- Department of Educational Technology, Faculty of Education, University of Ha’il, Ha’il, Saudi Arabia
Although m-learning applications have been widely used in universities, the factors that might affect the continuous intention to utilise them have not been fully addressed. Therefore, this study aimed to understand this by extending the expectation confirmation model (ECM) and incorporating self-regulated learning (SRL). A quantitative research design was adopted, and data were gathered using a structured questionnaire distributed to 227 undergraduate university students through simple random sampling. The data were analysed using structural equation modelling (SEM) in AMOS. The findings revealed that the proposed model has high explanatory power (79%), which explains the phenomena of influential factors. Additionally, students’ perceived usefulness (PU) and satisfaction had a positive direct significant effect on students’ continuous intention to use m-learning applications. However, perceived usefulness did not affect satisfaction but confirmation did. Furthermore, SRL had an indirect positive effect on continuous intention, while it had a direct significant effect on both perceived usefulness and confirmation. The practical and theoretical implications of this study are further discussed in detail.
Introduction
The establishment of mobile devices has resulted in the quick advancement of mobile learning applications. Advances in mobile tìhnologies and networking communications have changed people’s learning methods and lives (Chao, 2019; Liu et al., 2021a). Several researchers have stated that the integration of learning online and mobile learning applications is an essential aspect for both students and instructors (Hoi, 2020; Alshurideh et al., 2023). Due to the increased utilisation of mobile devices, their utilisation for learning has increased widely, and many mobile learning applications have been developed (Alshurideh et al., 2023). Learning via m-learning applications enables people to learn without limitations in space or time, which boosts their learning effectiveness and intentions (Liu et al., 2021b).
M-learning applications have been developed for the purpose of teaching specific knowledge and have become popular among university students (Hoi, 2020). There are several advantages to utilising m-learning applications, such as their flexibility, portability, accessibility and dealing with learners individually (Sung et al., 2015; Chen, 2018). For promoting learning and achieving educational objectives, some of these m-learning applications are designed specifically, such as situational mode, embedding engagement mode, providing recommendation services and including gamification (Chen, 2018; Reinders and Pegrum, 2017). These m-learning applications offer learners a convenient service, such as learning different tasks, examinations, preparing to work, etc. Some studies have assessed the effectiveness of m-learning platforms (Papadima-Sophocleous et al., 2012; Lee and Sylvén, 2021). For instance, in m-learning applications utilised for English learning, scholars have stated that m-learning assisted learners with reading and pronunciation (Cao and Deng, 2019), writing (Li and Hegelheimer, 2013), learning vocabulary (Klimova, 2021) and communication skills (Lee and Sylvén, 2021).
Based on the above-mentioned findings, it is confirmed that m-learning applications could offer huge benefits for learners, which can cultivate a positive attitude among learners towards using them (Yang and Wang, 2019). However, some learners still prefer learning through non-tech applications, such as physical books (Nie et al., 2020), which leads to the question that offering m-learning applications for learners might not necessarily lead to a continued intention to use them.
Furthermore, offering m-learning applications has some complexities that require careful consideration to maintain their effectiveness and lead to a continued desire to be used by learners (Obeid et al., 2024). One of these obstacles is ensuring that learners remain motivated to utilise m-learning applications. Thus, further studies are essential to assess the variables that may affect learners’ continued intention to utilise these m-learning applications (Foo et al., 2021; Obeid et al., 2024). Many scholars have stated that further understanding is needed regarding the influential factors that might affect or hinder students’ continuous intention to utilise m-learning (Goh and Yang, 2021; Obeid et al., 2024).
Additionally, to maintain the progress of m-learning applications and achieve their desired objectives, academics and decision-makers should investigate the variables that may affect students’ continued intention to utilise these m-learning applications. Furthermore, assessing and identifying the variables that may affect students’ continued intention to utilise m-learning applications would help decision-makers comprehend these influential factors and encourage them to develop solutions for addressing the obstacles. Thus, this study proposes a model for assessing the variables that might affect students’ continuous intention to utilise m-learning applications. The ECM is one of the most popular models utilised to examine users’ continuous intentions to use a specific technology (Mouakket, 2018).
Based on ECM, confirmation, PU and satisfaction are the main variables that are associated with users’ continuous intention to utilise information systems. In learning through technologies context, many researchers have confirmed that PU and confirmation predict users’ satisfaction, which leads to a higher continuous intention to utilise technologies (Joo et al., 2011; Alshammari and Alshammari, 2024). However, many other factors could play a role and affect students’ intentions. Students’ self-regulation focuses on their behaviour to monitor and control the learning process, which enables them to reach their educational goals.
To our knowledge, no study has combined ECM with self-regulation to assess its effects on students’ continuous intention to utilise m-learning applications. Thus, this study proposes a theoretical model relying on ECM and combines additional variables, namely self-regulation, to assess their effects on students’ continued intention to utilise m-learning applications.
This study makes two contributions. It theoretically advances the ECM framework by adding a learner centered variable, broadening and expanding its intended use in educational research field. This innovation bridges the gap between theories of user satisfaction and self-directed learning paradigms. The findings of this research also have practical implications for educators, instructional designers, and developers of m-learning applications. With a deeper understanding about how self-regulation influences prolonged engagement, stakeholders could build more adaptive, more effective learning systems to aid students in intervening on their way toward their educational goals. Therefore, this study provides the foundation for developing user friendly but pedagogically robust m-learning environments, which, in turn, will promote the long term adoption and enhanced learning outcomes.
Literature review and research model
Mobile learning applications aim at providing convenience to gain knowledge for learners (Hoi, 2020). The huge demands of these mobile applications have resulted in a rapid expansion to contain other apps such as e-books, games, entertainment, social media platforms and finance (Hsu and Lin, 2015). Recently, the number of learners using mobile learning applications for several learning objectives has increased rapidly (Chen, 2018; Nie et al., 2020). Students at universities are considered the largest users of these mobile learning applications (Liu et al., 2021a). Although the utilisation of mobile learning apps among students is widely increased (Bian and Liu, 2017), they could provide huge benefits in terms of improving the general knowledge level and professional level. However, there is a phenomenon that, although learners use these mobile learning applications for some time, they might stop utilising or even reject continuing to utilise them (Nie et al., 2020).
Some research evaluated the effectiveness of m-learning applications (Li and Hegelheimer, 2013; Chen, 2018) and the variables affecting learners’ initial adoption and acceptance (Nie et al., 2020; Bian and Liu, 2017); however, few studies focused on continuous use (Wang and Yu, 2024). Additionally, the investigation of users’ intentions towards utilising m-learning applications is considered a reliable indication of m-learning applications. Thus, greater intention is focused on assessing the continuous intention to utilise among users (Amir et al., 2020).
Several models and theories applied in previous studies focused on analysing the ongoing intention to accept technologies among users, such as UTAUT, TAM, TPB and ECM (Ding, 2019; Prasetya et al., 2021). However, assessing users’ determination in the post-adoption phase requires focused attention research (Sayyah Gilani et al., 2017). The ECM is considered one of the most popular frameworks used to assess continuous intention in the IS field, as it is established to assess the phase of post-adoption instead of the first acceptance and adoption phase (Steuer, 1995; Rabaa’I et al., 2021; Cheng, 2020).
According to Guo et al. (2023) and Bhattacherjee (2001), in the ECM, users’ PU and confirmation affect their satisfaction, which in turn influences users’ continuous intention. However, other factors could play a role in and affect students’ continuous intentions. Students’ self-regulation focused on their behaviour to monitor and control the learning process, which enabled them to reach their educational goals. To our knowledge, the combined ECM with self-regulation to assess their influences on the continuous intention to utilise mobile learning applications has not been examined. Thus, this study proposes a model by extending the ECM and combining self-regulation to assess students’ continuous intentions to utilise m-learning applications.
The ECM
The ECM was established by Bhattacherjee (2001) and drawn from expectation confirmation theory, which is widely utilised for understanding consumer behaviour post-purchase. The ECM is seen as a more solid and rigorous model for assessing continuous intention to accept technologies than other well-known models, such as the TAM and UTAU (Ambalov, 2018). Figure 1 shows ECM model.
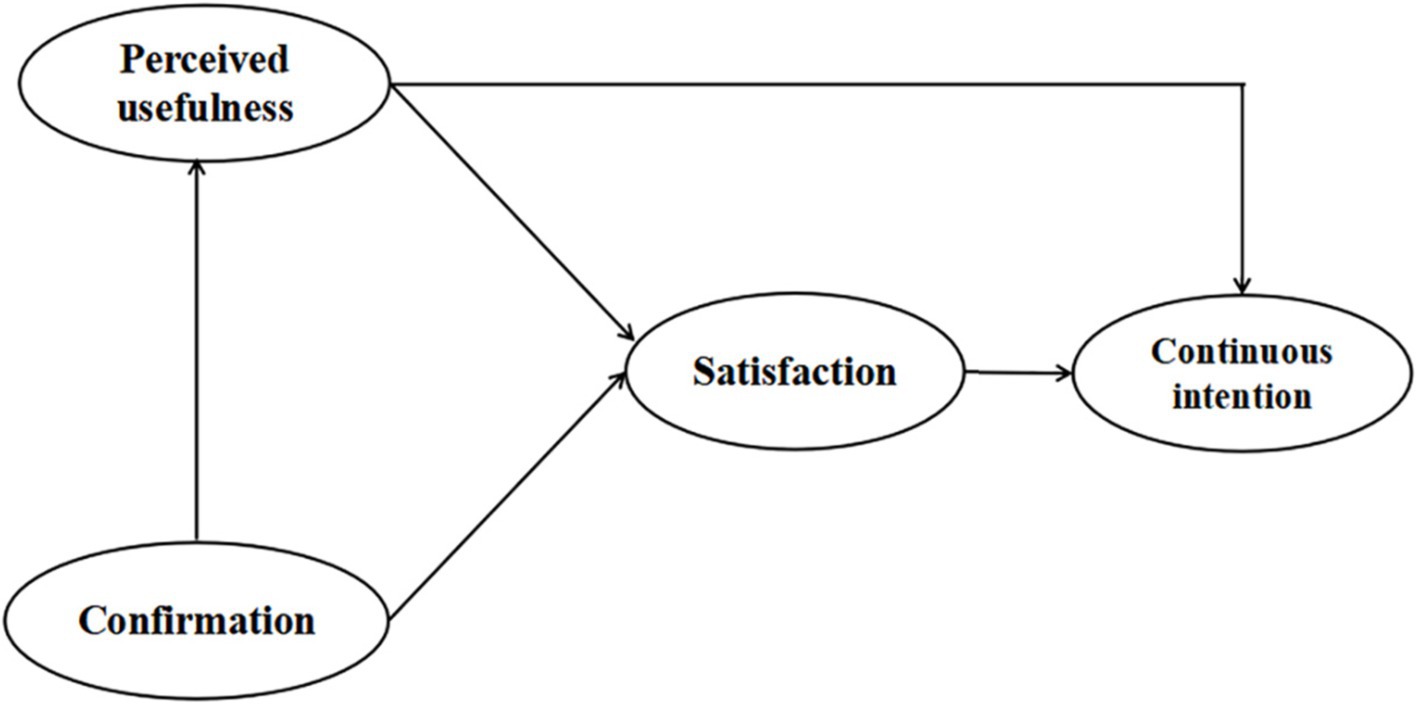
Figure 1. ECM model (Bhattacherjee, 2001).
According to the ECM, PU and confirmation affect satisfaction and both PU and satisfaction affects the continuous intention to use a given IS technology (Bhattacherjee, 2001).
PU, confirmation, satisfaction and continuous intention
PU refers to users’ evaluations of the advantages using IS technology, especially when it improves performance and outcomes (Scherer et al., 2019). It is a predicting key for technology adoption since it not only predicts continuous intention but also predicts satisfaction, which plays a mediating role in affecting continuous intention (Bhattacherjee, 2001). On the other hand, confirmation refers to users’ perceptions and expectations regarding the use of IS systems (Alshammari and Alshammari, 2024). Confirmation was a key factor that determined students’ satisfaction with IS technology (Alshammari and Alshammari, 2024; Stone and Baker-Eveleth, 2013; Limayem and Cheung, 2008).
Satisfaction is users’ assessment of their use experiences with IS systems (Alshammari and Alshammari, 2024; Bhattacherjee, 2001). It predicts the continuous intention to utilise a specific technology (Choi et al., 2019; Mouakket, 2015; Alshammari and Alshammari, 2024). Satisfied users with utilising a system are likelier to continue utilising it (Ashfaq et al., 2020; Alshammari and Alshammari, 2024). Few studies were conducted to assess the effect of satisfaction on continuous intention (Ifinedo, 2007; Ramadhan et al., 2022; Alshammari and Alshammari, 2024).
Based on this, the following hypotheses were formulated:
H1: Confirmation positively affects satisfaction.
H2: PU positively affects satisfaction.
H3: PU positively affects the continuous intention to utilise m-learning applications.
H4: Satisfaction positively affects the continuous intention to utilise m-learning applications.
Self-regulated learning
SRL refers to controlling students’ motivations, learning behaviors and thoughts to accomplish and achieve their learning objectives (Pintrich, 1995; Boekaerts et al., 1999). It deals with how students use cognitive and metacognitive learning approaches and strategies which are controlled by motivation (Pintrich and Schragben, 2012).
Hashim et al. (2015) found that when students had high self-motivation in blended online courses, this led to a more positive attitude towards using it. Several scholars have found that motivation influences students’ behavioral intention to study in online courses, based on self-determination theory (Zhou, 2016; Ifinedo, 2017; Joo et al., 2018). Thus, the following is hypothesised:
H5: Students’ SRL positively affects their PU.
H6: Students’ SRL positively affects their confirmation.
The proposed research model is presented in Figure 2.
Methodology
Research design
A quantitative research design was utilized to determine measurement metrics for analyzing relationships among variables and testing theoretical models through statistical analysis (Creswell and Creswell, 2017). Educational and behavioral research designs that are quantitative are most effective in creating generalizable insights and understanding how user behavior evolves in technology enhanced learning environments (Gall et al., 2007).
Participants
To gather data from respondents, Google forms (and URL links) were delivered via email to the targeted population during the first semester of 2024. A total of 227 students participated in filling out the questionnaires. A simple random technique was employed to select the samples to ensure that all samples were representative of the study participants. In order to have the students’ responses be relevant, they were targeted to be students who have had prior experience using m learning applications. Thus, all participating students had experience using m-learning applications. They filled out the questionnaires voluntarily and anonymously, as there was no information that directly led to the respondents’ personal information. All items were measured using a 5-point Likert scale.
Most of the respondents were female students (135; 59.5%) and 92 (40.5%) were male students. In terms of their years of study, most of the participants were enrolled in the first year (64: 28.2%), followed by those in the second year (55: 24.2%) and third year (46: 20.3%), while the lowest were enrolled in the fourth year (26: 11.5%). In terms of their educational level, most of them were enrolled in a bachelor’s degree programme (136: 59.9%), followed by those enrolled in a diploma programme (82: 36.1%), while the lowest were enrolled in a master’s degree programme (9: 4.0%). Regarding their colleges, most of them were enrolled in Applied College (81: 35.7%) followed by college of Education (31: 13.7%) and college of Business Administration (25: 11.0), while the lowest were enrolled in the preparation year (6: 2.6%). Regarding the m-learning applications they were using, most used the Blackboard application (221: 97.4%), followed by ChatGPT (5: 2.2%) and Coursera (1: 0.4%). Table 1 shows demographic information of respondents.
Instrumentation
The first part of the survey was specified for the respondent’s demographic information, which was self-created and included five questions regarding their gender, years of study, education level, college and most used m-learning applications, while the second part has 18 items to measure model’s five constructs (i.e., confirmation, PU, satisfaction and continuous intention), adapted from Bhattacherjee (2001) and Mouakket (2015), while the items measuring students’ self-regulation were adapted from Gökçearslan et al.’s (2016) study.
Due to the questionnaire being translated into Arabic, a “back-translation” was applied. Two bilingual experts (English and Arabic) were asked to translate the questionnaire, which was originally in English to Arabic, to maintain the equivalence of the translation. To ensure face and content validity, five professors were invited to check and comment on the instrument’s length, format and the scales’ correct wordings. Some of these items, due to their recommendations, were revised to better match the research objectives. The pre-test results showed that face and content validity were achieved.
Data analysis
For analysing the demographic information of the respondents, SPSS (Version 24.0) was used. To analyse the relationships of constructs, two steps in structural equation modelling (SEM) AMOS were used. Confirmatory factor analysis (CFA) was conducted to assess constructs’ validities. Then, SEM was applied to assess the constructs’ relationships and hypotheses.
Results
Confirmatory factor analysis
CFA is an analysis approach conducted to validate the measurement model concerning the handling of correlations, measurement errors and validities. According to Awang (2015), all validities must be checked during a CFA before moving to the second step to assess the relationships and test the hypothesis in SEM. Construct validity was met when all indices of the model met the recommended values suggested in the literature (Awang, 2015). Thus, the CFA was run after deleting one item (PU3) due to its low loading factor. The values of the indices in the model are shown as the output of CFA in Figure 3.
As shown in the above figure of the CFA output, all indices of the model met the required values in the literature “Chisq/df = 2.356 < 3.0; CFI = 0.965 > 0.90; TLI = 0.954 > 0.90; IFI = 0.965 > 0.90; RMSEA = 0.077 < 0.08.” Thus, construct validity was achieved (Hair et al., 2010; Awang, 2015).
Next, convergent validity is achieved once the composite reliability (CR) value is greater than (0.6) and average variance extracted (AVE) is greater than (0.5) (Hair et al., 2010). The output of these values, as shown in Table 2, confirms that the suggested values are met and, thus, convergent validity is achieved.
Lastly, discriminant validity is essential to ensure that each construct is distinct from other constructs. According to Awang (2015), discriminant validity is met once all values of the square root of AVE, which are in bold, are greater than all other values in its rasa bd column. As shown in Table 3, all bolded values met the suggested recommendations. Thus, discriminant validity was achieved.
Standardised estimate
A standardised estimate is necessary to measure the rigour and strength of relationships between constructs, factor loading and the R square of the dependent variable. The standardised estimate output is run and is presented in Figure 4.
The R square of the dependent variable in the model is 0.79, confirming that 79% of students’ continuous intention to use m-learning applications is explained by all other factors, namely satisfaction, PU, confirmation and self-regulation. According to Cohen (1988), the values of R square above 0.26 confirm the high explanatory power of the proposed mode. Thus, the proposed model is a robust model for explaining the phenomena of the factors that affect the utilisation of m-learning applications.
Unstandardised estimate
An unstandardised estimate run is needed to calculate the beta weight and critical ratio to test the hypothesis of this research. Thus, the unstandardised estimate is run, and its output is shown in Figure 5.
Hypotheses testing results
The results confirm that self-regulation had a positive significant effect on both PU and confirmation “β = 0.806, p < 0.05; β = 0.968, p < 0.05.” Thus, H5 and H6 are supported. Furthermore, confirmation had a positive significant effect on satisfaction “β = 0.940, p < 0.05.” Thus, H1 is supported. Also, PU positively affected the continuous intention “β = 0.297, p < 0.05.” Thus, H3 is supported. Furthermore, satisfaction had a significant positive effect on continuous intention “β = 0.638, p < 0.05.” Thus, H4 is supported. However, PU had an insignificant effect on satisfaction “β = 0.098, p > 0.05.” Thus, H2 was rejected. Table 4 presents the hypothesis results.
Discussion
This study assessed students’ continuous intention to use m-learning applications by combining ECM and SRL. The research model was proposed and the data of 227 respondents were collected and analysed using two steps in SEM AMOS. A detailed discussion is presented in the following sections.
The findings showed that PU and satisfaction directly affected the continuous intention to use m-learning applications, which confirms previous studies (Ambalov, 2018; Alshammari and Alshammari, 2024). These findings indicate that when students perceive using m-learning applications as useful and are satisfied with using them, this will lead to a continuous intention to use them. However, PU had an insignificant effect on satisfaction. These findings might explain why when students perceive the use of m-learning applications as useful, this does not necessarily make them feel satisfied with using such applications.
Furthermore, confirmation positively affected satisfaction, which confirms previous studies (Bhattacherjee, 2001; Alshammari and Alshammari, 2024). These findings indicate that when students had great experience with using m-learning applications, this would positively affect their satisfaction. In other words, when students confirm their positive experience and when these m-learning applications meet their expectations, they are likelier to be more satisfied with using these m-learning applications.
Additionally, SRL had a significant positive indirect effect on the continuous intention to use m-learning applications and a direct effect on both PU and confirmation. These findings are consistent with some prior studies (Hood, 2013; Lee, 2010). The findings might indicate that when students have a higher level of SRL, they are likelier to perceive m-learning applications as useful and confirm their positive experience, which leads to continuous use. Hood (2013) found that greater reliance on rehearsal, high work commitments and high critical thinking and self-regulation were core variables that predicted students’ continuous intention to utilise online lecturers.
Contributions and implications
This study has some main contributions. First, this is the first study to explore the role of SRL by combining it with the ECM model to investigate students’ continuous intention to use m-learning applications. It provides a theoretical framework which enhances the understanding of the phenomenon of the continuous use of m-learning applications and could be utilised as a basis for future studies.
Second, assessing the effects of self-regulation, PU, confirmation and satisfaction on the continuous intention to use m-learning applications assists in bridging the existing research gap in the literature on ECM and adoption of technologies. Another notable contribution is that the proposed model has a high explanatory power (R2 = 0.79 (79%)) when compared to the original proposed model by Bhattacherjee (2001), which explained 41%. This indicates that all constructs in the model, including the additional construct, SRL, played a significant role in explaining the phenomena of utilising m-learning applications.
The findings have practical implications for designers and practitioners of m-learning applications. The positive effect of both PU and satisfaction, which influenced the continuous intention to utilise m-learning applications, suggests that the designers of m-learning applications should pay attention to providing learners with useful content and materials and a pleasant environment. M-learning application developers could enhance PU and satisfaction by working on improving app-loading speeds, minimising response times and providing the materials that students would like to use for learning.
Confirmation affected students’ satisfaction. Thus, developers and instructors should ensure that students have positive experiences using m-learning applications. This could be done by considering students’ opinions regarding their use of m-learning applications and by finding out if they have any difficulties with their use that need to be solved. Doing this could lead to students being satisfied with using m-learning applications.
Furthermore, SRL affected both PU and confirmation. Thus, instructors should pay attention to enhancing students’ motivation through and to their learning behavior when using m-learning applications. When students have self-regulation and motivation, it could lead them to perceive these applications as useful and confirm their positive experiences with using them, which could lead to their continuous use.
Limitations and future research
Although this study has promising findings, there are some limitations. The study sample size is limited to one university in Saudi Arabia, which might affect the findings’ generalizability. Thus, future studies might include samples from other universities or from other contexts. Furthermore, this study focused on a quantitative method using questionnaires. Future studies could use a mixed-methods, which might provide deeper understanding regarding the phenomenon of the continuous use of m-learning applications. This study extended the ECM and included the SRL construct. Future studies might extend ECM and incorporate other factors which may affect the continuous intention of m-learning, such as instructor support and engagement.
Conclusion
This combines ECM with SRL to assess factors which affect students’ continuous intention to utilise m-learning applications. The findings showed that SRL had an indirect effect on students’ continuous intentions and a direct effect on both confirmation and PU. Furthermore, both PU and satisfaction affected students’ continuous intention to use m-learning applications. Thus, designers and developers of m-learning applications should consider these factors when designing m-learning applications because they directly or indirectly affect the continuous intention to utilise m-learning applications.
Furthermore, educational institutions and instructors should work on improving SRL among students by increasing their motivation, as a higher level of students’ SRL would positively affect their perception of m-learning applications as useful and lead to perceiving a positive experience that can be confirmed by them. Considering all of this could lead to the continuous intention to use m-learning applications.
Data availability statement
The datasets presented in this study can be found in online repositories. The names of the repository/repositories and accession number(s) can be found in the article/supplementary material.
Ethics statement
The studies involving humans were approved by Ethical approval committee at University of Ha’il. The studies were conducted in accordance with the local legislation and institutional requirements. The participants provided their written informed consent to participate in this study. Written informed consent was obtained from the individual(s) for the publication of any potentially identifiable images or data included in this article.
Author contributions
SuA: Conceptualization, Data curation, Funding acquisition, Investigation, Methodology, Software, Supervision, Writing – original draft, Writing – review & editing. SaA: Formal analysis, Funding acquisition, Project administration, Resources, Validation, Visualization, Writing – original draft, Writing – review & editing.
Funding
The author(s) declare that no financial support was received for the research, authorship, and/or publication of this article.
Conflict of interest
The authors declare that the research was conducted in the absence of any commercial or financial relationships that could be construed as a potential conflict of interest.
Generative AI statement
The author(s) declare that no Generative AI was used in the creation of this manuscript.
Publisher’s note
All claims expressed in this article are solely those of the authors and do not necessarily represent those of their affiliated organizations, or those of the publisher, the editors and the reviewers. Any product that may be evaluated in this article, or claim that may be made by its manufacturer, is not guaranteed or endorsed by the publisher.
References
Alshammari, S. H., and Alshammari, R. A. (2024). An integration of expectation confirmation model and information systems success model to explore the factors affecting the continuous intention to utilise virtual classrooms. Sci. Rep. 14:18491. doi: 10.1038/s41598-024-69401-8
Alshurideh, M., Al Kurdi, B., Salloum, S. A., Arpaci, I., and Al-Emran, M. (2023). Predicting the actual use of m-learning systems: a comparative approach using PLS-SEM and machine learning algorithms. Interact. Learn. Environ. 31, 1214–1228. doi: 10.1080/10494820.2020.1826982
Ambalov, I. A. (2018). A meta-analysis of IT continuance: an evaluation of the expectation-confirmation model. Telematics Inform. 35, 1561–1571. doi: 10.1016/j.tele.2018.03.016
Amir, L. R., Tanti, I., Maharani, D. A., Wimardhani, Y. S., Julia, V., Sulijaya, B., et al. (2020). Student perspective of classroom and distance learning during COVID-19 pandemic in the undergraduate dental study program Universitas Indonesia. BMC Med. Educ. 20, 1–8. doi: 10.1186/s12909-020-02312-0
Ashfaq, M., Yun, J., Yu, S., and Loureiro, S. M. C. (2020). I, Chatbot: modeling the determinants of users’ satisfaction and continuance intention of AI-powered service agents. Telematics Inform. 54:101473. doi: 10.1016/j.tele.2020.101473
Awang, P. (2015). SEM made simple: A gentle approach to learning structural equation modeling. Bangi: MPWS Rich Publication.
Bhattacherjee, A. (2001). Understanding information systems continuance: an expectation-confirmation model. MIS Q. 25, 351–370. doi: 10.2307/3250921
Bian, Y., and Liu, Y. (2017). Current situation of Chinese college students using English learning app. New Media Stud. 3, 21–22.
Boekaerts, M., Zeidner, M., and Pintrich, P. R. (Eds.) (1999). Handbook of self-regulation : Elsevier.
Cao, J., and Deng, X. (2019). Research on the influence of “Baicizhan” on college students’ English vocabulary Learniackng in the perspective of mobile learning. Technol. Enhanced Foreign Lang. 3, 43–48.
Chao, C. M. (2019). Factors determining the behavioral intention to use mobile learning: an application and extension of the UTAUT model. Front. Psychol. 10:1652. doi: 10.3389/fpsyg.2019.01652
Chen, C. P. (2018). Understanding mobile English-learning gaming adopters in the self-learning market: the uses and gratification expectancy model. Comput. Educ. 126, 217–230. doi: 10.1016/j.compedu.2018.07.015
Cheng, Y. M. (2020). Students' satisfaction and continuance intention of the cloud-based e-learning system: roles of interactivity and course quality factors. Education+. Training 62, 1037–1059. doi: 10.1108/ET-10-2019-0245
Choi, G., Nam, C., and Kim, S. (2019). The impacts of technology platform openness on application developers’ intention to continuously use a platform: from an ecosystem perspective. Telecommun. Policy 43, 140–153. doi: 10.1016/j.telpol.2018.04.003
Cohen, J. (1988). “The analysis of variance and covariance” in Statistical power analysis for the behavioural sciences.
Creswell, J. W., and Creswell, J. D. (2017). Research design: qualitative, quantitative, and mixed methods approaches : Sage publications.
Ding, Y. (2019). Looking forward: the role of hope in information system continuance. Comput. Hum. Behav. 91, 127–137. doi: 10.1016/j.chb.2018.09.002
Foo, C. C., Cheung, B., and Chu, K. M. (2021). A comparative study regarding distance learning and the conventional face-to-face approach conducted problem-based learning tutorial during the COVID-19 pandemic. BMC Med. Educ. 21, 1–6. doi: 10.1186/s12909-021-02575-1
Gall, M. D., Gall, J. P., and Borg, W. R. (2007). Educational research: an introduction (8. Utg.). USA: Pearson: AE Burvikovs.
Goh, T. T., and Yang, B. (2021). The role of e-engagement and flow on the continuance with a learning management system in a blended learning environment. Int. J. Educ. Technol. High. Educ. 18:49. doi: 10.1186/s41239-021-00285-8
Gökçearslan, Ş., Mumcu, F. K., Haşlaman, T., and Çevik, Y. D. (2016). Modelling smartphone addiction: the role of smartphone usage, self-regulation, general self-efficacy and cyberloafing in university students. Comput. Hum. Behav. 63, 639–649. doi: 10.1016/j.chb.2016.05.091
Guo, Q., Zeng, Q., and Zhang, L. (2023). What social factors influence learners' continuous intention in online learning? A social presence perspective. Inf. Technol. People 36, 1076–1094. doi: 10.1108/ITP-02-2021-0151
Hair, J. F., Black, W. C., Babin, B. J., and Anderson, R. E. (2010). Multivariate data analysis. 7th Edn. New York: Pearson.
Hashim, K. F., Tan, F. B., and Rashid, A. (2015). Adult learners' intention to adopt mobile learning: A motivational perspective. Br. J. Educ. Technol. 46, 381–390. doi: 10.1111/bjet.12148
Hoi, V. N. (2020). Understanding higher education learners' acceptance and use of mobile devices for language learning: A Rasch-based path modeling approach. Comput. Educ. 146:103761. doi: 10.1016/j.compedu.2019.103761
Hood, M. (2013). Bricks or clicks? Predicting student intentions in a blended learning buffet. Australas. J. Educ. Technol. 29. doi: 10.14742/ajet.415
Hsu, C. L., and Lin, J. C. C. (2015). What drives purchase intention for paid mobile apps?–an expectation confirmation model with perceived value. Electron. Commer. Res. Appl. 14, 46–57. doi: 10.1016/j.elerap.2014.11.003
Ifinedo, P. (2007). Investigating the antecedents of continuance intention of course management systems use among Estonian undergraduates. Int. J. Inform. Commun. Technol. Educ. 3, 76–92. doi: 10.4018/jicte.2007100107
Ifinedo, P. (2017). Examining students' intention to continue using blogs for learning: perspectives from technology acceptance, motivational, and social-cognitive frameworks. Comput. Hum. Behav. 72, 189–199. doi: 10.1016/j.chb.2016.12.049
Joo, Y. J., Lim, K. Y., and Kim, E. K. (2011). Online university students' satisfaction and persistence: examining perceived level of presence, usefulness and ease of use as predictors in a structural model. Comput. Educ. 57, 1654–1664. doi: 10.1016/j.compedu.2011.02.008
Joo, Y. J., So, H. J., and Kim, N. H. (2018). Examination of relationships among students' self-determination, technology acceptance, satisfaction, and continuance intention to use K-MOOCs. Comput. Educ. 122, 260–272. doi: 10.1016/j.compedu.2018.01.003
Klimova, B. (2021). Evaluating impact of mobile applications on EFL university learners’ vocabulary learning–A review study. Procedia Comput. Sci. 184, 859–864. doi: 10.1016/j.procs.2021.03.108
Lee, M. C. (2010). Explaining and predicting users’ continuance intention toward e-learning: an extension of the expectation–confirmation model. Comput. Educ. 54, 506–516. doi: 10.1016/j.compedu.2009.09.002
Lee, J. S., and Sylvén, L. K. (2021). The role of informal digital learning of English in Korean and Swedish EFL learners’ communication behaviour. Br. J. Educ. Technol. 52, 1279–1296. doi: 10.1111/bjet.13082
Li, Z., and Hegelheimer, V. (2013). Mobile-assisted grammar exercises: Effects on self-editing in L2 writing.
Limayem, M., and Cheung, C. M. (2008). Understanding information systems continuance: the case of internet-based learning technologies. Inf. Manag. 45, 227–232. doi: 10.1016/j.im.2008.02.005
Liu, C., He, J., Ding, C., Fan, X., Hwang, G. J., and Zhang, Y. (2021a). Self-oriented learning perfectionism and English learning burnout among EFL learners using mobile applications: the mediating roles of English learning anxiety and grit. Learn. Individ. Differ. 88:102011. doi: 10.1016/j.lindif.2021.102011
Liu, H., Shao, M., Liu, X., and Zhao, L. (2021b). Exploring the influential factors on readers' continuance intentions of E-book APPs: personalization, usefulness, playfulness, and satisfaction. Front. Psychol. 12:640110. doi: 10.3389/fpsyg.2021.640110
Mouakket, S. (2015). Factors influencing continuance intention to use social network sites: the Facebook case. Comput. Hum. Behav. 53, 102–110. doi: 10.1016/j.chb.2015.06.045
Mouakket, S. (2018). The role of personality traits in motivating users' continuance intention towards Facebook: gender differences. J. High Technol. Managem. Res. 29, 124–140. doi: 10.1016/j.hitech.2016.10.003
Nie, J., Zheng, C., Zeng, P., Zhou, B., Lei, L., and Wang, P. (2020). Using the theory of planned behavior and the role of social image to understand mobile English learning check-in behavior. Comput. Educ. 156:103942. doi: 10.1016/j.compedu.2020.103942
Obeid, A., Ibrahim, R., and Fadhil, A. (2024). Extended model of expectation confirmation model to examine users’ continuous intention toward the utilization of E-learning platforms. IEEE Access 12, 40752–40764. doi: 10.1109/ACCESS.2024.3373190
Papadima-Sophocleous, S., Georgiadou, O., and Mallouris, Y. (2012). iPod impact on oral reading fluency of university ESAP students.
Pintrich, P. R. (1995). Understanding self-regulated learning. Jossey-Bass 1995, 3–12. doi: 10.1002/tl.37219956304
Pintrich, P. R., and Schragben, B. (2012). “Students' motivational beliefs and their cognitive engagement in classroom academic tasks” in Student perceptions in the classroom (Routledge), 149–184.
Prasetya, F. H., Harnadi, B., Widiantoro, A. D., and Nugroho, A. C. (2021). Extending ECM with quality factors to investigate continuance intention to use E-learning. In 2021 sixth international conference on informatics and computing (ICIC) (pp. 1–7). IEEE.
Rabaa’i, A. A., Almaati, S. A., and Zhu, X. (2021). Students’ continuance intention to use Moodle: an expectation-confirmation model approach. Interdiscip. J. Inf. Knowl. Manag. 16, 397–434. doi: 10.28945/4842
Ramadhan, A., Hidayanto, A. N., Salsabila, G. A., Wulandari, I., Jaury, J. A., and Anjani, N. N. (2022). The effect of usability on the intention to use the e-learning system in a sustainable way: A case study at Universitas Indonesia. Educ. Inf. Technol. 27, 1489–1522. doi: 10.1007/s10639-021-10613-0
Reinders, H., and Pegrum, M. (2017). “Supporting language learning on the move” in SLA research and materials development for language learning. ed. B. Tomlinson (Routledge), 219–232.
Sayyah Gilani, M., Iranmanesh, M., Nikbin, D., and Zailani, S. (2017). EMR continuance usage intention of healthcare professionals. Inform. Health Soc. Care 42, 153–165. doi: 10.3109/17538157.2016.1160245
Scherer, R., Siddiq, F., and Tondeur, J. (2019). The technology acceptance model (TAM): A meta-analytic structural equation modeling approach to explaining teachers’ adoption of digital technology in education. Comput. Educ. 128, 13–35. doi: 10.1016/j.compedu.2018.09.009
Steuer, J. (1995). Defining virtual reality: Dimensions determining telepresence : Communication in the age of virtual reality/Lawrence Erlbaum and Associates.
Stone, R. W., and Baker-Eveleth, L. (2013). Students’ expectation, confirmation, and continuance intention to use electronic textbooks. Comput. Hum. Behav. 29, 984–990. doi: 10.1016/j.chb.2012.12.007
Sung, Y. T., Chang, K. E., and Yang, J. M. (2015). How effective are mobile devices for language learning? A meta-analysis. Educ. Res. Rev. 16, 68–84. doi: 10.1016/j.edurev.2015.09.001
Wang, X., and Yu, X. (2024). Art students’ technostress, perceived usefulness, satisfaction, and continuance intention to use Mobile educational applications. SAGE Open 14:21582440241260206. doi: 10.1177/21582440241260206
Yang, Y., and Wang, X. (2019). Modeling the intention to use machine translation for student translators: an extension of technology acceptance model. Comput. Educ. 133, 116–126. doi: 10.1016/j.compedu.2019.01.015
Keywords: m-learning applications, continuance intention, ECM, self-regulated learning, satisfaction, perceived usefulness
Citation: Alshammari SH and Alkhabra S (2025) Modelling the effects of self-regulation, perceived usefulness, confirmation and satisfaction on the continuous intention to utilise mobile learning applications. Front. Educ. 10:1506185. doi: 10.3389/feduc.2025.1506185
Edited by:
Todor Ganchev, Technical University of Varna, BulgariaReviewed by:
Eduardo Moraes Sarmento, University of Lisbon, PortugalJohn Mark R. Asio, Gordon College, Philippines
Copyright © 2025 Alshammari and Alkhabra. This is an open-access article distributed under the terms of the Creative Commons Attribution License (CC BY). The use, distribution or reproduction in other forums is permitted, provided the original author(s) and the copyright owner(s) are credited and that the original publication in this journal is cited, in accordance with accepted academic practice. No use, distribution or reproduction is permitted which does not comply with these terms.
*Correspondence: Sultan Hammad Alshammari, c3VsdGFuOTU3M0Bob3RtYWlsLmNvbQ==; c2guYWxzaGFtbWFyaUB1b2guZWR1LnNh