Erratum: Prediction and analysis of strength and economic feasibility of filling materials under the influence of mix proportion and curing age
- 1Xi’an Kedagaoxin University, Xi’an, Shaanxi, China
- 2Electrical and Control Engineering, Xi’an University of Science and Technology, Xi’an, Shaanxi, China
The research aims to investigate the effects of proportion and age of maintenance on the strength and economy of filling materials, in order to provide theoretical guidance and technical support for the management of coal mined-out area. Firstly, adjustments are made to the proportioning and maintenance age of the fill materials, and experiments are conducted to explore the strength performance of materials under different proportions. Secondly, GANs is utilized to expand the dataset, and an MLP-LSTM network is constructed to predict the strength of materials experiencing different maintenance ages under various proportions. Finally, integrating practical engineering applications, an economic viability prediction analysis is conducted to examine the cost of fill materials under different proportions and maintenance ages, along with their influencing factors. The results indicate that appropriate adjustments to proportioning can effectively enhance the strength of fill materials, whereas excessively high or low proportions may lead to unstable or surplus strength. Extending the maintenance age can to some extent improve the material’s strength, but it also increases maintenance costs, necessitating a comprehensive balance in terms of economic viability. Consequently, this research offers a theoretical foundation and practical guidelines for optimizing mixture proportions and selecting appropriate curing ages, providing valuable insights for enhancing the efficiency and cost-effectiveness of coal mined-out area filling treatments.
1 Introduction
The rapid economic growth has led to an increasing demand for natural resources, significantly driving the development of mining enterprises. Consequently, the scale of mineral resource extraction is expanding annually. In the coal mining at the same time will produce a large area of coal mined-out area, the large area of the mined-out area of the roof will trigger the roadway roof topping, underground water, mine earthquake and mine ground subsidence and other issues, the management of the mined-out area is also the focus of attention to the safety of coal mine mining (Zheng and Li, 2005; Zhou et al., 2022; Cao et al., 2022). In recent years, in order to meet the needs of the sustainable development strategy of the environment, the cementation filling method has become the most commonly used method for the treatment of coal mined-out area due to its ability to utilize solid waste resources (Guo et al., 2022). The conventional cementation filling method predominantly relies on cement as the binding material, which poses several challenges, including high costs, difficulties in determining the optimal curing age, and low utilization of solid waste. Notably, some mines utilize inappropriate material ratios, resulting in a misalignment between filling costs and performance. Additionally, extended curing times can lead to excessive strength, incurring unnecessary time costs. Consequently, there is a growing emphasis on the research and development of economical filling materials that can either partially or completely substitute cement. These materials should ideally be low-cost and high-performance while effectively utilizing solid waste, garnering significant attention from scholars both domestically and internationally (Zhao H. et al., 2024).
The strength of backfill materials refers to their resistance to external forces and is one of the crucial indicators for evaluating their quality and performance (Cao et al., 2015). The curing age refers to the duration after the pouring of backfill materials during which the moisture within the backfill constantly exchanges with the ambient air. During this period, the particles of the material undergo more thorough hydration, thereby enhancing the strength of the backfill material (Liu et al., 2021). A too short curing age can result in the backfill material failing to reach the desired strength requirements, while an excessively long curing age can lead to unnecessary time and cost consumption due to excessive strength (Cao et al., 2016). Wang C and his team conducted orthogonal experiments using coarse fly ash-based binder and coal gangue as aggregates. They performed range analysis on the strength and transportation requirements of the filling paste and determined the optimal mix ratio using the comprehensive balance method (Wang et al., 2019). Roy R and their team developed a new gravity grouting blind hole backfilling method for abandoned mine gap. Through laboratory experiments, they selected appropriate backfill materials and established empirical equations between void volume, solid flow velocity, and solid concentration in the gravity grouting method. They determined the optimal (30% bottom ash, 70% sand) and suboptimal (40% bottom ash, 60% sand) solid compositions of bottom ash and sand for blind hole backfilling (Roy et al., 2023). Hefni M utilized an air-entraining agent to incorporate prefabricated foam in the backfill mixture to develop a new type of mine backfill material, foam mine fill (FMF), which achieves a honeycomb structure and minimizes the adverse effects of potential backfill failure (Hefni and Hassani, 2020). Rong K and their team developed a filling material using hemihydrate gypsum instead of cement. The results showed that the strength of the backfill reached the expected target strength of 2.5 MPa after 3 days. The rapid solidification of the backfill material is advantageous for safe underground construction in mines (Rong et al., 2020). Hong Z and their team developed a novel filling material composed of a mixture of coal gangue and fly ash as the main components, with finely ground slag, quicklime, and gypsum mixture serving as the binder. Experimental results demonstrated that the new material exhibited high compressive strength. Even after damage and fracture along a certain angle, the strength could still recover (Hong et al., 2023). Kennedy C and their team used Nano-Silica (NS) as a mortar enhancement material and employed advanced machine learning techniques to predict its compressive strength (Onyelowe et al., 2024a). Ding Z and their team utilized ordinary Portland cement, sulphate aluminum cement, and alkali-activated cement as binders to prepare high-water content tailings materials with varying water-cement ratios for filling. They analyzed the influence of different binders and water-cement ratios on the strength (Ding et al., 2023).
In addition to the strength of backfill materials, the selection of the curing age also has a significant impact on the engineering quality. The strength of backfill materials is closely related to their mix ratio, while the curing age directly affects the early strength development and overall durability of the backfill materials. Choosing an appropriate curing age can effectively enhance the early strength development of backfill materials (Zhang et al., 2021; Onyelowe et al., 2022a). Qiu J and their team prepared backfill samples with varying tailings powder content and curing age. They conducted experimental tests to investigate the influence of different tailings powder content and curing time on the samples. The experimental results indicated that samples containing 50% tailings powder showed the most significant improvements in both macroscopic and microscopic properties (Qiu et al., 2023).
In mining backfill engineering, exploring appropriate mix ratios and curing age to optimize the strength and economy of backfill materials is a highly significant issue (Onyelowe et al., 2024b). The strength of backfill materials is closely related to their mix ratios, while the curing age directly affects the early strength development and overall durability of backfill materials. In order to accurately evaluate and predict the performance of backfill materials as well as their economic viability, empirical models and mathematical models have been established to predict the variation patterns of backfill body properties (Wagner and Rondinelli, 2016). Traditional empirical and mathematical models may require more manual intervention for feature selection and modeling, thus having limitations. Therefore, some scholars utilize deep learning networks for predictive analysis. The adaptability, nonlinear function fitting ability, and tunability of structural parameters in deep learning networks align well with the fuzzy complexity, irregular nonlinearity, and time-varying parameters of coal mine backfill material composition regarding strength and flowability (LeCun et al., 2015). Niaki M H and their team utilized deep neural networks (DNN) to predict the material strength of concrete composite materials, achieving high accuracy (Niaki et al., 2022). Feng F and their team constructed a three-layer neural network to predict the strength of CBSWF (coal-based solid waste filler) at different curing age, with the advantages of fast prediction speed and high accuracy (Feng et al., 2021).
This paper aims to conduct an in-depth study on the properties of backfill materials, predicting and analyzing the strength of different mixed backfill materials under various curing age. The goal is to explore the variation patterns of the main physical and mechanical properties of backfill materials at different curing age, providing a scientific theoretical basis for the selection of material ratios and curing age in mining backfill processes. Firstly, Generative Adversarial Networks (GANs) is employed to expand the strength data of backfill materials at different curing age, generating strength data with similar characteristics through adversarial networks to increase the diversity and quantity of the dataset. Then, a neural network model combining Long Short-Term Memory (LSTM) with Multilayer Perceptron (MLP) is selected. MLP and LSTM are combined to address the mixed problem of non-sequential and sequential data. Past strength data of backfill materials within certain time frames are used as input to predict the strength variation at different curing age. This method considers the temporal characteristics of backfill material strength and fully utilizes historical data and trend information to improve the accuracy and stability of predictions. The research technical route is shown in Figure 1.
By predicting the strength of filling materials at different curing ages, this study enables construction personnel to better plan the filling schedule and resource allocation, selecting the optimal curing age for filling construction to maximize the effectiveness and efficiency of the filling process.
2 Experimental materials and methods
2.1 Experimental materials and procedures
2.1.1 Experimental materials
In this experiment, cement-based backfill material was used, with the main components being coal gangue, cement, and fly ash, while the liquid component primarily consisted of water (H2O). X-ray fluorescence spectroscopy analysis was conducted separately on the solid materials, and the chemical compositions obtained are shown in Table 1.
In this experiment, coal gangue sourced from a Yulin City coal mine in Shaanxi Province was used as the aggregate for backfill material. It was initially crushed to under 50 mm with a jaw crusher and then further reduced to below 16 mm with an impact crusher, resulting in a particle size distribution of 26.8% at 0–0.30 mm, 65.7% at 0.30–4.75 mm, and 7.5% at 4.75–16.00 mm. Ordinary Portland cement, chosen for its strength, durability, cost efficiency, and controllability, served as the primary binder (Onyelowe et al., 2022b). Fly ash, sourced from a power plant in Yulin, was used as a supplementary cementitious material to enhance backfill performance, improve project efficiency, and promote environmental sustainability. Tap water from the laboratory was used throughout the experiment.
2.1.2 Experimental procedures
In order to investigate the effects of fly ash content and slurry mass concentration variations on the flow properties of backfill materials, a total of 575 sets of experimental samples were prepared according to the experimental ratios shown in Table 2. Here, the mass concentration represents the ratio of the mass of solids (including fly ash, cement, and coal gangue) to the total mass (including water, fly ash, cement, and coal gangue). The fly ash contents were 0%, 5%, 10%, 15%, 20%, 25%, 30%, and 35%, while the cement contents were 6%, 8%, 10%, 12%, and 14%. According to the ratios in Table 2, the respective raw materials were weighed and poured into a mortar mixer, followed by the addition of varying amounts of tap water (with solid mass to total mass ratios of 72%, 74%, 76%, 78%, and 80%) for mixing. After the slurry mixing was completed, it was injected into three-part plastic molds, with specific dimensions of 70.7 mm³ × 70.7 mm³ × 70.7 mm³. When the filled slurry injected into the molds was cured for about 24 h at room temperature, the samples were demolded and numbered. They were then placed in a standard curing chamber with a temperature of (20 ± 2) °C and relative humidity of (95% ± 1%) for curing. The curing ages were set as 3, 7, 14, 28, 56, and 90 days, and corresponding strength tests were conducted at the respective curing ages (Liu T. et al., 2024).
The strength of the filling material is tested according to GB/T50080-2016 “Standard Test Methods for the Performance of Ordinary Concrete Mixtures.” A computer-controlled ZJ-100 universal testing machine is used for strength testing, with the loading mode controlled by displacement. The maximum load is 100 kN, and the loading rate is 1 mm/min. Data for each test is recorded, including maximum load and displacement, for subsequent analysis.
The experimental design for backfill material ratios is grounded in practical conditions, leveraging historical data from previous experiments along with established theoretical insights to inform the design process. This approach aims to systematically examine the influence of multiple factors on backfill material performance while reducing the number of experiments needed, thereby optimizing cost and time efficiency. Using the ratios outlined in Table 2, experiments were conducted to generate the raw dataset for analysis.
2.2 Experimental data processing
2.2.1 Principles of Generative Adversarial Networks
Generative Adversarial Networks (GANs) is a special training framework proposed by Goodfellow et al. (2014). In the GANs, there are two neural networks competing with each other: the generator (G) and the discriminator (D). The generator receives random noise z as input and tries to generate fake data that looks similar to real data. The discriminator, on the other hand, attempts to distinguish between real data and fake data generated by the generator, outputting a scalar in the range [0,1] representing the probability that the data is real.
The model is trained by minimizing the competition between the generator and the discriminator. Specifically, the loss function is defined using a minimax approach, as shown in Equation 1.
Where
From Equation 1, it can be inferred that during the training process of the discriminator, the goal is to maximize the objective function, making the output probability of
During the training process, the goal of the generator is to generate “realistic data” as much as possible to deceive the discriminator, while the goal of the discriminator is to distinguish between the fake data generated by the generator and the real data as much as possible. In this way, the generator and the discriminator form a dynamic “game process”, and eventually the generator can generate data that is indistinguishable from real data. The framework structure of the GAN network is illustrated in Figure 2.
2.2.2 Results of GANs expanded dataset
There are 175 sets of raw data, obtained from experiments conducted according to the ratios in Table 1. Each set of data includes the backfill material ratios and the strengths of the backfill materials at curing ages of 3, 7, 14, 28, 56, and 90 days.
Since there is a need to predict the strength based on the backfill ratios data, the existing dataset is insufficient to obtain accurate training results. Therefore, Generative Adversarial Networks (GANs) are used for data augmentation. The training objective is to obtain the strengths corresponding to different ratios of backfill materials and different curing ages, facilitating subsequent analysis. In this study, traditional random noise is replaced by different ratios of backfill materials as the input to the generator. The generator learns the internal connections between inputs and outputs, generating new data that outputs the strengths corresponding to different ratios of backfill materials at different curing ages. Subsequently, the discriminator judges whether the generated new data has a similar data structure to real data. Using the trained generator, new ratios that have not been experimentally tested can be input to generate more data.
Figure 3 shows the loss function curves of the generator and discriminator during GANs training.
As shown in Figure 3, initially, the generator was unable to effectively capture the data features, and the discriminator’s discriminatory ability was poor, resulting in abrupt changes and fluctuations in the curves. As the adversarial process progressed, the generator gradually learned the features of real data, and the discriminator gradually learned to distinguish between the real distribution and the generated distribution. When the generator had iterated approximately 142 times, the loss gradually stabilized and approached 0. Similarly, when the discriminator had iterated approximately 167 times, the loss also gradually stabilized and approached 0, indicating that the adversarial game between the generator and discriminator had reached a balance. At this point, the generated data tended to be similar to the original data. Ideally, the generated data should be statistically similar to the original data.
To ensure that the generated data effectively improves the predictive performance of the model, it is necessary to ensure its similarity to the original data. To evaluate the similarity between the original and synthesized data, the T-SNE similarity measurement method was used to compare the real and generated data.
T-distributed stochastic neighbor embedding (T-SNE) is a nonlinear technique used for data dimensionality reduction and visualization (Cieslak et al., 2020). It maps high-dimensional data into a lower-dimensional space while preserving the local structure of the data points as much as possible. In high-dimensional space, T-SNE uses Gaussian distributions to represent the similarity between data points, while in low-dimensional space, it employs t-distributions to represent similarity.
To confirm the similarity between synthetic and real data, Figure 4 presents a comparison of the dimensionality-reduced distributions of real and synthesized gait data. There is a notable overlap between the generated data (blue samples) and the original data (red samples), which suggests that their distributions are similar (Onyelowe et al., 2023). The data distribution of the final dataset is shown in Figure 5.
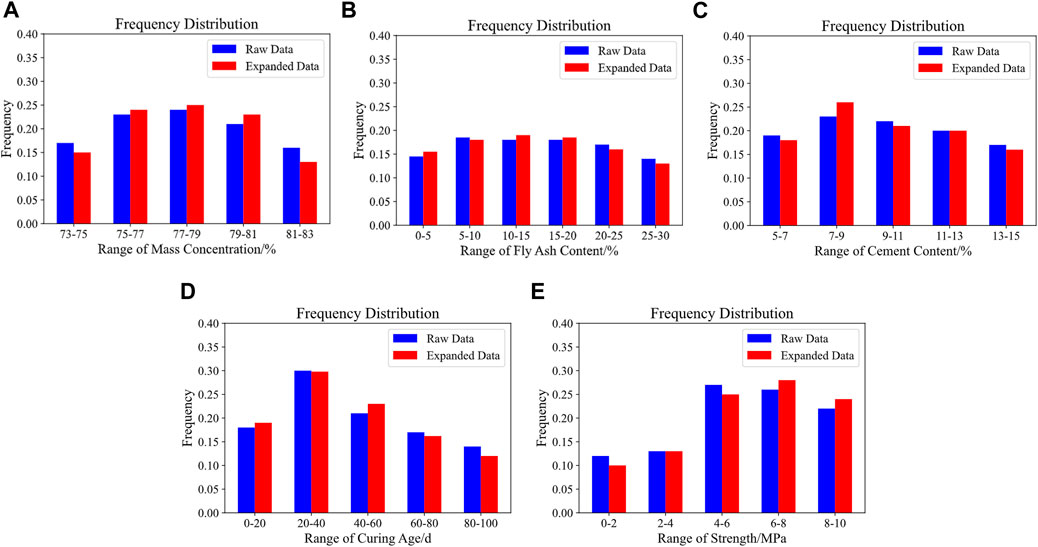
Figure 5. Distribution Map of dataset data. (A) Mass Concentration Distribution Map. (B) Fly Ash Content Distribution Map. (C) Cement Content Distribution Map. (D) Curing Age Distribution Map. (E) Strength Distribution Map.
In which, Figure 5A–D show the distribution of the input data, while Figure 5E shows the distribution of the output data. Analyzing Figures 4, 5, it can be seen that the data generated by GANs has a similar distribution to the original data, indicating homogeneity and suitability for subsequent model training.
2.3 Intensity prediction network construction
2.3.1 Principles of Multilayer Perceptron
The Multilayer Perceptron (MLP) is an artificial neural network model belonging to the category of feedforward neural networks (Taud and Mas, 2018). It consists of multiple layers of neural networks, with each layer containing multiple neurons (nodes), including an input layer, at least one or more hidden layers, and an output layer. The basic structure is depicted in Figure 6.
The MLP is trained using the backpropagation algorithm, with the objective of minimizing the error between the predicted output and the true labels by adjusting the network parameters. During the training process, the backpropagation algorithm utilizes gradient descent or its variants to update the weights and biases of the network.
2.3.2 Principles of Long Short-Term Memory
Long Short-Term Memory (LSTM) is a variant of Recurrent Neural Networks (RNNs) designed specifically for handling sequential data with the ability to maintain long-term dependencies (DiPietro and Hager, 2020). It is tailored for processing and predicting time series data. The core idea behind LSTM the problem of long-term dependencies by controlling the flowis to address of information. It introduces an internal memory unit called the “cell state” and a series of “gates”, including the forget gate, input gate, and output gate, to regulate the update of the cell state and selectively forget information. The structure of an LSTM unit is illustrated in Figure 7.
2.3.3 MLP-LSTM network construction and training
Predicting the strength based on the composition of the filling material and the curing time holds significant engineering implications. Through strength prediction, excessive material usage and time costs can be avoided, thus reducing construction expenses.
Currently, there are four inputs X1, X2, X3, T, where X1, X2, and X3 represent the proportions of fly ash, cement, and gangue, respectively, and T represents the curing age, measured in days. The output is the corresponding strength Y of the filling material. The curing times are currently set as T = 3, 7, 14, 28, 36, and 90 days, with their corresponding proportions of X1, X2, and X3. The original data structure is depicted in Figure 8.
In this scenario, selecting LSTM combined with MLP to construct the neural network, which has four input nodes and one output node, is appropriate. Using MLP as the neural network structure allows learning complex nonlinear relationships, demonstrating strong fitting capabilities. Meanwhile, the LSTM model can capture long-term dependencies between strength data and curing time data. Combining MLP and LSTM can handle mixed problems of non-sequence and sequence data. By leveraging the advantages of both MLP and LSTM, the network can learn complex feature representations and consider their temporal relationships, thus improving the accuracy of strength prediction for filling materials. The structure diagram of the MLP-LSTM network is illustrated in Figure 9.
The training process is as follows:
(1) Data preparation and pre-processing. The data used is augmented data obtained through GANs, consisting of a total of 3,400 sets, with the specific distribution shown in Figure 5. Each set of data includes the content of fly ash, cement, coal gangue, curing age (d), and strength (MPa). Correlation analysis is conducted to ensure that the material content and curing age are independent of each other, followed by normalization.
(2) The dataset is randomly divided into training, testing, and validation sets at a ratio of 7:3:1.
(3) The training data is fed into the constructed MLP-LSTM model, employing grid search and cross-validation methods, along with performance evaluation metrics to select the optimal parameters, and training begins.
(4) The trained model is then tested and evaluated.
The operating environment and the optimized parameter settings determined through experiments are shown in Table 3.
Statistical analysis is conducted on all data in the training and validation sets, and the box plots are shown in Figure 10.
Figure 10 provides a visual comparison of the mean positions of the training and validation sets. The upper and lower boundaries of the box plots display the range of the data, facilitating the observation of their coverage. The width of the boxes and the degree of data dispersion reflect the standard deviation and variance, indicating that the training and validation sets exhibit similar statistical characteristics and can be used for further training.
The loss curves for the training and validation sets during the training process are shown in Figure 11.
Figure 11 shows that around the 75th iteration, the validation set’s loss stabilizes, indicating the model has effectively learned the data features and can generalize to unseen data. Meanwhile, the training set’s loss continues to decrease, suggesting a potential risk of overfitting. Predictions were made on the test set using a random selection of 200 data sets for comparison, as illustrated in Figure 12.
We use Root Mean Square Error (RMSE) as evaluation metrics to assess the model. RMSE measures the square root of the average of the squared differences between predicted values and actual values. It reflects the magnitude of prediction errors, and its unit is the same as that of the dependent variable. A smaller RMSE indicates higher prediction accuracy. The formula for RMSE is shown in Equation 2.
The RMSE of the model is 0.0101, which is relatively small, indicating that the model’s predictions have a small average deviation from the observed values. The R2 value of 0.9822, close to 1, indicates that the model can explain the variance in the observed data very well, showing a very high level of fit. Overall, the model performs exceptionally well and can be used for subsequent predictions of the target variable.
3 Analysis of results and economics
3.1 Prediction results and analysis
Using the trained model for further prediction, we obtained the strength values of filling materials for different filling ratios with curing ages ranging from 3 to 90 days. We selected three sets of contents with solid mass concentrations of 74% and 76%, where the ash content was 20% and the cement content varied at 8%, 10%, and 12% respectively for prediction. The fitted curves of the prediction results are shown in Figure 13.
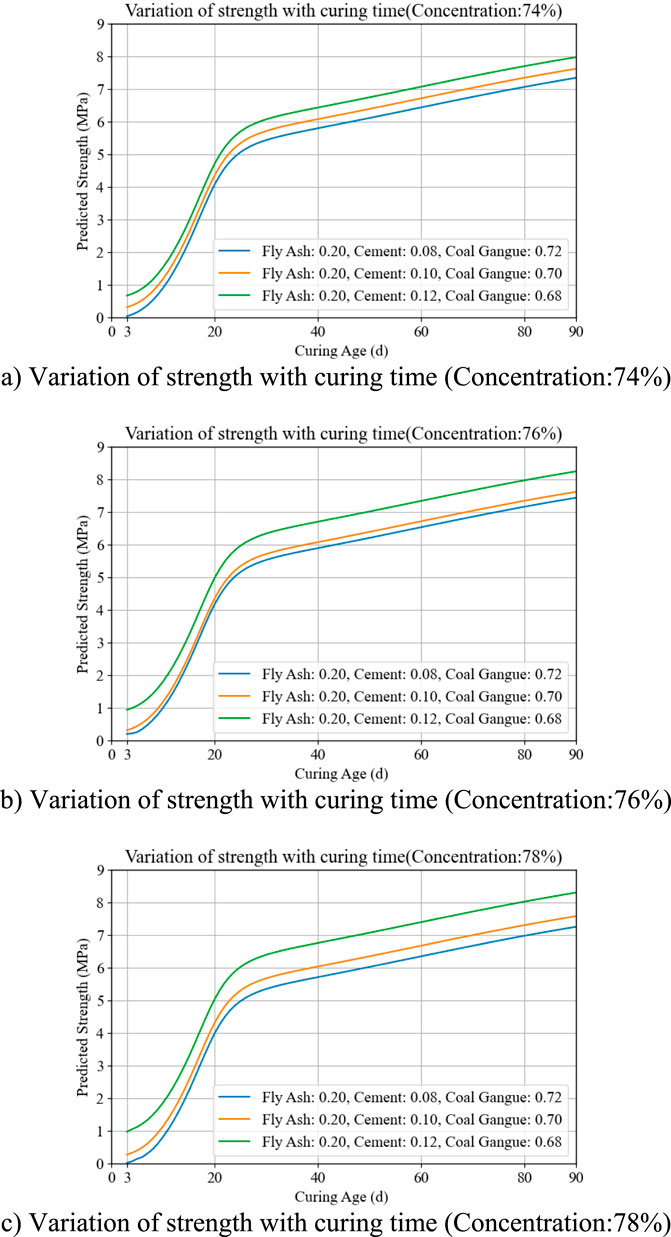
Figure 13. Predicted Results at Different Concentration Levels. (A) Variation of strength with curing time (Concentration:74%). (B) Variation of strength with curing time (Concentration:76%). (C) Variation of strength with curing time (Concentration:78%).
Based on Figure 13, it can be observed that with the extension of the curing age, the rate of change in the strength of the filling material initially increases and then decreases. This indicates that the curing age has a significant impact on the strength of the filling material. Under different mixing ratio conditions, the trend of strength variation in the filling material remains consistent. The predicted results are in accordance with the experimental results and consistent with the findings in literature (Zhou, 2022).
By reviewing the literature Zhou (2022), Cui et al. (2018) and analyzing Figure 10, the curing age is typically chosen around 28 days. Disregarding economic considerations for now, curing age of 14, 28, and 42 days are selected for quantitative analysis of the mixing ratios of the filling material. This analysis aims to investigate the relationship between different mixing ratios and the strength of the filling material. As shown in Figure 14.
At curing age of 14, 28, and 42 days, the average strengths of different filling ratios are obtained under various mass concentrations of the filling material, as illustrated in Figure 12. As depicted in Figure11, there exists a positive correlation between the strength of the filling material and its concentration, with consistent trends across different curing age. Moreover, after the curing age reaches 28 days, further extension of the curing age does not significantly increase the strength of the material, aligning with the predictions from Figure 10.
Under a filling material mass concentration of 78%, quantitative analysis of strength variation is conducted at different curing age while keeping the fly ash content and cement content fixed, as shown in Figure 15.
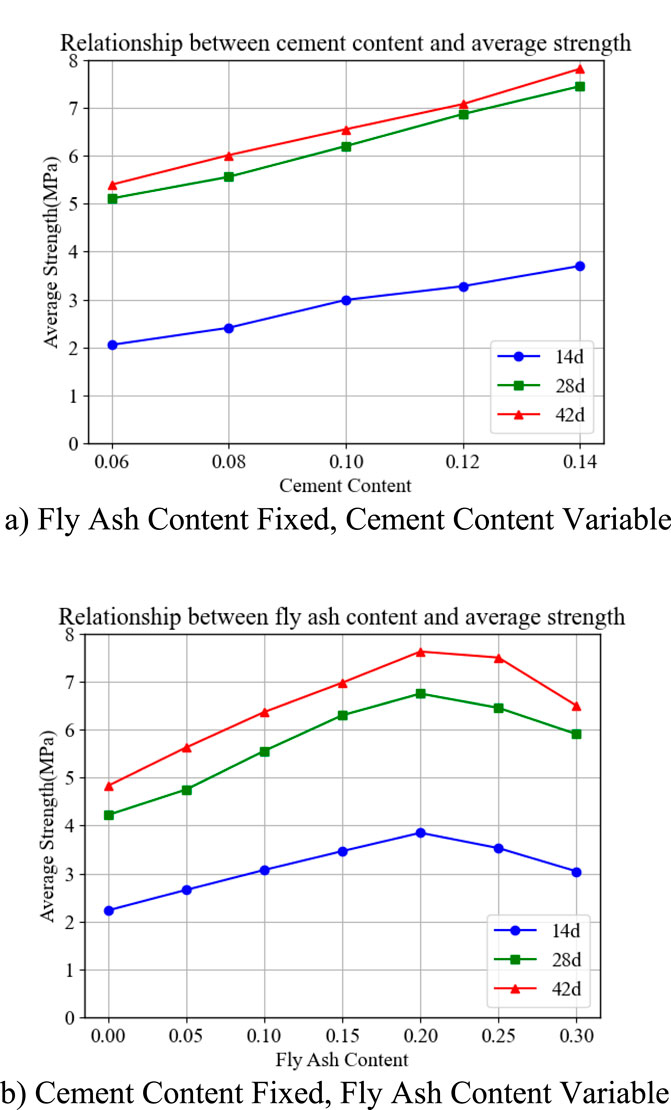
Figure 15. Trend of intensity with mass concentration. (A) Fly ash content fixed, cement content variable. (B) Cement content fixed, fly ash content variable.
Figure 15A depicts the strength variations of filling materials at different cement contents. This graph illustrates the changes in filling material strength under fixed concentrations of filling material and fly ash content but at different curing age. The plotted data points represent the averages of corresponding data from the original dataset. Consistently across different curing age, there is a notable increase in strength with an increase in cement content. This trend occurs because cement serves as the primary binder in filling materials and is the main source of strength (Feng et al., 2016).
Figure 15B illustrates the variation in filling material strength with fly ash content. This graph depicts changes in filling material strength under fixed concentrations of filling material and cement content but at different curing age, with averages taken from corresponding data in the original dataset. As shown in Figure 15B, the trends in strength variation are consistent across different curing age. Strength initially increases and then decreases with an increase in fly ash content. This is because fly ash, as a fine powder material, can fill the voids in concrete, forming a denser structure. In an alkaline environment, it reacts with calcium hydroxide (C-H) to produce hydrated calcium silicate (C-S-H), which helps improve material strength. However, excessive fly ash can hinder the formation of hydration products. Moreover, an excessive fly ash content can lead to a reduction in coal gangue content. Since fly ash is fine-grained, an excess of fine particle size fly ash gradually reduces the strength of the filling material (Yang et al., 2022). The inflection point occurs at a fly ash content of 20%, aligning with experimental data and actual conditions.
Analyzing Figure 15A, B, it is observed that with the extension of the curing age, there is an improvement in strength. However, the rate of strength increase slows down after a curing age of 28 days. Economic analysis is required on this basis to minimize project costs while ensuring material performance to the greatest extent possible.
3.2 Economic analysis
To conduct an economic analysis of the performance of filling materials, it is necessary to calculate their costs. The main components of the filling materials are cement, fly ash, coal gangue, and water, while other materials are disregarded. The prices are as follows: fly ash at 40 yuan/ton, cement at 300 yuan/ton, and coal gangue at 6 yuan/ton. The prices used are the average values in the Chinese market for the period from September 2023 to March 2024. The curing cost per ton of material is considered in terms of time cost, which lacks a specific monetary value estimation. However, generally, the lower the time cost, the better. Ideally, the cost per ton of filling material and its corresponding curing age can be calculated. The purpose of economic analysis is to minimize costs while maintaining suitable performance. Under the premise of appropriate strength, the goal is to select a shorter curing age that aligns more with engineering objectives.
Among these, cement is the main contributor to the cost of filling materials, and adding an appropriate amount of fly ash can enhance material strength. Therefore, to improve cost-effectiveness while ensuring that the strength meets engineering requirements, it is advisable to minimize the use of cement as much as possible. Changes in coal gangue content also affect the strength of the filling material. Increasing the coal gangue content can reduce cement consumption, but it may affect strength performance. Additionally, as the curing age increases, the strength of the filling material also increases, but the rate of increase slows down with longer durations. A curing age that is too short may result in insufficient strength, failing to meet engineering requirements, while an excessively long curing age may lead to unnecessary increases in project costs due to surplus strength.
Generally, the strength requirements for filling materials range between 5 and 8 MPa. It is known that the cost per ton of filling material and the material content are positively correlated with the curing age. Based on the analysis combining Figures 13–15, experimental data, and real-world scenarios, it is recommended to control the concentration between 77% and 79%, fly ash content between 17.9% and 22.7%, cement content between 10.8% and 12.6%, coal gangue content between 64.7% and 71.3%, and maintain the curing age between 28 and 48 days, as this range is more appropriate. Costs can be controlled within the range of ¥33.7 to ¥43.3 per ton.
4 Conclusion
(1) This study utilizes a Long Short-Term Memory network (LSTM) combined with a Multilayer Perceptron (MLP) to construct a neural network model for predicting the strength of filling materials that involve both non-sequential and sequential data. The model exhibits a small average difference between predicted and observed values, effectively explains the variance of the observed data, and demonstrates a high degree of fit, making it suitable for subsequent target variable predictions.
(2) Combining deep learning networks with practical considerations, the results indicate that the strength of filling materials increases with the concentration of filling materials and cement content. The strength initially increases and then decreases as the fly ash content increases. There is a positive relationship between the curing time and the strength of the filling materials; however, when the curing age extends to 28 days, the rate of strength growth slows down.
(3) The constructed predictive model provides reference strength for different filling material ratios at various curing ages, aiding in the selection of material ratios in practical engineering. This allows for optimization of the ratios and curing ages to meet technical requirements and economic benefits for filling. The study suggests maintaining concentrations between 77% and 79%, fly ash content between 17.9% and 22.7%, cement content between 10.8% and 12.6%, coal gangue content between 64.7% and 71.3%, and a curing age between 28 days and 48 days, with costs controlled between 33.7 and 43.3.
In future research, further exploration of the impact of different environmental conditions (such as temperature and humidity) on the strength of filling materials can be undertaken, as well as the introduction of additional variables into the deep learning model to enhance prediction accuracy. Additionally, the study could consider incorporating the characteristics of raw materials from different regions into the model to improve its broad applicability and practicality. Optimal ratios and curing ages can be selected based on actual filling requirements to maximize the effectiveness and economic benefits of the filling process.
Data availability statement
The raw data supporting the conclusions of this article will be made available by the authors, without undue reservation.
Author contributions
YZ: Data curation, Methodology, Writing–original draft. HL: Conceptualization, Investigation, Supervision, Writing–original draft. SF: Investigation, Software, Visualization, Writing–original draft. AZ: Investigation, Software, Visualization, Writing–review and editing. JY: Software, Writing–review and editing. XQ: Supervision, Writing–review and editing.
Funding
The author(s) declare that financial support was received for the research, authorship, and/or publication of this article. This project was supported by “Research on the Path of Optimization and Adjustment of Industrial Structure in Shaanxi Province under Low-carbon Constraints”, The Philosophy and Social Science Research Special Youth Project of Shaanxi Province, 2024, and the project number is 2024QN337.
Acknowledgments
The authors would like to thank the Xi’an Kedagaoxin University.
Conflict of interest
The authors declare that the research was conducted in the absence of any commercial or financial relationships that could be construed as a potential conflict of interest.
Publisher’s note
All claims expressed in this article are solely those of the authors and do not necessarily represent those of their affiliated organizations, or those of the publisher, the editors and the reviewers. Any product that may be evaluated in this article, or claim that may be made by its manufacturer, is not guaranteed or endorsed by the publisher.
References
Cao, H., Gao, Q., Zhang, X., and Guo, B. (2022). Research progress and development direction of filling cementing materials for filling mining in iron mines of China. Gels 8 (3), 192. doi:10.3390/gels8030192
Cao, S., Song, W. D., Xue, G. L., et al. (2015). Test of strength reduction of cemented tailing s filling considering layering character. Rock Soil Mech. 36 (10), 2869–2876. doi:10.16285/j.rsm.2015.10.017
Cao, S., Song, W. D., Xue, G. L., et al. (2016). Mechanical characteristics variation of stratified cemented tailing backfilling and its failure modes. J. China Univ. Min. and Technol. 45 (4), 717–722+728. doi:10.13247/j.cnki.jcumt.000537
Cieslak, M. C., Castelfranco, A. M., Roncalli, V., Lenz, P. H., and Hartline, D. K. (2020). t-Distributed Stochastic Neighbor Embedding (t-SNE): a tool for eco-physiological transcriptomic analysis. Mar. Genomics 51, 100723. doi:10.1016/j.margen.2019.100723
Cui, H. H., Fei, G. L., Yao, S. J., et al. (2018). Experimental study of mechanical properties of cement soil of different curing time subjected to freezing-thawing cycles. J. Glaciol. Geocryol. 40 (1), 110–115. doi:10.7522/j.issn.1000-0240.2018.0014
Ding, Z., Liu, P., Cui, P., and Hong, C. (2023). Strength development and environmental assessment of full tailings filling materials with various water-to-binder ratios. Metals 13 (1), 122. doi:10.3390/met13010122
DiPietro, R., and Hager, G. D. (2020). “Deep learning: RNNs and LSTM,” in Handbook of medical image computing and computer assisted intervention (Academic Press), 503–519. doi:10.1016/B978-0-12-816176-0.00026-0
Feng, F., Li, L., Zhang, J., Yang, Z., and Chi, X. (2021). Strength prediction of coal-based solid waste filler based on bp neural network. Front. Mater. 8, 767031. doi:10.3389/fmats.2021.767031
Feng, G. R., Jia, X. Q., Guo, Y. X., et al. (2016). Study on mixture ratio of gangue-waste concrete cemented paste backfill. J. Min. and Saf. Eng. 33 (6), 1072–1079. doi:10.13545/j.cnki.jmse.2016.06.017
Goodfellow, I. J., Pouget-Abadie, J., Mirza, M., Xu, B., Warde-Farley, D., Ozair, S., et al. (2014). Generative adversarial networks. Commun. ACM 63 (11), 139–144. doi:10.1145/3422622
Guo, L. J., Liu, G. S., Ma, Q. H., et al. (2022). Research progress on mining with backfill Technology of underground metalliferous mine. J. China Coal Soc. 47 (12), 4182–4200. doi:10.13225/j.cnki.jccs.2022.0720
Hefni, M., and Hassani, F. (2020). Experimental development of a novel mine backfill material: foam mine fill. Foam Mine Fill. Miner. 10 (6), 564. doi:10.3390/min10060564
Hong, Z., Li, Z., Du, F., Xu, L., and Zhu, C. (2023). Experimental investigation of the mechanical properties and large-volume laboratory test of a novel filling material in mining engineering. Geomechanics Geophys. Geo-Energy Geo-Resources 9 (1), 46. doi:10.1007/s40948-023-00582-8
LeCun, Y., Bengio, Y., and Hinton, G. (2015). Deep learning. Nature 521 (7553), 436–444. doi:10.1038/nature14539
Liu, S. L., Liu, G. L., Li, G. C., et al. (2021). Effect of curing conditions on the early mechanical properties and microstructure of cemented backfill. Nonferrous Met. Eng. 11 (8), p83. doi:10.3969/j.issn.2095-1744.2021.08.011
Liu, T., Zhao, G., Qu, B., and Gong, C. (2024). Characterization of a fly ash-based hybrid well cement under different temperature curing conditions for natural gas hydrate drilling. Constr. Build. Mater. 445, 137874. doi:10.1016/j.conbuildmat.2024.137874
Niaki, M. H., Ahangari, M. G., and Pashaian, M. (2022). A material-independent deep learning model to predict the tensile strength of polymer concrete. Compos. Commun. 36, 101400. doi:10.1016/j.coco.2022.101400
Onyelowe, K. C., Ebid, A. M., and Ghadikolaee, M. R. (2024b). GRG-optimized response surface powered prediction of concrete mix design chart for the optimization of concrete compressive strength based on industrial waste precursor effect. Asian J. Civ. Eng. 25 (1), 997–1006. doi:10.1007/s42107-023-00827-7
Onyelowe, K. C., Ebid, A. M., Mahdi, H. A., Onyelowe, F. K. C., Shafieyoon, Y., Onyia, M. E., et al. (2023). AI mix design of fly ash admixed concrete based on mechanical and environmental impact considerations. Civ. Eng. J. 9, 27–45. doi:10.28991/CEJ-SP2023-09-03
Onyelowe, K. C., Ebid, A. M., Mahdi, H. A., Soleymani, A., Jahangir, H., and Dabbaghi, F. (2022a). Optimization of green concrete containing fly ash and rice husk ash based on hydro-mechanical properties and life cycle assessment considerations. Civ. Eng. J. 8 (12), 3912–3938. doi:10.28991/CEJ-2022-08-12-018
Onyelowe, K. C., Ebid, A. M., Riofrio, A., Soleymani, A., Baykara, H., Kontoni, D. P. N., et al. (2022b). Global warming potential-based life cycle assessment and optimization of the compressive strength of fly ash-silica fume concrete; environmental impact consideration. Front. Built Environ. 8, 992552. doi:10.3389/fbuil.2022.992552
Onyelowe, K. C., Ebid, A. M., Shadi, H., et al. (2024a). The influence of nano-silica precursor on the compressive strength of mortar using Advanced Machine Learning for sustainable buildings. Asian J. Civ. Eng. 25 (2), 1135–1148. doi:10.1007/s42107-023-00832-w
Qiu, J., Luan, X., Cheng, K., Guan, X., Yang, M., and Xiao, Z. (2023). Study on the modification effect and mechanism of tailings powder on coal gangue-based mining cementitious filling material. Environ. Sci. Pollut. Res. 30 (16), 46038–46057. doi:10.1007/s11356-023-25459-x
Rong, K., Lan, W., and Li, H. (2020). Industrial experiment of goaf filling using the filling materials based on hemihydrate phosphogypsum. Minerals 10 (4), 324. doi:10.3390/min10040324
Roy, R., Chakraborty, S., Bisai, R., Pal, S. K., and Mishra, S. (2023). Gravity blind backfilling of abandoned underground mine voids using suitable mix proportion of fill materials and method of filling. Geotechnical Geol. Eng. 41 (3), 1801–1819. doi:10.1007/s10706-022-02371-8
Taud, H., and Mas, J. F. (2018). Multilayer perceptron (MLP). Geomat. approaches Model. Land change scenarios 2018, 451–455. doi:10.1007/978-3-319-60801-3_27
Wagner, N., and Rondinelli, J. M. (2016). Theory-guided machine learning in materials science. Front. Mater. 3. doi:10.3389/fmats.2016.00028
Wang, C., Liu, Y., Hu, H., Li, Y., and Lu, Y. (2019). Study on filling material ratio and filling effect: taking coarse fly ash and coal gangue as the main filling component. Adv. Civ. Eng. 2019. doi:10.1155/2019/2898019
Yang, Z. J., He, M., and Wu, Y. (2022). Mechanical properties and road performance of slag-fly ash geopolymer stabilized sludge. Bull. Chin. Ceram. Soc. 41 (2), 693–703+724. doi:10.3969/j.issn.1001-1625.2022.2.gsytb202202038
Zhang, G. Z., Ge, J. C., Zhang, C. X., et al. (2021). Review on the microstructure formation mechanism in concrete material under different curing regimes. Mater. Rep. 35 (15), 15125–15133. doi:10.11896/cldb.20060297
Zhao, H., Wang, Y., Liu, X., Wang, X., Chen, Z., Lei, Z., et al. (2024). Review on solid wastes incorporated cementitious material using 3D concrete printing Technology. Case Stud. Constr. Mater. 21, e03676. doi:10.1016/j.cscm.2024.e03676
Zheng, H. C., and Li, M. (2005). Analysis about the risk of underground mining area. Ground Press. Strata Control 2005 (4), 127–129. doi:10.3969/j.issn.1673-3363.2005.04.052
Zhou, P. F. (2022). Experimental study on compressive strength and failure mode of cemented tailings backfill at different curing temperatures. Nonferrous Met. Eng. 12 (8). doi:10.3969/j.issn.2095-1744.2022.08.022
Keywords: curing age, MLP, LSTM, strength, prediction, economic analysis
Citation: Zhang Y, Liang H, Fei S, Zhang A, Yu J and Qin X (2024) Prediction and analysis of strength and economic feasibility of filling materials under the influence of mix proportion and curing age. Front. Mater. 11:1428859. doi: 10.3389/fmats.2024.1428859
Received: 07 May 2024; Accepted: 31 October 2024;
Published: 14 November 2024.
Edited by:
Chady Ghnatios, Arts et Métiers Institute of Technology, FranceReviewed by:
Mahmood Ahmad, University of Engineering and Technology, Peshawar, PakistanAhmed M. Ebid, Future University in Egypt, Egypt
Copyright © 2024 Zhang, Liang, Fei, Zhang, Yu and Qin. This is an open-access article distributed under the terms of the Creative Commons Attribution License (CC BY). The use, distribution or reproduction in other forums is permitted, provided the original author(s) and the copyright owner(s) are credited and that the original publication in this journal is cited, in accordance with accepted academic practice. No use, distribution or reproduction is permitted which does not comply with these terms.
*Correspondence: Huizhen Liang, MjIyMDYwNDMwNDNAc3R1Lnh1c3QuZWR1LmNu