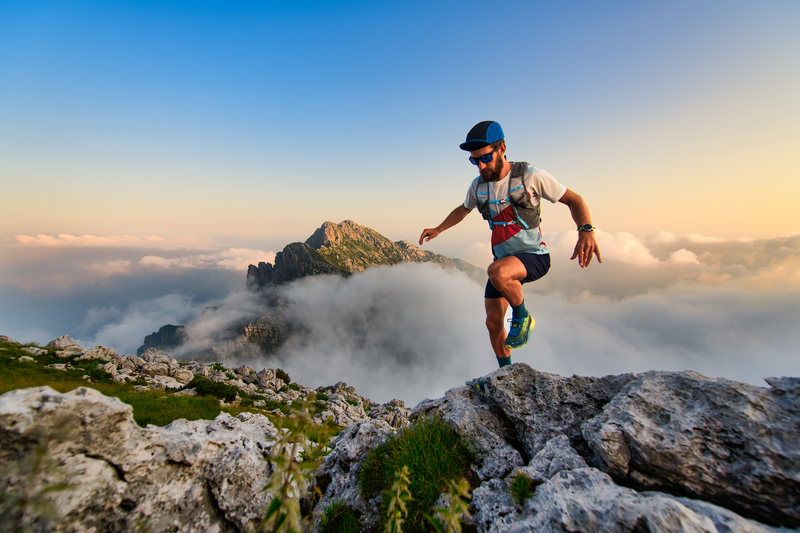
95% of researchers rate our articles as excellent or good
Learn more about the work of our research integrity team to safeguard the quality of each article we publish.
Find out more
ORIGINAL RESEARCH article
Front. Environ. Sci. , 11 December 2024
Sec. Environmental Economics and Management
Volume 12 - 2024 | https://doi.org/10.3389/fenvs.2024.1519258
This article is part of the Research Topic Advancing Carbon Reduction and Pollution Control Policies Management: Theoretical, Application, and Future Impacts View all 32 articles
Green innovation serves as a driving force for enterprises to enhance their competitiveness and may offer an effective pathway for reducing carbon emissions. This study, using data on green innovation and carbon emissions from publicly listed companies between 2000 and 2022, examines the impact of green innovation on enterprise carbon emissions and its specific transmission channels. The findings reveal that: 1) Green innovation significantly reduces enterprise carbon emissions, and this inhibitory effect remains robust across a series of stability tests. 2) Mechanistically, green innovation primarily decreases carbon emissions through improvements in energy efficiency and the specialization of enterprise management. 3) Compared to the application for green utility model patents, green innovation through the application for green invention patents is more effective in reducing enterprise carbon emissions. The carbon emission reduction effect of green innovation is found to be more pronounced in non-state-owned enterprises compared to state-owned enterprises. Furthermore, when comparing the pollution intensity and technological complexity of industries, the suppressive effect of green innovation on carbon emissions is stronger for enterprises operating in highly polluting and technologically complex industries. The findings of this study provide empirical evidence to support the promotion of green innovation for facilitating the green and low-carbon transition of manufacturing enterprises in developing countries.
After China joined the WTO in 2001, its market doors opened wider, prompting a surge in exports driven by the large international demand. China gradually became a manufacturing powerhouse, supplying industrial products worldwide. However, its export structure, primarily consisting of resource-based, labor-intensive products, along with weak domestic environmental regulations, led China to grow as a trading giant at the expense of mounting environmental issues. Notably, these include the low-value chain lock-in and associated carbon emissions transferred through global trade. The Paris Climate Agreement marked a new phase in global carbon reduction efforts (Guo and Xiong, 2024), with China—the world’s largest carbon emitter—placing significant emphasis on energy conservation and carbon reduction in recent years (Wang et al., 2023). In 2020, during the 75th United Nations General Assembly, China proposed its “dual-carbon” goal, aiming to peak carbon emissions by 2030 and achieve carbon neutrality by 2060. Accelerating progress towards these goals has underscored the critical importance of reducing enterprise carbon emissions, as companies play a micro-level role in pollution control and emissions reduction (Han et al., 2024). Meanwhile, green innovation has become a pivotal strategy for economies to pursue green transitions and develop new international competitive advantages. China’s Ministry of Industry and Information Technology (MIIT) has outlined specific emissions reduction targets in the “14th Five-Year Plan for Industrial Green Development,” emphasizing the need to accelerate technological innovation. Green innovation, which carries both knowledge and environmental spillover effects, has the potential to improve enterprise carbon performance (Cai and Li, 2018). Against this backdrop, whether green innovation aids in reducing enterprise carbon emissions is a question worthy of investigation, with particular interest in how it can effectively regulate corporate carbon emissions—a topic of growing societal concern.
Some scholars suggest that green innovation reduces environmental risks through the creation of new products and technologies (Castellacci and Lie, 2017; Chen and Xie, 2024). An increasing number of enterprises are integrating green innovation into their business strategies; however, the effects of green innovation remain debated among scholars. One group has investigated the positive impacts of green innovation on financial performance (Aguilera-Caracuel and Ortiz-de-Mandojana, 2013; De Azevedo Rezende et al., 2019; Vasileiou et al., 2022), knowledge acquisition (Martínez-Ros and Kunapatarawong, 2019), company performance (Küçükoğlu and Pınar, 2015; Arfi et al., 2018; Zhang et al., 2019), enterprise value (Hao et al., 2021; Chen et al., 2022), competitive advantage (Tu and Wu, 2021), energy efficiency (Wurlod and Noailly, 2018; Sun et al., 2019), financing constraints (Zhang et al., 2020), and business sustainability (Li L. et al., 2020). These researchers generally conclude that green innovation has a positive impact on enterprises.
In contrast, other scholars argue that green innovation is inherently uncertain (Li K. J. et al., 2020). Enterprises may lack the necessary financial resources, and those that adopt green innovations often face risks where investment costs exceed returns (Yang et al., 2015; Xia et al., 2020), potentially reducing their motivation for green innovation due to the associated risks (Geng and Zhao, 2020). A series of studies have examined the factors influencing carbon emissions, focusing primarily on green finance (Umar and Safi, 2023), financial accessibility (Wang and Fan, 2024), digital transformation (Zhang et al., 2024; Chen et al., 2024; Liu et al., 2024), the digital economy (Jiang et al., 2024; Li and Zhou, 2024; Yu et al., 2024), economic uncertainty (Ma and Zhu, 2024), monetary policy (Wu et al., 2024), information and communication technology (Wang et al., 2024), and digital infrastructure (Che et al., 2024). Building on this foundation, some scholars have examined the environmental impact of green innovation. For instance, Liu et al. (2022a), using data from 30 provinces, found that green innovation can reduce carbon emission intensity. Xu et al. (2021), analyzing data at the city level in China, reported that green innovation’s impact on carbon emission performance is primarily driven by industrial structure, urbanization, and foreign direct investment effects. Zhao et al. (2024) similarly examined urban data from China and identified the digital economy as a major channel through which green innovation influences carbon emission performance. Singh et al. (2020) explored the impact of green transformational leadership and green human resource management on environmental performance from an enterprise perspective. At the micro level, Wu et al. (2022) suggested that green innovation influences firms’ total factor productivity by affecting technological distance. Peng et al. (2020) believe that green innovation can reduce corporate costs and increase corporate profits to a certain extent, thereby indirectly reducing environmental pollution. Hojnik and Ruzzier (2016) also affirmed that green innovation can alleviate environmental pressure. Different from the above view, Zheng et al. (2023), Zhan and Pu (2024) believe that there is a U-shaped relationship between green innovation and carbon emissions, which initially reduces carbon emissions and promotes carbon emissions once the critical point is reached.
By reviewing existing literature, it is evident that no research has yet focused on the impact of green innovation on enterprises’ carbon dioxide emissions. This paper seeks to address this gap by analyzing the carbon reduction effects of green innovation from a micro-level perspective. The potential marginal contributions of this paper, compared with existing studies, are as follows: 1) The study extends research on green innovation and carbon dioxide emissions to the enterprise level. While current studies on green innovation and carbon dioxide emissions mainly focus on the provincial and urban levels, there is limited exploration at the enterprise level. Using data from publicly listed companies from 2000 to 2022, this study examines the relationship between green innovation and enterprises’ carbon emissions, thereby supplementing research on factors influencing corporate carbon reduction and enriching studies on the environmental benefits of green innovation in the low-carbon field. 2) The study expands the research on the mechanisms through which green innovation influences carbon emissions. Existing research often suggests that green innovation impacts carbon emissions through channels such as the digital economy (Zhao et al., 2024), industrial structure effects, urbanization effects, and foreign direct investment (Xu et al., 2021) at the regional and city levels. However, little attention has been given to mechanisms such as energy use efficiency and management specialization at the enterprise level. This paper explores the mechanisms of energy efficiency and management specialization in depth, contributing to a better understanding of the intrinsic link between green innovation and corporate carbon emissions. 3) The study enriches the research on the relationship between green innovation and carbon emissions. This study examines the heterogeneous effects of green innovation on carbon emissions in enterprises across four dimensions: types of green innovation, enterprise characteristics, the pollution intensity of the industry, and the technological intensity of the industry. This analysis not only supplements existing literature on green innovation and carbon emissions but also provides theoretical support for China’s “dual carbon” goals, offering significant insights for sustainable economic and social development in developing countries.
Enterprise green innovation is primarily reflected in two dimensions: green technological innovation and green managerial innovation. Green technological innovation can drive the development of new digital industries, further optimize industrial structures, (Guo et al., 2019) and reduce enterprises’ reliance on high-carbon-intensive operations, fundamentally altering carbon emissions. With continuous technological advancements, such as the adoption of clean technologies, enterprises can lower the cost of clean energy, decrease energy intensity during production, and ultimately reduce carbon emissions in the production process. Green managerial innovation, on the other hand, enables enterprises to adopt advanced energy management systems, increase the proportion of renewable energy in production planning, optimize resource utilization, and minimize raw material wastage, thereby reducing carbon emissions. Furthermore, enterprises enhance green supply chain management by implementing green procurement policies, setting clear environmental standards for supplier materials (Niu and Liu, 2021), and prioritizing suppliers with strong environmental performance. These measures incentivize suppliers to adopt stricter carbon emission management practices, further lowering overall emissions. Based on this, the following hypothesis is proposed:
H1. Green innovation significantly reduces carbon emissions.
When enterprises engage in green innovation, two primary approaches in the technological domain are observed. The first involves upgrading and modernizing existing technologies. For instance, enterprises focus on updating current equipment through continuous collaboration with technology suppliers, ensuring ongoing advancements in environmental technologies. Green technological innovation also facilitates the restructuring and optimization of industrial sectors, promoting the transformation of energy-intensive industries. For example, introducing advanced energy-saving technologies and equipment enables sectors such as steel and chemicals to significantly reduce energy consumption per unit of output, achieving a green transition. By consistently upgrading existing clean energy technologies, enterprises can effectively decrease their reliance on fossil fuels and enhance overall energy efficiency (Akther et al., 2024). Additionally, in the realm of production processes, enterprises leverage digital technologies—such as the industrial internet and artificial intelligence—to improve production design processes, retrofit existing equipment, optimize facility management, and achieve intelligent production. These advancements reduce marginal costs and further enhance energy efficiency.
The second approach involves adopting new technologies. This is exemplified by introducing cutting-edge energy-efficient equipment and employing the latest energy-saving technologies to reduce energy consumption. The integration of digital and intelligent technologies is also promoted (Gao et al., 2024). By utilizing digital technologies such as the Internet of Things (IoT), big data, and cloud computing, enterprises can monitor and control energy usage in real-time. Data analytics and intelligent algorithms optimize energy allocation (Hu J. et al., 2024; Hu S. et al., 2024), while advanced AI algorithms predict energy demand, enabling dynamic adjustments to energy supply strategies and minimizing unnecessary waste. Moreover, enterprises actively participate in the research and development of clean energy technologies, such as hydrogen and biomass energy, to explore diversified clean energy solutions. The development of efficient energy conversion technologies, including advanced internal combustion engines, high-efficiency motors, and inverters, also significantly reduces energy losses during conversion processes.
In general, industrial carbon emissions primarily originate from the combustion of fossil fuels (Sun and Onuh, 2024), which are used either as energy sources or as raw materials in production processes. Clearly, improving energy utilization efficiency can reduce carbon emissions at the source (Liu et al., 2022b). Based on this, the following hypothesis is proposed:
H2. Green innovation reduces enterprise carbon emissions by enhancing energy efficiency.
Enterprises engaged in green innovation typically develop systematic strategic plans that define clear goals, steps, and divisions of responsibility. Such well-defined planning enhances internal control mechanisms, facilitating more effective managerial coordination (Wang et al., 2023). During this process, enterprises perform accurate assessments of their resources, gaining a clearer understanding of their green innovation directions. This enables the adoption of targeted management measures to achieve strategic objectives. Additionally, green management innovation effectively mitigates environmental risks. By optimizing processes, enterprises improve their resilience to risks, enabling more efficient and timely responses to policy changes and market demands, thereby increasing the flexibility and precision of managerial specialization. Moreover, when implementing green innovation, enterprises enhance execution methods and performance standards, further professionalizing managerial practices. Green innovation also extends beyond internal operations to encompass external supply chain networks. Green initiatives along the supply chain are often achieved through collaboration among multiple enterprises or departments. To ensure these initiatives are realized, comprehensive inter-organizational cooperation is fostered, providing organizational support for activities. The coordination, task allocation, progress monitoring, and performance evaluation among different enterprises or departments compel enterprises to improve managerial specialization.
As managerial specialization advances, production strategies are adjusted to prioritize environmental impacts over purely economic objectives, promoting low-carbon production practices. Enhanced managerial specialization also leads to more stringent employee selection and training criteria, incorporating dimensions such as green experience and awareness. Enterprises allocate more budgets to employee incentives, fostering creativity and motivation for green initiatives and deepening efforts in low-carbon and emission reduction practices. Employees selected under such criteria are more committed to sustainable development, proactively adopting green technologies to reduce carbon emissions and support long-term sustainability goals. Implementing green supply chain management enhances the environmental performance of the entire production network, satisfying consumer demands for green products across diverse economies and complying with environmental regulations in various export markets. This optimizes supply chain structures, reduces carbon emissions across the supply chain, and fundamentally improves carbon emission performance. Based on this, the following hypothesis is proposed:
H3. Green innovation reduces enterprise carbon emissions by enhancing the professionalism of enterprise management.
The relationships among the three hypotheses proposed in this study are shown in Figure 1.
Considering data availability, this study examines A-share listed enterprises from 2000 to 2022. The data sources for this study are as follows:
First, Green innovation data from 2000 to 2022 year: this data originates from the State Intellectual Property Office (SIPO) of the People’s Republic of China. In 2010, the World Intellectual Property Organization (WIPO) introduced the International Patent Classification Green Inventory, an online tool to facilitate the search of environmentally friendly technology patents. Based on the United Nations Framework Convention on Climate Change, this classification divides green patents into seven main categories. Following this classification, we calculate the annual number of green patents for each enterprise. Second, Carbon emission data from 2000 to 2022 year: the annual carbon emissions data are calculated based on enterprises’ disclosures in social responsibility reports, sustainability reports, environmental reports, and the “Guidelines for Enterprise Greenhouse Gas Emissions Accounting and Reporting.” Third, Enterprise characteristic variable from 2000 to 2022 year: these variables are sourced from the China Stock Market and Accounting Research (CSMAR) database. Given data availability, this study selects A-share listed companies on the Shanghai and Shenzhen stock exchanges from 2000 to 2022 as the research sample. To ensure the reliability of the sample data and the accuracy of the regression results, the following procedures are implemented:
(1) Companies in the financial and insurance sectors are removed, as these enterprises do not operate within the real economy and have unique business models.
(2) ST, *ST, and SST companies are excluded because they are publicly listed enterprises with abnormal operational conditions.
(3) Companies with significant data gaps are also removed.
The dependent variable in this study is the enterprises’ carbon emissions. Drawing on methodologies from Bolton and Kacperczyk (2021) and Wang Y. et al. (2023), this research measures enterprises’ carbon emissions using data on annual direct, indirect, or total carbon emissions disclosed by publicly listed companies. For enterprises that do not directly disclose these data, carbon emissions are calculated according to the “Guidelines for Corporate Greenhouse Gas Emissions Accounting and Reporting.” Direct, indirect, and total carbon emissions are estimated based on company fossil fuel consumption, electricity use, and heat consumption. This study focuses on carbon emissions generated during the production process and utilizes the natural logarithm of total emissions minus emissions from land-use changes to assess overall carbon emissions (Bolton and Kacperczyk, 2021).
The independent variable in this study is green innovation, encompassing innovations in areas such as technology and production. This paper primarily focuses on green technological innovation. Following Qi et al. (2018), the level of green innovation in enterprises is measured using the ratio of the total number of green patents to the total number of independent patent applications filed within the same year.
Existing research has identified multiple factors influencing enterprises’ carbon emissions. To control for the effects of other variables, several critical control variables related to governance structure and financial conditions are incorporated into the model:
(1) Enterprise Size (SIZE): Measured by the natural logarithm of the total assets at the end of the year.
(2) Total Asset Growth Rate (TAGR): Calculated as the ratio of the difference between the total assets of the current year and the previous year to the total assets of the previous year.
(3) Cash Flow (FC): Represented by the ratio of net cash flow from operating activities to total assets at the end of the year.
(4) Board Size (BOD): Determined by the natural logarithm of the total number of board members.
(5) Return on Assets (ROA): Defined as the ratio of net profit to shareholders’ equity.
(6) Intangible Asset Ratio (ITANG): Measured as the ratio of net intangible assets to total assets.
(7) Management Fee Rate (MF): Calculated as the ratio of management expenses to main business income.
(8) Financial Leverage (FL): Measured by the ratio of total liabilities to total assets.
Descriptive statistics for the study data are presented in Table 1.
To investigate the impact of green innovation on enterprise carbon emissions, this study constructs the following econometric model for empirical testing:
In Equation 1, f and t represent the enterprise and year, respectively. The dependent variable, CEft, indicates the carbon emissions level of enterprise f in year t. The explanatory variable, GIft, reflects the overall internal control status of enterprise f in year t. Z denotes a set of control variables, φt represents the year fixed effects, θf denotes the enterprise fixed effects, and εft refers to the random error term.
Table 2 presents the baseline regression results. Column (1) shows the regression outcome of GI and CE without any control variables, while controlling for year and enterprise fixed effects. Column (2) incorporates a series of control variables on this basis. The empirical results indicate that, whether or not control variables are included, the estimated coefficient of the explanatory variable GI is significantly negative at the 1% level. This finding demonstrates that green innovation by enterprises can significantly reduce carbon emissions among Chinese enterprises.
To verify the stability of the empirical results and enhance their credibility, four robustness tests are conducted in this study: substituting explanatory variables, controlling for industry fixed effects, clustering robust standard errors, and applying a 1% bilateral winsorization.
To eliminate the interference of measurement errors in green innovation on regression results, this study adopts the approach of Wang and Wang (2021) to re-measure green innovation, setting it as the variable GI_new. Green innovation is measured by taking the natural logarithm of the sum of an enterprise’s independent green invention applications and independent green utility model applications for the year, adding one. Column (1) in Table 3 presents the corresponding estimation results, indicating that the coefficient of GI is significantly negative at the 1% level. This finding suggests that, even after replacing the explanatory variable, the regression results remain robust.
To eliminate the potential interference of carbon emission measurement errors on the regression results, this study adopts carbon emission intensity as an alternative measure of enterprise carbon emissions, denoted as the variable CE_new. Carbon emission intensity is calculated as the ratio of an enterprise’s annual carbon emissions to the GDP of the same year. The corresponding estimation results are presented in column (2) of Table 3. It is observed that the coefficient of GI on CE_new remains significantly negative, indicating that the regression results remain robust after replacing the dependent variable.
Considering the potential industry specificity of green innovation, this section controls for industry-fixed effects to examine any changes in the empirical results. As shown in Column (3) of Table 3, controlling for industry-fixed effects indicates that increasing green innovation still has a negative impact on reducing enterprises’ carbon emissions, remaining statistically significant at the 1% level. These findings suggest that, even after accounting for industry effects, enhancing green innovation continues to contribute to lowering enterprise carbon emissions. The empirical results are consistent with the mechanism and analysis presented earlier.
To further address potential correlations within the empirical sample and resolve heteroscedasticity issues, clustered robust standard errors are employed to enhance the credibility and authority of the empirical results. The findings are presented in column (4) of Table 3. Clearly, green innovation continues to significantly reduce enterprise carbon emissions, with statistical significance at the 1% level. The empirical results indicate that while heteroscedasticity cannot be entirely eliminated, the use of clustered standard errors confirms a significant negative impact of green innovation on enterprise carbon emissions. These findings are consistent with the mechanisms and empirical results discussed earlier, demonstrating the reliability and validity of the previous analysis.
To further exclude the possibility of correlation within the empirical sample and address heteroskedasticity, cluster-robust standard errors are applied, enhancing the credibility and authority of the empirical results. These results are displayed in column (5) of Table 3. Evidently, green innovation continues to contribute to reducing enterprise carbon emissions, with statistical significance at the 1% level. The empirical findings indicate that while heteroskedasticity cannot be entirely eliminated, a significant negative impact of green innovation on enterprise carbon emissions is observed when cluster standard errors are used. This empirical conclusion aligns with the theoretical mechanism and prior empirical results, reinforcing the reliability and authenticity of previous findings.
To ensure the accuracy of baseline regression, it is essential to address potential endogeneity issues in the econometric model, primarily arising from reverse causality and omitted variables. This paper employs the number of green trade agreements signed by enterprises’ export trade partner countries (Number) as an instrumental variable for green innovation, estimating the relationship using two-stage least squares. Given that the provisions included in international green trade agreements explicitly define environmental standards for various traded products, these agreements influence the types and quantities of green products exported by economies, thereby affecting the level of green innovation within enterprises located in those economies. This fully aligns with the relevance assumption of instrumental variables. Moreover, green trade agreements, signed by different economies to establish, maintain, and develop economic and trade relations while pursuing green benefits, are outcomes of political and economic equilibrium among nations. This characteristic satisfies the exclusivity requirement of instrumental variables. This study uses data from the WTO RTAIS (Regional Trade Agreements Information System) database, collating and processing the number of trade agreements that contain green clauses. As the number of green trade agreements is measured at the national level, this study also weights this variable by the trade volume of each enterprise’s exports to different economies, matching it to the enterprise level.
Table 4 reports the results of two-stage estimations. Column (1) presents the first-stage regression outcomes, where the estimated coefficients of the instrumental variables are all significantly negative at the 1% level. Given that the number of instrumental variables does not exceed the number of explanatory variables and the F-statistic values of the instrumental variables are well above the critical value at the 10% significance level in the Stock-Yogo test, weak correlation issues are excluded. Column (2) provides the second-stage regression results, showing that the sign and significance of the estimated coefficient for GI remain consistent with those in the baseline regression, further validating the findings of this study.
Green innovation includes applications for both green invention patents and green utility patents, each differing in technological innovation level and scope of protection, which may affect enterprises’ carbon emissions reduction in varying ways. Compared to green utility patents, green invention patents generally involve higher levels of technological R&D, a more complex review process, and a broader scope of protection, encompassing various aspects such as manufacturing methods, usage methods, and product structure (Li and Zheng, 2016). Based on this, we hypothesize that applying for green invention patents is more effective in reducing enterprises’ carbon emissions than applying for green utility patents.
This study explores the heterogeneous effects of different types of green innovation on reducing enterprises’ carbon emissions by distinguishing between applications for green invention patents and green utility patents. The quantity of green invention patent applications (INVA) and green utility patent applications (UMA) are used to measure the respective application activity for these types of patents. As shown in Table 5, Columns (1) and (2) report the effects of green invention patents and green utility patents on enterprises’ carbon emissions. Notably, the estimated coefficient for green invention patents is higher than that for green utility patents, supporting our hypothesis.
Given the variations in internal control strength among enterprises with different characteristics (Lin et al., 2024), enterprises may exhibit distinct responses when engaging in green innovation. Compared to non-state-owned enterprises (Non SOEs), state-owned enterprises (SOEs) benefit from stronger administrative protections, operate in more stable market positions, and face less competitive pressure. This, to some extent, reduces their inclination toward green innovation, as the implementation of policies is often limited by management structures and vested interests, resulting in outcomes that fall short of expectations. Consequently, we hypothesize that non-state-owned enterprises achieve greater carbon emissions reductions through enhanced green innovation than their state-owned counterparts.
In this study, following Hsieh and Song (2015), we construct a binary variable for enterprise ownership, defining an enterprise as state-owned if its state capital constitutes 50% or more of its paid-in capital, assigned a value of 1; otherwise, it is categorized as non-state-owned with a value of 0. Columns (1) and (2) of Table 6 report the impact of green innovation GI on CE, showing that the estimated coefficient for non-state-owned enterprises is higher than that for state-owned enterprises, thereby validating the above hypothesis.
Enterprises in industries with varying pollution levels may experience heterogeneity in the impact of green innovation on carbon emissions due to differences in pollution emission structures and policy support across industries. Enterprises in high-pollution industries typically engage in daily production activities associated with significant energy consumption and pollutant emissions, often resulting in higher levels of carbon output. Additionally, the government generally places stricter environmental policies and emission standards on high-pollution industries, incentivizing these enterprises to pursue green innovation more actively. Thus, we propose that green innovation is more effective in reducing carbon emissions for enterprises in high-pollution industries than for those in low-pollution sectors.
This study examines the heterogeneity in the impact of green innovation on enterprise carbon emissions by distinguishing the pollution levels of their respective industries. The dummy variable, POLLUTION, is used to measure pollution levels, with the industry median as the cutoff. Industries with pollution levels above the median are classified as high-pollution (POLLUTION = 1), while those below the median are classified as low-pollution (POLLUTION = 0). Results are presented in Table 7; columns (1) and (2) report the impact of GI on CE, revealing that the estimated coefficient for high-pollution industries exceeds that for low-pollution industries, thereby confirming the hypothesis outlined above.
Enterprises operating in industries with different levels of technological intensity may exhibit varying carbon reduction effects from green innovation due to differences in technological innovation capacity and resource consumption. Compared to enterprises in low-technological-intensity industries, those in high-technological-intensity industries typically possess stronger R&D capabilities and higher innovation capacity, enabling more comprehensive and advanced green innovation. Consequently, the carbon reduction effects of green innovation are more pronounced in high-technological-intensity industries. Furthermore, enterprises in high-technological-intensity industries often face substantial technological investments and higher levels of energy and resource consumption, which provide greater overall emission reduction potential. Based on this, it is hypothesized that the effect of green innovation on reducing carbon emissions is greater for enterprises in high-technological-intensity industries than for those in low-technological-intensity industries.
This study explores the heterogeneous impact of green innovation on enterprise carbon emissions by distinguishing enterprises based on their industry’s technological intensity. Following Lu and Dang (2014), a dummy variable TECH is used to measure technological intensity, calculated as the ratio of R&D expenditure to employee compensation. Industries are classified by the median technological intensity: industries above the median are categorized as high-technological-intensity (coded as 1), while those below the median are categorized as low-technological-intensity (coded as 0). The results, presented in Table 8, show that the estimated coefficients of GI on carbon emissions CE are higher for enterprises in high-technological-intensity industries than for those in low-technological-intensity industries. These regression results confirm the proposed hypothesis.
Empirical results demonstrate that green innovation significantly reduces carbon emissions in Chinese enterprises. Further investigation is conducted to explore the specific channels through which green innovation impacts carbon emissions in these enterprises. Based on theoretical analysis, energy utilization efficiency and management specialization are identified as critical pathways for green innovation to influence carbon emissions in Chinese enterprises, and empirical tests are carried out to examine these effects.
This study uses the intensity of energy consumption per unit of GDP to assess energy efficiency (Energy). A higher Energy value indicates a greater energy utilization efficiency for the enterprise. Table 9 presents the results of testing this mechanism. Column (1) estimates the impact of GI on Energy, with the coefficient showing a significant positive effect at the 1% level. This finding suggests that enhancing green innovation in enterprises contributes to improved energy utilization efficiency. Column (2) displays the estimated coefficient of Energy on CE, showing a significant negative effect at the 1% level, indicating that increasing an enterprise’s RISK level can significantly reduce its carbon emissions. Therefore, it can be inferred that strengthening green innovation in enterprises can reduce carbon emissions by improving energy utilization efficiency.
Based on the matching data from the WIOD and labor occupational databases, as referenced by Zhong et al. (2021), the forward decomposition method of trade value-added is applied to measure industry-specific management specialization levels through industry factor returns. Following the approach of Chor et al. (2021), management specialization levels for enterprises (Manage) are calculated using a weighted method, where higher values indicate stronger and more effective management. Table 10 presents the results of tests on the above influence mechanism. Column (1) estimates the effect of GI on Manage, with a positive coefficient significant at the 1% level, indicating that enhancing green innovation in enterprises promotes higher management specialization. Column (2) shows a negative and significant coefficient of Manage on CE at the 1% level, suggesting that improving enterprise management specialization significantly reduces carbon emissions. Thus, it can be inferred that strengthening green innovation in enterprises can lower carbon emissions by enhancing management specialization.
Based on panel data from A-share listed enterprises in China from 2000 to 2021, this study examines the impact of green innovation on enterprise carbon emissions. It also explores the heterogeneity of these effects by green innovation type, industry pollution level, and enterprise ownership, and investigates the specific mechanisms through which green innovation affects carbon emissions. The findings reveal that:
(1) Green innovation significantly reduces enterprise carbon emissions, a conclusion that remains robust across various analyses. This reduction primarily occurs through two channels: improved energy efficiency and enhanced enterprise management specialization.
(2) Compared to applying for green utility model patents, green innovation driven by applying for green invention patents is more effective in reducing enterprise carbon emissions. When examining enterprise ownership, the carbon emission reduction effect of green innovation is found to be more pronounced in non-state-owned enterprises than in state-owned ones. Furthermore, when comparing industries based on pollution levels and technological intensity, the carbon emission suppression effect of green innovation is stronger in enterprises operating in high-pollution and high-technology-intensive industries.
This paper provides empirical evidence identifying the impact of green innovation on enterprises’ carbon emissions, offering new insights for promoting sustainable enterprise development. Based on these findings, the following recommendations are proposed:
(1) The results of this study indicate that green innovation significantly reduces enterprise carbon emissions and enhances environmental benefits. Therefore, enterprises should proactively implement green innovation in the future. Internally, enterprises are encouraged to integrate the concept of green innovation into their corporate culture, embedding green elements such as environmental protection and low carbon into their core values and business strategies. This approach should promote awareness of green innovation among all employees. When formulating development strategies, enterprises are advised to move beyond solely pursuing economic benefits and increasingly prioritize environmental performance, aiming for the synergistic development of economic and environmental benefits. Externally, enterprises can consider learning from international best practices in green innovation and enhancing their own capabilities in this field. Collaboration with trading partners on research and application development in green innovation is also recommended.
(2) Previous studies have found that, compared to applying for green utility model patents, applying for green invention patents requires higher levels of innovation as well as greater technological depth and breadth. Therefore, green innovation involving green invention patents is more effective in reducing enterprise carbon emissions. Enterprises should tailor patent application strategies based on their specific circumstances, allocating budgets to prioritize green invention patents. When applying for these patents, focus should be placed on increasing both the quantity and quality of patents by refining technical plans and recruiting experienced teams for research and development. After obtaining patents, ongoing maintenance is essential. For utility model patents, enterprises should first broaden the scope of patents across fields and then deepen their application levels within each domain.
(3) Considering that the carbon emission reduction effect of green innovation is more pronounced in non-state-owned enterprises compared to state-owned enterprises, several findings are highlighted. Green innovation exerts a stronger inhibitory effect on carbon emissions in enterprises operating in highly polluting industries. Additionally, the suppressive effect is more significant in industries characterized by high technological intensity. Non-state-owned enterprises, as well as those in high-pollution and high-tech industries, should place greater emphasis on green innovation and actively adopt green practices. This includes introducing advanced green technologies to optimize energy structures, integrating green technologies into production processes, streamlining workflows, and reducing energy consumption and emissions. Enterprises should also focus on enhancing internal management specialization by assembling teams of executives with green expertise, providing regular training to employees, and disseminating the latest knowledge and methods of green innovation. In green supply chain management, preference should be given to suppliers with green production capabilities and environmental certifications. Low-carbon and environmentally friendly logistics solutions should be adopted to minimize energy consumption and emissions during transportation.
The data analyzed in this study is subject to the following licenses/restrictions: The datasets presented in this article are not readily available because the research data will also be used for subsequent research, and it is currently inconvenient to share the data with other researchers. Requests to access these datasets should be directed to Yindan Wang, eWluZGFuX3dhbmdAMTYzLmNvbQ==.
JX: Conceptualization, Data curation, Formal Analysis, Funding acquisition, Methodology, Writing–original draft, Project administration, Resources. YW: Methodology, Software, Supervision, Validation, Visualization, Writing–review and editing.
The author(s) declare that financial support was received for the research, authorship, and/or publication of this article. This work has been supported by the Social Science Foundation of Hunan Provincial (19JD25, 23YBQ139) and the Scientific Research Project of Hunan Education Department (23B0929).
The authors declare that the research was conducted in the absence of any commercial or financial relationships that could be construed as a potential conflict of interest.
The author(s) declare that no Generative AI was used in the creation of this manuscript.
All claims expressed in this article are solely those of the authors and do not necessarily represent those of their affiliated organizations, or those of the publisher, the editors and the reviewers. Any product that may be evaluated in this article, or claim that may be made by its manufacturer, is not guaranteed or endorsed by the publisher.
Aguilera-Caracuel, J., and Ortiz-de-Mandojana, N. (2013). Green innovation and financial performance: an institutional approach. Organ. Environ. 26 (4), 365–385. doi:10.1177/1086026613507931
Akther, S., Sultanuzzaman, M. R., Zhang, Y., Almutlaq, F., and Huq, M. E. (2024). Exploring the influence of green Growth and energy sources on “carbon-dioxide emissions”: implications for climate change mitigation. Front. Environ. Sci. 12, 1443915. doi:10.3389/fenvs.2024.1443915
Arfi, W. B., Hikkerova, L., and Sahut, J. M. (2018). External knowledge sources, green innovation and performance. Technol. Forecast. Soc. change 129, 210–220. doi:10.1016/j.techfore.2017.09.017
Bolton, P., and Kacperczyk, M. (2021). Do investors care about carbon risk? J. financial Econ. 142 (2), 517–549. doi:10.1016/j.jfineco.2021.05.008
Cai, W., and Li, G. (2018). The drivers of eco-innovation and its impact on performance: evidence from China. J. Clean. Prod. 176, 110–118. doi:10.1016/j.jclepro.2017.12.109
Castellacci, F., and Lie, C. M. (2017). A taxonomy of green innovators: empirical evidence from South Korea. J. Clean. Prod. 143, 1036–1047. doi:10.1016/j.jclepro.2016.12.016
Chor, D., Manova, K., and Yu, Z. (2021). Growing like China: firm performance and global production line position. J. Internati. Econom. 130, 103445. doi:10.1016/j.jinteco.2021.103445
Che, S., Wen, L., and Wang, J. (2024). Global insights on the impact of digital infrastructure on carbon emissions: a multidimensional analysis. J. Environ. Manag. 368, 122144. doi:10.1016/j.jenvman.2024.122144
Chen, J., Guo, Z., and Lei, Z. (2024). Research on the mechanisms of the digital transformation of manufacturing enterprises for carbon emissions reduction. J. Clean. Prod. 449, 141817. doi:10.1016/j.jclepro.2024.141817
Chen, Y., and Xie, Y. (2024). Environmental liability insurance, green innovation, and mediation effect study. Front. Environ. Sci. 12, 1363199. doi:10.3389/fenvs.2024.1363199
Chen, Z., Hao, X., and Chen, F. (2022). Green innovation and enterprise reputation value. Bus. Strategy Environ. 32 (4), 1698–1718. doi:10.1002/bse.3213
De Azevedo Rezende, L., Bansi, A. C., Alves, M. F. R., and Galina, S. V. R. (2019). Take your time: examining when green innovation affects financial performance in multinationals. J. Clean. Prod. 233, 993–1003. doi:10.1016/j.jclepro.2019.06.135
Gao, S., Li, X., Duan, H., Liu, S., and Sun, X. (2024). How digital technology affects regional energy intensity from the perspective of energy economy: a case study of the Yangtze River economic belt. Front. Environ. Sci. 12, 1468512. doi:10.3389/fenvs.2024.1468512
Geng, Y. J., and Zhao, X. X. (2020). Environmental Regulation,Green innovation and enterprise performance——evidence based on listed companies with heavy pollution. Finance Res. 02, 15–24. doi:10.14115/j.cnki.10-1242/f.2020.02.002
Guo, Q., Zhou, M., Liu, N., and Wang, Y. (2019). Spatial effects of environmental regulation and green credits on green technology innovation under low-carbon economy background conditions. Int. J. Environ. Res. Public Health 16 (17), 3027. doi:10.3390/ijerph16173027
Guo, Z., and Xiong, C. (2024). Allocating inter-provincial CEA in China based on the utility perspective--a method for improving the variable weight function. Front. Environ. Sci. 12, 1408970. doi:10.3389/fenvs.2024.1408970
Han, J. X., Qin, Z., and Li, J. (2024). ESG performance and corporate carbon emission intensity: based on panel data analysis of A-share listed companies. Front. Environ. Sci. 12, 1483237. doi:10.3389/fenvs.2024.1483237
Hao, X., Chen, F., and Chen, Z. (2021). Does green innovation increase enterprise value? Bus. strategy Environ. 31 (3), 1232–1247. doi:10.1002/bse.2952
Hojnik, J., and Ruzzier, M. (2016). What drives eco-innovation? A review of an emerging literature. Environ. innovation Soc. transitions 19, 31–41. doi:10.1016/j.eist.2015.09.006
Hsieh, C. T., and Song, Z. M. (2015). Grasp the large, let go of the small: The transformation of the state sector in China. NBER. doi:10.3386/w21006
Hu, J., Sun, Q., and Wang, W. (2024). The role of green and digital technology convergence on carbon emission reduction: evidence from China. Front. Environ. Sci. 12, 1490657. doi:10.3389/fenvs.2024.1490657
Hu, S., Zhang, Y., Hu, Y., and Wang, H. (2024). Born by water: does water resource reallocation promote entrepreneurship? China Econ. Rev. 87, 102234. doi:10.1016/j.chieco.2024.102234
Jiang, H., Elahi, E., Gao, M., Huang, Y., and Liu, X. (2024). Digital economy to encourage sustainable consumption and reduce carbon emissions. J. Clean. Prod. 443, 140867. doi:10.1016/j.jclepro.2024.140867
Küçükoğlu, M. T., and Pınar, R. İ. (2015). Positive influences of green innovation on company performance. Procedia-Social Behav. Sci. 195, 1232–1237. doi:10.1016/j.sbspro.2015.06.261
Li, C., and Zhou, W. (2024). Can digital economy development contribute to urban carbon emission reduction? Empirical evidence from China. J. Environ. Manag. 357, 120680. doi:10.1016/j.jenvman.2024.120680
Li, K. J., Dong, D. D., and Han, Y. F. (2020). The analysis on the environmental performance of green innovation. An examination based on spatial spillover effect and rebound effect. China Soft Sci. (7), 112–121. doi:10.3390/ijerph17217826
Li, L., Msaad, H., Sun, H., Tan, M. X., Lu, Y., and Lau, A. K. (2020). Green innovation and business sustainability: new evidence from energy intensive industry in China. Int. J. Environ. Res. Public Health 17 (21), 7826. doi:10.3390/ijerph17217826
Li, W. J., and Zheng, M. N. (2016). Is it substantive innovation or strategic innovation? impact of macroeconomic policies on micro-enterprises' innovation. Econ. Res. J. 51 (04), 60–73.
Lin, W., Tong, X., Hu, Y., and Wang, H. (2024). The flow of industrial lifeblood: the impact of the West-to-East oil transportation project on enterprise performance of China. Energy Econ. 129, 107274. doi:10.1016/j.eneco.2023.107274
Liu, H., Han, P., Wang, D., Wang, S., and Bao, H. (2024). Decoding enterprise digital transformation: external oversight and carbon emission reduction performance. J. Environ. Manag. 359, 121039. doi:10.1016/j.jenvman.2024.121039
Liu, J., Duan, Y., and Zhong, S. (2022a). Does green innovation suppress carbon emission intensity? New evidence from China. Environ. Sci. Pollut. Res. 29 (57), 86722–86743. doi:10.1007/s11356-022-21621-z
Liu, J., Liu, L., Qian, Y., and Song, S. (2022b). The effect of artificial intelligence on carbon intensity: evidence from China's industrial sector. Socio-Economic Plan. Sci. 83, 101002. doi:10.1016/j.seps.2020.101002
Lu, T., and Dang, Y. (2014). Corporate governance and innovation: differences among industry categories. Econ. Res. J. 49 (06), 115–128.
Ma, D., and Zhu, Y. (2024). The impact of economic uncertainty on carbon emission: evidence from China. Renew. Sustain. Energy Rev. 191, 114230. doi:10.1016/j.rser.2023.114230
Martínez-Ros, E., and Kunapatarawong, R. (2019). Green innovation and knowledge: the role of size. Bus. Strategy Environ. 28 (6), 1045–1059. doi:10.1002/bse.2300
Niu, M. C., and Liu, Y. (2021). Will higher sewage charges promote enterprise innovation? with implications for the environmental protection tax in China. Stat. Res. 38 (07). doi:10.19343/j.cnki.11-1302/c.2021.07.007
Peng, B., Zheng, C., Wei, G., and Elahi, E. (2020). The cultivation mechanism of green technology innovation in manufacturing industry: from the perspective of ecological niche. J. Clean. Prod. 252, 119711. doi:10.1016/j.jclepro.2019.119711
Qi, S., Lin, S., and Cui, J. (2018). Do environmental rights trading schemes induce green innovation? Evidence from listed firms in China. Econ. Res. J. 53 (12), 129–143.
Singh, S. K., Del Giudice, M., Chierici, R., and Graziano, D. (2020). Green innovation and environmental performance: the role of green transformational leadership and green human resource management. Technol. Forecast. Soc. change 150, 119762. doi:10.1016/j.techfore.2019.119762
Sun, H., Edziah, B. K., Sun, C., and Kporsu, A. K. (2019). Institutional quality, green innovation and energy efficiency. Energy policy 135, 111002. doi:10.1016/j.enpol.2019.111002
Sun, X., and Onuh, W. O. (2024). Regional energy consumption policy practice to promote low-carbon economy. Front. Environ. Sci. 12, 1423009. doi:10.3389/fenvs.2024.1423009
Tu, Y., and Wu, W. (2021). How does green innovation improve enterprises’ competitive advantage? The role of organizational learning. Sustain. Prod. Consum. 26, 504–516. doi:10.1016/j.spc.2020.12.031
Umar, M., and Safi, A. (2023). Do green finance and innovation matter for environmental protection? A case of OECD economies. Energy Econ. 119, 106560. doi:10.1016/j.eneco.2023.106560
Vasileiou, E., Georgantzis, N., Attanasi, G., and Llerena, P. (2022). Green innovation and financial performance: a study on Italian firms. Res. Policy 51 (6), 104530. doi:10.1016/j.respol.2022.104530
Wang, H., Li, Y., Lin, W., and Wei, W. (2023). How does digital technology promote carbon emission reduction? Empirical evidence based on e-commerce pilot city policy in China. J. Environ. Manag. 325, 116524. doi:10.1016/j.jenvman.2022.116524
Wang, Q., Hu, S., and Li, R. (2024). Could information and communication technology (ICT) reduce carbon emissions? The role of trade openness and financial development. Telecommun. Policy 48 (3), 102699. doi:10.1016/j.telpol.2023.102699
Wang, S. K., and Fan, W. C. (2024). Financial accessibility and carbon emissions:macro and micro evidence from financial geographical structure. J. Quantitative & Technol. Econ. 1, 67–89. doi:10.13653/j.cnki.jqte.20231117.005
Wang, Y., Hou, J., and Wang, Z. (2023). Digital trade rules and the position of Chinese enterprises in the global value chain. China Finance Econ. Rev. 12 (3), 106–128. doi:10.1515/cfer-2023-0018
Wang, X., and Wang, Y. (2021). Research on the green innovation promoted by green credit policies. J. Manag. World. 37 (06), 173–188+11. doi:10.19744/j.cnki.11-1235/f.2021.0085
Wu, J., Xia, Q., and Li, Z. (2022). Green innovation and enterprise green total factor productivity at a micro level: a perspective of technical distance. J. Clean. Prod. 344, 131070. doi:10.1016/j.jclepro.2022.131070
Wu, J., Yang, C., and Chen, L. (2024). Examining the non-linear effects of monetary policy on carbon emissions. Energy Econ. 131, 107206. doi:10.1016/j.eneco.2023.107206
Wurlod, J. D., and Noailly, J. (2018). The impact of green innovation on energy intensity: an empirical analysis for 14 industrial sectors in OECD countries. Energy Econ. 71, 47–61. doi:10.1016/j.eneco.2017.12.012
Xia, W. L., Chen, X. F., Li, Q., and Chen, X. (2020). Green technology innovation, media environmental supervision and corporate performance: empirical data from heavily polluting industries. Account. Newsl. 16, 38–42. doi:10.16144/j.cnki.issn1002-8072.2020.16.006
Xu, L., Fan, M., Yang, L., and Shao, S. (2021). Heterogeneous green innovations and carbon emission performance: evidence at China's city level. Energy Econ. 99, 105269. doi:10.1016/j.eneco.2021.105269
Yang, J., Liu, Q. H., and Shi, J. J. (2015). Research on the value of corporate green innovation strategy. Res. Manag. 36 (1), 18–25. doi:10.19571/j.cnki.1000-2995.2015.01.003
Yu, H., Shen, S., Han, L., and Ouyang, J. (2024). Spatiotemporal heterogeneities in the impact of the digital economy on carbon emission transfers in China. Technol. Forecast. Soc. Change 200, 123166. doi:10.1016/j.techfore.2023.123166
Zhan, K. Y., and Pu, Z. N. (2024). Does digital technology promote carbon emission reduction in the service industry: economic logic and empirical evidence from China. Heliyon 10 (4), e25686. doi:10.1016/j.heliyon.2024.e25686
Zhang, C., Fang, J., Ge, S., and Sun, G. (2024). Research on the impact of enterprise digital transformation on carbon emissions in the manufacturing industry. Int. Rev. Econ. & Finance 92, 211–227. doi:10.1016/j.iref.2024.02.009
Zhang, D., Rong, Z., and Ji, Q. (2019). Green innovation and firm performance: evidence from listed companies in China. Conservation Recycl. 144, 48–55. doi:10.1016/j.resconrec.2019.01.023
Zhang, Y., Xing, C., and Wang, Y. (2020). Does green innovation mitigate financing constraints? Evidence from China’s private enterprises. J. Clean. Prod. 264, 121698. doi:10.1016/j.jclepro.2020.121698
Zheng, R., Wu, G., Cheng, Y., Liu, H., Wang, Y., and Wang, X. (2023). How does digitalization drive carbon emissions? The inverted U-shaped effect in China. Environ. Impact Assess. Rev. 102, 107203. doi:10.1016/j.eiar.2023.107203
Zhao, Z., Zhao, Y., Shi, X., Zheng, L., Fan, S., Zuo, S., et al. (2024). Green innovation and carbon emission performance: The role of digital economy. Energy. Policy. 195, 114344. doi:10.1016/j.enpol.2024.114344
Keywords: green innovation, carbon emission, energy efficiency, specialization of enterprise management, sustainable development
Citation: Xie J and Wang Y (2024) Does enterprise green innovation contribute to the carbon emission reduction? Evidence from China. Front. Environ. Sci. 12:1519258. doi: 10.3389/fenvs.2024.1519258
Received: 30 October 2024; Accepted: 25 November 2024;
Published: 11 December 2024.
Edited by:
Hui Wang, Hunan University, ChinaReviewed by:
Bei Liu, Nanjing University of Posts and Telecommunications, ChinaCopyright © 2024 Xie and Wang. This is an open-access article distributed under the terms of the Creative Commons Attribution License (CC BY). The use, distribution or reproduction in other forums is permitted, provided the original author(s) and the copyright owner(s) are credited and that the original publication in this journal is cited, in accordance with accepted academic practice. No use, distribution or reproduction is permitted which does not comply with these terms.
*Correspondence: Yindan Wang, eWluZGFuX3dhbmdAMTYzLmNvbQ==
Disclaimer: All claims expressed in this article are solely those of the authors and do not necessarily represent those of their affiliated organizations, or those of the publisher, the editors and the reviewers. Any product that may be evaluated in this article or claim that may be made by its manufacturer is not guaranteed or endorsed by the publisher.
Research integrity at Frontiers
Learn more about the work of our research integrity team to safeguard the quality of each article we publish.