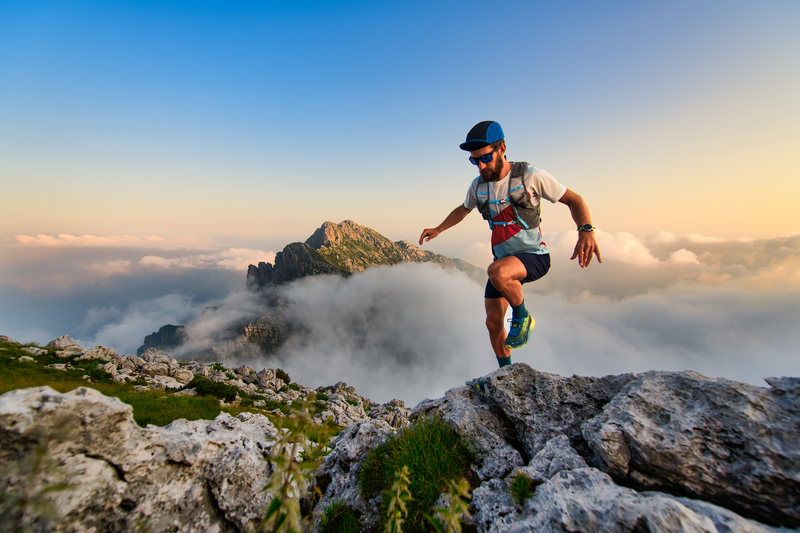
95% of researchers rate our articles as excellent or good
Learn more about the work of our research integrity team to safeguard the quality of each article we publish.
Find out more
ORIGINAL RESEARCH article
Front. Environ. Sci. , 26 August 2022
Sec. Environmental Economics and Management
Volume 10 - 2022 | https://doi.org/10.3389/fenvs.2022.969454
This article is part of the Research Topic Technology-Enabled Circular Economy Practices in Energy Production and Consumption Patterns View all 23 articles
The “Great Protection, Great Governance” strategy requires efficient environmental protection in the Yellow River Basin (YRB), enhancement of transformation between the old and new momentums, and high-quality development (HQD) of the region. This research explores the impact of the new momentum for data and government investment in technology on the HQD level of 62 cities in the YRB. The data used in this paper are collected from 2008 to 2020. We construct the index system and apply the analytic hierarchy process and entropy value method to calculate the HQD level of 62 cities in the YRB. We also construct the spatial Durbin model to investigate the influence mechanism of the above factors on the HQD of cities in the YRB and their effects from the perspectives of the new momentum for data and government investment in science and technology. According to the research results, the level of high-quality urban development of the YRB exhibits a stable increase in time series and an overall spatial distribution pattern of “higher in the east-west and lower in the middle” and “higher in the south and lower in the north”. The new momentum for data and government investment in technology significantly contribute to the HQD of cities in the YRB, with significant spatial spillover effects. The new momentum for data and government investment in technology have a positive impact on the HQD of a city and the neighboring cities. Meanwhile, the level of human capital, government intervention, scientific and technological (S&T) innovation, and openness contribute significantly to high-quality urban development. The government and policymakers should focus on the prominent role of new momentum for data and government investment in technology and design long-term feasible strategies to improve the high-quality urban development in a new stage.
Xi (2019) General Secretary of the Chinese Communist Party, pointed out that “Protection is also important when it comes to the governance of the Yellow River”. In January 2020, General Secretary Xi Jinping re-emphasized at the sixth meeting of the Central Financial and Economic Affairs Commission that great protection and governance should be carried out in the YRB and that HQD should be promoted in the central cities and megalopolises in the YRB. To achieve this goal, we must rely on innovation-driven organic growth, that is, to generate new development momentum with S&T innovation. Under the guidance of improving the transformation between the old and new momentums, the Fourth Plenary Session of the 19th Central Committee of CPC included data into the category of factors of production and clarified the importance of data for high-quality urban development. In the meantime, governments in the YRB continue their in-depth implementation of the innovation-driven development strategy, increase their investments in science and technology, and promote high-quality urban development and industrial transformation and upgrading. However, the governments fell short of innovation investment and efficiency. The 14th “Five-Year Plan” is a crucial stage to promote the ecological protection and HQD of the YRB, and it is both a theoretical and practical problem to be solved to highlight the important roles of the new momentum for data and government investment in technology for the high-quality urban development of the YRB. Therefore, accurately measuring the level of high-quality urban development and exploring the impact of new momentum for data and government investment in technology on high-quality urban development can provide not only a reference for the government and policymakers but also an important theoretical basis for the HQD of the YRB during the 14th Five Year Plan period. The existing studies are reviewed from three aspects: measurements of HQD and influencing factors, the relationship between new momentum for data and HQD, and the relationship between government investment in technology and HQD.
Accurately measuring the level of HQD and exploring the key factors affecting HQD have become the key to HQD research, and many scholars have conducted in-depth studies. Li et al. (2019) first analyze the statistical connotation of HQD and then construct an evaluation index system for HQD based on the social conflict between “people’s demands for a better life” and “the unbalanced and incomplete development”. Chen J. H. et al. (2020) construct a composite assessment indicator system for economic quality development based on five dimensions: innovation, harmonization, green, openness, and cooperation. Chen et al. (2022) and Yang and Zhang (2021) also construct an evaluation index system for high-quality economic development based on the “five development concepts” and conduct related research. Qu and Liu (2021) study the influence of environmental decentralization on high-quality economic development based on the evaluation index system for HQD they construct. Sun et al. (2022) explore the impact of industrial structure upgrading and environmental stewardship of HQD. Sarfraz et al. (2021) believe that the reduction of carbon emissions contributes to sustainable development. Zheng and Du (2020) study the effect of how human capital promotes the development of megalopolises. On this basis, the academic community explores the relationship between the new momentum for data and HQD and produces representative results: Jones and Tonetti (2020) explore how different models of data ownership affect economic growth rates; Grigorescu et al. (2021) argue that the digital economy will ultimately increase demographic well-being; Khan et al. (2022b) believe that Industry 4.0 technologies enhance circular economy practices in the current age of digitization. Many Chinese scholars are more inclined to theoretical research from a macroscopic perspective, and a few scholars have begun to argue for the realization of data through empirical analyses. Yu and Zhou (2021) explore the influence mechanism of the digital economy on the economic HQD in Henan Province in the context of “dual circulation”. Cao (2018) argues that the convergence of the digital economy and industry blazes a new path for high-quality economic development. Ding (2020) establishes a theoretical and analytical framework for high-quality economic development, which is driven by the digital economy. Zhang et al. (2021) explore the transmission mechanism and impact effects of the digital economy on the HQD of the Chinese economy. Li (2019) analyzes the theoretical mechanism of big data to promote HQD from a “macro-meso-micro” perspective.
At the same time, many scholars research the role of government investment in technology in high-quality urban development. Related research mainly provides two views on the relationship between government investment in technology and HQD. One is that government investment in technology can promote high-quality economic development. Ram (1986) and Aghion (2004) argue that a larger scale of government investment in science and technology may be a powerful driver of economic development. Niu et al. (2020) study the promotion effect of R&D investment on the HQD of megalopolises. Liu and Xia (2018) conclude that increasing R&D investment can promote stable economic growth. Boeing et al. (2022) argue that R&D subsidies not only affect business innovation but also promote economic growth and industrial structure upgrading.
The other view is that government investment in technology has a negative effect on economic development. Landau (1985) suggests that higher government expenditure may slacken economic growth. Roy (2009) explores the effect of government scale on economic growth in the United States and concludes that government spending has a notable reverse effect on economic growth.
The research results on HQD at home and abroad are fruitful and provide important guidance for this study, but there are still shortcomings in the existing research. Firstly, most of the academic research on high-quality urban development in the YRB is done at the provincial level, and fewer scholars have done such research on a specific city. Secondly, the current research doesn’t talk much about the spatial spillover effects of HQD of cities in the YRB. Thirdly, the index system of measuring the level of HQD of cities in the YRB is not uniform, which makes the empirical research less scientific. Hence, we plan to include the new momentum for data and government investment in technology in the study of the HQD of cities in the YRB, on which basis we identify critical elements influencing the HQD of cities in the YRB and explore new ways for HQD. This study aims to measure the level of HDQ in cities of YRB and explore the impact of new momentum for data and government investment in technology on the HQD level of 62 cities in the YRB. The potential marginal contributions of this paper are as follows: 1) constructing an index system to measure the level of HQD of the YRB from five dimensions—innovation, coordination, green, openness, and sharing—will enrich and improve the connotation of HQD; 2) constructing the spatial Durbin model and conducting empirical analyses on the impact of the new momentum for data and government investment in technology on the level of HQD of cities in the YRB is conducive to the study of regional economic integration; 3) the research results will provide a reference for governments to formulate policies and optimize paths for HQD in the YRB in a new stage.
General Secretary Xi Jinping has pointed out that “in the era of Internet economy, data is a new factor of production, a fundamental and strategic resource, and an important productivity”. The Fourth Plenary Session of the 19th Central Committee of CPC set out the requirement of “improving the mechanism of evaluating the contribution of labor, capital, land, knowledge, technology, management, data and other factors of production by the market and determining the remuneration according to the contribution”. As data is taken as a new momentum to drive high-quality urban development in the new period, local governments have also made it an important task to use the new momentum for data to promote HQD. In essence, data, as a new key factor in the new wave of technological revolution and industrial change, plays an active and positive role in promoting efficiency, supporting growth, and meeting demand in the process of high-quality urban development. For innovation development, the new momentum for data is reflected in the new quality industry formed by big data as a new factor, as well as the innovation of related industries and the upgrading of traditional industries attained through industrial association. On the other hand, the new momentum for data promotes cyclical technological innovation and urban innovation by way of increasing productivity (Griffith et al., 2004; Tranos et al., 2021). For coordinated development, the new momentum for data plays a crucial role in using valuable information carried by data to improve the synergy between labor, capital, and other factors, which is the most typical mechanism of action for data to improve operational efficiency at a micro-level. By reducing market failures arising from information asymmetry, the new momentum for data can improve the overall operational efficiency of the market, reduce financing costs in the financial market, and promote high-quality urban development (Cai and Ma, 2021). For green development, data is a non-polluting and renewable means of production, and innovation in the use of data resources can effectively eliminate excessive consumption of tangible resources, reduce environmental pollution and ecological damage, help create a green, low-carbon, and sustainable model for economic and social development, and thus promote high-quality urban development (Khan et al., 2022a; Khan et al., 2022c). For openness development, the new momentum for data serves as an essential part in promoting openness and sharing of data in governments, as well as standardizing social data collection. For sharing development, the new momentum for data creates more opportunities for disadvantaged groups to participate in modern economic activities and share the fruits of development, thus contributing to the balanced development of different regions and people and achieving common prosperity. Fully exploiting the knowledge sharing brought by data sharing, backward regions and disadvantaged groups can bring their late-mover advantages into full play without active participation in the division of labor and cooperation.
In summary, this paper proposes research hypothesis 1: new momentum for data promotes high-quality urban development.
The 18th National Congress of the CPC has clarified that “as a strategic guarantee to enhance social productivity and comprehensive national strength, sci-tech innovation must be put at the central position of overall development of the country”. The application of the innovation-driven development strategy and the increase of government investment in technology are of great importance to high-quality urban development. For innovation development, governments attach more importance to basic research, import high-level talents, and develop high-tech industries to improve a city’s infrastructure system for innovation development. At the same time, governments grant subsidies to enterprises to enhance their initiative in innovation and make full use of the positive externalities of R&D activities to boost urban innovation (Duguet, 2003; Lee, 2011; Lin and Luan, 2020). For coordinated development, government investment in technology can promote industrial technology innovation, which is a key factor in promoting coordinated industrial development and upgrading and sustainably supporting high-quality regional economic development. In the meantime, higher government investment and subsidies in science and technology can be conducive to collaborative innovation among enterprises, promote cooperation between companies and public research institutions, and facilitate coordinated urban development by establishing high-tech zones and science and technology parks (Engel et al., 2016; Ahn et al., 2020). For green development, government subsidies for enterprise R&D can stimulate enterprises’ independent research and development and promote enterprises’ transformation and upgrading, which can not only improve energy utilization efficiency and increase industrial output but also effectively manage industrial “three wastes” and boost green and high-quality urban development (Bjørner and Jensen, 2002; Mohsin et al., 2022; Sarfraz et al., 2022). For openness development, government investment in technology can encourage cities to reform and open to the outside world. It can also continuously improve the competitive advantages of cities in a new stage, strengthen the opening of sci-tech innovation resources to neighboring cities, help build a regional sci-tech innovation system, and further improve the openness of the cities. In addition, government subsidies for enterprise investment can attract foreign direct investment and further improve the total factor productivity and HQD of the cities (Tian, 2018; Spithoven and Merlevede, 2022). For sharing development, government investment in technology can enhance the efficiency of technology-oriented poverty alleviation and rural revitalization, close the rural-urban gap, and promote sharing development in urban areas.
In summary, this paper proposes research hypothesis 2: Government investment in technology promotes high-quality urban development.
According to existing literature, the current research mainly measures and evaluates HQD based on a comprehensive index evaluation system (Yang et al., 2021). Specifically, some research constructs evaluation index systems from the perspective of economic operation and social livelihood of cities (Chen and Chen, 2009; Li et al., 2020; Yang and Zhang, 2021). Some construct the systems based on the “Five Development Concepts” (Chen et al., 2022; Gan et al., 2022). Since the development quality of megalopolises is directly linked to development concepts, the index systems based on the “Five Development Concepts” have been widely recognized by academics.
Therefore, according to the new development concepts and previous studies, we believe that high-quality urban development features innovation, coordination, green, openness, and sharing. Specifically, innovation is the first driving force and important support for the HQD of cities in the YRB, as well as a key factor in the formation of a new development pattern (Yuan et al., 2021). In this paper, we select indicators to measure innovation development comprehensively in terms of patent achievements, senior talents, and innovation investment. Coordination is an endogenous feature of the HQD of cities in the YRB (Chen J. H. et al., 2020). We also select indicators such as coordination in rural and urban areas, industries, and people’s livelihoods for comprehensively measuring the coordinated development. Green is the vibrant color and necessary condition for the HQD of cities in the YRB, and adhering to the path of ecological priority and green development is an indispensable demand for implementing the new development concept and building a new development pattern in the new stage. In this paper, indicators are selected from the perspectives of energy consumption, pollution control, and people’s well-being to measure green development comprehensively. In the new development stage, cities must promote deep reform and HQD with a high level of openness. In this paper, such indicators as foreign trade, tourism freight, and foreign capital utilization are selected to measure openness development. Sharing is an inevitable requirement for the HQD of cities in the YRB. High-quality urban development can improve residents’ quality of life and benefit more people with the fruits of development (Li and Zhang, 2011). In this paper, indicators such as income, consumption, health, education, and leisure are selected to measure shared development. The index system for measuring the level of HQD of cities in the YRB consists of 41 indicators in 5 dimensions, as shown in Table 1.
The upper reaches of the YRB are from Heyuan to Guide, including parts of Qinghai, Sichuan, Gansu, Ningxia, and Inner Mongolia; the middle reaches are from Guide to Mengjin, including parts of Inner Mongolia, Shaanxi, Shanxi, and parts of Henan; the lower reaches are from Taohuayu of Zhengzhou to the Bohai Sea, including parts of Henan and Shandong. To make sure the measurement results of each city are complete, we divide Inner Mongolia into the upper reaches and Henan into the lower reaches. Since Sichuan Province has been included in the Yangtze River Economic Belt and the eastern part of Inner Mongolia has been included in the Northeast Area Revitalization Plan, they are not included in the YRB in this paper. Given data availability, we focus on studying 62 cities in the YRB, with a time span from 2008 to 2020.
The data is sourced from China City Statistical Yearbook, China Statistical Yearbook on Science and Technology, China Statistical Yearbook on High Technology Industry, China Statistical Yearbook on Environment, China Energy Statistical Yearbook, bulletins on national economic and social development in each city, and Economy Prediction System (EPS).
The weighting of indicators is important for measuring the level of HQD of cities in the YRB. Whether the weight determination method is reasonable immediately impacts the accuracy and scientificity of evaluation results. In this paper, we combine the analytic hierarchy process (AHP) and the entropy value method and use the average value of their weights as the final comprehensive weight of the index so that the two methods can complement each other in the process of obtaining the index weights. The specific calculation steps of the AHP and entropy value method refer to Sekovski et al. (2020), Xu and Wang (2017), Liu et al. (2020). Based on this, we use the comprehensive index method to calculate the HQD of cities in the YRB. The formula is as follows:
In Eq. 1,
In Eq. 2,
After calculation and collation, the evolutionary characteristics of the HQD of 62 cities in the YRB from 2008 to 2020 are shown in Figure 1. For the convenience of presentation, only the bar graphs of even years are listed.
FIGURE 1. Spatial and Temporal Distribution of HQD Index of 62 Cities in the YRB. Note: The plotted data are derived from the results measured based on Eq. 2. The spatial and temporal distributions in this paper are generated through ArcGIS 10.5. The sources of the vector diagram of the YRB came from the National Geomatics Center of China (NGCC).
As can be seen from Figure 1, the high-quality urban development level in the YRB increases dynamically during the sample period, and the average value of HQD in the YRB is 0.448. On the whole, the HQD of cities in the east, west, and north is higher than those in the middle and north.
K-means clustering method is used to classify the HQD index of 62 cities in the YRB in 2020 and study the spacial characteristics of HQD of cities in the YRB. The clustering results are shown in Table 2 below.
Based on the clustering results in Table 2, the HQD level of 62 cities can be divided into 4 categories with the order of cluster center size of 0.78, 0.7, 0.61, and 0.53. The first category includes 13 cities with the highest development level. Specifically, they are provincial capitals and cities in the lower reaches of the YRB such as Shandong and Henan, which are consistent with the evolutionary characteristics of the HQD of 62 cities in the YRB in Figure 1. The second category includes 25 cities with a high level of development. The third and fourth categories include 17 cities with an average development level and 7 cities with a poor development level, respectively. Based on the classification results, we draw the spatial variation of HQD of 62 cities in the YRB, as shown in Figure 2. Spatially, the overall distribution is “higher in the east and west and lower in the middle” and “higher in the south and lower in the north”, with the “center-periphery” feature of spreading from the provincial capital to neighboring cities.
FIGURE 2. Spatial variation of the level of HQD of 62 cities in the YRB in 2020. Note: The sources of the vector diagram of the YRB come from the NGCC.
The HQD indexes of cities in the YRB in 2008, 2012, 2016, and 2020 are colored on the map to highlight their spatial and temporal evolution characteristics (Figure 3). According to the composite index, the cities are divided into 5 levels, namely “high level”, “higher level”, “medium level”, “lower level”, and “low level”. Among them, the interval of low level is [0, 0.0999], the interval of lower level is [0.1, 0.2999], the interval of medium level is [0.3, 0.4999], the interval of higher level is [0.5, 0.6499], and the interval of high level is [0.65, 1].
FIGURE 3. (A) shows the spatial distribution of the high-quality development level of 62 cities in the Yellow River Basin in 2008; (B) shows the spatial distribution of the high-quality development level of 62 cities in the Yellow River Basin in 2012; (C) shows the spatial distribution of the high-quality development level of 62 cities in the Yellow River Basin in 2016; (D) shows the spatial distribution of the high-quality development level of 62 cities in the Yellow River Basin in 2020. Note: The sources of the vector diagram of the YRB came from the NGCC.
As shown in Figure 3, the HQD level of cities in the YRB has ameliorated significantly from 2008 to 2020. In terms of the improvement rate, the development features “lower reaches > upper reaches > middle reaches”. As shown in Figure 3A, the level of HQD of the cities in the upper reaches of the YRB is higher in 2008, and the level of HQD of the cities in the lower reaches of the YRB is higher than that in the middle reaches. Compared with 2008, there is an overall improvement in the HQD level of cities in the YRB in 2012, and the improvement is more obvious in the provincial capitals.
As shown in Figure 3C, the cities in the YRB reach the middle level of HQD in 2016. This “center-periphery” feature is especially obvious in the upper and lower reaches of the YRB, while the growth rate of the HQD level in the middle reaches slows down. Compared with 2016, 60 cities have reached a higher level of HQD in 2020, and the spatial characteristic that “cities of provincial capitals are centers, and neighboring cities are radiated” remains. From Figure 3D, we can see that cities in the lower reaches of the YRB are closely linked and have changed from the “center-periphery” radiation pattern to the “contiguous agglomeration” one.
According to the different regions, we plot a line graph of the historical HQD index for the upper, middle, and lower reaches of the YRB during the sample period, as shown in Figure 4.
FIGURE 4. Temporal evolution of the level of HQD in the upper, middle, and lower reaches of the YRB.
As can be seen from Figure 4, the level of HQD in the YRB and in the upper reaches to lower reaches during the sample period shows a steady increase in time series. In 2008, the overall HQD level of the YRB is 0.2295 and increases to 0.6706 in 2020, a cumulative increase of nearly twofold. Specifically, the trend of HQD levels in the upper, middle, and lower reaches of the YRB is basically the same as the overall trend of the basin, and the inter-regional links are relatively close. The level of HQD in the lower reaches of the YRB has grown fastest during the sample period, and the level of HQD in the lower reaches is only 0.1918 in 2008, which is at the lower level in the whole basin and has been steadily in the leading position of the basin as a whole since 2013, and the level of HQD in the lower reaches achieves 0.7179 in 2020. In terms of numerical size, the cities in the YRB in 2020 are ranked as lower reaches > upper reaches > middle reaches, with a little imbalance between cities in the middle and lower reaches.
Analyses are conducted on the spatiotemporal evolution of 62 cities in the YRB in terms of five subsystems.
From a time-series perspective, it can be found in Figure 5 that the five major subsystems show an overall upward trend during the sample observation period, each showing different time-series evolutionary characteristics.
Specifically, the innovation development level is characterized by a high time-series growth during the sample examination period, with 0.0146 in 2008 and 0.1434 in 2020 in the YRB. The average annual growth rate of innovation development level in the YRB during the sample examination period is 20.98%, ranking first in the five subsystems, and the innovation capability and the driving force for HQD in the YRB have been increasing.
In terms of coordinated development, the level of coordinated development increases from 0.0277 to 0.1195 during the sample observation period. In recent years, the Chinese government has been committed to improving the unbalanced development of regional, urban-rural, and industrial structures in its process of economic development. The rising level of coordinated development indicates that the livelihood and well-being of people in the YRB are improving comprehensively.
The green development level increases from 0.0987 to 0.1439 during the sample observation period. As we can see from the characteristics of the temporal change, the green development level grew before 2016 and then declined significantly in 2016 and 2017, which is because of the increasing energy consumption during the “13th Five-Year Plan” period. At that time, the industrial structure of the YRB was not fully upgraded, and the high energy consumption, low energy saving, and severe pollution exerted a huge impact on green development. From 2018 to 2020, the green development level in YRB increases steadily.
The level of openness development has increased from 0.0423 to 0.1590 during the sample period. Since September 2013, when China proposed the Belt and Road Initiative, it has provided new development opportunities for the YRB to open to the outside world, and the level of shared development has further improved since the year 2013. The YRB spans a wide area, and there are differences among cities in terms of opening duration, degree of opening, and implementation of opening policies. The level of openness development has improved insignificantly since 2017. In 2020, the level of openness development declined briefly, which was mainly due to the impact of the COVID-19 pandemic. In the same year, the total import and export volume of commodities and the scale of domestic tourism decreased to varying degrees, which exerted an impact on the level of openness development.
The level of shared development rises from 0.0462 to 0.1081 during the sample period. Many poor people in China live in the YRB, so effective implementation of poverty alleviation and social security is significant to the HQD of this area. However, the improvement of the level of shared development is relatively small due to the difficulty of structural adjustment.
To facilitate the analysis of the spatiotemporal evolution trends of the subsystems of HQD level of cities in the YRB, we conduct spatial visualization on the HQD level of each subsystem of cities in the YRB in 2008, 2012, 2016, and 2020 and use stacked bar graphs to display the development level values of each subsystem. The details are shown in Figures 6A–D below.
FIGURE 6. (A) shows the spatial distribution of the subsystem of HQD level of 62 cities in the YRB in 2008; (B) shows the spatial distribution of the subsystem of HQD level of 62 cities in the YRB in 2012; (C) shows the spatial distribution of the subsystem of HQD of 62 cities in the YRB in 2016; (D) shows the spatial distribution of the subsystem of HQD of 62 cities in the YRB in 2020. Note: The sources of the vector diagram of the YRB came from the NGCC.
As can be seen from Figure 6, the level of innovation development of cities in the YRB improved steadily from 2008 to 2020, and the overall development of the YRB was balanced. The innovation development trend in the YRB has obvious characteristics of spreading outward with cities of provincial capitals as the center, which is most obvious in 2020. Specifically, the innovation subsystem shows the spatial distribution characteristics of spreading around the cities of provincial capitals, with the central cities at a higher level of development, and the neighboring cities at a step-down level by distance. Comparing the spatial distribution of coordinated development in the YRB in 2020 and 2008, we know that the level of coordinated development of cities in Henan and Shandong provinces is significantly higher than that in other provinces. The characteristics of the green development level of cities in the YRB during the sample period are different from the spatial and temporal evolution characteristics of other subsystems. The figures have shown that the subsystem of green development shows a step distribution in space and a “rise—fall—rise” evolution in time. The subsystem of openness development has a spatial distribution characteristic of “high in the south and the east and low in the north and west”. The spatial and temporal distribution of the openness development level has the obvious characteristic that neighboring cities are driven to develop with cities of provincial capitals as centers, which is especially obvious in Henan Province and Gansu Province. The subsystem of shared development shows the spatial distribution characteristics of “lower reaches > upper reaches > middle reaches”. In general, the level of all HQD subsystems in the YRB has increased to different degrees during the sample period, with two major characteristics: “high in the east and the south and low in the west and the north” and “spreading outward from central cities”.
According to the literature review and theoretical analysis, new momentum for data and government investment in science and technology affect high-quality urban development (Griffith et al., 2004; Lee, 2011; Lin and Luan, 2020; Cai and Ma, 2021). However, the impact of these two factors on high-quality urban development needs to be analyzed through empirical research. Therefore, we construct the following fixed-effects model by referring to the studies of Li et al. (2021) and Wang et al. (2022):
To examine whether the impact of new momentum for data and government investment in technology on the HQD of cities in the region also causes similar or opposite changes in the HQD of neighboring cities, we construct the following spatial panel model:
In this equation,
High-Quality Urban Development (HQD): it is replaced by the HQD index of 62 cities in the YRB, which has been measured previously.
1) New Momentum for Data (NMD): new momentum for data means that data can be used as a new momentum for economic development. Data resources are essential factors of production, and new momentum for data can become an intrinsic driving force for economic growth, social change, and industrial optimization. Based on Chai and Li’s studies, this paper “replaces output with input” in the context of the digital industry and uses the logarithm of per-capita income generated by information transmission, software, and information technology services in each region as a proxy variable for new momentum for data (Chai and Li, 2020).
2) Government Investment in Technology (GI): we refer to the research method of Lee et al. (2020) to use the logarithm of internal R&D funding as a proxy variable for government investment in technology.
3) Interaction term between new momentum for data and government investment in technology (NMD*GI): it is the product of new momentum for data and internal R&D funding.
Based on the research of Zhang et al. (2021) and Qu and Liu (2021), this paper selects the following control variables that may affect high-quality urban development. Human capital (HUM) is measured as the share of R&D personnel in the total number of employees; government intervention (GOV) refers to the proportion of government intervention in local financial expenditure in local GDP; S&T innovation (IND) is measured as the number of patents granted; openness (FDI) is measured as the total amount of foreign capital utilized after logarithmization; the economic development level (PGDP) is measured as the regional GDP per capita after logarithmization.
Considering that the level of economic development is already included in the control variables, we use the adjacency matrix W to measure the spatial weight effect, that is, if region i and region j are adjacent, then
In this paper, the annual data of 62 cities in the YRB from 2008 to 2020 is taken as the sample, with a total of 806 observations. The relevant data are obtained from the China City Statistical Yearbook of previous years, bulletins on national economic and social development in each city, and EPS. Missing data of some cities are supplemented by the interpolation method. We use Stata17 to construct and test the panel fixed effects model and spatial Durbin model.
Table 3 reports the estimation results of the fixed effects of Eq. 3. It can be found that the regression coefficients of NMD are significantly positive at the 1% level regardless of whether control variables are included or not. This indicates that NMD has a significant positive impact on high-quality urban development, which verifies that hypothesis 1 is valid.
In models 1-6, the regression coefficients of GI are all significantly positive at the 1% level. This indicates that GI also has a positive effect on high-quality urban development, which verifies that hypothesis 2 is valid. The coefficients before the control variables indicate that human capital, S&T innovation, and the level of economic development also have a significant positive effect on high-quality urban development.
The global Moran’s I is used to test the spatial autocorrelation of the level of HQD of 62 cities in the YRB from 2008 to 2020, respectively. As shown in Figure 7, although there are fluctuations in the Moran’s I of the HQD level of 62 cities in the YRB from 2008 to 2020, most of them pass the 1% significance test and are basically positive, indicating that the level of high-quality urban development has the characteristics of significant positive spatial correlation, namely that high-level cities cluster together and the spatial dependence is more stable. Therefore, from an overall perspective, the spatial correlation is significant and the choice of spatial econometrics model is appropriate.
FIGURE 7. (A) shows the Moran’s of the HQD level of 62 cities in the YRB from 2008 to 2020; (B) shows the p-value and Z-value of the global Moran test of the HQD level of 62 cities in the YRB from 2008 to 2020.
To examine the degree of spatial correlation of a particular region, we draw the local Moran diagrams of the level of high-quality urban development. Due to limited space, only the results for the year 2008, 2012, 2016, and 2020 are reported.
As can be seen from Figure 8, the points corresponding to the HQD level of cities are mostly distributed in the first and third quadrants, i.e., the cities have a strong positive spatial promotion effect locally, which is consistent with the test result of the global Moran’s I. Therefore, the local spatial correlation is positively significant, and the spatial econometrics model should be selected considering the influence of spatial factors.
FIGURE 8. Local Moran diagrams of the level of high-quality urban development in 2008, 2012, 2016, and 2020. Note: the numbers 1–62 represent the 62 cities of the YRB, respectively.
As has been mentioned above, the level of high-quality urban development has a significant spatial correlation. By establishing a spatial econometrics model, we can measure the specific influence of NMD and GI on high-quality urban development. LM test and LR test are conducted, and the test results all rejected H0 at a 1% significance level. In summary, the spatial Durbin model with fixed effects is selected to explore the impact of NMD and GI on high-quality urban development.
According to the results of the SDM model in Table 4, the coefficient of NMD is positive and passes the hypothesis test at a 10% significance level, indicating that the higher a city’s NMD, the higher the city’s HQD level. The coefficient of the spatially lagged term of new momentum for data (W×NMD) is positive, and the original hypothesis is rejected at a 1% significance level, indicating that the NMD of the city has a significant spatial effect, i.e., the level of NMD of the city also has a significant contribution to the level of HQD of other cities. Hypothesis 1 is verified to be valid. The report of the 19th Party Congress points out that “China’s economy has shifted from the stage of high-speed growth to the stage of HQD”, which clearly defines the main direction of China’s current economic development. As a new key element of the new round of technological revolution and industrial change, data plays an active and positive role in promoting efficiency, supporting growth, and meeting demand in the process of high-quality urban development. In terms of innovation development, the NMD is reflected in the new quality industry formed by big data as a new element. On the other hand, it is related to the innovation of relevant industries and upgrading of traditional industries realized through industrial association. In terms of coordinated development, NMD plays the core role in using the valuable information carried by data to improve the synergy between labor, capital, and other factors and enhance the overall operational efficiency of the market. In terms of green development, data is a non-polluting and renewable means of production. Innovating the use of data resources can effectively eliminate the excessive consumption of tangible resources, reduce environmental pollution and ecological damage, and help promote the formation of a green, low-carbon, and sustainable economic and social development model. In terms of openness development, NMD plays an important role in promoting the openness and sharing of government data and the standardization of social data collection. In terms of shared development, NMD creates more opportunities for disadvantaged groups to participate in modern economic activities and share the fruits of development and for backward regions to give full play to their late-mover advantages, thus promoting the balanced development of different regions and achieving common prosperity. Fully exploiting the knowledge sharing brought by data sharing, backward regions and disadvantaged groups bring their late-mover advantages into full play without active participation in the division of labor and cooperation.
The coefficient of GI is significantly positive, indicating that the higher the GI of a city, the higher the level of HQD of this city. The coefficient of the lagged term of GI (W*GI) is positive, indicating that the GI of a city can improve the HQD of other cities. This verifies the validity of hypothesis 2. The Outline of the YRB’s Ecological Protection and HQD Plan released in 2021 emphasizes that the YRB must “enhance its support for S&T innovation”. In terms of the HQD of the YRB, studies that focus on supporting the HQD of the YRB with science and technology deserve greater attention. However, there are problems such as a low level of industrial development, the low pace of industrial transformation, and the low R&D capability of enterprises. Increasing GI can accelerate the urban infrastructure construction, effectively stimulate the vitality of market players, and promote high-quality urban development.
The coefficient of the interaction term of NMD and GI (NMD*GI) is significantly positive, which indicates that the stronger the interaction between NMD and GI of a city, the higher the level of HQD of the city. On one hand, government investment in science and technology can support the development of NMD and play a better multiplier effect on high-quality urban development. On the other hand, the development of NMD can enhance a city’s technological innovation, further promote the government’s governance ability, and improves the city’s HQD level.
As for the control variables, the coefficient of HUM is significantly positive, indicating that the level of human capital of a city has a significant positive effect on the level of HQD of the city; the coefficient of GOV is significantly positive, which indicates that government intervention positively impacts the level of HQD of the city; the coefficients of IND and FDI are also significantly positive, indicating that they positively affect the HQD of cities. Specifically, enhancing human capital can effectively guide the operation of physical capital and promote the development of productivity and labor productivity, the upgrading of industry transformation, and high-quality urban development. By continuously improving infrastructure and systems, the government promotes industrial upgrading and high-quality urban development. A high level of openness promotes the flow of commodities and factors, allowing them to fully circulate according to the laws of the market and promoting HQD through optimal allocation.
Since the spatial Durbin model explains the spatial economic correlation among cities, its parameter estimates do not directly reflect the direct effects and the real spatial spillover effects. Hence, we decompose the impact coefficients of the respective variables on the level of HQD into direct effects, indirect effects, and total effects.
In Table 5, the direct effects, indirect effects, and total effects of NMD are all significantly positive, indicating that NMD not only significantly promotes a city’s HQD but also has significant spatial spillover effects. If we ignore the interaction of spatial factors, the effect of NMD on the HQD of the city will be underestimated, which further validates the rationality of selecting the spatial econometrics model. The direct effects, indirect effects, and total effects of GI are all positive and pass the significance test, indicating that the GI of a city not only positively contributes to the level of HQD in the city but also positively impacts the level of HQD of neighboring cities. The direct effects of HUM are positive and pass the significance test, indicating that the increase in HUM of a city positively impacts the level of HQD of the city. Among the other control variables, the direct effects of GOV are positive, the indirect effects are negative, and the total effects are negative and pass the significance test, indicating that the GOV of a city positively affects the level of HQD of the city but negatively affects neighboring cities. The direct, indirect, and total effects of IND are all positive and pass the significance test, indicating that S&T innovation and economic development of a city not only positively promote the level of HQD of the city but also have significant spatial spillover effects, as well as having a significant positive impact on neighboring cities. The direct, indirect, and total effects of FDI are all positive and pass the significance test, indicating that the openness of a city not only positively contributes to the level of HQD of the city but also has significant spatial spillover effects, as well as having a significant positive impact on neighboring cities.
Based on theoretical analysis, measurement, and empirical tests, the following conclusions are drawn:
1) Overall, the level of HQD of cities in the YRB shows a steady increase in time series during the sample period. In terms of the size of the comprehensive index, cities in the lower reaches of the YRB rank among the top, and cities in the upper and middle reaches of the YRB rank low. This result is consistent with previous studies (Chen Y. et al., 2020; Chen et al., 2022; Gan et al., 2022).
2) In terms of subsystems, China’s strategies accelerate the innovation development during the sample period. Besides, coordinated development, openness development, and shared development improve steadily in time series, and green development shows fluctuations in its improvement. The ranking of the cities in terms of the degree of improvement is “innovation development > coordinated development > openness development > shared development > green development”. Regionally, the level of HQD of cities in the lower reaches is better than that of middle and upper cities. The disparity in the green development level between the middle and upper reaches is larger, and the gap between other subsystems is smaller. When comparing our results to those of previous studies (Qu and Liu, 2021; Yang and Zhang, 2021), we must point out that the ranking of subsystem development levels differs, which is because the different regions are studied and the calculation of weights in the measurement process is different.
3) From the viewpoint of the spatial regional correlation of each factor, the regression results of the spatial Durbin model show that NMD, GI, and the interaction term of NMD and GI have significant positive impacts on high-quality urban development, and the level of human capital, government intervention, openness, and S&T innovation also significantly contribute to the high-quality urban development. These findings are consistent with the research that shows GI and NMD are very important for the HQD of cities (Zhao et al., 2020; Yu and Zhou, 2021). From the perspective of the direct spatial effects, new momentum for data, government investment in technology, human capital, government intervention, S&T innovation, and openness significantly improve the HQD of a city; from the perspective of the indirect effects, new momentum for data, government investment in technology, S&T innovation, openness, and economic development level have significant positive spillover effects on the HQD of neighboring cities, while human capital and government intervention stifle the HQD of neighboring cities. This result is consistent with related studies which indicate that in the process of promoting high-quality urban development, it is important to pay attention not only to the local effects of these influences on cities but also to their spillover effects on neighboring cities (Zheng and Du, 2020; Yuan et al., 2021; Khan et al., 2022a; Khan and Cui, 2022; Mohsin et al., 2022; Sun et al., 2022).
The above findings imply that new momentum for data and government investment in technology significantly affect the level of HQD of cities in the YRB. This research has several recommendations.
First, the government should optimize the mechanism of new momentum for data to drive high-quality urban development. The driving mechanism of new momentum for data for high-quality urban development has three advantages. Firstly, as a production factor, data generates a substitution effect and plays a role in value creation at the stages of input, output, and distribution, enhancing efficient market operation. Secondly, integrating data and other factors will foster industrial integration and upgrading, boost high-efficiency innovation, and stimulate the multiplier effect of new momentum for data. Thirdly, efficient and dynamic data feedback facilitates scientific decision-making, promotes high-quality decision-making of enterprises, and improves government public governance. The government should encourage the development of common support platforms and industries for digital transformation, accelerate the development of new models and business forms such as platform economy and sharing economy, actively drive the digital transformation of traditional industries, boost the construction of new infrastructure, promote the free flow of data elements, drive the HQD of cities in the YRB, and enhance the level of HQD.
Second, the government should increase its input in science and technology. According to the previous empirical analyses, government investment in technology significantly promotes high-quality urban development. In the new period, local governments in the YRB should further increase their investment in science and technology in the three following aspects. Firstly, local governments should establish a long-term growth mechanism of government investment in technology and vigorously support the activities regarding S&T innovation in enterprises and research institutions. Secondly, local governments should improve the incentive of financial subsidies to facilitate the activities related to S&T innovation and create a virtuous cycle of environmental resources. Lastly, the government should enhance the conversion of the achievements of S&T innovation into real productivity, create a favorable environment for S&T innovation, invest more in the cultivation and training of talents for the conversion of the achievements of S&T innovation, and encourage the cooperation between industries, universities, and research institutions, thus realizing the high-quality urban development.
Third, the government should create an inclusive and liberal environment for the digital economy development. The digital economy has become a “new engine” to drive the world economic development in the era of the information revolution and will become the driving force of the world economic recovery and growth. It is also an important manifestation of a country’s comprehensive national power in the context of a new economy. The following two aspects can be taken into account to create an inclusive and liberal environment for the digital economy. On the one hand, the government should take the lead to remove the obstacles to the free flow of data elements, realize data interconnection, and deepen the application of the governmental Internet. On the other hand, the government should strengthen the regulation of the market and encourage society to value data management skills, so as to facilitate the development of the digital economy.
Fourth, more emphasis should be put on data protection. Privacy has been among the crucial concerns in the development of information technology. In the epoch of big data, if there are no reliable principles for data collection and use, then seemingly unrelated data points will be connected without the permission of users, in which case users’ personal information will be leaked. The government should formulate specific laws and regulations on personal privacy to protect citizens’ privacy, guarantee citizens’ right to know, and guide enterprises to use and deal with citizens’ information in an appropriate manner. In addition, the government should clarify the boundaries of the property rights of data resources and promulgate enforcement regulations on data sharing to remove the barriers to the implementation of data sharing.
There are some limitations in the current study, but it also provides directions for further research. First, the data used in this study is collected from 62 cities in the YRB of China, and the research results can only be extended to the YRB. Future studies can focus on the Yangtze River Delta, Beijing-Tianjin-Hebei, and Guangdong-Hong Kong-Macao Greater Bay Area in China and compare the results among urban clusters to produce more reliable results. Second, this paper uses the entropy weight method and hierarchical analysis to measure the HQD index of the YRB, and future studies can use methods such as the principal component analysis and TOPIS to do so. Third, this study uses a spatial econometric model to test the hypothesis, and future studies can take the mediating effect and threshold effect into consideration to extend the study.
The original contributions presented in the study are included in the article/supplementary material, further inquiries can be directed to the corresponding author.
Conceptualization, HJ and NY; Data curation, HJ; Formal analysis, HJ and NY; Methodology, HJ and NY; Supervision, NY; Software, HJ; Writing—original draft, HJ; Writing—review and editing, NY. All authors have read and agreed to the published version of the manuscript.
The authors declare that the research was conducted in the absence of any commercial or financial relationships that could be construed as a potential conflict of interest.
All claims expressed in this article are solely those of the authors and do not necessarily represent those of their affiliated organizations, or those of the publisher, the editors and the reviewers. Any product that may be evaluated in this article, or claim that may be made by its manufacturer, is not guaranteed or endorsed by the publisher.
Ahn, J. M., Lee, W., and Mortara, L. (2020). Do government R&D subsidies stimulate collaboration initiatives in private firms? Technol. Forecast. Soc. Change 151, 119840. doi:10.1016/j.techfore.2019.119840
Bjørner, T. B., and Jensen, H. H. (2002). Energy taxes, voluntary agreements and investment subsidies—a micro-panel analysis of the effect on Danish industrial companies’ energy demand. Resour. Energy Econ. 24 (3), 229–249. doi:10.1016/s0928-7655(01)00049-5
Boeing, P., Eberle, J., and Howell, A. (2022). The impact of China's R&D subsidies on R&D investment, technological upgrading and economic growth. Technol. Forecast. Soc. Change 174, 121212. doi:10.1016/j.techfore.2021.121212
Cai, Y., and Ma, W. (2021). How data influence high-quality development as a factor and the restriction of data flow. J. Quant. Tech. Econ. 3 (38), 64–83. doi:10.13653/j.cnki.jqte.2021.03.002
Cao, Z. Y. (2018). Research on new manufacturing mode to promote the high-quality development of China’s industry under the background of digital economy. Theor. Discuss. 2, 99–104. doi:10.16354/j.cnki.23-1013/d.2018.02.016
Chai, S., and Li, J. (2020). Monitoring research on the transformation of new and old driving force of China's economic development. Collect. Essays Finance Econ. 267 (12), 13. doi:10.13762/j.cnki.cjlc.2020.12.002
Chen, B., and Chen, G. Q. (2009). Emergy-based energy and material metabolism of the Yellow River basin. Commun. Nonlinear Sci. Numer. Simul. 14 (3), 923–934. doi:10.1016/j.cnsns.2007.05.034
Chen, J. H., Chen, Y., and Chen, M. M. (2020a). China’s high-quality economic development level, regional differences and dynamic evolution of distribution. J. Quant. Tech. Econ. 37 (12), 108–126. doi:10.13653/j.cnki.jqte.2020.12.006
Chen, Y., Zhu, M., Lu, J., Zhou, Q., and Ma, W. (2020b). Evaluation of ecological city and analysis of obstacle factors under the background of high-quality development: Taking cities in the Yellow River Basin as examples. Ecol. Indic. 118, 106771. doi:10.1016/j.ecolind.2020.106771
Chen, Y., Miao, Q., and Zhou, Q. (2022). Spatiotemporal differentiation and driving force analysis of the high-quality development of urban agglomerations along the Yellow River basin. Int. J. Environ. Res. Public Health 19 (4), 2484. doi:10.3390/ijerph19042484
Ding, Z. (2020). Research on the mechanism of digital economy driving high-quality economic development: A theoretical analysis framework. Mod. Econ. Res. 1, 85–92. doi:10.13891/j.cnki.mer.2020.01.011
Duguet, E. (2003). Are R&D subsidies a substitute or a complement to privately funded R&D? Evidence from France using propensity score methods for non-experimental data. University of Paris I Cahier de la MSE EUREQua Working Paper (2003.75).
Engel, D., Rothgang, M., and Eckl, V. (2016). Systemic aspects of R&D policy subsidies for R&D collaborations and their effects on private R&D. Industry Innovation 23 (2), 206–222. doi:10.1080/13662716.2016.1146127
Gan, W., Yao, W., and Huang, S. (2022). Evaluation of green logistics efficiency in jiangxi province based on three-stage DEA from the perspective of high-quality development. Sustainability 14 (2), 797. doi:10.3390/su14020797
Griffith, R., Redding, S., and Reenen, J. V. (2004). Mapping the two faces of R&D: Productivity growth in a panel of OECD industries. Rev. Econ. Stat. 86 (4), 883–895. doi:10.1162/0034653043125194
Grigorescu, A., Pelinescu, E., Ion, A. E., and Dutcas, M. F. (2021). Human capital in digital economy: An empirical analysis of central and eastern European countries from the European union. Sustainability 13 (4), 2020. doi:10.3390/su13042020
Jones, C. I., and Tonetti, C. (2020). Nonrivalry and the economics of data. Am. Econ. Rev. 110 (9), 2819–2858. doi:10.1257/aer.20191330
Khan, S. U., and Cui, Y. (2022). Identifying the impact factors of sustainable development efficiency: integrating environmental degradation, population density, industrial structure, GDP per capita, urbanization, and technology. Environ. Sci. Pollut. Res. Int. doi:10.1007/s11356-022-19809-4
Khan, S. A. R., Shah, A. S. A., Yu, Z., and Tanveer, M. (2022a). A systematic literature review on circular economy practices: challenges, opportunities and future trends. J. Entrepreneursh. Emerg. Econ. [Epub ahead of print]. doi:10.1108/jeee-09-2021-0349
Khan, S. A. R., Umar, M., Asadov, A., Tanveer, M., and Yu, Z. (2022b). Technological revolution and circular economy practices: A mechanism of green economy. Sustainability 14 (8), 4524. doi:10.3390/su14084524
Khan, S. A. R., Yu, Z., Umar, M., and Tanveer, M. (2022c). Green capabilities and green purchasing practices: A strategy striving towards sustainable operations. Bus. Strategy Environ. 31 (4), 1719–1729. doi:10.1002/bse.2979
Landau, D. L. (1985). Government expenditure and economic growth in the developed countries: 1952-76. Public Choice 47, 459–477.
Lee, N. R., Chung, S. H., Song, M. K., Kong, Y. H., Joo, C. U., and Kim, S. J. (2020). A comparative analysis of clinical screening test and language specific test in language delay children. Chonnam Med. J. 56 (1), 44–49. doi:10.4068/cmj.2020.56.1.44
Lee, C.-Y. (2011). The differential effects of public R&D support on firm R&D: Theory and evidence from multi-country data. Technovation 31 (5-6), 256–269. doi:10.1016/j.technovation.2011.01.006
Li, L., and Zhang, Y. (2011). The construction of evaluation index system and primary assessment of the transformation of China’s economic development pattern. China Ind. Econ. 4, 54–63. doi:10.19581/j.cnki.ciejournal.2011.04.006
Li, J., Shi, L., and Xu, A. (2019). Probe into the assessment indicator system on high-quality development. Stat. Res. 36 (1), 4–14. doi:10.19343/j.cnki.11-1302/c.2019.01.001
Li, B., Tian, C., Shi, Z., and Han, Z. (2020). Evolution and differentiation of high-quality development of marine economy: A case study from China. Complexity 2020, 1–11. doi:10.1155/2020/5624961
Li, X., Lu, Y., and Huang, R. (2021). Whether foreign direct investment can promote high-quality economic development under environmental regulation: evidence from the Yangtze River economic Belt, China. Environ. Sci. Pollut. Res. 28 (17), 21674–21683. doi:10.1007/s11356-020-12032-z
Li, H. (2019). The theoretical mechanism, practical basis and policy choice of big data to promote the high-quality development of my country’s economy. Economist 3, 52–59. doi:10.16158/j.cnki.51-1312/f.2019.03.007
Lin, B., and Luan, R. (2020). Are government subsidies effective in improving innovation efficiency? Based on the research of China's wind power industry. Sci. Total Environ. 710, 136339. doi:10.1016/j.scitotenv.2019.136339
Liu, C., and Xia, G. (2018). Research on the dynamic interrelationship among R&D investment, technological innovation, and economic growth in China. Sustainability 10 (11), 4260. doi:10.3390/su10114260
Liu, X., Zhang, W., Qu, Z., Guo, T., Sun, Y., Rabiei, M., et al. (2020). Feasibility evaluation of hydraulic fracturing in hydrate-bearing sediments based on analytic hierarchy process-entropy method (AHP-EM). J. Nat. Gas Sci. Eng. 81, 103434. doi:10.1016/j.jngse.2020.103434
Mohsin, M., Naseem, S., Sarfraz, M., Zia-Ur-Rehman, M., and Baig, S. A. (2022). Does energy use and economic growth allow for environmental sustainability? An empirical analysis of Pakistan. Environ. Sci. Pollut. Res. Int. doi:10.1007/s11356-022-19600-5
Niu, F., Yang, X., and Wang, F. (2020). Urban agglomeration formation and its spatiotemporal expansion process in China: From the perspective of industrial evolution. Chin. Geogr. Sci. 30 (3), 532–543. doi:10.1007/s11769-020-1094-3
Qu, X., and Liu, L. (2021). Impact of environmental decentralization economic development. Stat. Res. 38 (3), 16–29. doi:10.19343/j.cnki.11-1302/c.2021.03.002
Ram, R. (1986). Government size and economic growth: A new framework and some evidence from cross-section and time-series data. Am. Econ. Rev. 76 (1), 191–203.
Roy, A. G. (2009). Evidence on economic growth and government size. Appl. Econ. 41 (5), 607–614. doi:10.1080/00036840601007393
Sarfraz, M., Mohsin, M., Naseem, S., and Kumar, A. (2021). Modeling the relationship between carbon emissions and environmental sustainability during COVID-19: a new evidence from asymmetric ARDL cointegration approach. Environ. Dev. Sustain. 23 (11), 16208–16226. doi:10.1007/s10668-021-01324-0
Sarfraz, M., Mohsin, M., and Naseem, S. (2022). A blessing in disguise: new insights on the effect of COVID-19 on the carbon emission, climate change, and sustainable environment. Environ. Sci. Pollut. Res. 29 (20), 29651–29662. doi:10.1007/s11356-021-17507-1
Sekovski, I., Del Río, L., and Armaroli, C. (2020). Development of a coastal vulnerability index using analytical hierarchy process and application to Ravenna province (Italy). Ocean Coast. Manag. 183, 104982. doi:10.1016/j.ocecoaman.2019.104982
Spithoven, A., and Merlevede, B. (2022). The productivity impact of R&D and FDI spillovers: characterising regional path development. J. Technol. Transf., 1–31. doi:10.1007/s10961-022-09918-0
Sun, J., Tang, D., Kong, H., and Boamah, V. (2022). Impact of industrial structure upgrading on green total factor productivity in the Yangtze River economic Belt. Int. J. Environ. Res. Public Health 19 (6), 3718. doi:10.3390/ijerph19063718
Tian, Y. (2018). Optimal policy for attracting FDI: Investment cost subsidy versus tax rate reduction. Int. Rev. Econ. Finance 53, 151–159. doi:10.1016/j.iref.2017.10.018
Tranos, E., Kitsos, T., and Ortega-Argilés, R. (2021). Digital economy in the UK: regional productivity effects of early adoption. Reg. Stud. 55 (12), 1924–1938. doi:10.1080/00343404.2020.1826420
Wang, S., Liu, J., and Qin, X. (2022). Financing constraints, carbon emissions and high-quality urban development-empirical evidence from 290 cities in China. Int. J. Environ. Res. Public Health 19 (4), 2386. doi:10.3390/ijerph19042386
Xi, J. (2019). Speech at the symposium on ecological protection and high-quality development of the Yellow River Basin. China Water Resour. 20, 1–3. doi:10.16616/j.cnki.10-1326/TV.2019.11.01
Xu, X., and Wang, Y. (2017). Study on spatial spillover effects of logistics industry development for economic growth in the Yangtze River Delta city cluster based on spatial Durbin model. Int. J. Environ. Res. Public Health 14 (12), 1508. doi:10.3390/ijerph14121508
Yang, Y. W., and Zhang, P. (2021). Logic, measurement and governance in China’s high quality economic development. Econ. Res. 1, 26–42.
Yang, Y., Ren, L., Du, Z., and Tong, G. (2021). Measurement and spatiotemporal analysis of high-quality development of China's industry. PLoS One 16 (12), e0259845. doi:10.1371/journal.pone.0259845
Yu, G., and Zhou, X. (2021). The influence and countermeasures of digital economy on cultivating new driving force of high-quality economic development in Henan Province under the background of "double circulation. Ann. Oper. Res., 1–22. doi:10.1007/s10479-021-04325-4
Yuan, Y., Han, Z., and Yang, W. (2021). “A co-innovation network in Northeast China oriented to high-quality development,” in Technology analysis & strategic management, 1–18. doi:10.1080/09537325.2021.1991573
Zhang, W., Zhao, S., Wan, X., and Yao, Y. (2021). Study on the effect of digital economy on high-quality economic development in China. PLoS One 16 (9), e0257365. doi:10.1371/journal.pone.0257365
Zhao, M., Liu, F., Song, Y., and Geng, J. (2020). Impact of air pollution regulation and technological investment on sustainable development of green economy in eastern China: Empirical analysis with panel data approach. Sustainability 12 (8), 3073. doi:10.3390/su12083073
Keywords: yellow river basin (YRB), high-quality urban development, the new monmentum for data, government investment in technology, entropy value method
Citation: Jin H and Yang N (2022) New momentum for data, government investment in technology, and high-quality urban development in the yellow river basin. Front. Environ. Sci. 10:969454. doi: 10.3389/fenvs.2022.969454
Received: 15 June 2022; Accepted: 18 July 2022;
Published: 26 August 2022.
Edited by:
Syed Abdul Rehman Khan, Xuzhou University of Technology, ChinaReviewed by:
Muhammad Tanveer, Prince Sultan University, Saudi ArabiaCopyright © 2022 Jin and Yang. This is an open-access article distributed under the terms of the Creative Commons Attribution License (CC BY). The use, distribution or reproduction in other forums is permitted, provided the original author(s) and the copyright owner(s) are credited and that the original publication in this journal is cited, in accordance with accepted academic practice. No use, distribution or reproduction is permitted which does not comply with these terms.
*Correspondence: Hao Jin, YWhhcWpoNkAxNjMuY29t
Disclaimer: All claims expressed in this article are solely those of the authors and do not necessarily represent those of their affiliated organizations, or those of the publisher, the editors and the reviewers. Any product that may be evaluated in this article or claim that may be made by its manufacturer is not guaranteed or endorsed by the publisher.
Research integrity at Frontiers
Learn more about the work of our research integrity team to safeguard the quality of each article we publish.