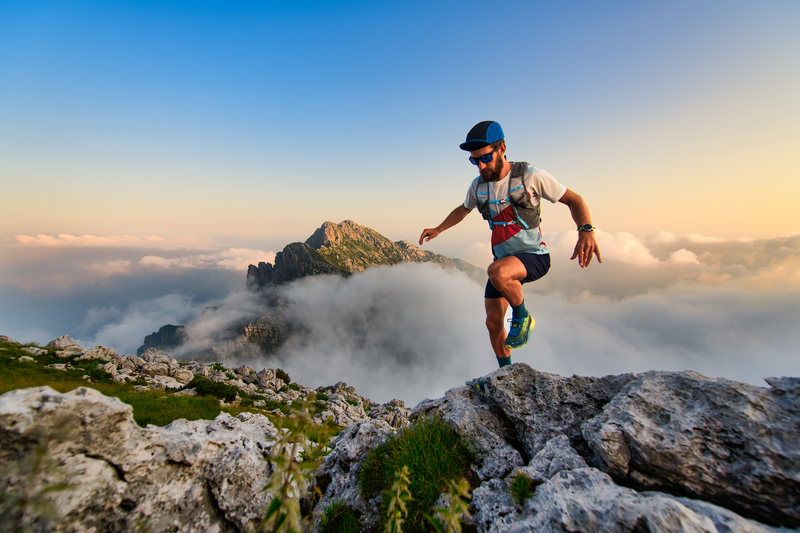
95% of researchers rate our articles as excellent or good
Learn more about the work of our research integrity team to safeguard the quality of each article we publish.
Find out more
ORIGINAL RESEARCH article
Front. Mar. Sci. , 15 November 2024
Sec. Marine Pollution
Volume 11 - 2024 | https://doi.org/10.3389/fmars.2024.1491242
This article is part of the Research Topic Water Resources Management and Pollution Control in Islands and Coastal Areas View all 3 articles
To understand the pollution characteristics and potential sources of heavy metal(loid)s in the nearshore sediments of Qionghai, 93 surface sediment samples were collected from the region. The concentrations of 20 elements, including Fe, Mg, Ca, Ti, Mn, Ba, Cr, Sr, Ni, Cu, Zr, As, Hg, Se, Be, Co, Mo, Cd, Ga, and Pb, were measured. The extent of contamination and ecological risk posed by these heavy metals/metalloids were evaluated using the geo-accumulation index, potential ecological risk index, and Nemerow comprehensive risk index. Additionally, correlation analysis, principal component analysis (PCA), and positive matrix factorization (PMF) were employed to identify the potential sources of these elements in the sediments. The findings reveal the following: (1) The mean concentrations of Fe, Ca, Mg, Ti, Cu, Sr, Zr, Mo, Cd, Pb, Hg, As, and Se exceed the background values for shallow sea sediments in China. Notably, Ca, Ti, Sr, Zr, Mo, Hg, and As exhibit coefficients of variation greater than 51%, indicating significant spatial variability primarily driven by anthropogenic activities. (2) The ecological risk assessment identifies Sr, Hg, and As as the principal pollutants and key potential ecological risk factors in the study area, necessitating prioritization in subsequent monitoring efforts. (3) Correlation and source analysis suggest that As and Mn primarily originate from agricultural activities, Sr, Ca, and Mg from aquaculture, Zr, Ti, Mo, Se, Pb, Be, Co, Cu, Ga, Ni, Fe, and Cd from natural sources, and Hg, Ba, and Cr from transportation sources. Additionally, this study identified Sr, Hg, and As as the primary pollutants in the Qionghai nearshore area, with sources predominantly linked to agriculture, aquaculture, and traffic. Regular monitoring will help track the effectiveness of implemented control measures and provide data for ongoing risk assessments, ensuring the protection and sustainability of the marine environment.
The nearshore marine area represents a zone of dynamic interaction between the ocean and land, encompassing diverse ecosystems including estuaries, bays, coral reefs, and seagrass beds. This region is characterized by a rich natural environment and abundant resources (Gao et al., 2014; Arikibe and Prasad, 2020). Since the mid-20th century, rapid economic and social development in coastal regions has driven significant growth in manufacturing, agriculture, transportation, and tourism. While these developments have provided substantial economic benefits and improved quality of life, they have also intensified environmental challenges in nearshore marine areas, with heavy metal pollution being a critical concern. Heavy metal(loid)s pose significant challenges due to their high toxicity, persistence, and resistance to degradation. Their ability to bioaccumulate through the food chain further amplifies their potential to cause serious ecological harm (Mirza et al., 2019; Lu et al., 2020).
Sediments serve as major sinks for heavy metal(loid)s, playing a critical role in the environmental cycling of these contaminants. Variations in environmental conditions, such as pH, redox potential, and organic matter content, along with processes like sediment resuspension, can trigger the release of heavy metal(loid)s from sediments into the overlying water column, resulting in secondary pollution (Gao et al., 2014; Tramonte et al., 2018). Once released, these contaminants can bioaccumulate and biomagnify through the food chain, posing significant threats to local biota and ecosystems. Consequently, understanding the spatial distribution and sources of heavy metal(loid) pollution in sediments is vital for assessing the potential ecological risks and contamination status of nearshore marine environments. Natural and anthropogenic sources are recognized as the two major contributors to heavy metal(loid) contamination in sediments. Natural sources encompass processes such as volcanic eruptions and the weathering of parent rocks (Niu et al., 2021), Anthropogenic sources, including industrial emissions, vehicular discharges, fossil fuel combustion, and the application of pesticides, fertilizers, and animal feed, are significant contributors to heavy metal(loid)s contamination (Sheng et al., 2022). Since the advent of industrialization, the release of heavy metal(loid)s from human activities has greatly exceeded natural sources, establishing itself as the primary contributor to environmental contamination (Nriagu, 1979). Given this, selecting appropriate source apportionment methods tailored to specific environmental conditions is critical for accurately identifying the origins of heavy metal(loid) pollutants and enabling targeted remediation efforts.
Among the widely adopted methods for source apportionment are receptor models, principal component analysis/factor analysis (PCA/FA), and positive matrix factorization (PMF) (Nan et al., 2011; Fang et al., 2016; Xiao et al., 2021; Ouyang et al., 2024). PMF, endorsed by the U.S. Environmental Protection Agency, is a quantitative source apportionment model with distinct advantages. It does not require detailed source profile information and can effectively manage missing or imprecise data. Additionally, PMF applies non-negative constraints to the factor decomposition matrix, ensuring that the resulting source profiles and contribution rates remain positive, thereby producing more accurate and interpretable results (Rizzo and Scheff, 2004).
Qionghai located in the eastern part of Hainan Province, is a key coastal tourism area where environmental quality is crucial for regional ecosystem stability and sustainable socio-economic development. In recent years, the rapid expansion of tourism, commerce, aquaculture, and land reclamation, driven by the growth and influence of the Boao Forum for Asia, has led to the overexploitation of bay resources. Concurrently, agricultural activities and urban wastewater discharge have intensified ecological pressure on the nearshore marine environment. Although research on trace metals in Hainan has grown, most studies have focused on river outlets and estuarine regions (Nan et al., 2011; Hu et al., 2013; Fu et al., 2013; Fu et al., 2023a; Jiang et al., 2023), often with limited sampling density and greater distances from the shore. This study aims to address these gaps by establishing 93 sediment sampling points in the nearshore area of Qionghai and analyzing the concentrations of heavy metal(loid)s in the sediments. The research assesses the pollution status and potential ecological risks associated with these elements and conducts a preliminary source analysis. This comprehensive assessment of the ecological quality of Qionghai’s nearshore sediments is intended to provide scientific evidence to support environmental protection and the sustainable use of coastal resources in the region.
In June and July 2020, 93 sediment sampling points were established in the nearshore waters of Qionghai. Surface sediment samples (0–10 cm) were collected using a grab sampler, with the sampling locations displayed in Figure 1. The procedures for sample collection, storage, and transportation were rigorously followed in accordance with the “Marine Monitoring Specifications Part 3: Sample Collection, Storage, and Transportation” (GB 17378.3-2007).
The sediment samples collected for this study were analyzed at Laboratory of the Golden Ninth Detachment of the Chinese People’s Police Armed Forces. Samples were air-dried, ground, and sieved through a 200-mesh screen before undergoing microwave digestion to prepare the test solutions. The concentrations of Fe, Mg, Ca, Ti, Mn, Ba, Cr, Sr, Ni, Cu, and Zr were measured using an X-ray fluorescence spectrometer (Axios Pw4400). As, Hg, and Se concentrations were determined using a dual-channel atomic fluorescence spectrometer (AFS-9800), while Be, Co, Mo, Cd, Ga, and Pb were analyzed using an inductively coupled plasma mass spectrometer (ICAPQ).
The Index of geo-accumulation (Igeo), also known as the Müller Index, is a comparative measure that evaluates the concentration of metals in soil samples against their background levels. Initially developed for assessing sediment pollution, this index has been widely adopted since the late 1960s for evaluating the pollution levels of heavy metal(loid)s in soils. A key advantage of the Igeo is its consideration of natural diagenetic processes that may cause variations in background values, distinguishing it from other pollution assessment methods. The calculation formula is as follows:
In Equation 1, represents the concentration of heavy metal(loid)s in the soil (mg·kg-1); K is a constant factor (typically K=1.5) that accounts for natural fluctuations in background values; and is the reference background concentration of heavy metal(loid)s in the soil (mg·kg-1). The study area is located in the nearshore region of eastern Hainan Island. Therefore, this study uses the background concentrations of heavy metal(loid)s in Chinese shallow sea sediments as reported by Zhao and Yan (1993). The background values for Fe, Mg, Mn, Ti, Ba, Cr, Cu, Ni, Sr, Zr, Co, Ga, Mo, Cd, Pb, Hg, As, and Se are as follows: 3.1%, 1.11%, 0.05%, 0.36%, 412 mg·kg-1, 61 mg·kg-1, 15 mg·kg-1, 24 mg·kg-1, 230 mg·kg-1, 210 mg·kg-1, 12mg·kg-1, 14 mg·kg-1, 0.52 mg·kg-1, 0.065 mg·kg-1, 20 mg·kg-1, 0.025 mg·kg-1, 7.7 mg·kg-1, 0.15mg·kg-1. The classification of the Igeo and the corresponding pollution levels are presented in Table 1.
The Nemerow Integrated Risk Index (NIRI) is a comprehensive tool for assessing the ecological risk levels of heavy metal(loid)s in regional soils. This index integrates the strengths of the Potential Ecological Risk Index (PERI) and the Nemerow Integrated Pollution Index (NIPI), incorporating both the toxicity of environmental pollutants to biological communities and the toxic response factors of each heavy metal(loid). As a result, NIRI offers a more precise evaluation of the environmental impact of multiple elements (Men et al., 2020). The calculation formula is as follows:
In Equations 2 and 3, represents the potential ecological risk factor for an individual metal. and are the same as defined in Equation 1. is the biological toxicity coefficient for different metals. Based on existing research and the heavy metal(loid) concentrations measured in this study, toxicity response coefficients were determined for 10 elements: Hg, Cd, As, Pb, Cu, Ni, Co, Cr, Mn, and Ti. The coefficients are 40, 30, 10, 5, 5, 5, 5, 2, 1, and 1, respectively (Xu et al., 2008). NIRI represents the Nemerow Index, is the maximum value among all single-factor pollution indices for heavy metal(loid)s and is the average value of all single-factor pollution indices. The classification standards for and NIRI are presented in Table 1.
CV serves as an indicator of the spatial variability of heavy metal(loid) elements in sediments. A CV below 20% signifies low variability, 20%-51% indicates moderate variability, 51%-100% represents high variability, and a CV greater than 100% denotes extremely high variability (Phil-Eze, 2010). Low variability typically suggests similar pollution sources or diffuse pollution, whereas high variability points to significant differences in pollution sources or the presence of prominent point source pollution.
PCA is a statistical technique used for dimensionality reduction or feature extraction. By applying linear transformations, it reduces the original set of numerous, correlated variables to a smaller set of uncorrelated principal components. These components capture the essential information from the original data, allowing for the explanation of the majority of the variance with fewer representative factors. This method is also effective in inferring potential pollution sources, making it a valuable tool in environmental studies.
In this study, there were 93 soil samples and each sample was analyzed for 20 heavy metals. The accumulations of each soil heavy metal are transformed into a dimensionless standardized form,
In Equation 4, Aik is the accumulation value of heavy metal i at location k, i and are the mean and standard deviation, respectively, of accumulations of heavy metal i in the study area. and the PCA model is expressed as
In Equation 5, k=1,…, p denote sources, and gki and hij are the factor loadings and factor scores, respectively. This equation is solved by eigenvector decomposition, Varimax rotation is often used to redistribute the variance and provide a more interpretable structure on the factors. It is worth noting that, in the present work, the PCA was applied on the spatial soil heavy metal accumulation distributions considered at dates other than the sampling date for source identification purposes using factor extraction with eigenvalues > 1 after varimax rotation.
PMF, developed by Paatero and Tapper (1994), is a quantitative source apportionment model grounded in multivariate factor analysis. PMF decomposes the sample data matrix (X) into two matrices: a factor contribution matrix (G) and a factor profile matrix (F). This approach enables the identification of factor profiles and the quantitative determination of factor contributions for each sample. The fundamental equation governing PMF is as follows:
In Equation 6, the sample concentration matrix (X) represents the concentrations of m compounds across n samples(n×m); G is the contribution matrix(n×p); F is the factor profile matrix(p×m)and E denotes the residual matrix(n×m). The equation can be transformed into the following form:
In Equation 7, represents the concentration of the jth element in the ith sample, which forms the sample concentration matrix. denotes the contribution of source k in the ith sample, forming the source contribution matrix. is the concentration of the jth element in source k, representing the source profile matrix. denotes the residual matrix, which corresponds to the portion of the concentration matrix E that cannot be explained by the PMF model.
The PMF model analyzes the matrices mentioned above by defining an “Objective Function” (Q) and minimizing its value. When the value of the objective function Q is minimized, the model decomposes the receptor concentration matrix X into matrix G matrix (factor contribution matrix) and the F matrix (factor profile matrix). The calculation formula is as follows:
In Equation 8, represents the uncertainty of the jth element in the ith sample. Uncertainty directly affects the weighting of particulate matter mass concentration and component concentration data input into the PMF model. The calculation formula is as follows:
For C≤MDL:
For C>MDL:
In Equations 9 and 10, EF represents the error factor, which typically ranges from 0.1 to 0.6. A larger error factor is used when sample concentrations are unstable or near the detection limit. Additionally, when there is a significant amount of missing concentration data, a larger error factor may be applied. In this study, EF is set to 0.1 based on the specific conditions. C denotes the measured element concentration, and MDL refers to the method detection limit.
Descriptive statistics were conducted using Microsoft Excel 2016. Correlation analysis and PCA modeling for source identification of heavy metal(loid)s in the soil were performed using SPSS 22.0. Source apportionment was carried out using EPA PMF 5.0 software. ArcGIS 10.0 was utilized for mapping the study area and sampling points, with Kriging spatial interpolation employed to create spatial distribution maps of soil heavy metal(loid) concentrations. All other figures were generated using OriginPro 2021.
The concentration characteristics of 20 elements in the sediment samples from the study area are summarized in Table 2. The mean concentrations, in descending order, are as follows: Ca (20.07%) >Fe (3.14%) >Mg (1.84%) >Ti (0.65%) >Sr (1581.5 mg·kg-1) >Zr (957.65mg·kg-1) >Ba (116.78 mg·kg-1) >Cr (45.67 mg·kg-1) >Pb (20.28mg·kg-1) >Cu (17.08 mg·kg-1) >Ni (16.34 mg·kg-1) >Ga (13.09 mg·kg-1) >As (11.94mg·kg-1) >Co (6.49mg·kg-1) >Be (1.29 mg·kg-1) >Mo (0.78 mg·kg-1) >Se (0.18 mg·kg-1) >Cd (0.09 mg·kg-1) >Hg (0.04 mg·kg-1). Notably, the mean concentrations of Fe, Ca, Mg, Ti, Cu, Sr, Zr, Mo, Cd, Pb, Hg, As, and Se exceed the background values for Chinese shallow sea sediments. Furthermore, the mean concentrations of Cr, Cu, Cd, Hg, and As are higher than the background values for Hainan Island soils. These findings indicate that the surface sediments in the study area may be contaminated with heavy metal(loid)s.
In this study, the CV for Fe, Mg, Cu, Cd, Pb, and Se fall within the 20%-51% range, indicating moderate variability. Similarly, the CV for Ca, Mn, Ba, Ni, Sr, Be, Co, Mo, Hg, and As are within the 51%-100% range, reflecting high variability. Notably, the CV for Ti, Cr, Zr, and Ga exceed 100%, indicating extremely high variability. Specifically, Ti, Zr, and Ga exhibit exceptionally high concentrations at stations qwbc80 and qwbc138, while Cr shows similarly high values at station qwbc85, suggesting prominent point source pollution. These findings indicate that the enrichment of heavy metal(loid)s in the sediments of the study area is potentially influenced by human activities. Guo et al. (2012) and Zhao et al. (2019) suggested that an increase in the coefficient of variation is indicative of a higher likelihood that soil heavy metals content is influenced by anthropogenic activities. Therefore, the coefficient of variation can serve as an initial indicator for determining whether heavy metal levels in the study area are impacted by human interference.
Igeo was used to assess the pollution levels of Fe, Mg, Ba, Sr, Zr, Be, Co, Mo, Cu, Ni, Cr, Cd, Pb, and As. The results are presented in Figure 2. The mean Igeo values of heavy metal(loid)s in sediments, in descending order, are: Sr (1.53) > Mg (0.11) > Cd (-0.32) > Hg (-0.33) = Ti (-0.33) > As (-0.47) > Cu (-0.48) > Zr (-0.50) > Fe (-0.76) > Pb (-0.91) > Mo (-1.16) > Mn (-1.29) > Ni (-1.59) > Cr (-1.72) > Co (-1.80) > Ba (-3.05).
Among the sediment samples, Ba and Co exhibited no pollution (Igeo ≤ 0) across all sites, while 98% of samples for Cr and Ni, and 97%, 92%, 88%, 86%, 84%, 73%, 67%, 53%, 46%, 43%, 40%, 30% for Mo, Fe, Mn, Pb, Cu, As, Cd, Hg, Zr, Ti, Mg, and Sr, respectively, also showed no pollution. A proportion of the samples exhibited no pollution to moderate pollution (0<Igeo ≤ 1), including 60% for Mg, 52% for Ti, 32% for Cd, 30% for Hg, and lower percentages for Zr, Cu, Pb, As, Mn, Fe, and Sr. Around 1% of samples for Cr, Ni, and Mo fell into this category.
Moderate pollution (1<Igeo ≤ 2) was observed in 27%, 17%, 15%, and 8% of samples for Zr, Hg, As, and Sr, respectively. Additionally, 3% of samples for Mn, Ti, and 1% for Ni, Mo, and Cd displayed moderate pollution. Moderate to heavy pollution (2<Igeo ≤ 3) was observed for 34% and 6% of samples for Sr and Zr, respectively, and 1% for Cr, Mo, and As. Heavy pollution (3<Igeo ≤ 4) was found in 23%, 2%, and 1% of samples for Sr, Ti, and Zr, respectively, while 8% of samples for Sr showed heavy to extremely heavy pollution (4<Igeo ≤ 5). Finally, 2% of Zr samples were categorized as having extreme pollution (Igeo > 5).
These results indicate that Ba, Cu, Co, Pb, Fe, and Mg are in a clean state and pose minimal risk of surface sediment contamination. However, Sr, Zr, Hg, and As present moderate to high pollution levels at over 65%, 37%, 17%, and 16% of the sampling sites, respectively.
The results of the potential ecological risk index for heavy metal(loid)s (Figure 3) indicate that the mean values for the elements in the sediments, in descending order, are as follows: Hg (66.31) > As (15.51) > Cu (5.69) > Pb (5.07) > Ni (3.40) > Co (2.71) > Ti (1.80) > Cr (1.50) > Mn (1.01) > Cd (0.13). Among these, the sediments from all sampling sites exhibited slight ecological risk for Cr, Cu, Ni, Co, Pb, Mn, Ti, and 92% and 40% of the sites for As and Hg, respectively (<40). Moderate ecological risk (<80) was observed for Hg and As at 24% and 8% of the sites, respectively. Hg presented a strong ecological risk (80≤<160) at 35% of the sites, and very strong ecological risk (160≤<320) at 1% of the sites. These findings suggest that Hg and As are the primary contributors to ecological risk in the surface sediments of the study area, particularly Hg, which aligns with the geo-accumulation index results. Although Hg’s pollution level is relatively low, its high potential ecological risk is attributed to its significant toxicity coefficient.
Except for the pollution and ecological risk assessment of the individual metal(loid)s, the integrated assessment was also performed. The Nemerow Integrated Risk Index (NIRI) results are shown in Figure 4. Figure 4A indicates that the contribution of to NIRI, ranked from highest to lowest, is: Hg (64.3%) > As (15.0%) > Cu (5.7%) > Pb (4.9%) > Ni (3.4%) > Co (2.7%) > Ti (1.8%) > Cr (1.5%) > Mn (1%) > Cd (0.1%). Analysis of the NIRI results for the 93 sediment samples (Figure 4B) reveals that 31% of the samples exhibit moderate ecological risk (40≤<80), and 20% exhibit strong ecological risk (80≤ <160). The ecological risk contribution of heavy metal(loid)s, as measured by (Li et al., 2022a), identifies Hg as the primary element contributing to ecological risk in the surface sediments. This finding is consistent with previous studies (Zhuang et al., 2020; Hu et al., 2024), both of which highlight the high ecological risk posed by Hg in sediments. As noted by Sun et al. (2022), even at very low concentrations, Hg can significantly impact human health and ecosystem stability. Therefore, Hg should be prioritized as a key focus in the ongoing management efforts in this study area. Recent studies frequently rely on RI values to evaluate the ecological risks of metal(loid)s in sediments (Ji et al., 2019; Zhang et al., 2019). However, since RI merely sums the risks of individual metal(loid)s, the integrated risk becomes significantly influenced by the number of metal(loid)s considered. In this study, the NIRI method is employed to correct the variability in RI due to the number of metal(loid)s, improving the comparability of results across different studies.
Correlation analysis and cluster analysis are commonly employed to qualitatively identify the sources of heavy metal(loid)s, as metals that share a common or composite source often exhibit significant correlations (Fu et al., 2023a). In this study, Spearman correlation analysis of heavy metal(loid) elements in the sediment samples reveals a strong positive correlation between Fe and common rock-forming elements such as Ti and Mn (p<0.001). This suggests that Fe in the sediments primarily originates from terrestrial detritus and is closely linked to the precipitation of authigenic Fe-Mn oxides. Additionally, the significant positive correlations between Fe and elements like Ba, Cr, Cu, Ni, Zr, Be, Co, Ga, Mo, Pb, and Hg indicate that these metals may be associated with terrestrial or allochthonous silicate minerals (Miola et al., 2016). Similarly, Ti, as a representative indicator of terrestrial detritus, shows positive correlations with other metals (like as Mn, Ba, Cr, Cu, Ni, Zr, Be, Co, Ga, Mo, Pb, and Hg), supporting a similar origin. In contrast, Sr exhibits significant negative correlations with other heavy metal(loid)s (like as Fe, Mn, Ti, Ba, Cr, Cu, Ni, Be, Co, Ga, Pb, Hg and Se), suggesting that Sr may have a different source or behavior. Previous research by Murray and Leinen (1993) found that Sr in marine sediments is typically biogenic, primarily derived from biological processes. As a biophilic element, Sr is mainly enriched in coarse-grained calcareous shells and detritus. The study area, located in a low-latitude region with a hot climate and high biological productivity, has a high biogenic carbonate content, which dilutes terrestrial detrital deposits and highlights biogenic sedimentation, leading to peaks in Sr content. The region’s high biological activity results in abnormally high CaCO3 levels, which in turn elevate Sr concentrations. These findings suggest that metal concentrations in sediments are influenced not only by the sources of metal input but also by various geochemical processes.
Cluster analysis using the “complete” method shows that the 20 heavy metal(loid) elements in the sediments can be grouped into four clusters (Figure 5B): the first cluster includes Ga, Ti, Zr, and Ba; the second cluster includes Co, Be, Hg, Cr, Pb, and Fe; the third cluster includes Cd, Mo, Ni, Mn, Se, and Cu; and the fourth cluster includes As, Mg, Sr, and Ca. Correlation analysis results reveal highly significant correlations (p<0.01) between Ga-Ti-Zr-Ba, Co-Be-Hg-Cr-Pb-Fe, Cd-Mo, Ni-Mn-Se-Cu, As-Mg and Sr-Ca, which are consistent with the cluster analysis findings. Based on these results, it can be preliminarily inferred that Ga, Ti, Zr, and Ba share a common or similar source, while Co, Be, Hg, Cr, Pb, and Fe likely originate from the same source. Although Cd, Mo, Ni, Mn, Se, Cu, As, Mg, Sr, and Ca may have a common source, their modes of migration could differ, which aligns with the correlation analysis conclusions. The sources of heavy metal(loid)s pollution in sediments are complex, and further in-depth analysis of different sources is required.
Figure 5. Correlative heat map of sediments heavy metal(loid)s content (A) and cluster analysis (B).
After normalizing the heavy metal(loid)s data in the sediments, the Kaiser-Meyer-Olkin (KMO) and Bartlett’s sphericity tests were conducted to assess the suitability of the data for factor analysis. A KMO value above 0.7 generally indicates that the data are appropriate for factor analysis. In this study, the KMO value was 0.7922, and Bartlett’s test of sphericity yielded a chi-square value of 22,948.226 with 190 degrees of freedom and a significance level of 0.000, which is well below the threshold of 0.05. These results confirm strong correlations among the heavy metal(loid) elements, making the data suitable for factor analysis. To improve the interpretation of the principal components, the Kaiser normalization method with varimax rotation was applied to the data, and the rotated data were used for further analysis. The results of the principal component analysis (PCA) for heavy metal(loid)s in surface sediments are presented in Table 3. Four factors with eigenvalues greater than 1 were extracted, which collectively account for 81.35% of the total variance, capturing most of the information related to the sources of heavy metal(loid)s. Specifically, PC1, PC2, PC3, and PC4 explain 36.52%, 22.11%, 14.70%, and 8.03% of the variance, respectively.
PC1 is primarily characterized by high loadings of Be, Sr, Ca, Co, Hg, Pb, Fe, Cr, Ba, Mg, and Se. Wei and Algeo (2020) noted that Sr can substitute for Ca in various rocks and minerals, including gypsum, plagioclase, aragonite, calcite, dolomite, celestite (SrSO4), and strontianite (SrCO3), due to the similarity in their ionic radii (Roden et al., 2002). The significant positive correlation between Sr and Ca observed in this study is consistent with these findings. Sr is most commonly found in sedimentary carbonates, while Mg is a widespread element in carbonate materials, further supporting the observed positive correlation between Mg and Sr. These results suggest that Sr, Mg, and Ca in the sediment samples are likely associated with the natural variation of carbonate components. In this study, the mean Hg content was found to exceed both the background values for Chinese shallow sea sediments and those for heavy metal(loid)s in Hainan Island soils (Table 2). The risk assessment indicates that Hg poses a potential ecological risk, suggesting the presence of an external pollution source for Hg in the sediments. Previous studies have demonstrated that Hg is highly volatile and can be emitted into the atmosphere during industrial processes. It can undergo long-range atmospheric transport before being deposited into the soil through dry and wet deposition processes (Tian et al., 2012; Fu et al., 2023b). Therefore, it is inferred that PC1 represents a mixed source, combining both natural and anthropogenic origins.
PC2 is primarily characterized by high loadings of Fe, Zr, Ti, Ga, Ba, and Mn. In soil environments, Ti typically originates from terrestrial detritus. Zr, an inert element with high chemical stability, is commonly enriched in oxides and silicates. During weathering, fine Zr particles remain in the soil, forming sand deposits widely distributed in nature (Liu et al., 2012). Ga in sediments is mainly found in clay and aluminum-bearing minerals, with its migration and precipitation largely influenced by the geochemical properties of aluminum (Breiter et al., 2013). Therefore, PC2 is interpreted as representing a natural source.
PC3 is primarily characterized by high loadings of Mn, As, Cd, Mg, Mo, and Ni. The significant loadings of Mn in both PCA2 and PCA3 suggest differences in its sources. Research by (Kikuchi et al., 2009) indicates that manganese pollution in water bodies is mainly associated with untreated domestic and industrial wastewater. Meanwhile, the primary source of As is agricultural pollution (Jiao et al., 2012). The use of arsenic-rich pesticides, fertilizers, and herbicides in agricultural activities likely accounts for the presence of As in the sediments of the study area. Therefore, PC3 is interpreted as representing anthropogenic sources.
PC4 is primarily characterized by high loadings of As, Cu, and Se. The low correlation between As and Se (p>0.05) suggests that PC4 represents multiple sources. The majority of sampling points show Cu in a non-polluted state, indicating that Cu in the sediments primarily originates from natural sources. In addition to accumulation from agricultural activities, As in the soil environment is also associated with aquaculture and the combustion of diesel and gasoline by ships (Hu et al., 2013; Soroldoni et al., 2018; Cheng et al., 2018). Se mainly originates from mining, wastewater discharge, and coal combustion (George et al., 2020). Considering the presence of numerous ports in the study area, including Qingge Port, Boao Port, Millennium Fishing Port, and Tanmen Port, it is possible that ship activities, such as hull corrosion, paint peeling, and fuel combustion, contribute to As and Se pollution. Therefore, PC4 is identified as a mixed source of natural and anthropogenic origins.
The PMF model enables quantitative analysis of pollutant sources in soils and other environmental media, as well as the determination of the contribution rate of each source. In this study, experimental data were processed using the EPA PMF 5.0 software. Notably, the number of factors set in the model scenarios significantly impacts the source apportionment results. In this study, different scenarios with varying numbers of factors (2 to 5) were initially set, and the number of runs was set to 20 to minimize the Q value and control the scaled residuals. The model achieved the smallest Qrobust/Qture value (2.45) when the number of factors was set to 5, with most residual values concentrated between -3 and 3. Thus, the optimal number of factors for this study was determined to be 5. Additionally, the r2 values for most elements, except for Cr, Cu, Ni, Mo, and Cd, were greater than 0.6 (Table 4), indicating a high level of confidence in the PMF model’s source apportionment results and that the chosen number of factors adequately explains the information contained in the original data. The results of the source apportionment for heavy metal(loid)s in sediments based on the PMF model are shown in Figure 6. The contribution rates of the five factors are as follows: Factor 1 (15%), Factor 2 (19%), Factor 3 (13%), Factor 4 (27%), and Factor 5 (26%).
In Factor 1, the primary loading elements are As and Mn, with contribution rates of 90.6% and 47.3%, respectively. Correlation analysis shows a significant positive correlation between As and Mn (p < 0.001), indicating a shared source. As discussed earlier, the accumulation of As in sediments is primarily influenced by agricultural activities (Li et al., 2022b). The study area is surrounded by extensive farmland, where large quantities of pesticides and insecticides are used in agricultural production, serving as the main source of As in the sediments. Therefore, Factor 1 potentially identified as an agricultural source.
In Factor 2, the main loading elements are Sr, Ca, and Mg, with contribution rates of 83.0%, 81.3%, and 36.7%, respectively. Sr, Mg, and Ca are primarily found in carbonate minerals, with Sr accumulating in association with calcium-rich biological materials. The study area shows elevated Sr levels, significantly above Chinese shallow sea sediment background values, with moderate to high pollution levels at more than half of the sampling sites. This indicates that Sr enrichment is influenced by both biological factors and anthropogenic activitie. Castillo et al. (2024) found that microplastics can adsorb environmental pollutants, including metals. The widespread aquaculture activities in the area, involving the use of PE floatation devices and fishing gear, contribute to Sr accumulation through microplastic pollution. Thus, Factor 2 suggests source as an aquaculture-related anthropogenic source.
In Factor 3, the primary loading elements are Zr and Ti, with contribution rates of 90.6% and 42.5%, respectively. Zr is mainly found in oxides and silicates, while Ti, as a rock-forming element, aids in Zr accumulation in sediments. Therefore, Factor 3 suggests source as a natural source related to parent material weathering.
In Factor 4, the main loading elements are Mo, Se, Pb, Be, Co, Cu, Ga, Ni, Fe, and Cd, with contribution rates of 59.4%, 51.1%, 46.7%, 45.7%, 43.4%, 41.3%, 38.1%, 37.5%, 35.8%, and 33.9%, respectively. The mean concentrations of these elements in sediments are either below or close to the background values for Chinese shallow sea sediments, indicating that their levels are primarily controlled by natural geological backgrounds. This conclusion is supported by the results from the geo-accumulation index and the potential ecological risk index, which show that these heavy metal(loid)s generally fall into the non-polluted or low ecological risk categories. Therefore, Factor 4 potentially identified as a natural source.
In Factor 5, the primary loading elements are Hg, Ba, and Cr, with contribution rates of 80.5%, 65.0%, and 52.5%, respectively. Since the pre-industrial era, human inputs of mercury into the oceans have increased significantly, with 90% of riverine mercury buried in coastal sediments (Yin et al., 2015). Research indicates that over two-thirds of Hg in the natural environment originates from human activities, with coal combustion being a major source (Rashid et al., 2022). The results from the geo-accumulation index and potential ecological risk index also indicate that sediment Hg at some sampling sites falls within the moderate to high ecological risk categories. Reports show that coal combustion is a major source of Hg emissions, accounting for 33.4% of all energy consumption in Hainan Province (Tian et al., 2012). Ba, often used in cleaning agents for diesel engines and other internal combustion engines, likely originates from traffic pollution. Based on this analysis, Factor 5 potentially identified as a traffic-related source.
A comparison of the PCA and PMF models reveals several similarities and differences in source apportionment. 1) Number of Identified Sources: PCA identified 4 principal components, while the PMF model determined that the optimal number of factors is 5, showing inconsistency between the two methods. 2) Identification of Marker Elements: The primary loading elements associated with each source are largely consistent between PCA and PMF. However, the PMF model provides a more detailed breakdown of anthropogenic sources, whereas PCA does not further distinguish these sources into separate factors. 3) Source Contribution Rates: Compared to PCA, the PMF model has the advantage of assigning weights to each data point based on its uncertainty. Additionally, it applies non-negativity constraints on factor loadings and scores during the solution process, preventing the occurrence of negative values during matrix decomposition. This approach enhances the interpretability and feasibility of the source contribution rates derived from the PMF model (Fei et al., 2020; Anh, 2022). In conclusion, the PMF model proves to be more advantageous for source apportionment of regional soil heavy metal(loid)s in this study. The comparison of the results from the two methods also improves the reliability of the source apportionment conclusions.
This study analyzed 93 surface sediment samples from the nearshore area of Qionghai, using geo-accumulation index, potential ecological risk index, correlation analysis, principal component analysis (PCA), cluster analysis, and Positive Matrix Factorization (PMF) to assess heavy metal(loid) pollution risks and sources. The key findings are:
The average concentrations of elements, from highest to lowest, are: Ca > Fe > Mg > Ti > Sr > Zr > Ba > Cr > Pb > Cu > Ni > Ga > As > Co > Be > Mo > Se > Cd > Hg. The mean concentrations of Fe, Ca, Mg, Ti, Cu, Sr, Zr, Mo, Cd, Pb, Hg, As, and Se exceed background levels for Chinese shallow sea sediments. Elements like Ca, Mn, Ba, Ni, Sr, Be, Co, Mo, Hg, As, Ti, Cr, Zr, and Ga show high variability (CV > 51%), suggesting potentially influence from human activities.
Sr, Hg, and As are identified as the primary pollutants and key ecological risk factors based on geo-accumulation index, potential ecological risk index, and Nemerow index.
Correlation and source apportionment analyses show that As and Mn are mainly from agricultural sources, Sr, Ca, and Mg from aquaculture, and Zr, Ti, Mo, Se, Pb, Be, Co, Cu, Ga, Ni, Fe, and Cd from natural sources. Hg, Ba, and Cr are primarily linked to traffic sources. The strong correlation between PMF-predicted and observed heavy metal(loid) concentrations confirms the effectiveness of PMF for source apportionment. The consistency between PCA and PMF results further supports the reliability of these findings. This version removes redundancy and streamlines the content while maintaining clarity and precision, making it more suitable for a Frontiers conference paper.
To sum up, there are certain pollution risks in the study area, and the main pollutants are Sr, Hg, and As. The accumulation of major pollutants in the environment is mainly affected by agricultural activities, aquaculture and traffic emissions. Therefore, in the follow-up management, corresponding measures should be taken in these aspects to reduce pollutant emissions and ensure the protection and sustainability of the Marine environment.
The original contributions presented in the study are included in the article/supplementary material. Further inquiries can be directed to the corresponding authors.
JJ: Writing – original draft, Writing – review & editing. MF: Data curation, Investigation, Writing – original draft. JY: Supervision, Writing – review & editing. YS: Funding acquisition, Supervision, Writing – review & editing. GF: Writing – review & editing. HW: Writing – review & editing. CL: Writing – review & editing. YW: Writing – review & editing.
The author(s) declare financial support was received for the research, authorship, and/or publication of this article. We acknowledge the financial support from the Comprehensive Survey of Natural Resources in “Hai Cheng Wen” Coastal Zone (DD20230414), and Science and Technology Innovation Fund of Command Center of Integrated Natural Resources Survey Center (Grant No. KC20220009), and National Natural Science Foundation of China (Grant No. 52371295).
The authors declare that the research was conducted in the absence of any commercial or financial relationships that could be construed as a potential conflict of interest.
All claims expressed in this article are solely those of the authors and do not necessarily represent those of their affiliated organizations, or those of the publisher, the editors and the reviewers. Any product that may be evaluated in this article, or claim that may be made by its manufacturer, is not guaranteed or endorsed by the publisher.
Anh N. N. (2022). An insight into source apportionment of metals in superficial sediments from the Tien Hai nature reserve of the Red River delta, Vietnam. Mar. Pollut. Bull. 185, 114278. doi: 10.1016/j.marpolbul.2022.114278
Arikibe J. E., Prasad S. (2020). Determination and comparison of selected heavy metal concentrations in seawater and sediment samples in the coastal area of Suva, Fiji. Mar. Pollut. Bull. 157, 111157. doi: 10.1016/j.marpolbul.2020.111157
Breiter K., Gardenová N., Kanický V., Vaculovic T. (2013). Gallium and germanium geochemistry during magmatic fractionation and post-magmatic alteration in different types of granitoids: A case study from the Bohemian Massif (Czech Republic). Geol. Carpathica. 64, 237–251. doi: 10.2478/geoca-2013-0018
Castillo A. B., El-Azhary M., Sorino C., LeVay L. (2024). Potential ecological risk assessment of microplastics in coastal sediments: Their metal accumulation and interaction with sedimentary metal concentration. Sci. Total. Environ. 906, 167473. doi: 10.1016/j.scitotenv.2023.167473
Cheng Z., Chen L.-J., Li H.-H., Lin J.-Q., Yang Z.-B., Yang Y.-X., et al. (2018). Characteristics and health risk assessment of heavy metals exposure via household dust from urban area in Chengdu, China. Sci. Total. Environ. 619–620, 621–629. doi: 10.1016/j.scitotenv.2017.11.144
Fang Y., Chen Y., Tian C., Lin T., Hu L., Li J., et al. (2016). Application of PMF receptor model merging with PAHs signatures for source apportionment of black carbon in the continental shelf surface sediments of the B ohai and Y ellow S eas, C hina. JGR. Oceans. 121, 1346–1359. doi: 10.1002/2015JC011214
Fei X., Lou Z., Xiao R., Ren Z., Lv X. (2020). Contamination assessment and source apportionment of heavy metals in agricultural soil through the synthesis of PMF and GeogDetector models. Sci. Total. Environ. 747, 141293. doi: 10.1016/j.scitotenv.2020.141293
Fu K., An M., Song Y., Fu G., Ruan W., Wu D., et al. (2023a). Soil heavy metals in tropical coastal interface of eastern Hainan Island in China: Distribution, sources and ecological risks. Ecol. Indic. 154, 110659. doi: 10.1016/j.ecolind.2023.110659
Fu Z., He N., Ma M., Bao Z., Xie S., Gu Y. (2023b). Source apportionment and probabilistic risk assessment of heavy metals in selenium-rich soils in Hainan Province, China. J. Geochem. Explor. 251, 107241. doi: 10.1016/j.gexplo.2023.107241
Fu J., Tang X.-L., Zhang J., Balzer W. (2013). Estuarine modification of dissolved and particulate trace metals in major rivers of East-Hainan, China. Continental. Shelf. Res. 57, 59–72. doi: 10.1016/j.csr.2012.06.015
Gao X., Zhou F., Chen C.-T. A. (2014). Pollution status of the Bohai Sea: An overview of the environmental quality assessment related trace metals. Environ. Int. 62, 12–30. doi: 10.1016/j.envint.2013.09.019
George A., Shen B., Kang D., Yang J., Luo J. (2020). Emission control strategies of hazardous trace elements from coal-fired power plants in China. J. Environ. Sci. 93, 66–90. doi: 10.1016/j.jes.2020.02.025
Guo X., Gu J., Gao H., Qin Q., Chen Z., Shao L., et al. (2012). Effects of Cu on metabolisms and enzyme activities of microbial communities in the process of composting. Bioresour. Technol. 108, 140–148. doi: 10.1016/j.biortech.2011.12.087
Hu B., Cui R., Li J., Wei H., Zhao J., Bai F., et al. (2013). Occurrence and distribution of heavy metals in surface sediments of the Changhua River Estuary and adjacent shelf (Hainan Island). Mar. Pollut. Bull. 76, 400–405. doi: 10.1016/j.marpolbul.2013.08.020
Hu C., Liu Y., Fang X., Zhou Z., Yu Y., Sun Y., et al. (2024). Assessing heavy metal pollution in sediments from the northern margin of Chinese mangrove areas: Sources, ecological risks, and health impacts. Mar. Pollut. Bull. 200, 116069. doi: 10.1016/j.marpolbul.2024.116069
Ji Z., Zhang H., Zhang Y., Chen T., Long Z., Li M., et al. (2019). Distribution, ecological risk and source identification of heavy metals in sediments from the Baiyangdian Lake, Northern China. Chemosphere 237, 124425. doi: 10.1016/j.chemosphere.2019.124425
Jiang J., Fu G., Feng Y., Gu X., Jiang P., Shen C., et al. (2023). Characteristics and causes of coastal water chemistry in qionghai city, China. Appl. Sci. 13, 5579. doi: 10.3390/app13095579
Jiao W., Chen W., Chang A. C., Page A. L. (2012). Environmental risks of trace elements associated with long-term phosphate fertilizers applications: A review. Environ. Pollut. 168, 44–53. doi: 10.1016/j.envpol.2012.03.052
Kikuchi T., Furuichi T., Hai H. T., Tanaka S. (2009). Assessment of heavy metal pollution in river water of Hanoi, Vietnam using multivariate analyses. Bull. Environ. Contamination. Toxicol. 83, 575–582. doi: 10.1007/s00128-009-9815-4
Li X., Bing J., Zhang J., Guo L., Deng Z., Wang D., et al. (2022a). Ecological risk assessment and sources identification of heavy metals in surface sediments of a river–reservoir system. Sci. Total. Environ. 842, 156683. doi: 10.1016/j.scitotenv.2022.156683
Li Y., Li B., Yuan Y., Lei Q., Jiang Y., Liu Y., et al. (2022b). Trends in total nitrogen concentrations in the Three Rivers Headwater Region. Sci. Total. Environ. 852, 158462. doi: 10.1016/j.scitotenv.2022.158462
Liu S., Liu Y., Yang G., Qiao S., Li C., Zhu Z., et al. (2012). Distribution of major and trace elements in surface sediments of Hangzhou Bay in China. Acta Oceanol. Sin. 31, 89–100. doi: 10.1007/s13131-012-0223-y
Lu Q., Bai J., Zhang G., Wu J. (2020). Effects of coastal reclamation history on heavy metals in different types of wetland soils in the Pearl River Delta: Levels, sources and ecological risks. J. Cleaner. Production. 272, 122668. doi: 10.1016/j.jclepro.2020.122668
Miola B., de Morais J. O., de Souza Pinheiro L. (2016). Trace metal concentrations in tropical mangrove sediments, NE Brazil. Mar. pollut. Bull. 102, 206–209. doi: 10.1016/j.marpolbul.2015.11.039
Mirza R., Moeinaddini M., Pourebrahim S., Zahed M. A. (2019). Contamination, ecological risk and source identification of metals by multivariate analysis in surface sediments of the khouran Straits, the Persian Gulf. Mar. pollut. Bull. 145, 526–535. doi: 10.1016/j.marpolbul.2019.06.028
Murray R. W., Leinen M. (1993). Chemical transport to the seafloor of the equatorial Pacific Ocean across a latitudinal transect at 135°W: Tracking sedimentary major, trace, and rare earth element fluxes at the Equator and the Intertropical Convergence Zone. Geochim. Cosmochim. Acta 57, 4141–4163. doi: 10.1016/0016-7037(93)90312-K
Nan X. I. A., Guicheng X. U. E., Yangrong F. U., Yi Y., Changzhu L. I. U., Ronglin M. A. (2011). Analysis of ecological risk and the content situation of heavy metals in surface sediments of Hainan Island’s inshore. Resour. Environ. Eng. 25, 244. doi: 10.16536/j.cnki.issn.1671-1211.2011.03.024
Niu L., Li J., Luo X., Fu T., Chen O., Yang Q. (2021). Identification of heavy metal pollution in estuarine sediments under long-term reclamation: Ecological toxicity, sources and implications for estuary management. Environ. Pollut. 290, 118126. doi: 10.1016/j.envpol.2021.118126
Nriagu J. O. (1979). Global inventory of natural and anthropogenic emissions of trace metals to the atmosphere. Nature 279, 409–411. doi: 10.1038/279409a0
Ouyang X., Wang Z., Liu X. (2024). Distribution patterns, risk assessment and potential sources of heavy metals in sediment in the Qiongzhou Strait, China. Mar. Pollut. Bull. 203, 116481. doi: 10.1016/j.marpolbul.2024.116481
Paatero P., Tapper U. (1994). Positive matrix factorization: A non-negative factor model with optimal utilization of error estimates of data values. Environmetrics 5, 111–126. doi: 10.1002/env.3170050203
Phil-Eze P. (2010). Variability of soil properties related to vegetation cover in a tropical rainforest landscape. J. Geogr. Regional. Plann. 3, 177–184. doi: 10.5897/JGRP.9000159
Rashid S., Shah I. A., Supe Tulcan R. X., Rashid W., Sillanpaa M. (2022). Contamination, exposure, and health risk assessment of Hg in Pakistan: A review. Environ. Pollut. 301, 118995. doi: 10.1016/j.envpol.2022.118995
Rizzo M. J., Scheff P. A. (2004). Assessing ozone networks using positive matrix factorization. Environ. Prog. 23, 110–119. doi: 10.1002/ep.10018
Roden E. E., Leonardo M. R., Ferris F. G. (2002). Immobilization of strontium during iron biomineralization coupled to dissimilatory hydrous ferric oxide reduction. Geochim. Cosmochim. Acta 66, 2823–2839. doi: 10.1016/S0016-7037(02)00878-5
Sheng D., Meng X., Wen X., Wu J., Yu H., Wu M. (2022). Contamination characteristics, source identification, and source-specific health risks of heavy metal(loid)s in groundwater of an arid oasis region in Northwest China. Sci. Total. Environ. 841, 156733. doi: 10.1016/j.scitotenv.2022.156733
Soroldoni S., Castro Í. B., Abreu F., Duarte F. A., Choueri R. B., Möller O. O., et al. (2018). Antifouling paint particles: Sources, occurrence, composition and dynamics. Water Res. 137, 47–56. doi: 10.1016/j.watres.2018.02.064
Sun J., Zhao M., Huang J., Liu Y., Wu Y., Cai B., et al. (2022). Determination of priority control factors for the management of soil trace metal(loid)s based on source-oriented health risk assessment. J. Hazardous. Mater. 423, 127116. doi: 10.1016/j.jhazmat.2021.127116
Tian H., Cheng K., Wang Y., Zhao D., Lu L., Jia W., et al. (2012). Temporal and spatial variation characteristics of atmospheric emissions of Cd, Cr, and Pb from coal in China. Atmospheric. Environ. 50, 157–163. doi: 10.1016/j.atmosenv.2011.12.045
Tramonte K. M., Figueira R. C. L., Majer A. P., de Lima Ferreira P. A., Batista M. F., Ribeiro A. P., et al. (2018). Geochemical behavior, environmental availability, and reconstruction of historical trends of Cu, Pb, and Zn in sediment cores of the Cananéia-Iguape coastal system, Southeastern Brazil. Mar. Pollut. Bull. 127, 1–9. doi: 10.1016/j.marpolbul.2017.11.016
Wei W., Algeo T. J. (2020). Elemental proxies for paleosalinity analysis of ancient shales and mudrocks. Geochim. Cosmochim. Acta 287, 341–366. doi: 10.1016/j.gca.2019.06.034
Xiao H., Shahab A., Xi B., Chang Q., You S., Li J., et al. (2021). Heavy metal pollution, ecological risk, spatial distribution, and source identification in sediments of the Lijiang River, China. Environ. Pollut. 269, 116189. doi: 10.1016/j.envpol.2020.116189
Xu Z., Ni S., Tuo X., Zhang C. (2008). Calculation of heavy metals’ Toxicity coefficient in the evaluation of potential ecological risk index. Environ. Sci. Technol. 02, 112–115. doi: 10.19672/j.cnki.1003-6504.2008.02.030
Yin R., Feng X., Chen B., Zhang J., Wang W., Li X. (2015). Identifying the sources and processes of mercury in subtropical estuarine and ocean sediments using Hg isotopic composition. Environ. Sci. Technol. 49, 1347–1355. doi: 10.1021/es504070y
Zhang M., He P., Qiao G., Huang J., Yuan X., Li Q. (2019). Heavy metal contamination assessment of surface sediments of the Subei Shoal, China: Spatial distribution, source apportionment and ecological risk. Chemosphere 223, 211–222. doi: 10.1016/j.chemosphere.2019.02.058
Zhao K., Fu W., Qiu Q., Ye Z., Li Y., Tunney H., et al. (2019). Spatial patterns of potentially hazardous metals in paddy soils in a typical electrical waste dismantling area and their pollution characteristics. Geoderma 337, 453–462. doi: 10.1016/j.geoderma.2018.10.004
Zhao Y. Y., Yan M. C. (1993). Chemical element abundance in shallow sea sediments in China. SSPC-B1 23, 1084–1090. doi: 10.1360/zb1993-23-10-1084
Keywords: heavy metal(loid)s, sediments, pollution assessment, source apportionment, nearshore area
Citation: Jiang J, Fu M, Yang J, Song Y, Fu G, Wang H, Lin C and Wang Y (2024) Spatial distribution characteristics, ecological risk assessment, and source analysis of heavy metal(loid)s in surface sediments of the nearshore area of Qionghai. Front. Mar. Sci. 11:1491242. doi: 10.3389/fmars.2024.1491242
Received: 04 September 2024; Accepted: 24 October 2024;
Published: 15 November 2024.
Edited by:
Xinchen Gu, Tianjin University, ChinaReviewed by:
Lu Yan, China University of Geosciences Wuhan, ChinaCopyright © 2024 Jiang, Fu, Yang, Song, Fu, Wang, Lin and Wang. This is an open-access article distributed under the terms of the Creative Commons Attribution License (CC BY). The use, distribution or reproduction in other forums is permitted, provided the original author(s) and the copyright owner(s) are credited and that the original publication in this journal is cited, in accordance with accepted academic practice. No use, distribution or reproduction is permitted which does not comply with these terms.
*Correspondence: Jianying Yang, amN5eWFuZ2ppYW55aW5nQG1haWwuY2dzLmdvdi5jbg==; Yanwei Song, c29uZ3lhbndlaUBtYWlsLmNncy5nb3YuY24=
†These authors have contributed equally to this work and share first authorship
Disclaimer: All claims expressed in this article are solely those of the authors and do not necessarily represent those of their affiliated organizations, or those of the publisher, the editors and the reviewers. Any product that may be evaluated in this article or claim that may be made by its manufacturer is not guaranteed or endorsed by the publisher.
Research integrity at Frontiers
Learn more about the work of our research integrity team to safeguard the quality of each article we publish.