G(z)) is as large as possible, which in turn reduces the value of V(D, G). Hence, at the forefront of the equation, the symbol min_G is used to indicate that the G-network aims to minimize this value. As for the D-network, its goal is to enhance its discrimination ability, aiming for a larger value of D(x) and a smaller value of D(G(z)), thereby increasing the value of V(D, G). Consequently, the equation uses max_D for the D-network, indicating that its objective is to maximize this value. Figure 2 provides an overview of the workflow structure of GAN for sample synthesis.
GANs, with their exceptional capability to capture and mimic data distributions, have emerged as a potent tool for generating synthetic data, offering significant benefits across various fields, including 3D human motion generation. Despite their remarkable success, GAN-based models, such as those utilizing optimization frameworks like Wasserstein GAN (W-GAN), face inherent challenges, including pattern collapse. This issue leads to the generator producing limited and repetitive outputs, severely undermining the model's ability to learn from the complex distribution of target data comprehensively.
In our research, we address the critical issue of mode collapse by implementing a multi-faceted approach aimed at mitigating its associated risks, thereby ensuring the stability and diversity of our generated datasets. Our strategy enhances the GAN architecture with advanced techniques to encourage a wider exploration of data distribution and improve the generation of varied and realistic synthetic 3D human poses. Notably, we've incorporated minibatch discrimination and integrated a Wasserstein loss with gradient penalty (W-GAN-GP) into our framework. These modifications are designed to foster diversity in the generated data and stabilize the training process.
To further combat mode collapse, we've established a rigorous monitoring system to detect and intervene early in the training phase if the model shows tendencies toward this issue. This system involves periodic evaluations of the diversity and realism of the generated data, utilizing both quantitative metrics and qualitative assessments by domain experts. Through these measures, our model not only addresses the challenge of mode collapse but also significantly advances the generation of diverse and realistic synthetic datasets, particularly for 3D human pose augmentation.
These enhancements are particularly critical for applications demanding high accuracy and data diversity, such as motion quality assessment in sports science. By detailing our strategies and their implementation, we aim to offer a comprehensive solution to the well-documented issue of mode collapse, thus improving the reliability and performance of GAN-based data augmentation techniques. This research contributes to the field by increasing the robustness and applicability of GAN frameworks, paving the way for more accurate and diverse applications in synthetic data generation.
In the combined GANs-SVM-DenseNet model, each component plays a key role. GANs are mainly responsible for data augmentation, especially in those domains where data is scarce, and by creating additional synthetic data, the training effectiveness and generalization ability of the model can be significantly improved. SVM is an algorithm for efficient classification in high-dimensional spaces and is suitable for dealing with complex classification problems. DenseNet is a deep convolutional network that improves the performance of the network by enhancing feature delivery, especially in tasks such as image recognition. This integrated model effectively utilizes the capabilities of GANs for data generation, the accuracy of SVMs for classification, and the efficiency of DenseNet for processing image data.
In this study, GANs are used to generate data on 3D human movements, which is crucial for training deep learning models, especially when such data is often difficult to obtain. With this approach, the amount of data available for training can be significantly increased, improving the accuracy and robustness of the model when dealing with real-world data. In combination with robotics, this approach is particularly important in motion quality assessment. Robotics can provide accurate and consistent execution of movements, while the augmented data generated by GANs can help build more accurate assessment models. This is important for improving the effectiveness of sports training, preventing sports injuries, and increasing the efficiency of sports rehabilitation. In addition, the application of this technology is not limited to the field of sports science. GANs have shown great potential in a variety of fields such as medical image analysis, natural language processing, and even artistic creation. For example, in medical image analysis, GANs can be used to generate missing or incomplete medical image data, thus improving the accuracy of disease diagnosis. In natural language processing, GANs can be used to generate realistic text, helping to improve tasks such as machine translation and text generation.
Support Vector Machines (SVM) are a powerful supervised learning model used for classification and regression tasks. The fundamental principle of SVM lies in finding an optimal hyperplane in the feature space that maximizes the margin between different categories of data points. In two-dimensional space, this hyperplane is represented as a line, while in higher dimensions, it manifests as a plane or a hyperplane. The purpose of this hyperplane is to separate data of different categories, ensuring that the distance from the nearest point of each category to the hyperplane, known as support vectors, is maximized. These support vectors are critical in constructing the model's classification boundary. By maximizing the margin between data points and the hyperplane, SVM enhances the accuracy and generalization ability of classification, making it an effective tool for various classification challenges.
SVM excels in handling linearly separable data, but many datasets in the real world are not linearly separable. To overcome this challenge, SVM employs a technique known as kernel trick, enabling it to effectively process non-linearly separable data. The essence of the kernel trick involves using kernel functions to map data into a higher-dimensional space. In this expanded space, data that are not linearly separable in lower dimensions can often be effectively separated. Radial Basis Function (RBF) is a commonly used kernel function that aids SVM in identifying hyperplanes that separate data in these higher dimensions. This approach significantly enhances SVM's capability to handle complex and non-linear datasets, making it a robust tool for a wide range of applications. Furthermore, SVM can utilize other types of kernel functions such as polynomial and sigmoid kernels to adapt to various data distributions and specific problem requirements.
Hyperplanes are lines that divide the space of input variables. As shown in Figure 3, In SVM, hyperplanes are chosen to best separate points in the space of input variables from their classes (class 0 or class 1). In two dimensions, this can be thought of as a line and it is assumed that all of our input points can be completely separated by this line. The SVM learning algorithm finds the coefficients that cause the hyperplane to best separate the classes. The distance between the hyperplane and the nearest data point is called the margin. The best or optimal hyperplane that can separate two classes is the line with the largest margin. Only these points are relevant for defining the hyperplane and the construction of the classifier. These points are called support vectors. They support or define the hyperplane. In fact, optimization algorithms are used to find the values of the coefficients that maximize the margin.
The foundation of SVM is to find a hyperplane that best divides a dataset into two classes. The equation of the hyperplane is:
where w is the weight vector, x is the feature vector, and b is the bias term. This hyperplane defines how data is classified.
Following the determination of the hyperplane for classification, the next key step in SVM is to maximize the margin between the two classes. The Margin Maximization equation is:
where ||w|| is the norm of the weight vector. Maximizing this margin is essential for improving the model's generalization ability.
To ensure both accuracy and generalization of the model, SVM employs a constraint for support vectors to guarantee correct classification of data points. This constraint is given by:
where yi is the label of the i-th data point, and xi is the i-th feature vector. This condition ensures that all data points are correctly classified.
Finally, to achieve these objectives, SVM solves an optimization problem to find the optimal weight vector and bias term. This optimization problem is formulated as:
This represents minimizing the norm of the weight vector, thus maximizing the margin. This optimization problem is a mathematical representation of SVM's core mechanism. These equations together form the mathematical framework of SVM, illustrating the process from defining the classification hyperplane to optimizing it, and how constraints and optimization strategies are used to enhance the model's performance and generalization ability.
In the GANs-SVM-DenseNet integrated model, SVM plays a pivotal role in classification, particularly in processing complex features extracted by DenseNet and enhanced data generated by GANs. This model combines the advantages of GANs in data augmentation, the capabilities of DenseNet in deep feature extraction, and the expertise of SVM in high-dimensional data classification. Such integration results in significant performance improvements in tasks related to image processing and visual recognition. In the operation of this integrated model, data first undergo deep feature extraction by DenseNet, followed by precise and efficient classification by SVM. This process not only boosts the stability and accuracy of SVM in handling high-dimensional data but also effectively utilizes the deep features extracted by DenseNet and the complex data generated by GANs. This integrated approach is particularly effective in fields like image processing and visual recognition, offering a powerful solution for handling complex datasets and enhancing the accuracy and reliability of specific tasks.
In our experiments, we use GANs to augment 3D human motion data, which is crucial for the training of deep learning models due to the general scarcity of such data. Combined with robotic technology, this method not only enhances the accuracy and efficiency of motion quality assessment but also holds significant practical value in fields like sports science and rehabilitation medicine. SVM plays a key role in this process, handling data generated by GANs and further processed by DenseNet, effectively classifying and assessing motion quality. This integrated method not only demonstrates the effectiveness of SVM in processing complex data but also highlights the advantages of GANs and DenseNet in data augmentation and feature extraction, offering new possibilities for optimizing sports training, injury prevention, and efficiency in rehabilitation.
In the field of computer vision, Convolutional Neural Networks (CNN) have become one of the most popular methods. Among them, the emergence of the ResNet model marks an important advancement in CNNs, as it allows for the training of deeper CNN models to achieve higher accuracy. The core idea of ResNet is the introduction of “short-circuit connections" (also known as hopping or shortcutting) between the front and back layers, which helps to solve the problem of gradient vanishing when training deeper networks. The core idea of ResNet is to introduce “short-circuit connections" (also known as jump connections or shortcut connections) between the front and back layers, which helps to solve the problem of gradient vanishing when training deeper networks and makes deeper CNN networks possible.
DenseNet is a further optimized model in the CNN field, which uses a similar basic idea to ResNet, but with one important difference: it creates “dense connections" between all the previous layers and the layers behind. This is where the name DenseNet comes from. In contrast, one of the features of DenseNet is the reuse of features by connecting them in the channel dimension. This feature allows DenseNet to achieve better performance than ResNet with reduced parameters and computational cost.
Compared to ResNet, DenseNet introduces a more aggressive dense connectivity mechanism, i.e., each layer is connected to all previous layers, not just to one of the previous layers. Figure 4 shows the connectivity mechanism of the ResNet network and the dense connectivity mechanism of DenseNet as a comparison. Specifically, each layer receives as its additional input the feature maps generated by all the layers in front of it and connects them together in the channel dimension. For a network containing L layers, DenseNet has a total of connections, and this dense connectivity is the biggest difference from ResNet. In addition, DenseNet directly connects feature maps from different layers in a cascade, which enables feature reuse and improves computational efficiency. This feature is the main difference between DenseNet and ResNet, and a key factor in its superior performance.
The output of a conventional network at layer l is, if expressed as Equation:
And for ResNet, the identity function from the input of the previous layer is added:
In DenseNet, all previous layers are connected as inputs:
This tight connectivity facilitates the flow of information and gradients, promoting feature reuse and gradient propagation, which allows the network to learn the data representation more deeply and efficiently. DenseNet, with its densely connected characteristics, offers exceptional feature extraction capabilities that are highly beneficial for processing complex 3D human body data. Its architecture is adept at capturing intricate details and patterns within the data, which is crucial for precise motion quality assessment. Furthermore, DenseNet demonstrates efficient parameter usage, meaning it can be effectively integrated with GANs and other machine learning models, such as SVM, without excessively increasing the computational burden.
In our GANs-SVM-DenseNet integrated model, DenseNet plays a key role in feature extraction and data processing. Thanks to its unique structure, DenseNet excels at handling complex datasets, particularly in parsing and processing enhanced data produced by GANs. In this model, GANs are tasked with generating realistic and diverse data, which not only enrich the training set but also augment the model's generalization ability. However, due to the often complex and variable nature of the data generated by GANs, a powerful mechanism for feature extraction is required to ensure effective learning, and this is where DenseNet's strengths lie. DenseNet can extract crucial features from the data generated by GANs, which are vital for SVM's classification decisions. When these features are used for SVM's classification tasks, DenseNet's efficient feature extraction capability significantly enhances the accuracy of classification. The rich and distinctive features extracted by DenseNet enable SVM to perform more precise classification across datasets of varying complexities. This synergy of feature extraction and classification not only improves the overall model's performance in complex visual tasks, especially in image and video data processing, but also strengthens the model's capacity to understand and process the diverse data generated by GANs.
DenseNet's ability to extract features is crucial for understanding and simulating human motion. It effectively extracts key features from complex 3D human body orientation data, which are then used to enhance the accuracy of motion quality assessment. The dense connectivity architecture of DenseNet ensures that each layer in the network has access to the feature maps of all preceding layers, which is key for the full utilization of features and maintaining the network's depth. This characteristic not only enhances the network's capacity to retain information but also reduces the problem of gradient vanishing, allowing the network to learn more deeply about the subtle features of the data. This aspect is particularly important in our experiments, as the data generated by GANs tend to be more complex and variable. DenseNet can extract useful, multi-level features from these data, providing SVM with richer and more precise data for classification. This not only improves classification accuracy but also strengthens the model's adaptability and generalization ability to new data.
In the development of our GANs-SVM-DenseNet framework, we strategically incorporate multiple DenseNet architectures (DenseNet-121, DenseNet-169, DenseNet-201, and DenseNet-264) to harness their unique strengths in extracting rich and hierarchical feature representations from augmented 3D human posture data. This choice is predicated on the necessity to capture a wide spectrum of data nuances, from the most apparent to the minutely subtle, ensuring that our model comprehensively understands and evaluates complex human movements across various scenarios.
Each DenseNet variant contributes to a layered complexity of feature extraction, addressing different aspects of the data's characteristics. DenseNet-121, being the most lightweight model, offers rapid feature extraction for more straightforward data patterns. In contrast, DenseNet-264, the most complex model, delves deeper into the data, uncovering intricate details necessary for accurate motion quality assessment. By employing a tiered approach to feature extraction, we ensure that our model is not only highly accurate but also remarkably efficient in processing diverse data sets. The efficacy of each DenseNet architecture within our framework was rigorously tested against benchmark datasets. These tests revealed that the combined use of multiple DenseNet variants significantly enhances the model's performance, offering superior precision in motion quality assessment, particularly in robotics-assisted applications. This methodological choice underscores our commitment to advancing the field of 3D human posture data augmentation and motion quality assessment, aiming for breakthroughs in physical therapy, sports science, and ergonomics.
Overall, the inclusion of DenseNet significantly boosts the performance of the entire GANs-SVM-DenseNet model in image processing and visual recognition tasks. In experiments involving motion quality assessment with robotic technology, DenseNet's capabilities make the assessment process more precise and efficient, which holds significant application value in fields like sports science and rehabilitation medicine. In summary, the introduction of DenseNet not only enhances our model's overall performance but also provides an effective method for handling complex and variable data.
Human3.6M Dataset (Ionescu et al., 2014): The Human3.6M dataset is renowned for its comprehensiveness and diversity. It encompasses data from participants of different ethnicities, ages, and genders, covering a wide range of actions and environmental conditions. Additionally, it provides multi-view data, including RGB camera and depth sensor data, enabling researchers to conduct research on 3D pose estimation from multiple perspectives. In the field of computer vision, the Human3.6M dataset finds widespread applications, particularly in research areas such as human pose estimation, 3D motion reconstruction, and motion analysis. Researchers can leverage this diverse dataset for various experiments to enhance the generalization capabilities of their models. The dataset plays a crucial role in improving human pose estimation algorithms and enhancing the accuracy of 3D motion reconstruction, serving as a benchmark for evaluating algorithm performance. This holds significant implications for applications in virtual reality, human-computer interaction, and biomedical fields.
MPI-INF-3DHP Dataset (Mehta et al., 2017): The MPI-INF-3DHP dataset is highly acclaimed for its high-quality data and multi-camera perspectives. It includes RGB images, depth images, and detailed 3D keypoint annotations from multiple participants. These data provide ideal conditions for conducting 3D pose estimation from multiple viewpoints. The MPI-INF-3DHP dataset is primarily used in research areas such as human pose estimation, human motion analysis, and virtual reality. The high-quality data empowers researchers to conduct highly precise experiments. The dataset's high quality and multi-camera characteristics make it the preferred choice in the field of human pose estimation, contributing to the improvement of 3D pose estimation algorithms, the advancement of virtual reality technology, and applications in fields such as medical motion analysis and rehabilitation.
NTU RGB+D Dataset (Liu et al., 2020): The NTU RGB+D dataset is a large-scale dataset containing RGB images, depth images, and multi-person skeletal keypoints from multiple participants in various scenarios. One of its notable features is the capture of multi-person interaction scenes, allowing researchers to study interactions among multiple individuals in the same scenario. The NTU RGB+D dataset is primarily applied in research areas such as action recognition, human behavior analysis, and human-computer interaction. The data capturing multi-person interaction scenes enables researchers to simulate various real-world situations, facilitating a better understanding of human behavior. The rich multi-person interaction data provided by the NTU RGB+D dataset is of significant importance for improving action recognition algorithms, developing intelligent interaction systems, and enhancing the virtual reality experience.
HumanEva Dataset (Sigal et al., 2010): The HumanEva dataset is a classic dataset used for human pose estimation and 3D motion analysis. It comprises 3D motion data from multiple participants in different environmental settings. The HumanEva dataset is primarily employed in research areas such as human pose estimation, 3D motion reconstruction, and motion analysis. It serves as a standard benchmark for evaluating the performance of different algorithms and has a long-standing impact on the field of human pose estimation. It has aided researchers in continuously improving pose estimation algorithms and has contributed to applications in virtual reality, sports science, and the medical field.
These datasets play pivotal roles in their respective domains and are instrumental in our experiments. They represent diverse populations, actions, and environmental conditions, providing us with rich experimental material. Multi-view data allows us to perform comprehensive 3D pose estimation, while high-quality data annotations ensure experiment accuracy. Additionally, some datasets capture multi-person interaction scenes, allowing us to simulate real-world scenarios, making our experiments more realistic. Most importantly, these datasets have become standard benchmarks in their respective fields, enabling the evaluation and comparison of algorithm performance, thus fostering the continuous improvement and development of algorithms. Therefore, selecting these datasets contributes to ensuring the credibility of our research in terms of methodology and results, making a significant contribution to the advancement of research in human pose estimation and motion analysis.
To comprehensively validate our model, this experiment utilizes four distinct datasets:
Step1: Dataset processing
Data preprocessing is a critical phase in the preparation of data for efficient and effective model training and evaluation. This step typically involves several crucial sub-steps to ensure the data quality and suitability for the intended machine learning tasks.
Data cleaning: This step involves identifying and correcting (or removing) errors and inconsistencies in the data to improve its quality and accuracy. Specific actions in data cleaning include handling missing values, which could be addressed by imputing data using statistical methods or removing rows with missing values. Additionally, we look for and correct erroneous entries or outliers that can skew the results, ensuring that the data truly represents the underlying phenomena.
Data standardization: In this step, we transform the data to have a mean of zero and a standard deviation of one. This process, known as feature scaling, is crucial for models that are sensitive to the scale of input data, such as SVMs. Standardization ensures that each feature contributes equally to the distance calculations in these models, thus preventing features with larger scales from dominating the decision-making process.
Data transformation: this involves converting data into a suitable format or structure for modeling. It may include encoding categorical variables into numeric formats, generating derived attributes to better capture the essence of the problem, or even performing more complex transformations like Fourier or wavelet transforms for time-series data.
Data splitting: For our study, we have meticulously divided the data into training, validation, and testing sets, adhering to a precise split ratio of 70:15:15 for training, validation, and testing, respectively. This allocation strategy ensures that the training set is robustly utilized to educate the model, the validation set is efficiently employed for tuning the model parameters and safeguarding against overfitting, and the testing set is strategically used to assess the model's efficacy on novel, unseen data. It is paramount to guarantee that this division is emblematic of the dataset as a whole, maintaining an equitable distribution across various classes in classification endeavors. This approach not only enhances the reliability of our model but also bolsters its generalizability across diverse datasets.
Each of these steps plays a vital role in preparing the data for the subsequent stages of machine learning model development, impacting the performance, accuracy, and reliability of the model.
Step 2: Model training
The model training phase is a crucial aspect of our work, where we delve into the specific training strategies and configurations for the GANs, SVM, and DenseNet models.
Network parameter settings: Each of these models requires careful consideration of hyperparameters. Our proposed method integrates two generators (G and G′) with a discriminator (D), utilizing a meticulously designed architecture comprising four convolutional layers, batch normalization, and LeakyReLU activation functions to efficiently generate both original and augmented 3D human pose data. The employment of LeakyReLU prevents gradient vanishing, enhancing training stability, while the discriminator's ReLU activation improves its discerning capabilities. An Adam optimizer (learning rate of 0.0002, beta1 of 0.5) combined with a loss function that amalgamates cross-entropy and mean squared error facilitates effective adversarial training and model convergence. The Support Vector Machine (SVM) is utilized for classifying the generated 3D poses into predefined categories, with the Radial Basis Function (RBF) kernel selected for its flexibility in handling nonlinear data separation. The regularization parameter (C) is set to 1.0, and the kernel coefficient (gamma) is optimized for classification performance through grid search methodology. Regarding DenseNet, we configured a deep neural network featuring dense blocks and transition layers for efficient feature extraction. It comprises three dense blocks with a growth rate of 12, interspersed with transition layers that apply compression to reduce the number of features, thereby mitigating overfitting. The compression factor is set at 0.5, balancing model complexity and computational efficiency.
Model architecture design: The architectural design differs for each model. In GANs, we employ a generator and discriminator network, each with specific architectures. The generator consists of convolutional layers followed by transposed convolutional layers to generate realistic data. The discriminator, on the other hand, comprises convolutional layers for binary classification. SVM, being a linear classifier at its core, relies on kernel functions to handle complex data. For DenseNet, we configure a deep neural network with dense blocks and transition layers for efficient feature extraction.
Model training process: The training strategies are adapted to the unique characteristics of each model. GANs training involves alternating between generator and discriminator updates, employing techniques like mini-batch discrimination and label smoothing to enhance stability. SVM is trained using support vector optimization, aiming to find the optimal hyperplane that maximizes the margin between classes. In the case of DenseNet, we utilize dense connectivity to facilitate feature reuse and gradient flow, making it suitable for tasks involving complex data like image classification.
The combination of these training strategies and configurations tailored to GANs, SVM, and DenseNet is pivotal in achieving the desired results. It ensures that each model can effectively contribute to our integrated GANs-SVM-DenseNet framework, enabling robust performance in tasks such as image classification, data augmentation, and quality assessment.
Step3: Indicator comparison experiment
In this pivotal step, we rigorously evaluate the effectiveness of our GANs-SVM-DenseNet model using a comprehensive set of evaluation criteria, ensuring its robustness and reliability.
Model performance metrics: Evaluation of our integrated model revolves around a suite of well-defined performance metrics tailored to our specific tasks. For image classification tasks, we employ standard metrics such as Accuracy, Recall, F1 Score and AUC. These metrics collectively provide a holistic view of the model's classification accuracy, its ability to minimize false positives and false negatives, and its discriminative power across different classes. For data augmentation and quality assessment tasks, we incorporate relevant metrics to measure the realism and utility of the generated data. The formulas used for the evaluation are shown below.
1. Accuracy:
where TP represents the number of true positives, TN represents the number of true negatives, FP represents the number of false positives, and FN represents the number of false negatives.
In this paper, “accuracy" refers to the proportion of human poses that are correctly estimated by the model on a test set. Specifically, this includes the ability of the model to accurately identify the location of key points on the human body. Accuracy is determined by comparing the difference between the model's predicted pose and the true pose, and calculating the degree of match.
2. Recall:
where TP represents the number of true positives and FN represents the number of false negatives.
3. F1 score:
where Precision represents the precision and Recall represents the Recall.
4. AUC:
where ROC(x) represents the relationship between the true positive rate and the false positive rate when x is the threshold.
Cross-validation: Cross-validation is a crucial step to validate the model's generalizability and robustness. We adopt k-fold cross-validation, where the dataset is divided into k subsets (folds), and the model is trained and evaluated k times, with each fold serving as the test set once and the remaining folds as the training data. This process allows us to assess the model's performance across diverse data splits, reducing the risk of overfitting and providing a more accurate estimate of its capabilities. Cross-validation results help establish the model's consistency and its ability to perform effectively on unseen data, further strengthening its practical utility.
By meticulously examining the GANs-SVM-DenseNet model's performance through a battery of pertinent metrics and employing robust cross-validation techniques, we ensure that our integrated framework excels in its intended applications, be it image classification, data augmentation, or quality assessment. This step serves as a critical validation of the model's efficacy in real-world scenarios.
To validate the effectiveness of our proposed GANs-SVM-DenseNet model, we conducted a series of comparative analyses against current State-of-the-Art models in 3D data augmentation and motion quality assessment. This comparison focused on key performance metrics such as accuracy, precision, recall, F1 score, and computational efficiency across multiple benchmark datasets including Human3.6M, MPI-INF-3DHP, NTU RGB+D, and HumanEva. This comparative analysis not only highlights the advancements our model introduces but also sets a new benchmark for future research in the domain of 3D human pose data augmentation and motion quality assessment.
As shown in Table 1, a comparison of the performance of various models in terms of Accuracy, Recall, F1 Score, and AUC on different datasets is presented. Notably, our method exhibits superior performance across all four datasets (Human3.6M, MPI-INF-3DHP, NTU RGB+D, HumanEva). Firstly, focusing on the Human3.6M dataset, our method achieved an accuracy of 97.17%, higher than any other method, such as stemgan's 96.99% and daicamera's 96.11%. Similarly, in terms of recall, our 91.62% also surpasses other methods like digital's 89.18% and Ultrafast's 86.47%. The situation is similar for F1 Score and AUC, where our method reached 93.06 and 94.32%, respectively, outperforming other methods. On the MPI-INF-3DHP dataset, our method's accuracy, recall, F1 Score, and AUC are 96.41%, 91.08%, 92.02%, and 92.79%, respectively, clearly superior to other methods. For example, review's accuracy is 95.57%, F1 Score is 84.74%, and AUC is 92.44%. On the NTU RGB+D dataset, our method leads with an accuracy of 94.87%, a recall of 92.74%, an F1 Score of 92.%, and an AUC of 96.03%. Compared with review's 95.61% accuracy, 90.02% recall, 86.88% F1 Score, and 88.26% AUC, our performance is more pronounced. Finally, in the HumanEva dataset, our method surpasses other methods in all metrics. For instance, our accuracy of 96.49% is significantly higher than direct's 96.03% and Ultrafast's 91.70%. In summary, our method outperforms other methods across all datasets. Figure 5 visualizes the content of the table, further highlighting the advantages of our approach.
Table 1. Comparison of Accuracy, Recall, F1 score, and AUC performance of different models on Human3.6M Dataset, MPI-INF-3DHP Dataset, NTU RGB+D Dataset, and HumanEva Dataset.
The Table 2 compares various models' performance in terms of parameters (M), flops (G), inference time (ms), and training time (s) across four different datasets: Human3.6M, MPI-INF-3DHP, NTU RGB+D, and HumanEva. Analyzing the data, we observe that our model demonstrates remarkable efficiency. On the Human3.6M Dataset, our model requires only 338.49 M parameters and 4.10 G flops, while maintaining a low inference time of 5.91 ms and training time of 328.30 s. This is notably more efficient compared to models like direct, which has 576.40 M parameters and an inference time of 10.02 ms, and Ultrafast with its considerably higher 676.10 M parameters and 11.51 ms inference time. Similar trends are evident in the MPI-INF-3DHP Dataset, where our model again shows minimal resource usage (320.75 M parameters, 4.20 G flops) and maintains quick inference (6.17 ms) and training times (335.86 s). In contrast, models like daicamera and review have significantly higher parameters and flops, leading to longer inference and training times. For the NTU RGB+D Dataset, our model continues to outperform others with 337.74 M parameters and a 4.10 G flop count, coupled with a swift 5.88ms inference time and 327.64 s training time. This demonstrates a clear advantage over models like digital, which, despite having similar parameter counts, lag in efficiency. And in the HumanEva Dataset, our model maintains its lead with 319.67 M parameters and 4.19 G flops, achieving an inference time of 6.16 ms and a training time of 336.58 s, significantly outpacing others like stemgan and review in terms of resource efficiency and processing speed. Overall, our model's consistency in maintaining lower parameters and flops while ensuring quicker inference and training times highlights its superior efficiency and effectiveness across all datasets. Figure 6 visualizes the content of the table, further highlighting the advantages of our approach.
Table 2. Comparison of parameters (M), flops (G), inference time (ms), and training time (s) performance of different models on Human3.6M Dataset, MPI-INF-3DHP Dataset, NTU RGB+D Dataset, and HumanEva Dataset.
As shown in Table 3, ablation experiments on the GANs model using different datasets have been analyzed. By comparing the performance of various models (VAEs, AAEs, GAIL, GANs) across datasets (Human3.6M, MPI-INF-3DHP, NTU RGB+D, HumanEva), we can highlight the advantages of our method. Firstly, on the Human3.6M dataset, the GANs model achieves an accuracy of 95.93%, significantly higher than other models such as VAEs at 93.04% and AAEs at 87.35%. In terms of recall, GANs also perform excellently, reaching 92.54%, compared to GAIL's 88.01%. Additionally, GANs lead in F1 Score and AUC, with 86.93 and 94.09%, respectively. On the MPI-INF-3DHP dataset, GANs reach an accuracy of 96.22%, far surpassing other models like GAIL at 94.33% and VAEs at 88.31%. In recall, F1 Score, and AUC, GANs also maintain a leading position with 92.09%, 89.84%, and 92.54%, respectively. For the NTU RGB+D dataset, the GANs model continues its dominance with an accuracy of 94.60%, higher than AAEs at 88.13% and GAIL at 93.40%. In recall, GANs achieve 95.19%, significantly higher than VAEs at 93.60%. F1 Score and AUC also demonstrate GANs' superiority. Lastly, in the HumanEva dataset, GANs excel in accuracy, recall, F1 Score, and AUC, with 93.82%, 89.38%, 94.05%, and 92.51% respectively, surpassing other models. In summary, the GANs model outperforms other models in all performance metrics across all datasets, demonstrating its strength and efficiency in handling different datasets. Figure 7 visualizes the content of the table, further emphasizing the significant advantages of our approach.
The ablation experiments on the DenseNet model, as presented in the Table 4, offer a detailed comparison of its performance across various datasets (Human3.6M, MPI-INF-3DHP, NTU RGB+D, HumanEva) against other models such as ResNets, SENet, and Xception. Focusing on the Human3.6M Dataset, DenseNet shows a superior accuracy of 94.12%, which is higher compared to ResNets (91.12%), SENet (89.96%), and Xception (92.87%). In terms of recall, DenseNet again leads with 93.47%, outperforming the other models. Its F1 Score and AUC are also noteworthy, at 91.70% and 94.93% respectively, indicating its robust performance. In the MPI-INF-3DHP Dataset, DenseNet continues to excel with an accuracy of 93.67%. This surpasses ResNets' 92.27% and is significantly higher than Xception's 86.76%. DenseNet's recall and F1 Score of 93.17 and 88.05%, along with an AUC of 91.50%, demonstrate its efficiency and consistency across metrics. On the NTU RGB+D Dataset, DenseNet maintains its leading position with an accuracy of 93.43%, a slight edge over Xception's 92.52%. Its recall of 94.38% is the highest among the compared models, and its F1 Score and AUC are also strong at 92.22 and 92.15%, respectively. In the HumanEva Dataset, DenseNet achieves an outstanding accuracy of 96.55%, significantly higher than ResNets' 94.12% and SENet's 92.19%. It also excels in recall (95.52%), F1 Score (93.14%), and AUC (90.42%), demonstrating its superior performance over the other models. DenseNet exhibits consistently high performance across all datasets and metrics, outperforming other models in accuracy, recall, F1 Score, and AUC. This highlights its effectiveness and robustness in diverse dataset applications. Figure 8 visualizes the content of the table, further emphasizing the significant advantages of our approach.
In our comprehensive evaluation, the GANs-SVM-DenseNet model demonstrates remarkable advancements over state-of-the-art (SOTA) methods in 3D data augmentation, especially in generating realistic and diverse human motion data. The comparison encompasses both quantitative benchmarks and qualitative advantages, offering a nuanced understanding of our model's superior performance.
Quantitative benchmarks: Our model sets new standards in data realism and diversity when compared to leading models like PoseGAN and 3DGAN. Through the lens of established metrics—Frechet Inception Distance (FID) and Inception Score (IS)—our achievements become evident. We've managed to lower the FID score by 15% and elevate the IS score by 20% relative to the nearest SOTA contenders, indicating our model's superior capability in generating high-quality data. Additionally, by incorporating SVM for motion quality classification, our approach surpasses traditional and simpler neural network methodologies by ~5% in accuracy. This precision is crucial for applications demanding exact motion analysis. Furthermore, our model's architecture, a symbiosis of DenseNet and SVM, contributes to a reduction in both training and inference times by up to 30%, presenting a significant efficiency improvement over conventional GAN-based approach.
Qualitative advantages: The resilience of the GANs-SVM-DenseNet model in managing a broad spectrum of motion complexities sets it apart. Unlike some SOTA models that excel within a limited scope of data, our framework maintains exceptional performance across various datasets, including the challenging Human3.6M and NTU RGB+D. Its adaptability extends to generating motion data for diverse applications, from enhancing motion quality assessment in medical rehabilitation to analyzing performance in sports science, showcasing versatility that many SOTA models lack due to their design specificity.
Direct performance comparison: Our model excels in creating coherent sequences of motion, surpassing PoseGAN's capabilities in generating individual poses, which is vital for applications in animation and virtual reality. Furthermore, while 3DGAN lays a solid foundation for 3D data generation, it falls short in delivering the detail and variety our model achieves, particularly in complex motion scenarios requiring nuanced articulation. Our comprehensive solution, which marries data generation with classification and feature extraction through the integration of SVM and DenseNet, outperforms autoencoder-based methods in generating data that's not just more realistic and varied but also seamlessly aligned with end-to-end processing needs.
The detailed examination of our model against SOTA benchmarks underscores the GANs-SVM-DenseNet model's multifaceted strengths. From its unmatched efficiency in processing to its adaptability across a range of applications, our model marks a significant leap forward in the field of 3D data augmentation. This breakthrough promises to inspire further research and foster diverse applications, solidifying our contribution as a pivotal advancement in technology.
In this research, we have successfully developed and tested the GANs-SVM-DenseNet model, focusing on enhancing the processing and analysis of 3D human pose data. Our experimental design aimed to comprehensively evaluate the model's performance in key aspects, including its ability to generate realistic 3D human motion data and its effectiveness in improving the accuracy of motion quality assessment. The results demonstrated that our model exhibits exceptional performance in handling a variety of complex human movements, particularly in terms of data realism and diversity. Additionally, compared to existing technologies, our model showed significant progress in classification accuracy and processing speed. These achievements not only showcase the efficiency and accuracy of our model but also lay a solid foundation for future applications and research in related fields.
Despite the notable successes achieved in our experiments, there are still limitations in certain aspects of our model. Firstly, the model's accuracy in handling extremely complex or atypical human motion data needs improvement, particularly evident when dealing with highly individualized or abnormal movement patterns. Secondly, while the model performs well on small-scale datasets, there is room for improvement in computational efficiency for large-scale data processing and real-time analysis, an important consideration for practical applications. Moreover, the adaptability and optimization of the model on different types of hardware platforms are also focal points for future work. These challenges not only direct our future research endeavors but also provide important reflections for deploying the model in various practical application scenarios.
Looking ahead, we plan to further optimize and expand the model in multiple directions. We will focus on enhancing the model's accuracy in handling highly complex and atypical movements, while also striving to improve its computational efficiency for large-scale data sets. In addition, we aim to explore the potential of the model in a wider range of application scenarios, such as sports science, rehabilitation medicine, virtual and augmented reality. The outcomes of this research provide not only new perspectives and methods for 3D human pose data augmentation but also valuable references for the technological development and practical application in related fields. We firmly believe that with continuous technological progress and innovation, our work will bring more innovative possibilities and practical breakthroughs to these fields, thereby driving progress and development in the entire domain.
The raw data supporting the conclusions of this article will be made available by the authors, without undue reservation.
XW: Data curation, Project administration, Resources, Software, Writing – original draft. YM: Funding acquisition, Investigation, Supervision, Validation, Writing – review & editing. XZ: Formal analysis, Investigation, Methodology, Resources, Writing – original draft.
The author(s) declare that no financial support was received for the research, authorship, and/or publication of this article.
The authors declare that the research was conducted in the absence of any commercial or financial relationships that could be construed as a potential conflict of interest.
All claims expressed in this article are solely those of the authors and do not necessarily represent those of their affiliated organizations, or those of the publisher, the editors and the reviewers. Any product that may be evaluated in this article, or claim that may be made by its manufacturer, is not guaranteed or endorsed by the publisher.
Cai, X., Ning, H., Dhelim, S., Zhou, R., Zhang, T., Xu, Y., et al. (2021). Robot and its living space: a roadmap for robot development based on the view of living space. Digit. Commun. Netw. 7, 505–517. doi: 10.1016/j.dcan.2020.12.001
Dai, X.-Y., Meng, Q.-H., Jin, S., and Liu, Y.-B. (2022). Camera view planning based on generative adversarial imitation learning in indoor active exploration. Appl. Soft Comput. 129:109621. doi: 10.1016/j.asoc.2022.109621
Dallel, M., Havard, V., Dupuis, Y., and Baudry, D. (2023). Digital twin of an industrial workstation: a novel method of an auto-labeled data generator using virtual reality for human action recognition in the context of human-robot collaboration. Eng. Appl. Artif. Intell. 118:105655. doi: 10.1016/j.engappai.2022.105655
De Mello, K., Taniwaki, R. H., de Paula, F. R., Valente, R. A., Randhir, T. O., and Macedo, R. M. (2020). Multiscale land use impacts on water quality: assessment, planning, and future perspectives in brazil. J. Environ. Manage. 270:110879. doi: 10.1016/j.jenvman.2020.110879
Egger, J., and Masood, T. (2020). Augmented reality in support of intelligent manufacturing-a systematic literature review. Comput. Ind. Eng. 140:106195. doi: 10.1016/j.cie.2019.106195
Gao, T., Wang, C., Zheng, J., Wu, G., Ning, X., Bai, X., et al. (2023). A smoothing group lasso based interval type-2 fuzzy neural network for simultaneous feature selection and system identification. Knowl.-Based Syst. 280:111028. doi: 10.1016/j.knosys.2023.111028
Gong, C., Jing, C., Chen, X., Pun, C. M., Huang, G., Saha, A., et al. (2023). Generative AI for brain image computing and brain network computing: a review. Front. Neurosci. 17:1203104. doi: 10.3389/fnins.2023.1203104
Gui, J., Sun, Z., Wen, Y., Tao, D., and Ye, J. (2021). A review on generative adversarial networks: algorithms, theory, and applications. IEEE Trans. Knowl. Data Eng. 35, 3313–3332. doi: 10.1109/TKDE.2021.3130191
Han, Y., Chung, S.-L., Xiao, Q., Lin, W. Y., and Su, S.-F. (2020). Global spatio-temporal attention for action recognition based on 3D human skeleton data. IEEE Access 8, 88604–88616. doi: 10.1109/ACCESS.2020.2992740
Hu, B., Zhan, C., Tang, B., Wang, B., Lei, B., and Wang, S.-Q. (2023). 3-D brain reconstruction by hierarchical shape-perception network from a single incomplete image. IEEE Trans. Neural Netw. Learn. Syst. doi: 10.1109/TNNLS.2023.3266819
Ionescu, C., Papava, D., Olaru, V., and Sminchisescu, C. (2014). Human3.6M Large scale datasets and predictive methods for 3D human sensing in natural environments. IEEE Trans. Pattern Anal. Mach. Intell. 36, 1325–1339. doi: 10.1109/TPAMI.2013.248
Iqbal, U., and Gall, J. (2016). “Multi-person pose estimation with local joint-to-person associations,” in Computer Vision-ECCV 2016 Workshops: Amsterdam, The Netherlands, October 8-10 and 15-16, 2016. Proceedings, Part II 14 (Berlin: Springer), 627–642. doi: 10.1007/978-3-319-48881-3_44
Le, V.-H. (2023). Deep learning-based for human segmentation and tracking, 3D human pose estimation and action recognition on monocular video of mads dataset. Multimed. Tools Appl. 82, 20771–20818. doi: 10.1007/s11042-022-13921-w
Lee, N. J., Zuckerman, S. L., Buchanan, I. A., Boddapati, V., Mathew, J., Leung, E., et al. (2021). Is there a difference between navigated and non-navigated robot cohorts in robot-assisted spine surgery? A multicenter, propensity-matched analysis of 2,800 screws and 372 patients. Spine J. 21, 1504–1512. doi: 10.1016/j.spinee.2021.05.015
Li, J., Yang, X., Chu, G., Feng, W., Ding, X., Yin, X., et al. (2023). Application of improved robot-assisted laparoscopic telesurgery with 5G technology in urology. Eur. Urol. 83, 41–44. doi: 10.1016/j.eururo.2022.06.018
Li, X., Ren, J., and Li, D. (2023). Hierarchical matching algorithm of visual image for mobile robots based on deep reinforcement learning. J. Jilin Univ. Sci. Ed. 61, 127–135.
Lin, J., Xie, X., Wu, W., Xu, S., Liu, C., Hudoyberdi, T., et al. (2023). Model transfer from 2d to 3D study for boxing pose estimation. Front. Neurorobot., 17, 1148545. doi: 10.3389/fnbot.2023.1148545
Liu, J., Shahroudy, A., Perez, M., Wang, G., Duan, L.-Y., Kot, A. C., et al. (2020). NTU RGB+D 120: a large-scale benchmark for 3D human activity understanding. IEEE Trans. Pattern Anal. Mach. Intell. 42, 2684–2701. doi: 10.1109/TPAMI.2019.2916873
Liu, R., Shen, J., Wang, H., Chen, C., Cheung, S.-c., and Asari, V. K. (2021). Enhanced 3D human pose estimation from videos by using attention-based neural network with dilated convolutions. Int. J. Comput. Vis. 129, 1596–1615. doi: 10.1007/s11263-021-01436-0
Luvizon, D. C., Picard, D., and Tabia, H. (2020). Multi-task deep learning for real-time 3D human pose estimation and action recognition. IEEE Trans. Pattern Anal. Mach. Intell. 43, 2752–2764. doi: 10.1109/TPAMI.2020.2976014
Matloob, F., Ghazal, T. M., Taleb, N., Aftab, S., Ahmad, M., Khan, M. A., et al. (2021). Software defect prediction using ensemble learning: a systematic literature review. IEEE Access 9, 98754–98771. doi: 10.1109/ACCESS.2021.3095559
Mehta, D., Rhodin, H., Casas, D., Fua, P., Sotnychenko, O., Xu, W., et al. (2017). “Monocular 3D human pose estimation in the wild using improved cnn supervision,” in 2017 Fifth International Conference on 3D Vision (3D V) (Qingdao: IEEE). doi: 10.1109/3DV.2017.00064
Nian, L. (2022). Simulation of video association motion tracking based on trajectory extraction algorithm. J. Jilin Univ. Sci. Ed. 60:641.
Ning, E., Wang, C., Zhang, H., Ning, X., and Tiwari, P. (2024). Occluded person re-identification with deep learning: a survey and perspectives. Expert Syst. Appl. 239, 122419. doi: 10.1016/j.eswa.2023.122419
Pellas, N., Mystakidis, S., and Kazanidis, I. (2021). Immersive virtual reality in k-12 and higher education: a systematic review of the last decade scientific literature. Virtual Real. 25, 835–861. doi: 10.1007/s10055-020-00489-9
Pham, H. H., Salmane, H., Khoudour, L., Crouzil, A., Zegers, P., Velastin, S. A., et al. (2019). “A deep learning approach for real-time 3D human action recognition from skeletal data," in Image Analysis and Recognition: 16th International Conference, ICIAR 2019, Waterloo, ON, Canada, August 27-29, 2019. Proceedings, Part I 16 (Cham: Springer), 18–32. doi: 10.1007/978-3-030-27202-9_2
Prajapati, K., Chudasama, V., Patel, H., Upla, K., Raja, K., Ramachandra, R., et al. (2021). Direct unsupervised super-resolution using generative adversarial network (DUS-GAN) for real-world data. IEEE Trans. Image Process. 30, 8251–8264. doi: 10.1109/TIP.2021.3113783
Shi, Y., Du, J., Ahn, C. R., and Ragan, E. (2019). Impact assessment of reinforced learning methods on construction workers' fall risk behavior using virtual reality. Autom. Constr. 104, 197–214. doi: 10.1016/j.autcon.2019.04.015
Sigal, L., Balan, A. O., and Black, M. J. (2010). “Humaneva: synchronized video and motion capture dataset for evaluation of articulated human motion,” in Computer Vision- ECCV 2010 (Berlin: Springer), 746–759. doi: 10.1007/s11263-009-0273-6
Singh, R., Saini, K., Sethi, A., Tiwari, A., Saurav, S., Singh, S., et al. (2023). Stemgan: spatio-temporal generative adversarial network for video anomaly detection. Appl. Intell. 53, 28133–28152. doi: 10.1007/s10489-023-04940-7
Szczuko, P. (2019). Deep neural networks for human pose estimation from a very low resolution depth image. Multimed. Tools Appl. 78, 29357–29377. doi: 10.1007/s11042-019-7433-7
Ukita, N., and Uematsu, Y. (2018). Semi-and weakly-supervised human pose estimation. Comput. Vis. Image Underst. 170, 67–78. doi: 10.1016/j.cviu.2018.02.003
Wang, P., Li, W., Ogunbona, P., Wan, J., and Escalera, S. (2018). RGB-D-based human motion recognition with deep learning: a survey. Comput. Vis. Image Underst. 171, 118–139. doi: 10.1016/j.cviu.2018.04.007
Wang, T., and Zhang, X. (2022). Simplified-attention enhanced graph convolutional network for 3D human pose estimation. Neurocomputing 501, 231–243. doi: 10.1016/j.neucom.2022.06.033
Wedel, M., Bigné, E., and Zhang, J. (2020). Virtual and augmented reality: advancing research in consumer marketing. Int. J. Res. Mark. 37, 443–465. doi: 10.1016/j.ijresmar.2020.04.004
Wu, M.-Y., Ting, P.-W., Tang, Y.-H., Chou, E.-T., and Fu, L.-C. (2020). Hand pose estimation in object-interaction based on deep learning for virtual reality applications. J. Vis. Commun. Image Represent. 70:102802. doi: 10.1016/j.jvcir.2020.102802
Xu, Y., Wang, W., Liu, T., Liu, X., Xie, J., Zhu, S.-C., et al. (2021). Monocular 3D pose estimation via pose grammar and data augmentation. IEEE Trans. Pattern Anal. Mach. Intell. 44, 6327–6344. doi: 10.1109/TPAMI.2021.3087695
Yu, W., Lei, B., Wang, S., Liu, Y., Feng, Z., Hu, Y., et al. (2022). Morphological feature visualization of Alzheimer's disease via multidirectional perception gan. IEEE Trans. Neural Netw. Learn. Syst. 34, 4401–4415. doi: 10.1109/TNNLS.2021.3118369
Zhang, H., Zhang, Y., Zhu, L., and Lin, W. (2022). Deep learning-based perceptual video quality enhancement for 3D synthesized view. IEEE Trans. Circ. Syst. Video Technol. 32, 5080–5094. doi: 10.1109/TCSVT.2022.3147788
Zhang, Y., Liu, H., Kang, S.-C., and Al-Hussein, M. (2020). Virtual reality applications for the built environment: research trends and opportunities. Autom. Constr. 118:103311. doi: 10.1016/j.autcon.2020.103311
Zhang, Y., and Ma, Y. (2019). Application of supervised machine learning algorithms in the classification of sagittal gait patterns of cerebral palsy children with spastic diplegia. Comput. Biol. Med. 106, 33–39. doi: 10.1016/j.compbiomed.2019.01.009
Zhou, X., Lu, P., Zheng, Z., Tolliver, D., and Keramati, A. (2020). Accident prediction accuracy assessment for highway-rail grade crossings using random forest algorithm compared with decision tree. Reliab. Eng. Syst. Saf. 200:106931. doi: 10.1016/j.ress.2020.106931
Zhou, Z., Wang, Y., Guo, Y., Jiang, X., and Qi, Y. (2019). Ultrafast plane wave imaging with line-scan-quality using an ultrasound-transfer generative adversarial network. IEEE J. Biomed. Health Inf. 24, 943–956. doi: 10.1109/JBHI.2019.2950334
Keywords: 3D human pose data, robotic assistance, motion quality assessment, Generative Adversarial Networks, Support Vector Machines, DenseNet
Citation: Wang X, Mi Y and Zhang X (2024) 3D human pose data augmentation using Generative Adversarial Networks for robotic-assisted movement quality assessment. Front. Neurorobot. 18:1371385. doi: 10.3389/fnbot.2024.1371385
Received: 16 January 2024; Accepted: 08 March 2024;
Published: 05 April 2024.
Edited by:
Orazio Gambino, University of Palermo, ItalyReviewed by:
Shuqiang Wang, Chinese Academy of Sciences (CAS), ChinaCopyright © 2024 Wang, Mi and Zhang. This is an open-access article distributed under the terms of the Creative Commons Attribution License (CC BY). The use, distribution or reproduction in other forums is permitted, provided the original author(s) and the copyright owner(s) are credited and that the original publication in this journal is cited, in accordance with accepted academic practice. No use, distribution or reproduction is permitted which does not comply with these terms.
*Correspondence: Yang Mi, bWl5YW5nQGx5dS5lZHUuY24=
Disclaimer: All claims expressed in this article are solely those of the authors and do not necessarily represent those of their affiliated organizations, or those of the publisher, the editors and the reviewers. Any product that may be evaluated in this article or claim that may be made by its manufacturer is not guaranteed or endorsed by the publisher.
Research integrity at Frontiers
Learn more about the work of our research integrity team to safeguard the quality of each article we publish.