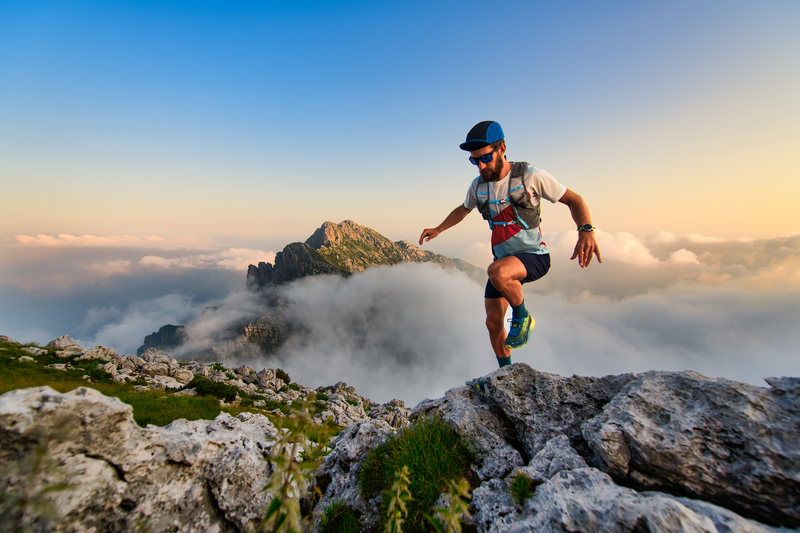
94% of researchers rate our articles as excellent or good
Learn more about the work of our research integrity team to safeguard the quality of each article we publish.
Find out more
ORIGINAL RESEARCH article
Front. Genet.
Sec. Computational Genomics
Volume 16 - 2025 | doi: 10.3389/fgene.2025.1532651
This article is part of the Research Topic The Application of Multi-omics Analysis in Translational Medicine View all 3 articles
The final, formatted version of the article will be published soon.
You have multiple emails registered with Frontiers:
Please enter your email address:
If you already have an account, please login
You don't have a Frontiers account ? You can register here
Motivation: How to predict the response of cell lines to characteristic drugs based on multi-omics gene information has become the core problem of precision oncology. At present, drug response prediction with multi-omics gene data mainly faces the following three challenges: First, how to design a gene probe feature extraction model with biological interpretation and high performance. Second, how to design multi-omics weighting modules for reasonable fusion of genetic data of different lengths and noise conditions. Third, how to design deep learning models in the case of small samples to avoid the risk of possible overfitting.We propose an innovative drug response prediction model (NMDP). Firstly, NMDP model proposes an interpretable semi-supervised weighted SPCA module to solve the feature extraction problem of multi-omics gene data. Next, we construct a multi-omics data fusion framework based on sample similarity network, bimodal test and variance information, which solves the data fusion problem and enables the NMDP model to focus on more important genomic data. Finally, we combined onedimensional convolution and KANs (Kolmogorov-Arnold Networks) method to predict drug response. We conduct 5 sets of real data experiments and compare 7 advanced drug response prediction methods which show that NMDP has the best performance, its sensitivity and specificity can reach 0.92 and 0.93, due to the comparison model 11%-57%. Bioenrichment experiments strongly proved that the NMDP model has good biological interpretation and can select potential drug activity prediction targets.
Keywords: Drug response prediction, feature extraction, Sparse PCA, KANS, DATA FUSION
Received: 02 Dec 2024; Accepted: 24 Feb 2025.
Copyright: © 2025 Miao, Zhong, Mei, Dong, Ou, Liang, Yu, Wang and Dong. This is an open-access article distributed under the terms of the Creative Commons Attribution License (CC BY). The use, distribution or reproduction in other forums is permitted, provided the original author(s) or licensor are credited and that the original publication in this journal is cited, in accordance with accepted academic practice. No use, distribution or reproduction is permitted which does not comply with these terms.
* Correspondence:
Rui Miao, Zunyi Medical University, Zunyi, China
Disclaimer: All claims expressed in this article are solely those of the authors and do not necessarily represent those of their affiliated organizations, or those of the publisher, the editors and the reviewers. Any product that may be evaluated in this article or claim that may be made by its manufacturer is not guaranteed or endorsed by the publisher.
Research integrity at Frontiers
Learn more about the work of our research integrity team to safeguard the quality of each article we publish.