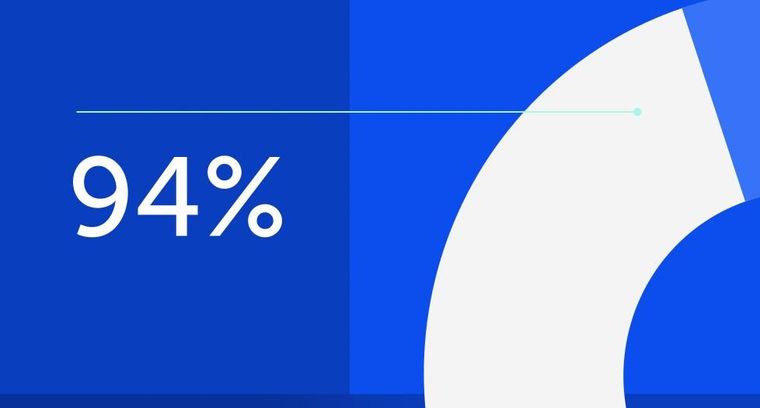
94% of researchers rate our articles as excellent or good
Learn more about the work of our research integrity team to safeguard the quality of each article we publish.
Find out more
PERSPECTIVE article
Front. Mar. Sci., 20 August 2021
Sec. Ocean Observation
Volume 8 - 2021 | https://doi.org/10.3389/fmars.2021.647368
This article is part of the Research TopicSustainable Development Goal 14 - Life Below Water: Towards a Sustainable OceanView all 25 articles
Coastal observing systems are typically nationally funded and built around national priorities. As a result, there are presently significant differences between countries in terms of sustainability, observing capacity and technologies, as well as methods and research priorities. Ocean observing systems in coastal areas must now move toward an integrated, multidisciplinary and multiscale system of systems, where heterogeneity should be exploited to deliver fit-for-purpose products that answer the diversity and complexity of the requirements from stakeholders and end-users. Essential elements of such distributed observation systems are the use of machine-to-machine communication, data fusion and processing applying recent technological developments for the Internet of Things (IoT) toward a common cyberinfrastructure. This perspective paper illustrates some of the challenges for sustained coastal observations and provides details on how to address present gaps. We discuss the role of collaborative robotics between unmanned platforms in coastal areas and the methods to benefit from IoT technologies. Given present trends in cost-effective solutions in ocean sensors and electronics, and methods for marine automation and communication, we consider that a distributed observation system can effectively provide timely information in coastal regions around the world, including those areas that are today poorly observed (e.g., developing countries). Adaptation in space and time of the sensing nodes, and the flexibility in handling different sensing platforms can provide to the system the ability to quickly respond to the rapid changes in oceanic and climatic processes, as well as to promptly respond to evolving stakeholder and end-user requirements.
Coastal areas are the most dynamic and productive parts of the oceans, which makes them a significant source of resources and services for mankind. Coastal waters are located immediately in contact with human populations and exposed to anthropogenic disturbances, placing these resources and services under threat (e.g., Lynch et al., 2014). These concerns explain why, in several coastal regions, a rapidly increasing number of observing systems have been implemented in the last decade (Moltmann et al., 2019). Expansion of coherent and sustained coastal observations has been fragmented and driven by national and regional policies and is often undertaken through short-term research projects (Farcy et al., 2019). This results in significant differences between countries both in terms of sustainability and observing technologies, methods and research priorities.
Unlike the open ocean, where challenges are rather well-defined and stakeholders are fewer and well-identified, coastal processes are complex, acting on several spatial and temporal scales, with numerous and diversified users and stakeholders, often with conflicting interests. To adapt to such complexity coastal ocean observing system must be an integrated, multidisciplinary and multiscale system of systems (GOOS, 2012). But the diversification in data acquisition, handling and storage can inevitably create problems in data management and delivery, hampering interoperability and limiting opportunities to advance our knowledge on coastal processes and resource management.
Looking at the future, system’s diversification will likely increase as new sensors and platforms become available, activities in new sectors of the ocean economy are developed, and an increasing number of users will provide heterogeneous societal demands for specific observations. In this perspective we present some of the challenges for sustained coastal observations and illustrate methods to address them. We suggest an approach to exploit current and future system heterogeneity while serving the needs of a sustainable and robust coastal observation. The specific aim of such a system should be harmonized and autonomous acquisition, use of best-practices for data handling and storage and to provide information to enable ecosystem based management of the ocean while responding to data requirements of different blue-economy sectors.
Coastal systems demands the use of diverse observing platforms for the collection of relevant oceanographic variables, i.e., Essential Ocean Variables (EOVs, Lindstrom et al., 2012; Bax et al., 2018). Present solutions are based on different approaches which include a limited number of cabled observatories, collecting high frequency data on a larger number of EOVs, supplemented by a variety of other platforms typically observing a lower number of variables (Figure 1). Similarly, the expected expansion of citizen science programs (Kelly et al., 2020) into the ocean domain can enable in the future many more devices observing selected variables. This intrinsic heterogeneity in observation systems is a challenge for centralized management. But, when managed in a coordinated way, it could offer more flexibility and the opportunity to optimally respond to future ocean data needs. Indeed, a network of heterogeneous systems can better adapt and promptly react to diverse and complex coastal processes and the diverse user demands and priorities, hence delivering fit-for-purpose data and products.
Figure 1. Schematic of the number of observed essential ocean variables (EOVs) from a specific platform vs. the available number of these platforms. A distributed system for observations will use all its nodes to deliver fit- for- purpose information, products and services. Cobs, cabled observatories; RVs, research vessels; AnBS, animal borne sensors; CS, citizen science. Note: additional dimensions could be included in the plot, e.g., observation range, cost of the platforms, etc.
In this perspective we present methods to move toward a distributed system for coastal ocean observations composed of a network of fixed and mobile heterogeneous nodes, which can coordinate data acquisition tasks and data management. Diversity and heterogeneity are two key characteristics which can increase system resilience, as evidenced during the COVID-19 pandemic when the overall observation system performance was rather unaffected although some platforms were impacted. Nodes of the network are stand-alone platforms (e.g., buoys, moorings), cabled observatories, research vessels, FerryBoxes, autonomous underwater and surface vehicles, gliders, bio-logging, satellites and different low-cost, low-power sensors systems, e.g., from citizen science initiatives. Communications in the network are enabled exploiting Internet of Things (IoT) technologies. Data management is performed over a web-infrastructure with near-real-time (nRT) communications, long term storage, secure data retrieval and services for data processing that are offered online by exploiting cloud technologies and services.
An important feature is machine-to-machine (m2m) communication among the nodes to enable adaptive sampling. When specific signals are recorded by one node (e.g., harmful algal bloom, oil spills, ocean heatwaves, etc.) other nodes can refine the event’s data acquisition strategy (e.g., extend geographical coverage, provide higher sampling rate, etc.) using collaborative robotic solutions. The distributed structure will also ensure a better tolerance to individual node failures and enable the flexibility to satisfy specific tasks (e.g., aquaculture monitoring, support to tourism, etc.). The complexity of the network structure and functioning is generally hidden to the final users (Carpenter and Cannady, 2004; Crise et al., 2018; Montella et al., 2018) and this process of abstraction is relevant to deliver a portfolio of products. Special interest groups may have specific needs that can be met by these ad hoc products and the requirements can change in time and space (e.g., the constant evolution of marine directives) along with the technological advances. Services on the cyberinfrastructure are customizable by the end-users according to the requirements of the specific use (i.e., precision, accuracy, sampling frequency, etc.). Thus, both data and products have to follow FAIR principles (Findable, Accessible, Interoperable, Reusable; Wilkinson et al., 2016), although regional economic, legal and security aspects could in same cases limit data access.
In this context IoT solutions could be exploited for the interconnection at different scales: locally (e.g., sensors), regionally (e.g., platforms) and globally (e.g., observatories). In particular, they include the coordinated (common objective—yet separate execution) and cooperative (common objective—frequent interaction) operations of the components including domain specific high computing facilities for numerical ocean modeling tasks. In the future deployment bottlenecks in the area of interoperability (i.e., common calibration and communication standards) are to be expected.
An extended review of the challenges for global ocean observing system is presented elsewhere (e.g., Moltmann et al., 2019). The use of best practices and recent technology for sensors and data interoperability (Buck et al., 2019), unmanned marine platforms (Testor et al., 2019), cabled observatories (Howe et al., 2019), and marine observatories (Crise et al., 2018) have all been identified to contribute toward implementation of sustainable ocean observations. Here we focus on the role of m2m coordination and IoT techniques as essential elements for the operationalization of a sustained distributed ocean sensing system in coastal regions.
The nodes of the distributed system are sensing platforms that have standardized metadata information accessible via a common cyberinfrastructure (e.g., a dashboard), including geographical as well as technical specifications on sensors and platforms. Sensor-web architectures (see del Río et al., 2017) are used to achieve process automation, sensor interoperation, and service synergy. Autonomy in the system is expanded relying on a range of unmanned platforms for surface- and underwater-operations (Domingo, 2012; Whitt et al., 2020). Cooperative and collaborative robotics approaches have been developed for autonomous marine vehicles, using different m2m communication and decision-making paradigms (Thompson and Guihen, 2019). Those approaches are not mutually exclusive although generally optimized for different data gathering missions. Specifically:
- Cooperative solutions work on a single or a small set of similar tasks to accelerate or optimize aspects of the mission (e.g., minimizing completion time; maximizing the coverage area). In these cases, decision-making and m2m communication focus on enforcing a control system that governs each participating platform. Ocean survey missions have particularly benefited from cooperative control methods resulting in solutions that are effective for monitoring over extended periods (Leonard, 2016; Ocean Infinity, 20201; Simetti et al., 2020).
- Collaborative solutions focus on complex missions that have a “deep” sequence of dependent and interdependent tasks. The m2m communication is shaped to achieve machine-consensus on task allocation and sequencing between platforms based on their metadata profiles and constraints. Collaborative robotics and m2m communications have enabled adaptive sampling (Branch et al., 2019) and extended operations (Lima et al., 2019) in coastal areas, provided an abstract mission planning paradigm.
Different multi-marine platform mission planning tools are available to specify the above tasks that can be executed by individual marine platforms (e.g., Neptus, Pereira et al., 2006; LSTS Toolchain, Pinto et al., 2013; MOOS-IvP, Benjamin et al., 2010; JANUS, Petroccia et al., 2017).
Improved onboard machine intelligence (e.g., nRT data processing, mission planning and optimization, fault response and risk management) is possible with present development in miniaturized electronics and power-efficient algorithms providing direct feedback to the control system of the sensing platform (Zhou et al., 2019). The developments of technology for underwater communication (Song et al., 2019), autonomous-docking, -calibration and -power supply (Yazdani et al., 2020) as well as bio-inspired algorithms for collective behavior and optimal search (Tholen and Nolle, 2017), can open interesting perspectives for the operations of a large fleet of autonomous platforms in coastal areas with little or no human supervision (Schmidt et al., 2016).
Nonetheless, significant technical, operational, economical and legislative challenges must be solved before conducting unmanned coastal observations as a sustainable and long-term program. The technical issues are typically related to limitations in the available power, suitable navigation solutions (for mobile systems), and sensor stability. Existing communication solutions are often expensive and power hungry. Operational challenges are often related to fouling, such as bio-fouling but also fouling due to floating or submerged debris (e.g., ghostnets). Coastal observations often suffer from effects of surface traffic, leading to collisions, unwanted recoveries or a lack thereof, thus leading to limitations in communications, maintenance and recovery. Additionally, existing legislation in coastal regions might limit the operations with unmanned vehicles. The extremely dynamic coastal domain requires significant sensor density to establish an adequate observation capability. Despite recent developments in cost-effective ocean sensors and platforms (Wang et al., 2019), often the cost of sensors alone poses a severe economic challenge to the establishment of a sustainable and comprehensive coastal observation system.
In open ocean environments satellites are the only viable communications means, but they are often expensive solutions and can present high latency. Recently, several new initiatives have promised cheaper and faster satellite-based solutions for data collection and continuous monitoring in the ocean. Nevertheless, in coastal areas other technologies are available that offer significant advantages in terms of cost, throughput and latency. For applications in which a limited number of small messages are adequate, long-range low-power wide-area networks (LPWAN) are an inexpensive solution that can reach considerable distances from the coastline.
Among the several LPWAN implementations, the most prominent are Sigfox, LoRaWAN, NB-IoT, and LTE-M (Mekki et al., 2018). Sigfox is currently deployed in more than 70 countries in all five continents, able to cover 1.3 B people2, while LoRaWAN is currently deployed in 162 countries3. When choosing among the different options, the main factors to be considered are: communication range, data rate, spectrum usage, number and size of messages, energy consumption, and cost (Mekki et al., 2019). Sigfox is a proprietary long-range low-power solution widely used for IoT, but it is quite limited in the number of messages per day and the amount of data that can be transferred. LoRa is also based on a proprietary technology, but only at the physical layer, while the data transmission protocol, namely LoRaWAN, is open and free to use and deploy. As for cellular communications, they have been traditionally leveraged for IoT, but their high-power consumption limits their use in battery-operated devices. Newer cellular technologies (e.g., NB-IoT, LTE-M) consume less power, offer longer ranges, and are protected from interference thanks to their use of licensed frequencies. Therefore, where these cellular technologies are available, they can offer a viable solution, but currently they are not yet widely deployed.
Given the characteristics of these long-range technologies (reviewed in Mekki et al., 2019; Parri et al., 2019; Cecílio et al., 2020; and Park et al., 2020) it appears that LoRa implementations are the most effective solutions for IoT-based coastal monitoring systems. A considerable advantage of LoRa is the use of unlicensed spectrum at frequencies below 1 GHz, which are available in most countries and may remove a significant factor in the cost of the communications services. This allows even small organizations to install, operate and maintain the network, independently of commercial communication providers. This can be particularly appealing for building sustainable observing systems with common protocols worldwide, including developing countries where commercial communication services can be unaffordable, or even lacking in certain areas. The use of unlicensed spectrum poses, however, limits to the maximum allowable transmission power and channel occupancy time, to allow sharing the resource among concurrent users. These limitations are country-specific but as exemplified by the great success of WiFi do not constitute a major obstacle for its widespread usage.
The open LoRaWAN protocol offers variable transmission speeds from about 300 bps up to 50 kbps and paves the way for a complete solution from the sensing ocean platform to the network server and from there to a number of application-specific web accessible servers (Figure 2). Experiments on coastal areas have already shown that LoRaWAN-based systems yield promising results (Petajajarvi et al., 2015; Parri et al., 2019). Recently, the impact of the height of the nodes when deployed in the water have been investigated (Cecílio et al., 2020), demonstrating that tides have an impact on the communication distance and reliability. A factor that needs to be considered when building the system, by for example positioning the end-nodes as high as possible.
Figure 2. Schematics of the IoT communication network in the ocean. The names in red identify dedicated platforms, in blue cooperative platforms, in green cabled platforms. The dashed lines represent the communication channels connecting platforms to the gateway using a specific wireless communication protocol depending on local availability and cost. The gateway is connected to the network server by any IP communication technology available. The network server connects to a number of specific application servers which make the data accessible through a web browser. The transmission channel employs an end-to-end AES encryption protocol. The schematics includes gliders that in the future may be connected to the system via LoRaWAN satellite communication as the other open-ocean platforms.
The LoRaWAN gateways and servers can be installed and managed directly by the interested party, but their functionalities can also be obtained from commercial providers or leveraging crowdsourcing initiatives such as the TheThingsNetwork4, a global endeavor that provides LoRaWAN services at no cost. Several end-devices installed on the sensing platform send messages to one or more LoRaWAN gateways, which will then forward them to the network server using IP-based connections (Seid et al., 2020). Nevertheless, when there is a need for higher data rates (e.g., transmission of videos) modified WiFi for long-distance offers a low-cost solution that can be directly installed by the interested organization (Pietrosemoli et al., 2014). Finally delay-tolerant transmission protocols (Msaad et al., 2020) should be considered since some devices, due to their mobile nature, might be connected with the on-land infrastructure only intermittently.
The IoT enabled devices will be crucial in the framework of Digital Ocean initiatives as they will provide the means to assimilate ocean measurements including the potential ability to modify the sampling behavior, on temporal and spatial scales, according to the needs of the digital ocean initiatives (such as the Digital Ocean Twin). This is also a prime example of a cooperative vs. coordinated system.
Essential elements toward the implementation of a distributed and autonomous architecture for ocean monitoring and observation are described to achieve the ability to measure physical, chemical, and biological variables across a range of spatial and temporal scales in coastal areas. Cooperative autonomous devices with on-board system management and data processing should be combined with low-power long-range communication technologies, to coordinate data acquisition and management and enable machine-machine interactions, to deliver fit-for-purpose information to a range of end-users with complex and diverse requirements. These transformative changes in the use of marine robotics, communication technology and autonomous data handling, can strengthen modern coastal ocean observing systems by supporting their economic viability while addressing overlapping interests of end-user groups from science, technology, industry, and policymakers.
The global ARGO program is a prime example of how such step-change can be achieved, demonstrating the successful implementation and operation of a global blue ocean observation system, technologically and scientifically, over the past 20 years with major perspectives for future developments (Roemmich et al., 2019). Albeit being a homogeneous observation system it illustrates the realization of key functionalities such as simple m2m communications, on-board data preprocessing, data quality and sensor standards as well as model integrations and bespoke data products for the open ocean.
Coastal observatories have different economic challenges compared to the open ocean, as they are nationally funded and serve the requirements of several stakeholders; e.g., water authorities, legislative bodies, aquaculture companies, etc. Hence a modular and flexible structure in the observational system is needed to adapt to local requirements while ensuring a coherent data access and interoperability. The Balearic Islands Coastal Ocean Observing and Forecasting System (SOCIB, Tintoré et al., 2013) provides operational solutions for a multi-platform, integrated and multidisciplinary observing system which is able to leverage system’s diversity and automation to better respond to end-user needs (Heslop et al., 2019). To achieve integration in such heterogenous system communication and coordination among nodes is paramount (Leonard, 2016; Thompson and Guihen, 2019) and semi-autonomous planning and monitoring tools should evolve to integrate numerical ocean and atmospheric models as well as in situ data in order to coordinate and optimize usage of the individual nodes. Underlying all of these attributes should be the adoption of standard methods and best practices to create a foundation for the desired interoperability (Pearlman et al., 2019).
The capacity of a network of heterogeneous system to transfer data is a critical issue, since the quantity of data produced by the platforms is expected to be large. Delay tolerant methods and protocols allowing local data storage and retrieval of the platform could be needed to secure collection of large data. Moreover, modified WiFi could enable near-real time long-distance information transfer of broadband data (Pietrosemoli et al., 2014). Additionally, communication of data or information from mobile systems can rely on surface radio-frequency communications or underwater communication to cabled seafloor communication nodes. While acoustic underwater communication will improve in terms of reliability and in effective bandwidth, high-bandwidth, low-energy, long-range communication (>10 km) will most likely not be achieved (Song et al., 2019). Low-power on-board data processing, fusion and data compression algorithms can alleviate some of the shortcomings imposed by the physics of the acoustic communication channel. Similarly, underwater optical communications will play in the future a more prominent role providing short range point-to-point high bandwidth (>10 Mbps) line-of-sight connectivity. It is foreseen that combined (optical, acoustic) communication systems could provide the means for reliable underwater communication for a range of environmental conditions such as those found in coastal regions.
The ability to benefit from a wide range of diverse sensing platforms to monitor EOVs, could greatly expand our ability to achieve sustained coastal observations in the global ocean. Indeed, the potential low cost of the distributed observational framework can enable data collection, handling and storage in areas that are currently poorly observed (e.g., in developing countries). The arctic region is an important example of where advanced in communication and automation can contribute to design and implementation of sustained observing system (Lee et al., 2019). A network of moored, wirelessly connected platforms, complemented by autonomous vehicles with advanced control systems and a range of low-cost sensors could provide the baseline for sustained coastal observations in many regions. Those systems might initially not have all the EOVs but could be expanded upon availability of reliable sensors to fill the EOV gaps.
The need for sensors in the coastal zone is essentially the same as for the offshore environment, however, the concentrations of the compounds of interest, nutrients, pollutants, etc., are generally higher in the shelf areas. The coastal observatories may then require more frequent servicing due to the faster degradation of sensor performances as a result of biofouling, adverse impacts of oil slicks, marine litter, etc. It has been demonstrated that regular servicing of the observatory and sensors could be performed with unmanned autonomous vehicles (Barceló-Llull et al., 2019; Scoulding et al., 2020) which could also collect samples for ground-truthing to be analyzed using standard techniques to ensure that the data collected are reliable. The availability of reliable and cost-effective ocean sensors is central to the implementation of large distributed observation networks. Some of the required sensors to monitor EOVs are not yet available, but considering the fast evolving technology, many of the variables not covered now, will be likely covered in the near future (Wang et al., 2019).
The original contributions presented in the study are included in the article, further inquiries can be directed to the corresponding author/s.
PM and AC provided the main concept with contributions from RB, SK, and EP. PM drafted the first version of the manuscript. AC and PM primarily introduced the network of distributed observations for coastal areas. RB, ED, and FT primarily contributed to the automation and collaborative robotics parts. EP and SK primarily contributed to IoT communication technologies. All authors provided collaborative effort for this work. All authors contributed to the text for the introduction, discussions and in providing relevant concepts and references for the different sections.
PM and RB acknowledge the contribution of the European Union’s Horizon 2020 Research and Innovation Project MISSION ATLANTIC under grant agreement no. 862428. ED acknowledges the contribution of the European Union’s Horizon 2020 Research and Innovation Project “JERICO-S3” under grant agreement no. 871153. We acknowledge the support of EUROMARINE network under the foresight workshop grant.
The reviewer AG declared a shared committee with authors ED, JP, and GP at the time of review.
The remaining authors declare that the research was conducted in the absence of any commercial or financial relationships that could be construed as a potential conflict of interest.
All claims expressed in this article are solely those of the authors and do not necessarily represent those of their affiliated organizations, or those of the publisher, the editors and the reviewers. Any product that may be evaluated in this article, or claim that may be made by its manufacturer, is not guaranteed or endorsed by the publisher.
This manuscript is the outcome of the Euromarine workshop ASIMO “Autonomous systems for integrated marine and maritime coastal observations,” Gran Canaria, Spain, September 17–19, 2018. We would like to thank Valentina Mosetti for generating the original Figure 2 in the manuscript.
Barceló-Llull, B., Pascual, A., Ruiz, S., Escudier, R., Torner, M., and Tintoré, J. (2019). Temporal and spatial hydrodynamic variability in the Mallorca channel (western Mediterranean Sea) from 8 years of underwater glider data. J. Geophys. Res. 124, 2769–2786. doi: 10.1029/2018jc014636
Bax, N. J., Appeltans, W., Brainard, R., Duffy, J. E., Dunstan, P., Hanich, Q., et al. (2018). Linking capacity development to GOOS monitoring networks to achieve sustained ocean observation. Front. Mar. Sci. 5:346.
Benjamin, M. R., Schmidt, H., Newman, P. M., and Leonard, J. J. (2010). Nested autonomy for unmanned marine vehicles with MOOS-IvP. J. Field Robot. 27, 834–875. doi: 10.1002/rob.20370
Branch, A., Flexas, M. M., Claus, B., Thompson, A. F., Zhang, Y., Clark, E. B., et al. (2019). Front delineation and tracking with multiple underwater vehicles. J. Field Robot. 2019, 568–586. doi: 10.1002/rob.21853
Buck, J. J., Bainbridge, S. J., Burger, E. F., Kraberg, A. C., Casari, M., Casey, K. S., et al. (2019). Ocean data product integration through innovation - The next level of data interoperability. Front. Mar. Sci. 6:32. doi: 10.3389/fmars.2019.00032
Carpenter, S., and Cannady, J. (2004). “Tool for sharing and assessing models of fusion-based space transportation systems,” in Proceedings of the 40th AIAA/ASME/SAE/ASEE Joint Propulsion Conference and Exhibit (New York, NY), doi: 10.2514/6.2004-3535
Cecílio, J., Ferreira, P. M., and Casimiro, A. (2020). Evaluation of LoRa Technology in Flooding Prevention Scenarios. Sensors 14:4034. doi: 10.3390/s20144034
Crise, A., Ribera, d’Alcalà, M., Mariani, P., Petihakis, G., Robidart, J., et al. (2018). A Conceptual Framework for Developing the next Generation of Marine OBservatories (MOBs) for Science and Society. Front. Mar. Sci. 5, 1–8. doi: 10.3389/fmars.2018.00318
del Río, J., Toma, D. M., Martínez, E., O’Reilly, T. C., Delory, E., Pearlman, J. S., et al. (2017). A sensor web architecture for integrating smart oceanographic sensors into the semantic sensor web. IEEE J. Oceanic Eng. 43, 830–842. doi: 10.1109/JOE.2017.2768178
Domingo, M. C. (2012). An overview of the internet of underwater things. J. Netw. Comput. Appl. 35, 1879–1890. doi: 10.1016/j.jnca.2012.07.012
Farcy, P., Durand, D., Charria, G., Painting, S. J., Tamminen, T., Collingridge, K., et al. (2019). Towards a European Coastal Observing Network to provide better answer to science and to societal challenges; the JERICO/JERICO-NEXT Research Infrastructure. Front. Mar. Sci. 6:529. doi: 10.3389/fmars.2019.00529
GOOS (2012). Requirements for Global Implementation of the Strategic Plan for Coastal GOOS. Rep. Glob. Ocean Observ. Syst. 193:200.
Heslop, E., Tintoré, J., Rotllan, P., Álvarez-Berastegui, D., Fontera, B., Mourre, B., et al. (2019). SOCIB integrated multi-platform ocean observing and forecasting: from ocean data to sector-focused delivery of products and services. J. Operat. Oceanogr. 12, S67–S79.
Howe, B. M., Arbic, B. K., Aucan, J., Barnes, C., Bayliff, N., Becker, N., et al. (2019). Smart Cables for Observing the Global Ocean: Science and Implementation. Front. Mar. Sci. 6, 1–27. doi: 10.3389/fmars.2019.00424
Kelly, R., Fleming, A., Pecl, G. T., von Gönner, J., and Bonn, A. (2020). Citizen science and marine conservation: a global review. Philosoph. Trans. R. Soc. B 375:20190461. doi: 10.1098/rstb.2019.0461
Lee, C. M., Starkweather, S., Eicken, H., Timmermans, M. L., Wilkinson, J., Sandven, S., et al. (2019). A framework for the development, design and implementation of a sustained arctic ocean observing system. Front. Mar.Sci. 6:451. doi: 10.3389/fmars.2019.00451
Leonard, N. E. (2016). “Cooperative Vehicle Environmental Monitoring,” in Springer Handbook of Ocean Engineering, eds N. I. Dhanak and M. R. Xiros (Berlin: Springer), 441–458. doi: 10.1007/978-3-319-16649-0_19
Lima, K., Dias, P. S., Costa, M., Pinto, J., Marques, T., Sousa, J. B., et al. (2019). “REP18 Atlantic–A Large Scale Exercise Using Unmanned Systems, Field Report,” in OCEANS 2019-Marseille (New York, NY: IEEE), 1–7.
Lindstrom, E., Gunn, J., Fischer, A., McCurdy, A., Glover, L., Alverson, K., et al. (2012). A Framework for Ocean Observing. By the Task Team for an Integrated Framework for Sustained Ocean Observing, UNESCO 2012, IOC/INF-1284. Available online at: http://www.oceanobs09.net/foo/FOO_Report.pdf (accessed March 20, 2021)
Lynch, T. P., Morello, E. B., Evans, K., Richardson, A. J., Rochester, W., et al. (2014). IMOS National Reference Stations: A Continental-Wide Physical, Chemical and Biological Coastal Observing System. PLoS One 9:e113652. doi: 10.1371/journal.pone.0113652
Mekki, K., Bajic, E., Chaxel, F., and Meyer, F. (2019). A comparative study of LPWAN technologies for large-scale IoT deployment. ICT Exp. 5, 1–7. doi: 10.1016/j.icte.2017.12.005
Mekki, K., Bajic, E., and Meyer, F. (2018). “Overview of cellular LPWAN technologies for IoT deployment: sigfox, LoRaWAN, and NB-IoT,” in Procedings of the 2018 IEEE International Conference on Pervasive Computing and Communications Workshops (PerCom Workshops), 197–202. doi: 10.1109/PERCOMW.2018.8480255
Moltmann, T., Zhang, H. M., Turton, J. D., Nolan, G., Gouldman, C. C., Griesbauer, L., et al. (2019). A Global Ocean Observing System (GOOS), delivered through enhanced collaboration across regions, communities, and new technologies. Front. Mar. Sci. 6:291. doi: 10.3389/fmars.2019.00291
Montella, R., Kosta, S., and Foster, I. (2018). “DYNAMO: Distributed leisure yacht-carried sensor-network for atmosphere and marine data crowdsourcing applications,” in 2018 IEEE International Conference on Cloud Engineering (IC2E) (New York, NY: IEEE), 333–339. doi: 10.1109/IC2E.2018.00064
Msaad, M., Hambly, A., Mariani, P., and Kosta, S. (2020). Mobile and Delay Tolerant Network for LoRa at Sea. In Proceedings of the Student Workshop (CoNEXT’20). New York, NY: Association for Computing Machinery, 3–4. doi: 10.1145/3426746.3434053
Park, S., Ling, T. C., Cha, B., and Kim, J. (2020). Design of containerized marine knowledge system based on IoT-Cloud and LoRaWAN. Pers Ubiquit. Comput. 2020:8. doi: 10.1007/s00779-020-01381-8
Parri, L., Parrino, S., Peruzzi, G., and Pozzebon, A. (2019). Low power wide area networks (LPWAN) at Sea: Performance analysis of offshore data transmission by means of LoRaWAN connectivity for marine monitoring applications. Sensors 19:3239. doi: 10.3390/s19143239
Pearlman, J., Bushnell, M., Coppola, L., Buttigieg, P. L., Pearlman, F., Simpson, P., et al. (2019). Evolving and Sustaining Ocean Best Practices and Standards for the Next Decade. Front. Mar. Sci. 6:277. doi: 10.3389/fmars.2019.00277
Pereira, F. L., Pinto, J., Sousa, J. B., Gomes, R. M. F., Gonçalves, G. M., and Dias, P. S. (2006). “Mission planning and specification in the Neptus framework,” in Proceedings 2006 IEEE International Conference on Robotics and Automation, 2006 (New York, NY: IEEE), 3220–3225.
Petajajarvi, J., Mikhaylov, K., Roivainen, A., Hanninen, T., and Pettissalo, M. (2015). “On the coverage of LPWANs: range evaluation and channel attenuation model for LoRa technology,” in 2015 14th International Conference on ITS Telecommunications (ITST), Copenhagen, (Denmark), 55–59. doi: 10.1109/ITST.2015.7377400
Petroccia, R., Alves, J., and Zappa, G. (2017). JANUS-based services for operationally relevant underwater applications. IEEE J. Oceanic Eng. 42, 994–1006. doi: 10.1109/joe.2017.2722018
Pietrosemoli, E., Zennaro, M., Bastianini, M., and Minuzzo, T. (2014). BLOP: Broadband Link to an Offshore Platform in the Venice Lagoon In ExtremeComm’14. Pacific Ocean: Galapagos Islands, Ecuador, 2014.
Pinto, J., Dias, P. S., Martins, R., Fortuna, J., Marques, E., and Sousa, J. (2013). The LSTS Toolchain for Networked Vehicle Systems In 2013 MTS/IEEE OCEANS-Bergen. New York, NY: IEEE, 1–9.
Roemmich, D., Alford, M. H., Claustre, H., Johnson, K., King, B., Moum, J., et al. (2019). On the future of Argo: a global, full-depth, multi-disciplinary array. Front. Mar. Sci. 6:439. doi: 10.3389/fmars.2019.00439
Schmidt, H., Benjamin, M. R., Petillo, S. M., and Lum, R. (2016). Nested Autonomy for Distributed Ocean Sensing. Cham: Springer, 459–480.
Scoulding, B., Kloser, R., and Gastauer, S. (2020). Evaluation of unmanned surface vehicle acoustics for gas seep detection in shallow coastal waters. Internat. J. Green. Gas Contr. 102:103158. doi: 10.1016/j.ijggc.2020.103158
Seid, S., Zennaro, M., Libsie, M., Pietrosemoli, E., and Manzoni, P. (2020). “A low cost edge computing and LoRaWAN real time video analytics for road traffic monitoring,” in Procedings of the 16th International Conference on Mobility, Sensing and Networking (MSN) (Piscataway, NJ: IEEE), 762–767. doi: 10.1109/MSN50589.2020.00130
Simetti, E., Indiveri, G., and Pascoal, A. M. (2020). WiMUST: A cooperative marine robotic system for autonomous geotechnical surveys. J. Field Robot. 2020:21986. doi: 10.1002/rob.21986
Song, A., Stojanovic, M., and Chitre, M. (2019). Underwater acoustic communications: Where we stand and what is next? IEEE J. Ocean. Eng. 44, 1–6. doi: 10.1109/joe.2018.2883872
Testor, P., de Young, B., Rudnick, D. L., Glenn, S., Hayes, D., Lee, C. M., et al. (2019). OceanGliders: A Component of the Integrated GOOS. Front. Mar. Sci. 6:422. doi: 10.3389/fmars.2019.00422
Tholen, C., and Nolle, L. (2017). “Parameter Search for a Small Swarm of AUVs Using Particle Swarm Optimisation,” in Artificial Intelligence XXXIV. SGAI 2017. Lecture Notes in Computer Science, Vol. 10630, eds M. Bramer and M. Petridis (Cham: Springer), doi: 10.1007/978-3-319-71078-5_32
Thompson, F., and Guihen, D. (2019). Review of mission planning for autonomous marine vehicle fleets. J. Field Robot. 36, 333–354. doi: 10.1002/rob.21819
Tintoré, J., Vizoso, G., Casas, B., Heslop, E., Pascual, A., Orfila, A., et al. (2013). SOCIB: the Balearic Islands coastal ocean observing and forecasting system responding to science, technology and society needs. Marine Technology Society Journal 47, 101–117. doi: 10.4031/mtsj.47.1.10
Wang, Z. A., Moustahfid, H. A., Mueller, A., Mowlem, M. C., Michel, A. P. M., Glazer, B. T., et al. (2019). Advancing observation of ocean biogeochemistry, biology, and ecosystems with cost-effective in situ sensing technologies. Front. Mar. Sci. 6:519. doi: 10.3389/fmars.2019.00519
Whitt, C., Pearlman, J., Polagye, B., Caimi, F., Muller-Karger, F., Copping, A., et al. (2020). Future vision for autonomous ocean observations. Front. Mar. Sci. 7:697.
Wilkinson, M. D., Dumontier, M., Aalbersberg, I. J., Appleton, G., Axton, M., Baak, A., et al. (2016). The FAIR Guiding Principles for scientific data management and stewardship. Sci. Data 3, 1–9. doi: 10.1038/sdata.2016.18
Yazdani, A. M., Sammut, K., Yakimenko, O., and Lammas, A. (2020). A survey of underwater docking guidance systems. Robot. Auton. Syst. 124:103382. doi: 10.1016/j.robot.2019.103382
Keywords: marine automation, oceanography, robotics, internet of things, communication systems
Citation: Mariani P, Bachmayer R, Kosta S, Pietrosemoli E, Ardelan MV, Connelly DP, Delory E, Pearlman JS, Petihakis G, Thompson F and Crise A (2021) Collaborative Automation and IoT Technologies for Coastal Ocean Observing Systems. Front. Mar. Sci. 8:647368. doi: 10.3389/fmars.2021.647368
Received: 29 December 2020; Accepted: 26 July 2021;
Published: 20 August 2021.
Edited by:
Tomaso Fortibuoni, Istituto Superiore per la Protezione e la Ricerca Ambientale (ISPRA), ItalyReviewed by:
Sophia Merrifield, University of California, San Diego, United StatesCopyright © 2021 Mariani, Bachmayer, Kosta, Pietrosemoli, Ardelan, Connelly, Delory, Pearlman, Petihakis, Thompson and Crise. This is an open-access article distributed under the terms of the Creative Commons Attribution License (CC BY). The use, distribution or reproduction in other forums is permitted, provided the original author(s) and the copyright owner(s) are credited and that the original publication in this journal is cited, in accordance with accepted academic practice. No use, distribution or reproduction is permitted which does not comply with these terms.
*Correspondence: Patrizio Mariani, cG1hckBkdHUuZGs=
Disclaimer: All claims expressed in this article are solely those of the authors and do not necessarily represent those of their affiliated organizations, or those of the publisher, the editors and the reviewers. Any product that may be evaluated in this article or claim that may be made by its manufacturer is not guaranteed or endorsed by the publisher.
Research integrity at Frontiers
Learn more about the work of our research integrity team to safeguard the quality of each article we publish.