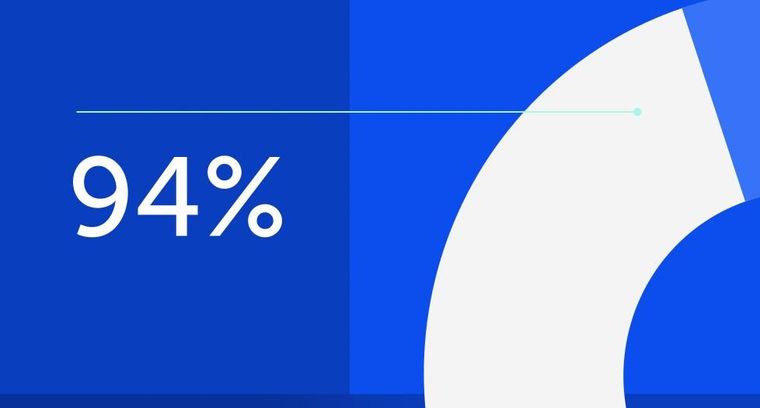
94% of researchers rate our articles as excellent or good
Learn more about the work of our research integrity team to safeguard the quality of each article we publish.
Find out more
ORIGINAL RESEARCH article
Front. Med., 28 March 2025
Sec. Geriatric Medicine
Volume 12 - 2025 | https://doi.org/10.3389/fmed.2025.1535443
This article is part of the Research TopicFrom bench to bedside: Inflammation in Neurovascular Disorders and StrokeView all 9 articles
Objective: To develop and validate a nomogram based on systemic inflammation response index (SIRI) and clinical risk factors to predict short-term prognosis in very elderly patients with hypertensive intracerebral hemorrhage (HICH).
Methods: A total of 324 very elderly HICH patients from January 2017 to June 2024 were retrospectively enrolled and randomly divided into two cohorts for training (n = 227) and validation (n = 97) according to the ratio of 7:3. Independent predictors of poor prognosis were analyzed using univariate and multivariate logistic regression analyses. Furthermore, a nomogram prediction model was built. The area under the receiver operating characteristic curves (AUC), calibration plots and decision curve analysis (DCA) were used to evaluate the performance of the nomogram in predicting the prognosis of very elderly HICH.
Results: By univariate and stepwise multivariate logistic regression analyses, GCS score (p < 0.001), hematoma expansion (p = 0.049), chronic obstructive pulmonary disease (p = 0.010), and SIRI (p = 0.005) were independent predictors for the prognosis in very elderly patients with HICH. The nomogram showed the highest predictive efficiency in the training cohort (AUC = 0.940, 95% CI: 0.909 to 0.971) and the validation cohort (AUC = 0.884, 95% CI: 0.813 to 0.954). The calibration curve indicated that the nomogram had good calibration. DCA showed that the nomogram had high applicability in clinical practice.
Conclusion: The nomogram incorporated with the SIRI and clinical risk factors has good potential in predicting the short-term prognosis of very elderly HICH.
Hypertensive intracerebral hemorrhage (HICH) is one of the common diseases in neurosurgery, which refers to hemorrhage caused by the rupture of blood vessels in the brain parenchyma, with high rates of mortality and poor functional outcome (1). It accounts for approximately 17.1–55.4% of all strokes (2). Currently, surgical treatment is one of the main treatments for HICH, which can reduce intracranial pressure and thus minimize the extent of damage to brain tissue (3).
ICH incidence increases with age, posing a higher assistance burden in countries with aging population (4–6). However, besides age, a large number of factors need to be considered for risk stratification, including comorbidities, ICH volume and site, and neurological status (5). In recent literature, there have been reports of a correlation between systemic inflammation and poor outcomes in patients with ICH (7, 8). Studies have indicated that systemic inflammation and immune response may exert an indispensable role in brain injury and post-stroke recovery (9). The systemic inflammation triggers secondary injury mechanisms after ICH, which directly contribute to the unfavorable prognosis of patient (10, 11).
The systemic inflammation response index (SIRI) index is a novel inflammatory biomarker, which includes peripheral lymphocytes, neutrophils and monocytes, has been described (12). SIRI index has been shown to be more sensitive for predicting outcome, particularly in cancer patients, than other available methods that use only one or two cell subtypes (13). The predictive value of SIRI index for short-term functional outcome in patients with HICH, however, has not been fully studied. In clinical practice, numerous predictive models have been developed in an attempt to accurately predict the prognosis of HICH (14–16). Wang et al.’s research revealed that a nomogram model consisting of the black hole sign, Glasgow Coma Scale (GCS) score, midline shift, hematoma volume, and Rad-score can accurately predict the short-term prognosis of patients with HICH (15). However, nomograms based on SIRI and clinical risk factors from very elderly HICH patients have not been reported, and their effectiveness in predicting short-term prognosis remains questionable. Therefore, this study attempted to develop and validate a nomogram model based on SIRI and clinical risk factors for predicting poor outcome in very elderly patients with HICH.
This was a multicenter retrospective study conducted at two hospitals in Anhui, China (Fuyang Fifth People’s Hospital and Linquan County People’s Hospital). Very elderly (≥80 years old) patients with HICH admitted from January 2017 to June 2024 were enrolled in the study. The patients included in the study were divided into two groups using random allocation: a training set consisting of 227 patients and a validation set consisting of 97 patients, with a ratio of 7:3. This study was approved by the ethics committee of Fuyang Fifth People’s Hospital (Ethics approval number: NO.2023052). The inclusion criteria for this study were as follows: age ≥ 80 years, with a history of hypertension or a diagnosis of hypertension during this hospitalization. Hypertension was defined by systolic blood pressure ≥ 140 mmHg, or diastolic blood pressure ≥ 90 mmHg (17); clinically diagnosed with ICH by computed tomography (CT); and admitted to the hospital within 24 h of symptom onset. The exclusion criteria were as follows: ICH caused by head trauma, cerebral aneurysm, vascular malformation, and brain tumor; long-term use of antiplatelet or anticoagulant drugs; used immunosuppressants; had active infection within the 2 weeks before admission; missing imaging data; previous history of ICH or other neurological diseases such as ischemic stroke; other systematic diseases such as renal dysfunction, hepatic dysfunction, cancer, and hematological disorders; incomplete baseline clinical data; and patients who refused to follow-up clinical assessment after being discharged from hospital.
The following data were collected from the patients: gender, age, time from symptom onset to baseline CT scan, history of diabetes and/or coronary heart disease, GCS score at admission, body temperature, pulse, blood pressure at admission (systolic and diastolic blood pressure), location of hematoma, hematoma volume, perihematomal edema volume, modified Graeb Score (mGS), hematoma expansion (HE), chronic obstructive pulmonary disease (COPD), presence of stress ulcer, and treatment. Presence of COPD was defined and treated according to published guidelines (18, 19). Laboratory examinations included white blood cell count, red blood cell count, platelet count, serum calcium concentration, albumin, and blood glucose on admission. We calculated the neutrophil-lymphocyte ratio (NLR) (20), platelet-lymphocyte ratio (PLR) (20), prognostic nutritional index (PNI) (21), systemic immune-inflammation index (SII) (22) and SIRI according to the following equations: NLR = neutrophil count/lymphocyte count; PLR = platelet count/lymphocyte count; PNI = albumin +5 × total lymphocyte count; SII = (neutrophil count × platelet count)/lymphocyte count; and SIRI = (neutrophil count × monocyte count)/lymphocyte count.
Perihematomal edema volume was measured following the method of Gusdon et al. (23) using semiautomated planimetry with a three-dimensional slicer. Hematoma volume was calculated by the ABC/2 method (24). The mGS was calculated based on three factors, namely, location of intraventricular hemorrhage, hematoma volume in each ventricle, and ventricular dilatation, with a total score of 32 points (25). HE was defined as hematoma enlargement ≥6 mL or ≥ 33% within 24 h (26). The imaging evaluation was performed by two experienced neuroradiologists who were unaware of the other variables and outcomes. Data collection of laboratory results used the first-time examination at admission (within 24 h after admission). Surgical interventions mainly included hematoma evacuation with craniotomy and external ventricular drainage.
All patients with HICH were treated stringently following the guidelines for the management of ICH (27). Patient outcome was evaluated by the Glasgow Outcome Scale (GOS) score at 3 months following HICH. A good outcome was defined as an GOS score of 4–5, and a poor outcome was defined as an GOS score of 1–3 (28).
Statistical analyses were performed using SPSS 26.0 software (SPSS, INC; Chicago, United States), and nomogram establishment was undertaken by R 3.5.1 programming language.1 Categorical variables were presented as numbers with percentages and analyzed with an x2 test or Fisher’s exact test, whereas continuous variables were expressed as mean ± standard deviation or median (interquartile range) and analyzed using Student’s t-test or Mann–Whitney U test. Logistic regression analyses were used to determine the influence of risk factors on outcomes in patients with HICH. Variables with p < 0.05 in univariate analysis were included in multivariate logistic regression. Since SII, NLR, and SIRI share common component factors, there may be bias when they were included together in multivariate logistic regression analysis. Therefore, only SIRI was included in multivariate logistic regression analysis in the study. The nomogram was constructed based on multivariable logistic regression results. The performance of the nomogram was assessed by discrimination and calibration. The discriminative ability of the nomogram was evaluated by the area under the receiver operating characteristic curve (AUC), which ranged from 0.5 (no discrimination) to 1 (perfect discrimination) (29). The calibration was evaluated using calibration curves and Hosmer-Lemeshow tests (30). Both discrimination and calibration were evaluated using bootstrapping methods with 1,000 resamples. Furthermore, decision curve analysis (DCA) was used to evaluate the net benefit of the model for patients (31). Two-sided p-values <0.05 was considered significant.
A total of 324 very elderly HICH patients were enrolled in this study, and they were randomly assigned to the training cohort (n = 227) and the validation cohort (n = 97) (Figure 1). Out of the 227 patients in the training group, 130 (57.3%) had poor outcomes and the mean age was 84 years. In contrast, 55 (56.7%) out of the 97 patients in the validation group had poor outcomes and the mean age was 84 years. The clinical characteristics of patients in the training and validation groups are summarized in Table 1.
In the training group, the data of 227 patients were used to establish the nomogram. Of these patients, 130 (57.3%) had poor outcomes. Between good outcomes and poor outcomes, there were significant differences in time from onset to CT (p < 0.001), history of coronary heart disease (p < 0.001), GCS score (p < 0.001), systolic blood pressure (p < 0.001), baseline HICH volume (p < 0.001), perihematomal edema volume (p < 0.001), mGS (p < 0.001), hematoma expansion (p < 0.001), COPD (p < 0.001), presence of stress ulcer (p < 0.001), white blood cell count (p < 0.001), blood glucose (p = 0.002), NLR (p = 0.020), SII (p = 0.016), and SIRI (p < 0.001) (Table 2). Patients in the poor outcome group showed significantly higher SIRI values, as shown in Figure 2. A multivariate logistic regression analysis revealed that GCS score (odds ratio [OR], 1.968; 95% confidence interval [CI], 1.489–2.602; p < 0.001), hematoma expansion (OR, 0.216; 95% CI, 0.047–0.992; p = 0.049), COPD (OR, 0.193; 95% CI, 0.055–0.677; p = 0.010), and SIRI (OR, 0.709; 95% CI, 0.558–0.900; p = 0.005) were independent risk factors for poor outcome of HICH (Table 3). Based on the four independent predictors of poor outcome identified by multivariate logistic regression modeling, we constructed the nomogram for poor outcome in very elderly HICH patients (Figure 3). The predicted probability of poor outcome can be derived after adding up the scores corresponding to each variable and locating them on the total point scale.
Table 2. Characteristics and comparison between the good outcome group and poor outcome group in training cohort.
Figure 2. Boxplots of SIRI showing the distribution in the good outcome group (n = 139) and poor outcome group (n = 185). The SIRI of the poor outcome group was higher than that of the good outcome group (<0.001).
Figure 3. Predictive nomogram model for assessing the short-term prognosis in very elderly patients with hypertensive intracerebral hemorrhage.
In the training and validation cohorts, the AUC under the receiver operating characteristic curve was 0.940 (95% CI: 0.909, 0.971), and 0.884 (95% CI: 0.813, 0.954), respectively, which indicated that the nomogram had a good discriminative ability (Figure 4). Calibration curves confirmed that the probabilistic forecasting of poor outcome aligned with the observed integration value for both the training and validation cohorts (Figure 5). The Hosmer-Lemeshow test showed no statistical significance in the training (p = 0.799) and validation (p = 0.644) cohorts. A DCA revealed that the nomogram for predicting the short-term prognosis provided greater net benefit than the treat-all-patients and the treat-none-patients in the training and validation cohorts, which suggested that the nomogram had high clinical utility in practical applications (Figure 6).
Figure 4. Receiver operating characteristic curve of predicting the short-term prognosis in very elderly patients with hypertensive intracerebral hemorrhage by the nomogram model. Training group (A), validation group (B).
Figure 5. Calibration curve for predicting the short-term prognosis in very elderly patients with hypertensive intracerebral hemorrhage by the nomogram model. Training group (A), validation group (B).
Figure 6. Decision curve analysis for predicting the short-term prognosis in very elderly patients with hypertensive intracerebral hemorrhage by the nomogram model. Training group (A), validation group (B).
This study demonstrated that GCS score, hematoma expansion, COPD, and SIRI were significant predictors of poor outcome in very elderly HICH patients. Recently, although some studies have established different prognostic prediction models on patients with HICH, there are relatively few studies that focus on very elderly patients with HICH. In view of this, we included very elderly patients with HICH from two centers and constructed a nomogram based on SIRI and clinical risk factors for evaluating short-term prognosis. The nomogram showed good performance in training and validation cohorts and was an easy-to-use personalized decision-making tool.
Neuroinflammation has been linked to neurological diseases, especially cerebrovascular disease (32). Previous studies have demonstrated that inflammatory response plays a crucial role in the pathologic mechanism of ICH and is associated with poor functional outcome (33). There is increasing evidence that easily available serum biomarkers of inflammation can serve as reliable predictors of outcome in patients with ICH and can improve the outcome prediction when added to validated prognostic scales (34). The SIRI is a novel biomarker of inflammatory process. A few studies have found that in patients with ischemic stroke and traumatic spinal cord injury, the mortality rate of patients with high SIRI is higher than that of patients with low SIRI (35, 36). Wang et al. (37) have investigated the relationship between SIRI and clinical outcome of ICH, and found that the SIRI was the best predictor for poor outcomes at discharge in ICH patients. In our study, both univariate and multivariate logistic analyses revealed that SIRI was positively correlated with an unfavorable outcome following HICH. A high level of inflammatory factors such as NLR and PLR are associated with poor outcome of ICH (38, 39). The prevalence of ICH is high in the elderly, chronic and complex comorbidities among whom are somewhat common. SIRI is an important inflammatory indicator, which can be used to evaluate the severity of the comorbidities (40). An elevation of the SIRI is associated with the short-term prognosis of elderly HICH.
Consistent with the previous reports, we found that the admission GCS score was associated with poor clinical outcome. Øie et al. (41) reported that a GCS score of <9 on admission was a risk factor for poor prognosis at 3 months in patients with ICH. In addition, the hematoma expansion could be a predictor of poor outcome, which was similar to the results of Morotti and colleagues (42). It was found that HE was associated with a greater risk of poor outcome in ICH. HE is a common early and severe complication of ICH (43, 44). Several studies indicate that HE occurs in approximately one-third of patients with ICH and is associated with in-hospital mortality and poor outcome (45, 46). Believed to occur hours after symptom onset and initial hemorrhage, HE occurs with an increase in intracranial pressure, resulting in mass effect, midline shift, and herniation (47). In addition, it is also one of the main causes of secondary brain injury, as iron and heme from lysed erythrocytes create a highly oxidative and cytotoxic environment, damaging brain tissue (48, 49). These all lead to significant neurological deficits and worse functional outcomes following ICH. It is well recognized that ICH outcomes differ significantly depending on their location (50, 51). Traditionally, ICH prognostication based on location is separated crudely into infratentorial and supratentorial (52). However, recent studies have demonstrated that the prognostication of ICH outcomes should be classified into more specific anatomic sites (53, 54). Although studies have produced inconsistent results, for supratentorial ICH, the prognosis is generally better for lobar ICH (51, 55, 56) but worse for thalamic (57, 58). Importantly, since ICH volume is also another crucial modifier of ICH outcomes, it is becoming more evident that a location-specific ICH volume would better predict ICH outcomes (59). A small strategic bleed affecting the thalamus may have devastating neurological deficits, while a similar-sized ICH at the frontal lobe could have a more favorable neurological outcome (60). In addition, the risk of HE is also associated with the hemorrhage location. Thalamic ICH had a smaller volume at baseline CT and a low risk of HE, but lobar ICH was likely to develop HE (61, 62). Therefore, as the interaction between ICH location and volume significantly impacts neurological outcomes, using location-specific hematoma volumes for patient selection could better guide the selection of patients who may best benefit from treatment.
The findings of current study revealed the role of COPD in short-term prognosis. COPD is a common disease in the elderly, which has been reported to be detrimental for patients with ICH (63). The mechanism of COPD in poor outcomes may be because of ventilator-associated pneumonia. COPD has an adverse impact on respiratory muscle function, and it may be amplified during neurocritical illness (64). Ozyuvaci et al. (65) reported that patients with COPD exhibited an increased partial pressure of carbon dioxide (PaCO2) that reached the highest level after extubation and did not return to baseline levels. Besides, COPD is an inflammatory disease with complex pathobiology where various underlying mechanisms are implicated (66, 67). Increased numbers of inflammatory cells such as macrophages, neutrophils, and lymphocytes have been reported in COPD patients which play critical roles in the development and pathogenesis of COPD inflammation, directly contributing to airway remodeling and a decrease in lung function (68). Makris et al. (69) has been reported COPD is associated with longer intensive care unit stay and mortality. The present study also revealed that COPD was an independent predictor for poor prognosis in elderly HICH patients. Therefore, very elderly patients with HICH who present with COPD should be monitored closely for any fluctuations in PaCO2 during hospitalization, and appropriate clinical interventions should be employed to enhance patient prognosis.
Early identification and treatment is important for preventing the development of poor prognosis. This is best accomplished by identifying high-risk patients. Consequently, these patients need to be under observation, followed up, and should undergo early intervention. The nomogram is one of the methods to present the predicted model as the graphic scoring. After performing the multivariate regression analysis, we included GCS score, hematoma expansion, COPD, and SIRI, a total of four factors, as nomogram score points. By combining SIRI with clinical features, a novel nomogram was developed to promote further clinical application and accurately predict the prognosis of HICH. Compared with a single index or risk factor, this nomogram has further improved the performance of predicting the prognosis of HICH and achieved higher accuracy. The AUCs of the training and validation cohorts were 0.940 and 0.884, respectively. Furthermore, the calibration curve and DCA curve showed that the nomogram had good consistency and potential clinical applicability, and the maximum benefit was obtained under all thresholds. The doctor can add the scores of each prediction variable and get the total score according to the individual differences of patients, so as to better help clinical decision-making and enable clinicians to develop personalized treatment plans for elderly HICH patients.
This study has several limitations. First, this was a retrospective analysis and may therefore have a certain degree of selection bias. Second, the sample size of this study is not very large. Third, blood parameters were recorded only once on admission and were not monitored dynamically. Fourth, our model only focuses on the short-term prognosis of elderly patients with HICH, which, although important for clinical practice, does not assess long-term prognosis. Last, the prediction model was only subjected to internal validation, lacking external validation, it is necessary to conduct large sample and multicenter studies to prove the feasibility of the nomogram and increase the possibility of extensive popularization of the model.
The results of this study indicate that SIRI is an independent risk factor for poor outcome. The nomogram including GCS score, hematoma expansion, COPD, and SIRI was reliable for predicting the short-term prognosis of very elderly patients with HICH.
The original contributions presented in the study are included in the article/supplementary material, further inquiries can be directed to the corresponding authors.
The studies involving humans were approved by the Ethics Committee of the Fuyang Fifth People’s Hospital. The studies were conducted in accordance with the local legislation and institutional requirements. The ethics committee/institutional review board waived the requirement of written informed consent for participation from the participants or the participants’ legal guardians/next of kin because since this is a retrospective study, the Ethics Committee of the Fuyang Fifth People’s Hospital waived the need for informed consent.
SW: Conceptualization, Formal analysis, Methodology, Writing – original draft. RW: Conceptualization, Formal analysis, Methodology, Writing – original draft. XWL: Data curation, Writing – review & editing. XL: Data curation, Writing – review & editing. JL: Data curation, Writing – review & editing. HS: Conceptualization, Project administration, Supervision, Writing – review & editing. HH: Resources, Supervision, Writing – review & editing.
The author(s) declare that no financial support was received for the research and/or publication of this article.
The authors declare that the research was conducted in the absence of any commercial or financial relationships that could be construed as a potential conflict of interest.
The authors declare that no Gen AI was used in the creation of this manuscript.
All claims expressed in this article are solely those of the authors and do not necessarily represent those of their affiliated organizations, or those of the publisher, the editors and the reviewers. Any product that may be evaluated in this article, or claim that may be made by its manufacturer, is not guaranteed or endorsed by the publisher.
HICH, hypertensive intracerebral hemorrhage; SIRI, systemic inflammation response index; GCS, Glasgow coma scale; CT, computed tomography; mGS, modified Graeb Score; HE, hematoma expansion; COPD, chronic obstructive pulmonary disease; NLR, neutrophil-lymphocyte ratio; PLR, platelet-lymphocyte ratio; PNI, prognostic nutritional index; SII, systemic immune-inflammation index; GOS, Glasgow outcome scale; AUC, area under the receiver operating characteristic curve; DCA, decision curve analysis; OR, odds ratio; CI, confidence interval; PaCO2, partial pressure of carbon dioxide.
1. Hostettler, IC, Seiffge, DJ, and Werring, DJ. Intracerebral hemorrhage: An update on diagnosis and treatment. Expert Rev Neurother. (2019) 19:679–94. doi: 10.1080/14737175.2019.1623671
2. Ziai, WC, and Carhuapoma, JR. Intracerebral hemorrhage. Continuum (Minneap Minn). (2018) 24:1603–22. doi: 10.1212/con.0000000000000672
3. Schrag, M, and Kirshner, H. Management of Intracerebral Hemorrhage: Jacc focus seminar. J Am Coll Cardiol. (2020) 75:1819–31. doi: 10.1016/j.jacc.2019.10.066
4. van Asch, CJ, Luitse, MJ, Rinkel, GJ, van der Tweel, I, Algra, A, and Klijn, CJ. Incidence, case fatality, and functional outcome of intracerebral Haemorrhage over time, according to age, sex, and ethnic origin: a systematic review and meta-analysis. Lancet Neurol. (2010) 9:167–76. doi: 10.1016/s1474-4422(09)70340-0
5. Forti, P, Maioli, F, Domenico Spampinato, M, Barbara, C, Nativio, V, Coveri, M, et al. The effect of age on characteristics and mortality of intracerebral hemorrhage in the oldest-old. Cerebrovasc Dis. (2016) 42:485–92. doi: 10.1159/000448813
6. Inoue, Y, Miyashita, F, Minematsu, K, and Toyoda, K. Clinical characteristics and outcomes of intracerebral hemorrhage in very elderly. J Stroke Cerebrovasc Dis. (2018) 27:97–102. doi: 10.1016/j.jstrokecerebrovasdis.2017.08.006
7. Bader, ER, Pana, TA, Barlas, RS, Metcalf, AK, Potter, JF, and Myint, PK. Elevated inflammatory biomarkers and poor outcomes in intracerebral hemorrhage. J Neurol. (2022) 269:6330–41. doi: 10.1007/s00415-022-11284-8
8. Mackey, J, Blatsioris, AD, Saha, C, Moser, EAS, Carter, RJL, Cohen-Gadol, AA, et al. Higher monocyte count is associated with 30-Day case fatality in intracerebral hemorrhage. Neurocrit Care. (2021) 34:456–64. doi: 10.1007/s12028-020-01040-z
9. Saand, AR, Yu, F, Chen, J, and Chou, SH. Systemic inflammation in hemorrhagic strokes – a novel neurological sign and therapeutic target? J Cereb Blood Flow Metab. (2019) 39:959–88. doi: 10.1177/0271678x19841443
10. Chen, S, Yang, Q, Chen, G, and Zhang, JH. An update on inflammation in the acute phase of intracerebral hemorrhage. Transl Stroke Res. (2015) 6:4–8. doi: 10.1007/s12975-014-0384-4
11. Chu, H, Huang, C, Zhou, Z, Tang, Y, Dong, Q, and Guo, Q. Inflammatory score predicts early hematoma expansion and poor outcomes in patients with intracerebral hemorrhage. Int J Surg. (2023) 109:266–76. doi: 10.1097/js9.0000000000000191
12. Zhang, Y, Xing, Z, Zhou, K, and Jiang, S. The predictive role of systemic inflammation response index (Siri) in the prognosis of stroke patients. Clin Interv Aging. (2021) 16:1997–2007. doi: 10.2147/cia.S339221
13. Qi, Q, Zhuang, L, Shen, Y, Geng, Y, Yu, S, Chen, H, et al. A novel systemic inflammation response index (Siri) for predicting the survival of patients with pancreatic Cancer after chemotherapy. Cancer. (2016) 122:2158–67. doi: 10.1002/cncr.30057
14. Sun, R, Liu, J, Hui, P, Zhang, Y, and Xiao, Z. Development and validation of a prognostic nomogram based on the hub genes of patients with hypertensive intracerebral hemorrhage. Am J Transl Res. (2024) 16:5296–310. doi: 10.62347/cuwd4200
15. Wang, J, Zhou, L, Chen, Y, Zhou, H, Tan, Y, Zhong, W, et al. Prediction of short-term prognosis of patients with hypertensive intracerebral hemorrhage by Radiomic-clinical nomogram. Front Neurol. (2023) 14:1053846. doi: 10.3389/fneur.2023.1053846
16. Fang, C, An, X, Li, K, Zhang, J, Shang, H, Jiao, T, et al. A nomogram based on Ct Radiomics and clinical risk factors for prediction of prognosis of hypertensive intracerebral hemorrhage. Comput Intell Neurosci. (2022) 2022:9751988. doi: 10.1155/2022/9751988
17. Chobanian, AV, Bakris, GL, Black, HR, Cushman, WC, Green, LA, Izzo, JL Jr, et al. Seventh report of the joint National Committee on prevention, detection, evaluation, and treatment of high blood pressure. Hypertension. (2003) 42:1206–52. doi: 10.1161/01.HYP.0000107251.49515.c2
18. Bach, PB, Brown, C, Gelfand, SE, and McCrory, DC. Management of Acute Exacerbations of chronic obstructive pulmonary disease: a summary and appraisal of published evidence. Ann Intern Med. (2001) 134:600–20. doi: 10.7326/0003-4819-134-7-200104030-00016
19. Vestbo, J, Hurd, SS, Agustí, AG, Jones, PW, Vogelmeier, C, Anzueto, A, et al. Global strategy for the diagnosis, management, and prevention of chronic obstructive pulmonary disease: gold executive summary. Am J Respir Crit Care Med. (2013) 187:347–65. doi: 10.1164/rccm.201204-0596PP
20. Feng, H, Wang, X, Wang, W, and Zhao, X. Risk factors and a prediction model for the prognosis of intracerebral hemorrhage using cerebral microhemorrhage and clinical factors. Front Neurol. (2023) 14:1268627. doi: 10.3389/fneur.2023.1268627
21. Jhang, SW, Liu, YT, Kor, CT, Wu, YP, and Lai, CH. Low prognostic nutritional index predicts in-hospital complications and case fatality in patients with spontaneous intracerebral hemorrhage: a retrospective study. Nutrients. (2024) 16:1841. doi: 10.3390/nu16121841
22. Trifan, G, and Testai, FD. Systemic immune-inflammation (Sii) index predicts poor outcome after spontaneous Supratentorial intracerebral hemorrhage. J Stroke Cerebrovasc Dis. (2020) 29:105057. doi: 10.1016/j.jstrokecerebrovasdis.2020.105057
23. Gusdon, AM, Gialdini, G, Kone, G, Baradaran, H, Merkler, AE, Mangat, HS, et al. Neutrophil-lymphocyte ratio and Perihematomal edema growth in intracerebral hemorrhage. Stroke. (2017) 48:2589–92. doi: 10.1161/strokeaha.117.018120
24. Kothari, RU, Brott, T, Broderick, JP, Barsan, WG, Sauerbeck, LR, Zuccarello, M, et al. The Abcs of measuring intracerebral hemorrhage volumes. Stroke. (1996) 27:1304–5. doi: 10.1161/01.str.27.8.1304
25. Morgan, TC, Dawson, J, Spengler, D, Lees, KR, Aldrich, C, Mishra, NK, et al. The modified Graeb score: An enhanced tool for intraventricular hemorrhage measurement and prediction of functional outcome. Stroke. (2013) 44:635–41. doi: 10.1161/strokeaha.112.670653
26. Brott, T, Broderick, J, Kothari, R, Barsan, W, Tomsick, T, Sauerbeck, L, et al. Early hemorrhage growth in patients with intracerebral hemorrhage. Stroke. (1997) 28:1–5. doi: 10.1161/01.str.28.1.1
27. Hemphill, JC 3rd, Greenberg, SM, Anderson, CS, Becker, K, Bendok, BR, Cushman, M, et al. Guidelines for the Management of Spontaneous Intracerebral Hemorrhage: a guideline for healthcare professionals from the American Heart Association/American Stroke Association. Stroke. (2015) 46:2032–60. doi: 10.1161/str.0000000000000069
28. Wilson, JT, Pettigrew, LE, and Teasdale, GM. Structured interviews for the Glasgow outcome scale and the extended Glasgow outcome scale: guidelines for their use. J Neurotrauma. (1998) 15:573–85. doi: 10.1089/neu.1998.15.573
29. Harrell, FE Jr, Califf, RM, Pryor, DB, Lee, KL, and Rosati, RA. Evaluating the yield of medical tests. JAMA. (1982) 247:2543–6. doi: 10.1001/jama.1982.03320430047030
30. Kramer, AA, and Zimmerman, JE. Assessing the calibration of mortality benchmarks in critical care: the Hosmer-Lemeshow test revisited. Crit Care Med. (2007) 35:2052–6. doi: 10.1097/01.Ccm.0000275267.64078.B0
31. Vickers, AJ, and Holland, F. Decision curve analysis to evaluate the clinical benefit of prediction models. Spine J. (2021) 21:1643–8. doi: 10.1016/j.spinee.2021.02.024
32. Xanthos, DN, and Sandkühler, J. Neurogenic Neuroinflammation: inflammatory Cns reactions in response to neuronal activity. Nat Rev Neurosci. (2014) 15:43–53. doi: 10.1038/nrn3617
33. Wang, J, and Doré, S. Inflammation after intracerebral hemorrhage. J Cereb Blood Flow Metab. (2007) 27:894–908. doi: 10.1038/sj.jcbfm.9600403
34. Lattanzi, S, Cagnetti, C, Rinaldi, C, Angelocola, S, Provinciali, L, and Silvestrini, M. Neutrophil-to-lymphocyte ratio improves outcome prediction of acute intracerebral hemorrhage. J Neurol Sci. (2018) 387:98–102. doi: 10.1016/j.jns.2018.01.038
35. Lin, KB, Fan, FH, Cai, MQ, Yu, Y, Fu, CL, Ding, LY, et al. Systemic immune inflammation index and system inflammation response index are potential biomarkers of atrial fibrillation among the patients presenting with ischemic stroke. Eur J Med Res. (2022) 27:106. doi: 10.1186/s40001-022-00733-9
36. Wang, C, Chen, M, Wang, T, Wang, Y, Zhu, Y, Cui, T, et al. Prognostic value of the systemic inflammatory index (Sii) and systemic inflammatory response index (Siri) in patients with traumatic spinal cord injury. Eur Spine J. (2024) 33:1245–55. doi: 10.1007/s00586-023-08114-4
37. Li, Y, Liu, X, Wang, J, Pan, C, and Tang, Z. A nomogram model for predicting prognosis in spontaneous intracerebral hemorrhage patients. J Integr Neurosci. (2023) 22:42. doi: 10.31083/j.jin2202042
38. Shi, M, Li, XF, Zhang, TB, Tang, QW, Peng, M, and Zhao, WY. Prognostic role of the neutrophil-to-lymphocyte ratio in intracerebral hemorrhage: a systematic review and Meta-analysis. Front Neurosci. (2022) 16:825859. doi: 10.3389/fnins.2022.825859
39. Zhao, G, Gu, Y, Wang, Z, Chen, Y, and Xia, X. The clinical value of inflammation index in predicting Icu mortality of critically ill patients with intracerebral hemorrhage. Front Public Health. (2024) 12:1373585. doi: 10.3389/fpubh.2024.1373585
40. Dziedzic, EA, Gąsior, JS, Tuzimek, A, Paleczny, J, Junka, A, Dąbrowski, M, et al. Investigation of the associations of novel inflammatory biomarkers-systemic inflammatory index (Sii) and systemic inflammatory response index (Siri)-with the severity of coronary artery disease and acute coronary syndrome occurrence. Int J Mol Sci. (2022) 23:9553. doi: 10.3390/ijms23179553
41. Øie, LR, Madsbu, MA, Solheim, O, Jakola, AS, Giannadakis, C, Vorhaug, A, et al. Functional outcome and survival following spontaneous intracerebral hemorrhage: a retrospective population-based study. Brain Behav. (2018) 8:e01113. doi: 10.1002/brb3.1113
42. Morotti, A, Boulouis, G, Nawabi, J, Li, Q, Charidimou, A, Pasi, M, et al. Association between hematoma expansion severity and outcome and its interaction with baseline intracerebral hemorrhage volume. Neurology. (2023) 101:e1606–13. doi: 10.1212/wnl.0000000000207728
43. Balami, JS, and Buchan, AM. Complications of intracerebral Haemorrhage. Lancet Neurol. (2012) 11:101–18. doi: 10.1016/s1474-4422(11)70264-2
44. Kazui, S, Naritomi, H, Yamamoto, H, Sawada, T, and Yamaguchi, T. Enlargement of spontaneous intracerebral hemorrhage. Incidence and time course. Stroke. (1996) 27:1783–7. doi: 10.1161/01.str.27.10.1783
45. Al-Shahi Salman, R, Frantzias, J, Lee, RJ, Lyden, PD, Battey, TWK, Ayres, AM, et al. Absolute risk and predictors of the growth of acute spontaneous intracerebral Haemorrhage: a systematic review and Meta-analysis of individual patient data. Lancet Neurol. (2018) 17:885–94. doi: 10.1016/s1474-4422(18)30253-9
46. Davis, SM, Broderick, J, Hennerici, M, Brun, NC, Diringer, MN, Mayer, SA, et al. Hematoma growth is a determinant of mortality and poor outcome after intracerebral hemorrhage. Neurology. (2006) 66:1175–81. doi: 10.1212/01.wnl.0000208408.98482.99
47. LoPresti, MA, Bruce, SS, Camacho, E, Kunchala, S, Dubois, BG, Bruce, E, et al. Hematoma volume as the major determinant of outcomes after intracerebral hemorrhage. J Neurol Sci. (2014) 345:3–7. doi: 10.1016/j.jns.2014.06.057
48. Aronowski, J, and Zhao, X. Molecular pathophysiology of cerebral hemorrhage: secondary brain injury. Stroke. (2011) 42:1781–6. doi: 10.1161/strokeaha.110.596718
49. Xiong, XY, Wang, J, Qian, ZM, and Yang, QW. Iron and intracerebral hemorrhage: from mechanism to translation. Transl Stroke Res. (2014) 5:429–41. doi: 10.1007/s12975-013-0317-7
50. Kuohn, LR, Witsch, J, Steiner, T, Sheth, KN, Kamel, H, Navi, BB, et al. Early deterioration, hematoma expansion, and outcomes in deep versus lobar intracerebral hemorrhage: the fast trial. Stroke. (2022) 53:2441–8. doi: 10.1161/strokeaha.121.037974
51. Castellanos, M, Leira, R, Tejada, J, Gil-Peralta, A, Dávalos, A, and Castillo, J. Predictors of good outcome in medium to large spontaneous Supratentorial intracerebral Haemorrhages. J Neurol Neurosurg Psychiatry. (2005) 76:691–5. doi: 10.1136/jnnp.2004.044347
52. Hemphill, JC 3rd, Bonovich, DC, Besmertis, L, Manley, GT, and Johnston, SC. The Ich score: a simple, reliable grading scale for intracerebral hemorrhage. Stroke. (2001) 32:891–7. doi: 10.1161/01.str.32.4.891
53. Ironside, N, Chen, CJ, Dreyer, V, Christophe, B, Buell, TJ, and Connolly, ES. Location-specific differences in hematoma volume predict outcomes in patients with spontaneous intracerebral hemorrhage. Int J Stroke. (2020) 15:90–102. doi: 10.1177/1747493019830589
54. Teo, KC, Fong, SM, Leung, WCY, Leung, IYH, Wong, YK, Choi, OMY, et al. Location-specific hematoma volume cutoff and clinical outcomes in intracerebral hemorrhage. Stroke. (2023) 54:1548–57. doi: 10.1161/strokeaha.122.041246
55. Kim, KH. Predictors of 30-Day mortality and 90-Day functional recovery after primary intracerebral hemorrhage: hospital based multivariate analysis in 585 patients. J Korean Neurosurg Soc. (2009) 45:341–9. doi: 10.3340/jkns.2009.45.6.341
56. Hårdemark, HG, Wesslén, N, and Persson, L. Influence of clinical factors, Ct findings and early management on outcome in Supratentorial intracerebral hemorrhage. Cerebrovasc Dis. (1999) 9:10–21. doi: 10.1159/000015890
57. Tsai, YH, Hsu, LM, Weng, HH, Lee, MH, Yang, JT, and Lin, CP. Functional diffusion map as an imaging predictor of functional outcome in patients with primary intracerebral Haemorrhage. Br J Radiol. (2013) 86:20110644. doi: 10.1259/bjr.20110644
58. Delcourt, C, Sato, S, Zhang, S, Sandset, EC, Zheng, D, Chen, X, et al. Intracerebral hemorrhage location and outcome among Interact2 participants. Neurology. (2017) 88:1408–14. doi: 10.1212/wnl.0000000000003771
59. Magid-Bernstein, J, Girard, R, Polster, S, Srinath, A, Romanos, S, Awad, IA, et al. Cerebral hemorrhage: pathophysiology, treatment, and future directions. Circ Res. (2022) 130:1204–29. doi: 10.1161/circresaha.121.319949
60. Leasure, AC, Sheth, KN, Comeau, M, Aldridge, C, Worrall, BB, Vashkevich, A, et al. Identification and validation of hematoma volume cutoffs in spontaneous, Supratentorial deep intracerebral hemorrhage. Stroke. (2019) 50:2044–9. doi: 10.1161/strokeaha.118.023851
61. Deng, L, Chen, K, Yang, L, Deng, Z, and Zheng, H. Different effects of hematoma expansion on short-term functional outcome in basal ganglia and thalamic hemorrhages. Biomed Res Int. (2021) 2021:9233559. doi: 10.1155/2021/9233559
62. Yogendrakumar, V, Demchuk, AM, Aviv, RI, Rodriguez-Luna, D, Molina, CA, Blas, YS, et al. Location of intracerebral Haemorrhage predicts Haematoma expansion. Eur Stroke J. (2017) 2:257–63. doi: 10.1177/2396987317715836
63. Huttner, HB, Kohrmann, M, Berger, C, Georgiadis, D, and Schwab, S. Predictive factors for tracheostomy in Neurocritical care patients with spontaneous Supratentorial hemorrhage. Cerebrovasc Dis. (2006) 21:159–65. doi: 10.1159/000090527
64. Rabe, KF, Hurd, S, Anzueto, A, Barnes, PJ, Buist, SA, Calverley, P, et al. Global strategy for the diagnosis, management, and prevention of chronic obstructive pulmonary disease: gold executive summary. Am J Respir Crit Care Med. (2007) 176:532–55. doi: 10.1164/rccm.200703-456SO
65. Ozyuvaci, E, Demircioglu, O, Toprak, N, Topacoglu, H, Sitilci, T, and Akyol, O. Comparison of transcutaneous, arterial and end-tidal measurements of carbon dioxide during laparoscopic cholecystectomy in patients with chronic obstructive pulmonary disease. J Int Med Res. (2012) 40:1982–7. doi: 10.1177/030006051204000540
66. Makris, D, Lazarou, S, Alexandrakis, M, Kourelis, TV, Tzanakis, N, Kyriakou, D, et al. Tc2 response at the onset of Copd exacerbations. Chest. (2008) 134:483–8. doi: 10.1378/chest.07-2626
67. Makris, D, Vrekoussis, T, Izoldi, M, Alexandra, K, Katerina, D, Dimitris, T, et al. Increased apoptosis of neutrophils in induced sputum of Copd patients. Respir Med. (2009) 103:1130–5. doi: 10.1016/j.rmed.2009.03.002
68. Wang, Y, Xu, J, Meng, Y, Adcock, IM, and Yao, X. Role of inflammatory cells in airway remodeling in Copd. Int J Chron Obstruct Pulmon Dis. (2018) 13:3341–8. doi: 10.2147/copd.S176122
Keywords: very elderly hypertensive intracerebral hemorrhage, poor prognosis, systemic inflammation response index, risk factors, nomogram
Citation: Wang S, Wang R, Li X, Liu X, Lai J, Sun H and Hu H (2025) A nomogram based on systemic inflammation response index and clinical risk factors for prediction of short-term prognosis of very elderly patients with hypertensive intracerebral hemorrhage. Front. Med. 12:1535443. doi: 10.3389/fmed.2025.1535443
Received: 28 November 2024; Accepted: 17 March 2025;
Published: 28 March 2025.
Edited by:
Mohd Kaisan Mahadi, National University of Malaysia, MalaysiaReviewed by:
Gengzhao Ye, First Affiliated Hospital of Fujian Medical University, ChinaCopyright © 2025 Wang, Wang, Li, Liu, Lai, Sun and Hu. This is an open-access article distributed under the terms of the Creative Commons Attribution License (CC BY). The use, distribution or reproduction in other forums is permitted, provided the original author(s) and the copyright owner(s) are credited and that the original publication in this journal is cited, in accordance with accepted academic practice. No use, distribution or reproduction is permitted which does not comply with these terms.
*Correspondence: Haicheng Hu, 289527807@qq.com; Hongtao Sun, sunhttj@163.com
†These authors share first authorship
Disclaimer: All claims expressed in this article are solely those of the authors and do not necessarily represent those of their affiliated organizations, or those of the publisher, the editors and the reviewers. Any product that may be evaluated in this article or claim that may be made by its manufacturer is not guaranteed or endorsed by the publisher.
Research integrity at Frontiers
Learn more about the work of our research integrity team to safeguard the quality of each article we publish.