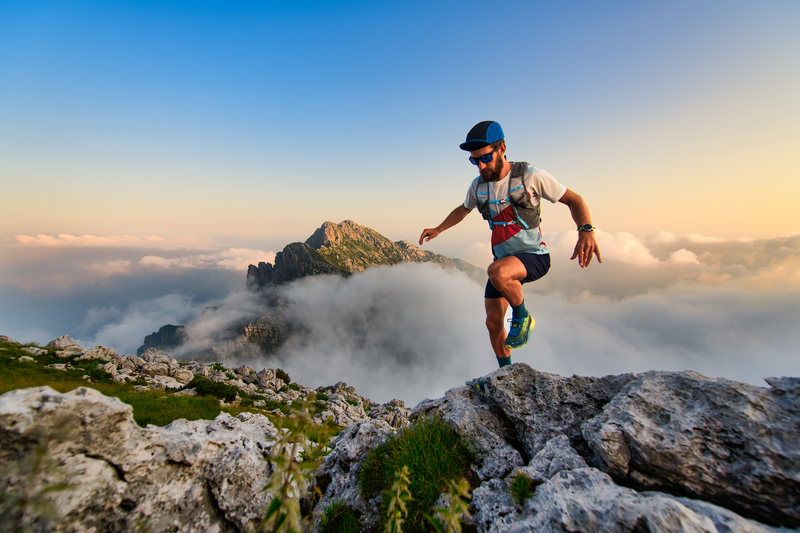
94% of researchers rate our articles as excellent or good
Learn more about the work of our research integrity team to safeguard the quality of each article we publish.
Find out more
METHODS article
Front. Mar. Sci. , 22 July 2021
Sec. Marine Conservation and Sustainability
Volume 8 - 2021 | https://doi.org/10.3389/fmars.2021.619898
This article is part of the Research Topic Open Citizen Science Data and Methods View all 28 articles
Within the United Nations Sustainable Development 2030 agenda, sustainable growth in the marine and maritime sector needs sea water quality monitoring. This is a very demanding and expensive task which results in the sea being largely undersampled. MaDCrow is a research and development project supported by the European Regional Development Fund, that involves citizens as data collectors while aiming to improve public environmental awareness and participation in scientific research. Its goal is to create an innovative technological infrastructure for real-time acquisition, integration and access of data, thus generating knowledge on sea water quality and marine ecosystem of the Gulf of Trieste. Data acquisition is based on an autonomous and removable device, developed within the project, that can be deployed on any small size sailing boat, recreational vessel, or fishing boat. The device holds low-cost sensors to measure pH, temperature, dissolved oxygen and salinity and the hardware and software to acquire, georeference and transmit the environmental data without interfering with the activities of the boats. In this work we analyze the use, capabilities and advantages of low-cost sensors but also their limitations, comparing, with a special focus on pH, their performances with those of the traditional ones. Applying the paradigm in a highly anthropized area such as the Gulf of Trieste, which is characterized also by a very high spatial and temporal variability of environments, we point out that this new approach allows to monitor sea water quality and highlight local anomalies with a resolution and spatial and temporal coverage that was not achievable with previous procedures, but yet at very low costs. Once received, data are then processed and submitted to a mediation flow that contextualizes and disseminates them for public use on a website. The final products have been customized to reach stakeholders such as tourists, fishermen and policy makers. The availability of information understandable to everyone, while fostering environmental awareness, stimulates, at the same time, involvement and participation of citizen scientists in the initiative. In the future, while committing to enlarge the number of participants, we will extend the analysis also toward other types of sensors.
Ocean waters cover over 70% of the Earth’s surface. They are responsible for more than 50% of the oxygen production on Earth and are fundamental in regulating the overall Earth climate. They have the capacity to absorb and, through the sea currents, distribute around the world, vast amounts of matter, carbon dioxide (CO2) and half of the heat reaching the Earth from the Sun. A large part of the global population lives along the coast. The anthropogenic pressure on coastal areas is heavily increasing so that understanding the effects that this can have on the sea is of paramount importance at multiple scales and for all of us (Halpern et al., 2012).
Within the Ocean Decade Framework1, ocean science needs to achieve key societal outcomes and a clean, safe, healthy and resilient, sustainably harvested, productive, predictable, and open data-transparent ocean. Understanding the ocean’s health is important in order to manage it while harvesting its resources and promoting its protection at the planetary level. The scientific community is deeply committed to the endeavor of monitoring the sea but experiences restrictions in studying the geographic and temporal coverage of phenomena, due to the limited funding of traditional methods based on expensive infrastructures, sensors, logistics and personnel (Lauro et al., 2014).
As often happens, issues can revert to be opportunities when a new approach is devised. This new paradigm can be built outsourcing activities outside the so far closed scope of the scientific community. The exchange of data, information and knowledge between researchers and laypeople can solidly ground the environmental awareness of the latter and improve their will to participate in the project (Nelms et al., 2017; Schleicher and Schmidt, 2020).
The mass media show a general commitment to highlight the problem of climate changes (Michael et al., 2016) but at the same time can give resonance to illusory and misleading views (Boykoff, 2013). This can be confusing and detrimental to the environmental awareness of the general public, which needs instead to be informed correctly in order to act consistently. Undoubtedly, the best way for the public to understand and appreciate science is to participate in it (Silvertown, 2009). Many scientific research projects took the citizen-science turn demonstrating very promising outcomes, as have been reported for example by McKinley et al. (2015); Schleicher and Schmidt (2020), and many other authors. These projects span a wide range of topics: from species observations to air quality measurements to asteroids and comets observation and identification.
If marine monitoring is very important to understand human impact on the environment, the availability of innovative methods that can improve the spatial and temporal coverage of natural phenomena over what available with traditional methods can be crucial toward the achievement of the United Nations Sustainable Development Goals (SDG) 2030 agenda2. Fraisl et al. (2020) conducted a very detailed mapping of all SDG goals, targets and indicators to understand where alternative methods such as crowdsourcing and citizen-science would be beneficial.
Among the firsts to introduce the citizen-science approach in the field of oceanography, Lauro et al. (2014) understood perfectly the opportunity offered by the availability of multiple private vessels, and in particular of sailing yachts or leisure boats that could enhance massively the coverage of an area. Underlying these efforts, important questions were posed such as: can meaningful data be collected with the kind of narrowly focused, low-cost instrumentation that is easily mass produced and deployable [on such types of platforms]? If so, what vessels will carry it, and what personnel will operate it?
Within the field of marine environment monitoring only few initiatives that follow this vision have taken place so far. Among those we can recall Chang et al. (2019), focused on weather data, the freshwaterwatch sampling initiative3, Bärlocher (2013) that describes the activities of the OpenSeaMap, a project devoted to the production of free-of-charge nautical maps that is mainly maintained by experienced volunteers. Another successful story is OSD, Ocean Sampling Day (Kopf et al., 2015) where worldwide scientists and citizens collected marine samples in a contemporaneous fashion, following the same standardized protocols for assessing marine microbial diversity. Luccio et al. (2020) propose a project where an Internet of Things (IoT) based system is tuned for the marine case [Internet of the Floating Things (IoFT)] building on top of a specific and already existent network of sensors that must be based on the industrial standards NMEA and Signal K. Other initiatives based on low cost solution are Spotter and Trident, from Sofar ocean, a commercial company, or the Smartfin initiative.
MaDCrow is a research and development project supported by the European Regional Development Fund - ERDF. Being the result of a regional implementation by the Italian region Friuli Venezia Giulia (North East of Italy), the project has a specific focus on the Gulf of Trieste (Northern Adriatic Sea-Mediterranean Sea), but it can be easily reconfigured and scaled to other possible areas.
A very important aspect of the project is that it aims to support open innovation between public institutions and private companies to stimulate economic activities related to the oceans and seas in the perspective of the Blue Economy. Private companies comprise, in fact, half of the partnership of the project, while the other half is constituted by research institutions, in a synergy between these two worlds that has been very beneficial to both of them.
The MaDCrow project aims at developing methods and technologies to improve geographic and time coverage of environmental marine monitoring involving volunteers that host, on their small vessels or leisure boats, a specific autonomous monitoring system developed within the project. This system is quasi-real time, platform agnostic, self-sufficient, interoperable and based on open technologies. The MaDCrow project aims at demonstrating that this paradigm can be a solution to the aforementioned problems while introducing at the same time the positive outcome of the improvement of the environmental awareness of volunteers and of the public at large. MaDCrow has to be intended as a proof of concept that, at the local levels, relevant stakeholders have joined forces to create a product that can use scientific data and inform citizens, fishermen and managers on the state of the sea. MaDCrow at the moment is not fully complying with the level of accuracy and precision and standardization protocols required by the SDG indicators, while in the future the project wishes to reach those goals thus being fully integrated in the SDG indicator 14.3.1 (Average marine acidity: pH). Furthermore, within the project portfolio, we envision future development to tackle explicitly the water-quality and climate risk-management related to harmful algal bloom and ocean acidification. Within this framework, this paper explores the opportunities and limitations of the proposed approach in comparison with the traditional methods, from the point of view of the performances of measurements and the improvement in resolution and spatial and time coverage of the designated area.
The MaDCrow infrastructure consists of several integrated modules that cover all the technological steps from environmental parameters acquisition to data and information access by the end users. As in Figure 1, modules can be gathered in three segments and namely: (I) acquisition and transmission (II) processing and mapping, (III) contextualization and end user access.
Modules have been developed independently by the partners of the project leveraging each partner’s previous specific experiences and backgrounds. Particular care has been taken to grant seamless integration between modules using robust open standards technologies, in order to allow easy refinements or possible additions of further new modules.
Modularization responds also to the needs of different communities of end users. Raw data, for example, can be used by scientists for data assimilation, model development and maintenance and to integrate them with the data they already have or acquire using traditional methods. On the other hand, it will be very difficult for the general public, or for non-technical communities, to understand the information and knowledge contained at this level. It is necessary, then, to address the needs of end users creating specific products that will reduce complexity through a process of representation (Callender and Cohen, 2006; Diviacco, 2014). This can be implemented by developing different co-existent modules that match the needs of each designated community.
As mentioned before the acquisition system was devised to be hosted on leisure boats or small vessels owned by volunteers/citizen scientists. Following Silvertown (2009) a very important factor to consider in designing acquisition systems to be used within projects where laypeople are involved is the usability of all the tools made available. We decided to keep the system as simple and automatic as possible, and in fact, not considering switching it on and off, essentially no intervention from volunteers is necessary, as the system acquires and transmits data autonomously and continuously.
A fundamental issue to consider planning the choice of sensors is cost. High end multiparametric probes can cost up to several tens of thousands of dollars; considering that the acquisition system has to be deployed on many platforms at the same time, the multiplicative effect that this can have on the budget can be frustrating. To address this problem MaDCrow focused on employing low-cost sensors only. In this perspective there was no need to develop yet another set of probes since the market offers already existing solutions.
An important choice to be made is whether to measure parameters in situ or taking samples to be measured on the ship. Byrne (2014) recommends the former while warns that any other practice can strongly affect the values taken. We therefore have chosen to deploy the instruments directly at sea using a frame that aims at maintaining constant, as much as possible, the boundary conditions of measurements. This choice unfortunately introduces other issues. Since the sensors are constantly immersed in seawater, a residue forms on the transducer so that eventually, the sensor may begin to report erratic measurements. In addition to this, bio-fouling further isolates sensors from the environment, adding progressive drift to measurements. Professional probes are equipped with self-cleaning devices that are too expensive and energy consuming to be used in our case. To address such problems, sensors need to be removed from the sea when not used and substituted when degraded in order to carefully clean and recalibrate them at the laboratory.
The specific sensors to be used within MaDCrow have been chosen upon the possibility to correspond to several characteristics, and namely: (i) to be low cost (it was estimated that each sensor should cost at most around a hundred dollars) (ii) to use the same data communication interface (iii) to be robust, lightweight, of small dimension and that can remain submerged in salt water indefinitely; (iv) to provide the information needed to produce values of the marine environment quality indicator defined by the Apparent Oxygen Utilization (AOU) (see section 2.1.10); (v) to provide easy calibration of sensors with dedicated calibration liquids.
At the beginning of the project (2018), after an extensive market research, we selected a set of sensors produced by Atlas Scientific because, at that time, those met all requirements set above. This of course should not be considered an endorsement to any specific model or company. In this work, in fact, it was our concern to always highlight the limitations of the tools adopted and of the paradigm in general (see the following section “The issue of precision, accuracy, and calibration of low-cost sensors”). Other models from other companies will for sure appear in the future, or possibly have even already appeared meanwhile, that will respond to the needs set above as well as those chosen in this work.
The use of low-cost sensors brings the issue of their precision and accuracy. In Table 1 we list the nominal values of resolution and accuracy of the sensors chosen for this work, as reported by the manufacturer.
Table 1. Nominal values of resolution and accuracy of the sensors chosen for this work, as reported by the manufacturer.
All sensors, except the temperature one, are shipped with a set of reference liquids that allow a quick but rather accurate calibration. Probes have to be immersed in the liquids and the reference value has to be stored in the board that manages the probe itself.
The pH probe has a flexible calibration protocol, which provides three successive calibration levels: single point, two points and three points. Three calibration solutions have been used, with pH 4 (low), 7 (mid), and 10 (high) respectively.
The dissolved oxygen (DO) was calibrated using two reference points, storing the value while the probe is in the air, and using a calibration solution (DO = 0).
Similarly also the conductivity probe was calibrated using a two points procedure, where the two calibration solutions used were respectively 1,413 and 12,880 μS.
The temperature probe was calibrated using boiling water, to correctly establish the value of 100°C.
The acquisition boards need a further setting for temperature or salinity compensation. More specifically, pH, DO, and EC sensor boards require temperature compensation (default is 20°C) and DO requires an additional salinity compensation (default is 0 μS for conductivity). This operation is performed periodically and automatically as new measures of temperature and conductivity become available.
A common opinion on low-cost sensors is that their accuracy and precision is low. This is certainly true under laboratory conditions, while at sea the situation can be less clear since other factors might further affect the measurements making low-cost sensors’ low performances less relevant. Among those factors we have to keep in mind that the paradigm we are exploring postulates the acquisition of data from mobile platforms. The side effects of this configuration adds to the limitations of low cost sensors affecting further their precision and accuracy. Within this work we were able to study in detail the case of pH probes that is particularly important for SDG indicator 14.3.1. While in future works we plan to study in detail the case of the other types of sensors, since very likely they have a similar behavior to pH sensors, in this study we assumed they behave the same.
The pH measures the degree of acidity or alkalinity of a solution with an adimensional number ranging from 0 to 14, while for sea water it is important to be able to discriminate phenomena within a range between 7.5 and 8.5 (Marion et al., 2011). Following Yang et al. (2014) the accuracy of different types of technologies, corresponding to different ranges of costs, can scale down from ±0.001 to ±0.1. Okazaki et al. (2017) maintained that at sea, where temperature, gas exchange, pressure, and biological processes simultaneously affect seawater, precision and accuracy is not easy to be controlled, so that in these conditions, the gap between low-cost and high-end probes is moved from measurement accuracy and precision more toward repeatability and stability.
A further layer of complication is introduced by the fact that sensors are bound to a moving acquisition platform. Since opportunistic crowdsourcing of voluntary leisure boats is at the very core of the acquisition method, the idea was to avoid interfering with the activities of volunteers, so that within the MaDCrow project no restriction on velocity during acquisition was imposed on volunteers. This can end up in measurements being affected by the motion of the platform hosting the sensors. Specific tests have been made to assess the range of velocities within which measurements can be considered acceptable. These tests took place in a delimited area where measurements done with high-end instrumentation reported reasonably constant value and consisted in a certain number of profiles carried out with a small boat that mounted the MaDCrow acquisition system. Within each profile, velocity was kept ‘ideally’ constant while it was changed from one test to the other. We say that the velocity was kept ‘ideally’ constant since controlling the platform motion can be challenging. Operating the engine can be rather arbitrary and other factors, such as currents, waves and ship motion can further make measurements unclear. As mentioned above, the MaDCrow system allows to associate each measurement with its GPS position and speed so that during the analysis it was possible to gather data upon GPS speed. Notwithstanding the fact that tests were performed under ideal weather conditions of no wind and flat sea, it has been very difficult, as a matter of fact, to discriminate velocities with a resolution below 1 km/h.
To understand the dependence of measurements with motion, we can refer to the normalized distribution of seawater pH measurements made with the low-cost sensor in relation to platform speed in Figure 2. Each curve corresponds to the distribution of measurements retrieved during a specific test held at constant velocity. Curves are normalized in order to allow comparison between tests with a different number of samples.
Figure 2. Normalized distribution of seawater pH measurements in relation to platform speed. Velocities from 0 to 13 km/h are plotted. For ease of reading some of them are colored in order to highlight the trend: blue for the 0 km/h curve, green (4 km/h), orange (10 km/h), red (11 km/h) and in dark red the curves corresponding to velocities above 11 km/h. The graph shows that lower velocities exhibit peaks at lower pH values, while increasing the speed, the distribution of pH measurements moves toward higher values.
For ease of reading, Figure 2 shows five colored cases that are representative of a specific behavior: blue (0 km/h), green (4 km/h), orange (10 km/h), red (11 km/h) and darker red for velocity values above 11 km/h. It is easy to see how within the range between 0 and 4 km/h, pH measurements peak approximately around a value of 7.7. Within the range between 4 and 10 km/h the peaks move toward a pH value of 7.8, while for values higher that 10 km/h the situation quickly changes, and the pH measurements show a trend that exceeds values of 8. At the same time also the dispersion of measurements shows a quite distinctive trend in the graph of Figure 2, and namely that higher velocities show reducing peaks and more dispersed measurements.
Following Urbini et al. (2020), the North Adriatic Sea, due, probably to river discharges, shows pH values that decrease from West (8.4) to East (8.15), with pH measurements decreasing from the sea surface to the bottom, while, in detail, in the area of the Gulf of Trieste, in winter, pH values are higher (8.2) while in summer they are lower (7.9). During the velocity tests made with low-cost sensors, we measured a reference value with a top-end pH probe system. This provided a value of 7.9. Figure 3 shows the average, the median and the standard deviation of all sensed raw pH measurements as a function of velocity. Low-cost sensors raw data acquired within the tests tend to have an almost constant drift of approximately –0.2 units compared to the reference values, up to the velocity of 10–11 km/h, when it starts to change steeply. This regular trend is even more clear considering the median value instead of the average of pH measurements. Following Okazaki et al. (2017) and considering the intrinsic limitations of low-cost sensors this behavior seems reasonable, while the degradation after 11 km/h could be related to different fluid flow status and could be important in order to plan the future development of new shapes for the sensor case. This behavior suggests that when the velocity of the platform remains below 10 km/h data acquired with low-cost sensors are consistent and could possibly be bulk corrected using professional high-end probes as reference measurements. Data acquired when velocity exceeds 10 km/h need corrections that are not easy to define so that within MaDCrow they are flagged out and do not enter the following steps of processing.
Figure 3. Average, median, and standard deviation of all sensed raw pH measurement as a function of velocity.
The control board for the whole MaDCrow acquisition system is based on an Arduino Pro Mini 5V/16MHz. A switching regulator provides stable power at 5V DC to every component, using a 12V lead-battery as main power source. The Arduino microcontroller communicates with the sensors, processes and redirects the data through RS232 up to the GPS and communication device (Figure 4). Every unit is endowed with the acquisition board, additional boards for the electric insulation (needed to avoid interferences among the probes), BNC waterproof cables and the actual sensors.
The boards require 5V DC powering and are able to communicate through I2C protocol with a control board. The control software performs a periodic reading with each sensor board, followed by a period of sleep mode, in order to reduce consumption.
With respect to factory presets, the sensor boards require a setup at the laboratory, before being ready to be used at sea: the I2C protocol must be activated (the default is RS232) and a calibration procedure must be performed, by means of reference samples and lab measures.
After the acquisition by the control board, data are sent every 10 s through a simple unidirectional serial protocol to the transmission device, connected by RS232. Data transmission is based on a dual SIM industrial modem unit with 2/3/4G GSM technology. The availability of a dual SIM unit is a key feature for seas or body waters that cross several countries, such as the North Adriatic Sea, where three countries (with several GSM providers) share a relatively small sea region.
The transmission system adopted by MaDCrow is based on a high-gain GSM antenna that guarantees an open GPRS socket in case of long distances. The internal firmware selects automatically the proper GSM provider over 2, 3, or 4G technology, so that the board unit can rely on different types of communication technologies. In addition, the specific modem device chosen offers multiple I/O connectivity (1Wire, RS 232 and SD card reader) for future further development.
The on-board unit is equipped also with a GPS and GLONASS satellite navigation device and sends data every 10 s or 10 m or 15° bearing movement, according to an adaptive path algorithm. This approach aims to gather sensor measurements independently from the vessel status, in order to maximize geo-referenced environmental data acquisition.
When the system insists in an area that is not covered by the mobile phone network, it can store up to 16,000 measurements that later can be sent onshore when the network is reached again.
The communication server is separated from the processing server and is accessed remotely, using secure authentication and by means of a set of customized Rest-API that transfer data in JSON format (Figure 5).
In order to estimate the average power consumption and the autonomy of the battery during the testing process, we separated two modules, and namely the sensor circuit on one side and the transmission system on the other.
The sensor circuit has two modes (Figure 6): active and sleep. In the active period (sensing and processing) we measured an average current of 76 mA. During the sleep period, we measured a value of approximately 60 mA. As active and sleep periods are approximately the same the average current was (76 mA + 60 mA/2) = 68 mA. The transmission, then, consumes alone 182 mA which makes the whole system consume in total 250 mA. When available, the acquisition system can be powered by the vessel’s electric supply, using for example the cigarette lighter or other means. When this is not available the system can be operated on batteries only. Using a standard 12V 9Ah motorcycle battery the energy consumption of the whole system is 3Wh, which guarantees 36 h uptime. In order to extend this period, we studied and tested different renewable energy generation methods such as solar energy, wind power and water turbines. Using renewable energy, we keep the system independent from the boat/sailboat power supply system while using sources that are inexhaustible, clean and sustainable.
Figure 6. Power consumption of the MaDCrow acquisition and transmission system in sleep and active mode.
We tested a 100 W solar panel connected to a solar charger controller and a 12V 9Ah battery. This configuration allowed us to fully charge the battery on a sunny day so that in these conditions the system can run indefinitely. Of course, in case of reduced exposure to solar radiation, as often happens during the winter season, this cannot be fully guaranteed. A more powerful set of solar panels and batteries should be used in this case, which, on the other hand, can create deployment issues and could be perceived by the volunteers as too invasive.
We also studied the possibility of using wind generators. Several commercial solutions tailored for leisure boats are available but with a higher cost compared to solar systems. In general wind generators are complementary to solar panels. In fact, in wintertime, they will keep on generating power even on cloudy days. They are operative also when the ship is docked/anchored, and they are also largely maintenance-free. On the downside, the relationship between wind speed and the energy these generators can produce is cubic, so power decreases exponentially if the wind regime is not sufficient.
We also analyzed the chance of improving power supply using water turbines and hydro generators designed for boats and sailboats. This technology is very attractive because it can charge the batteries during all the time the boat is sailing, even in wintertime, without wind or daylight. Unfortunately, these generators are rather expensive in comparison with solar panels and wind turbines, they need high speed for high power output, and in addition, of course, they cannot produce energy while the boat is docked.
Summing up when electric supply is available from the boat, this solution is preferable. When this is not the case, considering that since a large number of platforms has to be employed costs have to be reduced at minimum, the best solution is to use solar panels, or in case the single vessel can bear the costs complement these with wind generators or water turbines.
The sensor case (Figure 7) has been designed taking into account these fundamental requirements: waterproofness, impact resistance, flexibility of use, ease of installation and attractive design.
Figure 7. The MaDCrow acquisition device and case: the lower segment contains sensors and is immersed; the upper segment contains all the electronics.
To deal with these requirements, the developed case consists of two sections, an upper one, which contains the electronics for data transmission and batteries, and a lower one, which houses the sensors and enters directly into the water. Two straps, placed in the upper part, and two lateral steel eyelets, allow the fixing of the MaDCrow device case on a wide range of boats. Sensors are deployed at approximately 50 cm depth, which is a tradeoff resulting after testing several configurations where higher depths imposed too much tension on the assembly, while more shallower ones were too much influenced by the pitch and roll of the ship. The fixed and rather shallow depth of the sensor deployment of course implies further limitations on the possible information collected within the proposed paradigm.
Within the processing phase data are retrieved from the communication server and stored into a relational database (Figure 8). After a set of processing steps specifically developed within the project, products are made available through an interactive web interface and through machine-to-machine web services.
The server was implemented in Linux (Red Hat Enterprise), a Postgres + PostGIS relational database, an Apache/Tomcat web server, a GeoServer GIS, and some PHP + HTML + CSS + Javascript code. The so-called “LAPP” stack solution provides reliability, scalability and is totally open-source. The engine of the system is a collection of PHP scripts, scheduled with crontab, which harvest the data from the communication server, perform all the required data processing and store the products into the database. The PostGIS extensions of Postgres database allow to perform complex geographical queries, to deal with projection and reference systems and to store processed data into database geometrical features such as points, lines or polylines.
MaDCrow web-based data access relies on GeoServer software. This is a web GIS, based on Tomcat, capable of connecting to the Postgres + PostGIS database where crowdsourced data are stored, that allows to manage the geographical representation of data and maps and eventually to provide several web services. This allows an easy integration with web interfaces like OpenLayers or Cesium (for an interactive geographical view based on Javascript libraries) and with other automated systems, such as the Decision Support System (DSS) developed within the project.
The MaDCrow system makes use of standard web services defined by the OGC (Open Geospatial Consortium). WMS (Web Map Service) dynamically generates georeferenced maps, both vectorial (SVG) and raster (PNG, JPG, TIF, GeoTIFF). WFS (Web Feature Service) is defined as an XML protocol for geographical data exchange, based on GML (Geography Markup Language – ISO 19136). GeoJSon is an open-source format, based on a Javascript Open Notation (RFC 79146) and describing properties and geographical objects.
A pilot platform for visualization, with query and mapping capabilities, has been developed, based on open source Javascript library OpenLayers (Figure 9).
Figure 9. Coverage of the processed data and Geographic distribution of a single parameter (Conductivity) as can be seen for the pilot web portal of the MaDCrow Project. The portal allows to retrieve single measurements, aggregate gridded data and details for a single cell.
Participation of the general public, not only to increase the number of volunteers, but also to improve environmental awareness is at the very core of the project. We are convinced that, in this perspective, involvement must be based on a reward mechanism where the needs of many possible communities should be addressed by a production chain of knowledge focusing, at this stage of the project, on replying to easy to be understood sentences. After analyzing the outcomes of a web-based questionnaire submitted to the stakeholders, three use cases, have been identified: (1) “Let’s go to the beach!”, (2) “Vitality of the sea,” (3) “Be careful at sea!”. The first one gives information about the conditions of a specific area in terms of pleasantness of going to the beach and swim, the second one describes the status of the water in order to assess if fishes or mussels are under a stress, and the third one gives information about a potential oil spill in the sea. This, somehow, mirrors the above-mentioned identification of the main economic activities in the Gulf of Trieste (tourism, aquaculture, and marine transport).
Temperature, salinity, pH, DO data collected by MaDCrow are integrated with external sources and the Apparent Oxygen Utilization (AOU) indicator (Broecker and Peng, 1982; Ito et al., 2004) within a Decision Supporting System (DSS). The AOU is the difference between the measured DO concentration and its equilibrium saturation concentration in water with the same physical and chemical properties (as defined in the Biological and Chemical Oceanography Data Management Office4). This derived concept is influenced by physics and chemistry and by biology (see Supplementary Materials). The external sources used in the DSS are the ARPA environmental agency and other international bodies that provide forecast models based on real data such as the United States National Oceanic and Atmospheric Administration (NOAA).
The output of the DSS is a set of Key Performance Indicator (KPI) that can be used to deliver to stakeholders direct replies to the three questions mentioned above. For each scenario, the system provides an indication on the opportunity to make this choice in a given area, providing an indication with three values, green if positive, red if negative, yellow if the conditions are not totally negative but should be carefully considered (Figure 10).
Figure 10. DSS web portal. Here data acquired within the MaDCrow project is integrated with information from other sources. To make it understandable and respond to contextualization knowledge is represented using the metaphor of a semaphore.
Within the “Let’s go to the beach!” scenario the DSS integrates MaDCrow crowdsourced data together with other parameters such as chlorophyll concentration, Ostreopsis ovata (harmful microalga, that blooms in the summer months and produce a powerful toxin), coliforms abundances (harmful bacteria that are discharged by the wastewater systems), local weather conditions (air temperature and wind) and the AOU. In the “Vitality of the sea” scenario, MaDCrow temperature, salinity, oxygen and pH and AOU are used, together with chlorophyll concentration and Ostreopsis ovata abundances. For the “Be careful at sea!” scenario all MaDCrow temperature, salinity, oxygen, pH and AOU data are used.
To test the proposed approach, we deployed the developed system in a specific area of the Gulf of Trieste that can roughly be associated with the bay of Panzano, that corresponds to the coastal area between the river outlet of the Isonzo river and the Natural Marine Reserve of Miramare (Figure 11). The area is densely anthropized with the prominent urban area of the city of Monfalcone and several touristic marinas and resorts that discharge their sewage in the area. The resulting enrichment of water in nutrients (eutrophication) causes structural changes to the ecosystem such as: increased production of algae and aquatic plants, oxygen depletion (hypoxia), general deterioration of water quality, changes in ambient light environment and depletion of fish species (Alexander et al., 2017).
The bay of Panzano is home also of industrial activities such as very important and active shipyards, a papermill and a thermoelectric plant.
All these factors are a threat to the environment, but at the same time endanger also human activities such as fishery and mussel farming.
The shallow and semi-closed area takes to the extremes the peculiarities of the Northern Adriatic Sea where the dynamics of ecosystems are strongly dependent on air, land and sea interactions and prone to fall into worst case scenarios due to climate warming (Riedel et al., 2008). In detail, the study area is characterized by a very high spatial and temporal variability of environments due to the interaction between fresh-water river inputs and saltwater which is often responsible for the formation of strong salinity gradients (25–38 PSU) (Cozzi et al., 2012). River inflows are due mainly to the influence of the Isonzo river discharges. These can be estimated on average between approximately 90 and 120 m3/s (Comici and Bussani, 2007), with two typical flood periods: one in spring, due to snowmelt and one in autumn due to heavy rainfalls when it can exceed 2500 m3/s (Sirca et al., 1999; Covelli et al., 2004). Minor influxes are related to the Timavo river, which has a Karstic regime, and re-emerges from several springs with an average influx of approximately 30 m3/s (Gabrovšek and Peric, 2006). Currents in the Gulf of Trieste can be described through the layered gyre-type circulation pattern composed of a weak (2–3 cm/s) permanent cyclonic (counterclockwise) circulation below 10 m and a wind driven alternating cyclonic/anticyclonic flow in the surface (approx 5 m thick layer) proposed by Stravisi (1983). In the bay of Panzano this model becomes even more complex, dynamic and sensitive to climate change due to the very shallow sea and the proximity with the outlet of the Isonzo river. The whole Gulf of Trieste is often swept in winter by a very strong Bora wind (NNE) characterized by the formation of alternating jets and wakes associated with the coastal orography (Vozila et al., 2019).
Land borne nutrients follows the distribution of the haline fronts, showing complex horizontal and vertical gradients (Cozzi et al., 2012) sustaining productivity of the coastal zones and triggering frequent phytoplankton blooms and hypoxia, especially in late winter and autumn (Cozzi and Giani, 2011), which can result in negative impacts on tourism, fishery and mussel farms hosted in the area. Following Vidović et al. (2016) long term organic enrichment in this area is witnessed by the changes in the presence of foraminiferal species while Tomašovych et al. (2018) studied hypoxia periods through time that seemingly have not been induced only by human activities. Dynamics and extension of river plumes conditions also the formation of mucilaginous aggregations (Giani et al., 2005). In addition, the Isonzo river is of interest, also because it has been a source of mercury coming from cinnabar mining activity since the sixteenth century (Covelli et al., 2006) in the Slovenian hinterland. The shallow sea of the bay is characterized by sediments that range from pebble enriched sands in the nearshore areas to an increasing pelitic fractions in the foreset beds.
The complexity of the designated area results in a very dynamic environment which calls for high resolution and high spatial and temporal coverage. While this is very difficult and expensive to obtain with traditional methods, considering what said above, the proposed paradigm seems a very promising perspective.
Monitoring the sea is a very complex and wide research domain. Considering that within MaDCrow data acquisition has to take place within a crowdsourcing paradigm, it was necessary to narrow the range of the possible environmental parameters to acquire, taking great care, at the same time, to avoid hampering scalability and preserving the possibility to apply straightforwardly the system elsewhere. In this sense, the Gulf of Trieste and in detail the Bay of Panzano can be considered representative of many coastal areas where the economic activities, ad possible harms to the sea are centered on industries, tourism, aquaculture and maritime transport but where also there is a high spatial and temporal variability of the environments due to the interaction between river inputs and saltwater. These economic activities do not always coexist without problems so that the participative monitoring model MaDCrow proposes could be very effective also from the point of view of policy makers.
We recognized science and society should aim at a quantum leap strategy in monitoring the sea by measuring in quasi real-time the Environmental Essential Ocean Variables (EOVs, Miloslavich et al., 2018) in accordance with the GOOS (Global Ocean Observing System, Global Ocean Observing System – GOOS) measurements, and in this perspective MaDCrow has the potential to fill the gap of the ongoing monitoring efforts increasing local high resolution of primary variables and associated products both from a spatial and temporal point of view.
Considering all these factors, the choice of the environmental parameters to measure was on temperature, salinity, DO, and pH.
The four parameters can capture the most important physical and chemical properties of seawater. Chlorophyll and turbidity sensors were also considered since they offer information about microalgae and the presence of particles in the sea, but the implementation of sensors oriented toward biology have been delayed until an initial test phase with the first series of sensors have reached sufficient robustness. In this perspective, the choice of the environmental parameters to measure was a tradeoff between the availability and affordability of sensors, the technical integrability of hardware components and the actual scientific targets that were considered. In the detail of the study area (the Bay of Panzano), the scientific target was the assessment of the marine environment status to monitor algal blooms and hypoxia through the AOU indicator that is calculated from crowdsourced data. In this perspective the choice of sensors matched the scientific purpose.
During 3 days in February 2019 (13, 14, and 20) the designated area was surveyed using three voluntary boats mounting the MaDCrow acquisition device. Data collection for all platforms was simultaneous and lasted during the whole daytime. The three boats were very different; one of them was a Archambault A35 racing boat that acquired data mostly while sailing; another one was an outboard motor inflatable rubber boat, while the third vessel was a Carolina Skiff motorboat. They are generally docked in different marinas and run by different people that were enrolled specifically for the survey. As mentioned above, no obligation was imposed to the owners of the boats except to navigate within the designated area and, considering the limitations highlighted in section “The issue of precision, accuracy and calibration of low-cost sensors,” to avoid, if possible, velocities above 13 km/h. The installation of the acquisition device on the rubber boat and motorboat were simple and efficient while we realized that some attention must be paid in the case of the sailing boat, because ship rolling can, during certain sailing maneuvers, project the sensors outside the sea. The coverage of the area was a concern but eventually exceeded expectations. This probably could be due to the different areas of provenance of the boats that forced them to enter the designated area from different directions. We realized that there is a slight prevalence of coverage on the North East part of the designated area. This is easy to explain since that is the more touristic portion of the bay, which demonstrates that some motivational strategies, from gamification to economic rewarding should be considered to extend coverage in less pleasant areas.
The first step of data processing is data validation. Values that fall outside an acceptable interval must be dropped (for instance a water temperature of 50°C). In order to mitigate the effect of random spikes, the time series undergo a smoothing process. In this we tested several methods and the moving median proved to be the most robust and effective method, as displayed in Figure 12, where the blue line is the original time series, and the red line represents the filtered values.
After the validation, the measurements are a set of different scalar values for each measured parameter, marked with a timestamp (in UTC), latitude and longitude (based on GPS standard, WGS84). The database is populated by measurements acquired by all the available vessels and the objective of this processing step is to statistically aggregate these data (Figure 13).
Figure 13. Production workflow of the MaDCrow project. From level 0 to level 3 raw data is transformed into statistically validated environmental quality indicators such as the Apparent Oxygen Utilization (AOU).
We have chosen a geographical reference system tuned for the North-East Adriatic Sea, based on the Universal Transverse Mercator projection UTM 33N (EPSG:32633) with a regular square grid with side length of 200 m. Considering time intervals of one UTC hour (e.g., from 13:00:00 to 14:00:00), we may define our datacube as the subset of measured values within one square cell and within one specific hour. All values within a datacube are aggregated in order to generate processed data products on a cell-by-cell basis and namely: the mean and median value, the number of samples, the standard deviation and the estimated percent error. These aggregated data (primary products) are then stored into the database and made available through the web interfaces.
Further processing of primary products generates “secondary products” such as salinity (in pss, a function of temperature, pressure and electric conductivity) and the AOU environmental quality indicator.
A very important factor to consider in order to understand the importance of the use of crowdsourced data is how they relate to the available data acquired with traditional methods, how the former and the latter can address the scientific questions that rise in the specific environment and how they match the needs of the end users. The relation between traditional and crowdsourced data can change depending on the different environment. In the case of wide oceanic areas with slowly changing parameters, for example, the resolution and coverage needed will be much lower than that required in dynamic coastal and shallow water environments. While there is no limitation in the use of low-cost sensors in oceanic environments, the advantages of the low-cost approach in terms of logistics, costs and increase in resolution and coverage become less evident. Tuning the integration of the two approaches on the specific environment under study, potentially can result in great improvements from proceeding separately.
We will here consider the case of the study area starting from what data is currently available, what is missing and how the crowdsourcing approach can not only fill gaps but also increase dramatically spatial and temporal coverage and resolution.
Currently, environmental data, within the bay of Panzano, can be obtained essentially from the Territorial Environmental Protection Agencies (ARPA-FVG) and the Copernicus data services. Other possible sources of observations are the oceanographic buoys located in the Gulf of Trieste that are rather distant from the study area and the Emodnet services which cover the Gulf with a single cell and therefore cannot have the needed resolution to account for the high variability of the environment of the bay.
The FVG regional environmental agency of ARPA-FVG5 monthly monitors 28 marine historical stations, 13 stations that have been defined according to the MSFD and 19 transitional water stations. Three are the sampling depths (surface, intermediate and deep) where temperature, salinity, pH, oxygen saturation, chlorophyll, PAR (photosynthetically active radiations) are measured. In situ values are spatially interpolated between sampling stations, while monthly acquisitions leave large gaps between observations.
The Copernicus Marine Service, Ocean Products service (Escudier et al., 2020) offers daily averaged data products generated by a numerical system composed of an hydrodynamic model, supplied by the Nucleus for European Modelling of the Ocean (NEMO) and a variational data assimilation scheme (OceanVAR) for temperature and salinity vertical profiles and satellite Sea Level Anomaly along track data. The resolution of the data is approximately 4 km by 4 km.
Comparison of the resolution and coverage obtainable from crowdsourced data and traditional methods available in the study area can be seen in Figure 14.
Figure 14. Spatial distribution of the four environmental parameters (Temperature, Salinity, pH, Dissolved Oxygen) considered in the MaDCrow project from three data sources (i) the crowdsourced dataset with a resolution of 200 m by 200 m grid (ii) the Copernicus model with a resolution of 4 km by 4k m grid (iii) the positions of the ARPA stations whose measurements are taken monthly and have to be inter/extrapolated geographically and in time. (A,B) Shows the three datasets superimposed highlighting the improvement of the resolution and coverage of the crowdsourced data. (C,D) Shows only the crowdsourced data since no data is available from the other sources.
Figure 14A reports on the distribution of temperature collected during the survey mentioned in section “Data collection.” It shows superimposed the available datasets, and namely (i) the high resolution (200 m × 200 m) measurements obtained using 3 crowdsourcing boats; (ii) the Copernicus model (Mediterranean Sea Physics Reanalysis) with a lower resolution 4 km × 4 km grid; (iii) the network of ARPA observation stations (the black triangles in Figure 14) that in the study area comprises only point measurements acquired monthly, that need to be later inter/extrapolates in the area. In addition, unfortunately no data is available from this source in the designated period of the test survey.
During the 3 days, the routes of the three MaDCrow boats covered almost randomly the area of interest since no constraint was imposed on their activities. Notwithstanding this, they reconstructed a very detailed, although of course not complete, distribution of measurements in the area. A larger number of volunteers could have covered almost all the area, but it is difficult to estimate the ideal number of boats needed because, as mentioned above, if no constraint is imposed on volunteers, they tend to avoid unpleasant areas. With a more systematic approach, instead, we estimate that already with less than five boats it could be possible to cover in great detail the whole area.
From the comparison of the different datasets in Figure 14A it is possible to see the large improvement in resolution and coverage provided by the crowdsourced dataset, which allows to highlight the influences of the varying regime and extension of freshwater plumes and how they relate with saltwater masses, of the depth of the seafloor, and the effects due to the presence of the small marinas. The values from Copernicus and crowdsourcing are rather similar in the open sea areas with differences approximately below the unit Celsius degree while they diverge significantly in the highly dynamic areas.
Figure 14B shows the distribution of salinity using Copernicus and crowdsourced data. There is a very good correlation between the two datasets in open sea areas while the crowdsourced dataset is very successful in highlighting the local variations due to river inflows or marinas. This is very important because as mentioned before land borne nutrients follow the distribution of the haline fronts.
Referring to Figures 14C,D, unfortunately we have to highlight that Copernicus service does not provide data for pH and DO, so we can only map the positions of ARPA station, that unfortunately has no coverage in the time period of the survey.
MaDCrow ideally has the potential to increase the temporal and spatial resolution of the data available with traditional methods in a study area considering the scenario of a fleet of citizen scientists sailing the area for fishing and bathing activities or just enjoying the breeze. We recognize that as humans we tend to be very active during the daylight and the holidays. Nevertheless, data will be generated at unprecedented spatial and temporal resolution and once integrated within larger databases (e.g., Emodnet and Copernicus) will deepen our knowledge and understanding of temperature, salinity, DO and pH dynamics in this area.
The MaDCrow project has been devised to develop mainly the technologies that could support crowdsourcing/citizen science initiatives in marine environmental data acquisition and access. Following EU codes for Technology Readiness Level (TRL) MaDCrow was planned and funded to produce a result that corresponds to level 6 – Technology demonstrated in a relevant environment. We were able to go well beyond this goal and are currently working on the preparation of a full-scale crowdsourcing/citizen science initiative that will be entirely based on the outcomes of MaDCrow.
During the development of the project we have been able to identify several issues at different levels and namely: scientific, technical, and participative. We have been able to tackle most of them, while several are still under scrutiny.
From a scientific point of view, we were able to address the main goal of the MaDCrow project, that was to demonstrate that the acquisition of data within a crowdsourcing paradigm allows effectively to cover large areas and ranges of time with small investments. Besides this we have been able also to address other non-trivial questions such as ‘what is the real value of crowdsourced data?,’ ‘How does the lack of accuracy and precision of low cost sensors affect results?’ and ‘How can we address this issue?’; questions that are in line with those posed by Lauro et al. (2014) and that have been mentioned in section “The infrastructure” of this work, regarding which, it is possible to say, yes; low cost instrumentation can produce meaningful data and can be easily deployed on a large range of types of boats where almost anyone can operate them. We have seen that the comparison between low-cost sensors and high-end probes cannot be straightforwardly extended from the laboratory to the sea. In the latter case, in fact, stability and replicability of the measurements can be themselves an issue, so that intrinsic precision and accuracy starts to be less relevant. As a matter of fact, considering the deployment device we have developed and the specific sensors we have used, we carefully analyzed the behavior of the MaDCrow system in comparison with the traditional methods. If the former is mounted on a marine platform moving at velocities below 12 km/h (which is often the case for leisure or small boats) we demonstrated that the sensor drift is almost constant and therefore crowdsourced measurements can be corrected using reference values acquired with traditional methods. In this perspective it is possible to extend the quality of local measurements obtained with high end instrumentation to the areas covered by means of crowdsourcing methods only. At the same, time, since the spatial distribution of crowdsourced data preserves its high spatial resolution and even most importantly, values does not result from an intra/extrapolation of sparse points but from an actual measurement, crowdsourced data are able to highlight features that would be overlooked in the case of undersampled data acquired with traditional methods.
On a technical level we have demonstrated that the entire workflow from data acquisition to data access through web-based portal and services is feasible and can be developed using open-source hardware, programming languages and software solutions only. At this level an issue that could be relevant is the power consumption of the acquisition and transmission device. When an external power supply line is not available, we demonstrated that an easy solution can be achieved using 100 W solar panels coupled to 12 V batteries. This set up of course requires space onboard that is not always easy to find and is, therefore, a solution that needs to be agreed upon with the boat owners. Alternative solutions have been explored that having some advantages over solar power, impose higher costs; a perspective which is against the philosophy of the project.
Although MaDCrow was mainly aiming at developing solutions at technical and scientific levels we studied carefully also the participative aspects of the initiative. This will be extensively reported in a related publication (Diviacco et al., 2021), while we would like here to highlight what is the rationale behind our choices. The importance of considering openly the goals of participants and stakeholders in these types of projects has been pinpointed by several authors such as for example Bruyere and Rappe (2007) and Ellwood et al. (2017) with a specific focus on public awareness of environmental issues, where people contribute both because the environment has an intrinsic value and because they want to learn and gain knowledge.
If learning and knowledge is therefore central in the perspective of volunteering to initiatives such as MaDCrow and ultimately in environmental awareness (the other main goal of the project) it is of paramount importance to be able to reach the public with messages that can be easily understandable. Raw data (complex products in Figure 2) are useful for scientists but cannot be used directly to address laypeople. They need to be translated into easy to be read products (Figure 2). To convey these messages, we identified some questions that can be linked to the main economic activities and interests of the area of the Gulf of Trieste (tourism, aquaculture, and maritime transportation) and built a DSS that integrates Crowdsourced data, information from external sources and expert’s knowledge in order that each community of end users will then find easy to understand answers for their needs. We estimate that the availability of these end user-oriented products besides nurturing environmental awareness in the general public will also stimulate participation in the project through identification with the processes of knowledge creation. We think this is of paramount importance since a very unfortunate phenomenon that can be easily identified in contemporary society is the detachment between science and people, which can give rise to unmotivated and deplorable habits, such as propagating news and views that are not based upon scientific research.
The MaDCrow project has been able to reply to many questions and to address many issues. Some results are still to be fine-tuned while others are still open.
The main weakness MaDCrow has to address as soon as possible is to extend the fleet of participating volunteer vessels. As mentioned above, the project aimed mainly at developing the technologies behind the infrastructure, leaving the actual implementation to a specific second round. As a matter of fact, only a restricted fleet of three testing vessels took part in the acquisitions done so far, while reaching a critical mass of platforms is important for several reasons.
From a scientific perspective, of course, the availability of a larger fleet will allow to cover larger areas and improve statistics.
From a development point of view, we would have the possibility to better test the scalability of the infrastructure in terms of robustness and needs of resources, to test the processing module with larger datasets, to further test the behavior of sensors in different conditions and to improve the design of the acquisition device frame. Regarding this latter topic, while so far, the frame we used to deploy the acquisition system at sea revealed to be efficient on several classes of vessels we highlighted that on motorboats its design should be revisited. As already mentioned, we’d be very interested in studying a different shape and other means to physically attach it to the hull of the boat in order to reduce turbulence and possibly improve the range of velocities within which data can be considered acceptable.
From a participative perspective, we think that reaching a critical mass of volunteers could be very important. Following Maund et al. (2020), in fact, individuals are more motivated to engage into large-scale citizen science projects, while Sutherland et al. (2015) maintains that if initiatives fail to engage or retain enough contributors, they are unlikely to achieve good results.
We are currently planning to implement a full scale MaDCrow initiative, and to address the extension of the fleet of voluntary platforms following multiple paths. The first one will be to use the current restricted fleet and the data that has been acquired to demonstrate the possibilities of the initiative to the larger public. In this sense we already started to promote MaDCrow in the broadcast media, at local, regional and national level. We were also successful in gaining visibility on national newspapers and planned a dense outreach program aiming at science fairs, schools, public talks, lectures and discussions. Unfortunately, the Covid 19 outbreak heavily reduced the possibility to follow this specific direction, which demonstrated to be very efficient.
Another path we are following, is to enroll volunteers within a commercial perspective. This is in line with the expectations of the ERDF framework to develop business, competences and jobs in the perspective of the blue economy. The idea behind this path is essentially an extension of the mechanism to reach end users through simplified and tailored data products, and consists in identifying specific classes of users, tune the infrastructure to solve their specific needs, while involving them in the acquisition of raw data that will become an asset of the project.
The data collected and their subsequent reprocessing represent the heart of the project, but the success of the MaDCrow model will depend on the way in which information that is truly relevant to end users will be identified and then disseminated, exploiting a disruptive network effect.
All data acquired and used within the MaDCrow project can be freely accessed through the web based portal http://madcrow.inogs.it/madcrow and through the OGC compliant WMS/WFS web services it offers at the following URLs: http://madcrow.inogs.it/geoserver/wms and http://madcrow.inogs.it/geoserver/madcrow/ows.
PD led, organized, and wrote most of the manuscript. AN and LG were responsible for data transmission. MI was responsible and wrote the parts related to data processing and web mapping. RC was responsible and wrote the part related to power supply and together with AP developed and wrote on hardware development. MB gathered and mapped all data available in the study area. AB was responsible for IT system management. MN was responsible and wrote together with PD the part regarding the DSS development. AM developed and wrote the business oriented part. FM was responsible and wrote together with PD the parts related to the biological and oceanographic phenomena and processes. All the authors contributed to the article and approved the submitted version.
The MaDCrow project has been funded by POR ERDF/FESR 2014-2020, DGR FVG n. 849, 13/5/2015.
AN and LG was employed by the company Transpobank S.r.l.
The remaining authors declare that the research was conducted in the absence of any commercial or financial relationships that could be construed as a potential conflict of interest.
The authors would like to acknowledge MareFVG and in particular Raphaela Gutty and Elizabeth Gergolet for their help and the manuscript reviewers for their very useful comments and suggestions.
The Supplementary Material for this article can be found online at: https://www.frontiersin.org/articles/10.3389/fmars.2021.619898/full#supplementary-material
Alexander T. J., Vonlanthen, P., and Seehausen, O. (2017). Does eutrophication-drivenevolution change aquatic ecosystems? Phil. Trans. R. Soc. B 372:20160041. doi: 10.1098/rstb.2016.0041
Boykoff, M. (2013). Public Enemy No. 1? Understanding media representations of outlier views on climate change. Am. Behav. Sci. 57, 796–817. doi: 10.1177/0002764213476846
Broecker, W. S., and Peng, T. H. (1982). Tracers in the Sea. Palisades, N. Y: Lamont-Doherty Earth Observatory.
Bruyere, B., and Rappe, S. (2007). Identifying the motivations of environmental volunteers. J. Environ. Plan. Manag. 50, 503–516. doi: 10.1080/09640560701402034
Byrne, R. H. (2014). Measuring Ocean acidification: new technology for a new era of ocean chemistry. Environ. Sci. Technol. 48, 5352–5360. doi: 10.1021/es405819p
Callender, C., and Cohen, J. (2006). There is no special problem about scientific representation. Theoria 21, 67–85.
Chang, S. J., Huang, C. H., and Chang, S. M. (2019). AIS-assisted service provision and crowdsourcing of marine meteorological information. Trans. Nav. 13, 63–67. doi: 10.12716/1001.13.01.05
Comici, C., and Bussani, A. (2007). Analysis of the River Isonzo discharge (1998-2005). Boll. Geofis. Teor. Appl. 48, 435–454.
Covelli, S., Piani, R., Faganeli, J., and Brambati, A. (2004). Circulation and suspended matter distribution in a microtidal deltaic system: the Isonzo river mouth (northern Adriatic Sea). J. of Coastal Res. SI 41, 130–140.
Covelli, S., Piani, R., Kotnik, J., Horvat, M., Faganeli, J., and Brambati, A. (2006). Behaviour of Hg species in a microtidal deltaic system: the Isonzo River mouth (northern Adriatic Sea). Sci. Total Environ. 368, 210–223. doi: 10.1016/j.scitotenv.2005.09.089
Cozzi, S., Falconi, C., Comici, C., Čermelj, B., Kovac, N., Turk, V., et al. (2012). Recent evolution of river discharges in the Gulf of Trieste and their potential response to climate changes and anthropogenic pressure. Estuar. Coast. Shelf Sci. 115, 14–24. doi: 10.1016/j.ecss.2012.03.005
Cozzi, S., and Giani, M. (2011). River water and nutrient discharges in the Northern Adriatic Sea: current importance and long term changes. Cont. Shelf Res. 31, 1881–1893. doi: 10.1016/j.csr.2011.08.010
Diviacco, P. (2014). “Reconciling knowledge and collaborative e-research,” in Collaborative Knowledge in Scientific Research Networks, eds P. Diviacco, P. Fox, C. Pshenichny, and A. Leadbetter (Pennsylvania: IGI Global), 1–20. doi: 10.4018/978-1-4666-6567-5.ch001
Diviacco, P., Nadali, A., Nolich, M., Molinaro, A., Iurcev, M., Carbajales, R., et al. (2021). Citizen Science and Crowdsourcing in the Field of Marine Scientific Research - the MaDCrow Project. Sgonico: National Institute of Oceanography and Applied Geophysics.
Ellwood, E. R., Crimmins, T. M., and Miller-Rushing, A. J. (2017). Citizen science and conservation: recommendations for a rapidly moving field. Biol. Conserv. 208, 1–4. doi: 10.1016/j.biocon.2016.10.014
Escudier, R., Clementi, E., Omar, M., Cipollone, A., Pistoia, J., Aydogdu, A., et al. (2020). Mediterranean Sea Physical Reanalysis (CMEMS MED-Currents) (Version 1) [Data set]. Copernicus Monitoring Environment Marine Service (CMEMS). doi: 10.25423/CMCC/MEDSEA_MULTIYEAR_PHY_006_004_E3R1.
Fraisl, D., Campbell, J., See, L., Wehn, U., Wardlaw, J., Gold, M., et al. (2020). Mapping citizen science contributions to the UN sustainable development goals. Sustain. Sci. 15, 1735–1751. doi: 10.1007/s11625-020-00833-7
Gabrovšek, F., and Peric, B. (2006). Monitoring the flood pulses in the epiphreatic zone of karst aquifers: the case of Reka river system, Karst plateau, SW Slovenia. Acta Carsol. 35, 35–45. doi: 10.3986/ac.v35i1.241
Giani, M., Rinaldi, A., Degobbis, D., and Vollenweider, R. (2005). Mucilages in the adriatic and tyrrhenian seas. Sci. Total Environ. 353, 1–2. doi: 10.1016/j.scitotenv.2005.09.007
Halpern, B. S., Longo, C., Hardy, D., McLeod, K. L., Samhouri, J. F., Katona, S. K., et al. (2012). An index to assess the health and benefits of the global ocean. Nature 488:615.
Ito, T., Follows, M. J., and Boyle, E. A. (2004). Is AOU a good measure of respiration in the oceans? Geophys. Res. Lett. 31, 1–4. doi: 10.1029/2004GL020900
Kopf, A., Bicak, M., Kottmann, R., Schnetzer, J., Kostadinov, I., Lehmann, K., et al. (2015). The ocean sampling day consortium. GigaSci 4:27. doi: 10.1186/s13742-015-0066-5
Lauro, F. M., Senstius, S. J., Cullen, J., Neches, R., Jensen, R. M., Brown, M. V., et al. (2014). The common oceanographer: crowdsourcing the collection of oceanographic data. PLoS Biol. 12:e1001947. doi: 10.1371/journal.pbio.1001947
Luccio, D. D., Riccio, A., Galletti, A., Laccetti, G., Lapegna, M., Marcellino, L., et al. (2020). Coastal marine data crowdsourcing using the internet of floating things: improving the results of a water quality model. IEEE Access 8, 101209–101223. doi: 10.1109/ACCESS.2020.2996778
Marion, G. M., Millero, F. J., Camões, M. F., Spitzer, P., Feistel, R., and Chen, C.-T. A. (2011). pH of seawater. Mar. Chem. 126, 89–96. doi: 10.1016/j.marchem.2011.04.002
Maund, P. R., Irvine, K. N., Lawson, B., Steadman, J., Risely, K., Cunningham, A. A., et al. (2020). What motivates the masses: understanding why people contribute to conservation citizen science projects. Biol. Conserv. 246:108587. doi: 10.1016/j.biocon.2020.108587
McKinley, D. C., Miller-Rushing, A. J., Ballard, H. L., Bonney, R., Brown, H., Evans, D. M., et al. (2015). Investing in citizen science can improve natural resource management and environmental protection. Issues Ecol. 2015, 1–27.
Michael, K. G., Littler, J., Brockington, D., and Boykoff, M. (2016). Spectacular environmentalisms: media, knowledge and the framing of ecological politics. Environ. Commun. 10, 677–688. doi: 10.1080/17524032.2016.1219489
Miloslavich, P., Bax, N. J., Simmons, S. E., Klein, E., Appeltans, W., Aburto-Oropeza, O., et al. (2018). Essential ocean variables for global sustained observations of biodiversity and ecosystem changes. Glob. Change Biol. 24, 2416–2433. doi: 10.1111/gcb.14108
Nelms, S. E., Coombes, C., Foster, L. C., Galloway, T. S., Godley, B. J., Lindeque, P. K., et al. (2017). Marine anthropogenic litter on British beaches: a 10-year nationwide assessment using citizen science data. Sci. Total Environ. 579, 1399–1409. doi: 10.1016/j.scitotenv.2016.11.137
Okazaki, R., Sutton, A., Feely Andrew, R., Dickson, G., Simone, R., Alin, L., et al. (2017). Evaluation of marine pH sensors under controlled and natural conditions for the Wendy Schmidt Ocean Health XPRIZE. Limnol. Oceanogr. Methods 15:2017. doi: 10.1002/lom3.10189
Riedel, B., and Zuschin, M. and Stachowitsch M. (2008). “Dead zones: a future worst-case scenario for Northern Adriatic biodiversity,” in Climate Warming and Related Changes in Mediterranean Marine Biota, CIESM Workshop Monographs 35, ed F. Briand, (Paris: CIESM Publisher), 152.
Schleicher, K., and Schmidt, C. (2020). Citizen science in germany as research and sustainability education: analysis of the main forms and foci and its relation to the sustainable development goals. Sustainability 12:6044. doi: 10.3390/su12156044
Silvertown, J. (2009). A new dawn for citizen science. Trends Ecol. Evol. 24, 467–471. doi: 10.1016/j.tree.2009.03.017
Sirca, A., Horvat, M., Rajar, R., Covelli, S., Zagar, D., and Faganeli, J. (1999). Estimation of mercury mass balance in the Gulf of Trieste. Acta Adriatica 40, 75–85.
Stravisi, F. (1983). Some characteristics of the circulation in the Gulf of Trieste. Thalassia Jugosl. 19, 355–363.
Sutherland, W. J., Roy, D. B., and Amano, T. (2015). An agenda for the future of biological recording for ecological monitoring and citizen science. Biol. J. Linn. Soc. 115, 779–784. doi: 10.1111/bij.12576
Tomašovych, A., Gallmetzer, I., Haselmair, A., Kaufman, D. S., Kralj, M., Cassin, D., et al. (2018). Tracing the effects of eutrophication on molluscan communities in sediment cores: outbreaks of an opportunistic species coincide with reduced bioturbation and high frequency of hypoxia in the Adriatic Sea. Paleobiology 44, 575–602. doi: 10.1017/pab.2018.22
Urbini, L., Ingrosso, G., Djakovac, T., Piacentino, S., and Giani, M. (2020). Temporal and spatial variability of the CO2 system in a riverine influenced area of the mediterranean Sea, the Northern Adriatic. Front. Mar. Sci. 7:679. doi: 10.3389/fmars.2020.00679
Vidović, J., Nawrot, R., Gallmetzer, I., Haselmair, A., Tomašových, A., Stachowitsch, M., et al. (2016). Anthropogenically induced environmental changes in the northeastern Adriatic Sea in the last 500 years (Panzano Bay. Gulf of Trieste). Biogeosciences 13, 5965–5981. doi: 10.5194/bg-13-5965-2016
Vozila, A. B., Güttler, I., Ahrens, B., Obermann-Hellhund, A., and Prtenjak, M. T. (2019). Wind over the adriatic region in CORDEX climate change scenarios. J. Geophys. Res. Atmos. 124, 110–130. doi: 10.1029/2018jd028552
Keywords: crowdsourcing, citizen science (CS), sea water quality, infrastructure, environmental awareness, Gulf of Trieste (North Adriatic Sea)
Citation: Diviacco P, Nadali A, Iurcev M, Carbajales R, Busato A, Pavan A, Burca M, Grio L, Nolich M, Molinaro A and Malfatti F (2021) MaDCrow, a Citizen Science Infrastructure to Monitor Water Quality in the Gulf of Trieste (North Adriatic Sea). Front. Mar. Sci. 8:619898. doi: 10.3389/fmars.2021.619898
Received: 21 October 2020; Accepted: 21 June 2021;
Published: 22 July 2021.
Edited by:
Sven Schade, European Commission, Joint Research Centre (JRC), ItalyReviewed by:
Steven A. Loiselle, University of Siena, ItalyCopyright © 2021 Diviacco, Nadali, Iurcev, Carbajales, Busato, Pavan, Burca, Grio, Nolich, Molinaro and Malfatti. This is an open-access article distributed under the terms of the Creative Commons Attribution License (CC BY). The use, distribution or reproduction in other forums is permitted, provided the original author(s) and the copyright owner(s) are credited and that the original publication in this journal is cited, in accordance with accepted academic practice. No use, distribution or reproduction is permitted which does not comply with these terms.
*Correspondence: Paolo Diviacco, pdiviacco@inogs.it
Disclaimer: All claims expressed in this article are solely those of the authors and do not necessarily represent those of their affiliated organizations, or those of the publisher, the editors and the reviewers. Any product that may be evaluated in this article or claim that may be made by its manufacturer is not guaranteed or endorsed by the publisher.
Research integrity at Frontiers
Learn more about the work of our research integrity team to safeguard the quality of each article we publish.