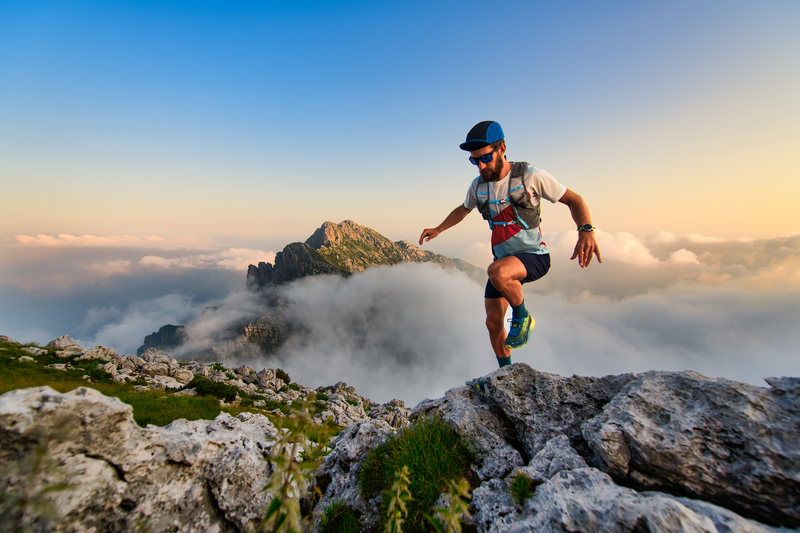
94% of researchers rate our articles as excellent or good
Learn more about the work of our research integrity team to safeguard the quality of each article we publish.
Find out more
ORIGINAL RESEARCH article
Front. Educ. , 16 October 2024
Sec. Digital Learning Innovations
Volume 9 - 2024 | https://doi.org/10.3389/feduc.2024.1461362
Feedback is an integral part of learning in higher education and is increasingly being provided to students via modern technologies like Large Language Models (LLMs). But students’ perception of feedback from LLMs vs. feedback from educators remains unclear even though it is an important facet of feedback effectiveness. Further, feedback effectiveness can be negatively influenced by various factors; For example, (not) knowing certain characteristics about the feedback provider may bias a student’s reaction to the feedback process. To assess perceptions of LLM feedback and mitigate the negative effects of possible biases, this study investigated the potential of providing provider-information about feedback providers. In a 2×2 between-subjects design with the factors feedback provider (LLM vs. educator) and provider-information (yes vs. no), 169 German students evaluated feedback message and provider perceptions. Path analyses showed that the LLM was perceived as more trustworthy than an educator and that the provision of provider-information led to improved perceptions of the feedback. Furthermore, the effect of the provider and the feedback on perceived trustworthiness and fairness changed when provider-information was provided. Overall, our study highlights the importance of further research on feedback processes that include LLMs due to their influential nature and suggests practical recommendations for designing digital feedback processes.
Feedback is a central component of learning in (higher) education (Hattie and Timperley, 2007; Wisniewski et al., 2020) and can influence different outcomes, such as a learner’s affective and motivational reactions as well as their performance (Henderson et al., 2019; Wisniewski et al., 2020). In this vein, feedback can promote the development of various skills, including written argumentation skills (Latifi et al., 2019; Fleckenstein et al., 2023). Argumentation skills become increasingly important in our society, as they allow for knowledge construction and perspective-taking and can guide learners when dealing with modern technologies (Federal Ministry of Education and Research, 2023; Redecker, 2017). In this context, (student) teachers have a unique role: They need to acquire argumentation skills themselves, and at the same time act as models and promote their students’ argumentation skills.
While feedback that helps learners hone argumentative skills is critical in our technological society, interestingly, the rise of certain modern technologies is also affecting feedback environments. For example, artificially intelligent (AI) systems, more specifically large language models (LMMs), enormously impact feedback environments, as they can be implemented to provide immediate feedback to learners (Brown et al., 2020; Chiu et al., 2023) and thereby save educators time and resources (Cavalcanti et al., 2021; Kasneci et al., 2023; Wilson et al., 2021).
Yet, a feedback process is rather complex and does not only comprise the feedback message and the feedback provider: As summarized by Panadero and Lipnevich (2022), feedback encompasses characteristics of the message, implementation, student, context, and agents. All these aspects can influence the effectiveness of feedback, which is complex in itself. Henderson et al. (2019) summarized three broad categories of feedback effectiveness: cognitive, affective, and relational. Thus, the feedback provider can potentially affect learners’ perceptions of the feedback and feedback provider and, in turn, affect learners’ performance. Because feedback processes are continuously developing and the complexity of feedback interactions partly explains why the effectiveness of feedback is highly variable (Panadero and Lipnevich, 2022; Wisniewski et al., 2020), the question arises as to whether differences exist in learners’ cognitive, affective, and/or relational reactions toward feedback provided by a human versus an AI-system, particularly an LLM.
Whether feedback is effective for a learner depends not only on the original feedback provider but also the providers’ individual characteristics, like expertise (Lechermeier and Fassnacht, 2018; Lucassen and Schraagen, 2011; Winstone et al., 2017). To prevent learners from having socially biased responses to feedback providers, anonymous feedback processes are often considered. However, such anonymity does not always prevent bias (e.g., Panadero and Alqassab, 2019). Further, for educators or LLMs, providing feedback anonymously can be rather unrealistic, because learners know whose classes they take, and data protection laws require at least minimal information about algorithms and their implementation. Therefore, here we investigate the opposite of anonymity: We explored whether providing additional information about the feedback provider (‘s characteristics) can promote feedback effectiveness.
Specifically, in a 2×2 between-subjects design with the factors feedback provider (educator vs. LLM) and provider information (yes vs. no), we investigated how to minimize learners’ biases related to the feedback provider by shedding light on the questions alluded to above: How might learners’ perceptions of feedback providers and the feedback itself vary based on (1) whether the provider is an educator or an LLM, and (2) whether information about the provider is available?
Feedback is indispensable in educational contexts and is seen as a promising strategy to improve various learning outcomes (Hattie and Timperley, 2007; Wisniewski et al., 2020). Particularly, written and elaborated feedback (in contrast to corrective feedback) plays a crucial role in promoting higher-order learning outcomes like argumentation skills (Van Der Kleij et al., 2011).
As generally defined, feedback includes information about several components from several sources that works best if learners actively engage with it (Lipnevich and Panadero, 2021, p. 25). Thus, feedback is not only about the feedback message itself. As summarized by Panadero and Lipnevich (2022), feedback constitutes characteristics of the message, implementation, student, context, and agents involved. All these elements are crucial for the feedback process and influence its effectiveness. Due to this complexity, the factors that negatively influence feedback effectiveness are hard to pin down, even though they exist (Kluger and DeNisi, 1996; Winstone et al., 2017; Wisniewski et al., 2020).
In this study, we consider the feedback provider as possible (negative) factor influencing feedback effectiveness, particularly because modern technology has made it, so feedback is now not only provided by humans, e.g., educators, but by AI-systems (Chiu et al., 2023). Furthermore, feedback processes are inherently social (Ajjawi and Boud, 2017), and learners’ social biases, e.g., those stemming from the feedback provider, seem to be worth closer investigation, as they have not been thoroughly researched so far (Panadero and Lipnevich, 2022).
One of the most important factors influencing the effectiveness of feedback seems to be the feedback provider (Ilgen et al., 1979; Panadero and Lipnevich, 2022; Winstone et al., 2017), which has hardly been researched (Lechermeier and Fassnacht, 2018; Panadero and Lipnevich, 2022). Traditionally, educators have been learners’ sole feedback providers, but next to practical reasons such as the lack of resources to provide adequate feedback to learners, educators’ hierarchy above learners, specifically related to their authority, expertise, and experience, can hinder the effectiveness of feedback. The hierarchy can, for example, prevent learners from asking for clarifications (Carless, 2006; Winstone et al., 2017), and educators’ feedback is seldom questioned (Lechermeier and Fassnacht, 2018), perhaps due to their expert status (Metzger et al., 2016). Overall, the interaction between the educator as the feedback provider and the learner as the feedback recipient seems to play a crucial role in feedback processes.
While educators are the traditional feedback sources for learners, (generative) AI-systems, e.g., LLMs, that offer feedback are emerging (Bozkurt, 2023; Chiu et al., 2023). Such modern technologies are increasingly implemented, for example, in educational administration, as chatbots, or even in assessment (Chiu et al., 2023). AI-systems rapidly developed (Zawacki-Richter et al., 2019), and now, thanks to technological developments in natural language processing, LLMs can give feedback on (short) textual answers [e.g., ArgueTutor (Wambsganss et al., 2021], AcaWriter [Knight et al., 2020), or even ChatGPT (OpenAI, 2023)]. Automated feedback itself is not new, but thus far was pre-programmed and less dialogic (e.g., Azevedo and Bernard, 1995). Compared with that, LLMs are AI-systems that have been trained on huge amounts of data and are capable of analyzing existing patterns in language and imitate as well as understand human language (Brown et al., 2020; Kasneci et al., 2023). Users can thus easily interact with LLMs by using their own natural language (Kasneci et al., 2023). This interaction highly resembles a human-human – or educator-student – interaction. In this vein, building on Reeves and Nass (1996), even though LLMs are clearly non-human, people tend to ascribe them human characteristics (e.g., trust) based on similar cues (e.g., expertise). There is evidence that LLM-and instructor-feedback align (Dai et al., 2023). However, LLMs can be biased by their developers, the training data, and/or any learning that occurs during the LLM’s lifecycle (i.e., aspects determining the LLM’s competence). This can lead to the LLM’s output, i.e., the feedback being false, biased, or untransparent (see Chang et al., 2023). These flaws can affect trust users have in the AI-system (Grassini, 2023; Kaur et al., 2022). All in all, the implementation of LLMs in (higher) education contexts has huge potential. In fact, educators are increasingly supported by LLMs to provide feedback to students, because these can, for example, promote self-regulated learning or save educators time and resources (Cavalcanti et al., 2021; Kasneci et al., 2023; Wilson et al., 2021).
One prerequisite of effective feedback interactions is the flawlessness of the human-computer/AI interaction. A flawless interaction requires AI-literate users (for more information see, for example, Long and Magerko, 2020) that trust the LLM and, at the same time, feel agentic and able to reflect on their use of the system (Khosravi et al., 2022). Additionally, to lead to progress, the interaction should be humanlike and empathetic (Grassini, 2023), a given in interactions with LLM (see above).
When assessing texts to provide feedback, both humans and AI-systems rely on their experience, but their approach differs: AI-systems have a clear statistical approach, e.g., they come to a decision on structure by counting paragraphs and length (e.g., Yang et al., 2023), which resembles a rubric. In this process, (most) AI-systems neglect the actual content and do not reliably provide correct information (Grassini, 2023). Humans, on the other hand, more intuitively evaluate the text, even if they use rubrics which could make them less credible. Alongside investigating the functionality and reliability of AI-systems, research on AI-systems in higher education (Chiu et al., 2023; Grassini, 2023), e.g., as providers of elaborate feedback, should be examined, as this development has the potential to influence the effectiveness of feedback (Panadero and Lipnevich, 2022).
As mentioned above, feedback providers and their characteristics can influence the effectiveness of feedback. Similar to the feedback process itself, the effectiveness of feedback is complex: It encompasses cognitive, affective, and relational aspects as summarized by Henderson et al. (2019). Thus, effective feedback not only involves learners using it and improving their performance (i.e., cognitive aspect), but effective feedback also requires the provider to be seen as trustworthy and/or the feedback message to be perceived as fair (i.e., relational and affective aspects). This view on feedback effectiveness, in line with the definition of feedback, highlights the often overlooked fact that learners, as the recipients of feedback, are active recipients, in that they actively engage with the feedback (Lipnevich et al., 2016; Lipnevich et al., 2021; Tsai, 2022; Winstone et al., 2017). Thus, how learners perceive the various elements (i.e., MISCA elements) of the feedback process, like the feedback provider and the feedback itself, is thus crucial for their engagement with the feedback (Van der Kleij and Lipnevich, 2021; Strijbos et al., 2021).
As learners are in the center of the feedback process (Panadero and Lipnevich, 2022), a crucial element that determines the effectiveness of such a process is how the learners perceive the feedback. Feedback message perceptions include cognitive, provider-cognitive, motivational, and/or affective reactions (Strijbos et al., 2021, p. 2) and, thus, ‘capture how students comprehend, perceive, and value a feedback message and how they experience and receive feedback’ (Van der Kleij and Lipnevich, 2021, p. 349). Feedback message perceptions are part of the effectiveness of feedback, as they determine how learners engage with the feedback (Van der Kleij and Lipnevich, 2021). As aspects of feedback effectiveness, feedback message perceptions can be influenced by any element of the process, i.e., the message, implementation, student, context, or agents (Panadero and Lipnevich, 2022). In this vein, learners’ perceptions of feedback can be influenced by the feedback provider and their characteristics (Van der Kleij and Lipnevich, 2021). For example, Dijks et al. (2018) investigated how the (peer) feedback provider’s perceived expertise affected the feedback recipients’ perceptions and found a positive link between these two, concluding that learners might be biased by their knowledge about the feedback provider. Similarly, Strijbos et al. (2010) also found evidence that (peer) feedback providers’ expertise influences perceptions of feedback. Thus, it seems likely that the feedback provider as well as knowledge about their characteristics influence how learners perceive feedback.
Because AI-systems will be implemented in educational contexts more and more to support educators and learners (Bozkurt, 2023; Chiu et al., 2023), it is crucial to examine how learners will react to these providers considering their different (power) relationships (see 2.2). Particularly important is understanding the extent to which learners trust the feedback provider (Carless, 2012; Davis and Dargusch, 2015; Ilgen et al., 1979; Winstone et al., 2017), as this is a prerequisite that helps learners decide whether to use the feedback (Boud and Molloy, 2013; Carless, 2006; Holmes and Papageorgiou, 2009; Carless, 2012; Davis and Dargusch, 2015). For example, feedback from trustworthy sources positively relates to feedback acceptance and motivation (see Lechermeier and Fassnacht, 2018).
For humans, trust constitutes perceived benevolence, integrity, and expertise (Hendriks et al., 2015). Since AI-systems are evaluated like humans, the same criteria can be applied to them (Reeves and Nass, 1996). Generally, the development of trust in the context of feedback is facilitated by the learner’s perception of certain characteristics about the feedback provider, such as their expertise, experience, or status (Hoff and Bashir, 2015; Lechermeier and Fassnacht, 2018; Lucassen and Schraagen, 2011; Van De Ridder et al., 2015). Further, trustworthiness can also be influenced by such observable cues (Kaplan et al., 2023).
While these concepts apply equally to humans and AI-systems, the development of trust in each of them differs (see Madhavan and Wiegmann, 2007). For humans, as the relationship between two people progresses, trustworthiness usually increases (Hoff and Bashir, 2015). By contrast, AI-systems often enjoy a positivity bias initially, meaning that people trust the system in the beginning due to, for example, its label (Langer et al., 2022) or assumed abilities and objectivity (Swiecki et al., 2022). In this vein, a previous study by Ruwe and Mayweg-Paus (2023) found that AI-systems were perceived as more trustworthy as humans after a first interaction. Learners’ interactions with AI-systems are also affected by the quality of the system (Cai et al., 2023), whereby students often have certain expectations toward AI-systems (like ChatGPT) which the system may or may not meet, thereby affecting learners’ behavior toward the system (Strzelecki, 2023). In education settings, both teachers and students have tended to meet AI-systems with skepticism (Chiu et al., 2023), as many are unsure about the benefits of AI (Clark-Gordon et al., 2019; Shin et al., 2020). In these cases, trust can develop with increasing interaction as learners get to know the system (Cai et al., 2023; Nazaretsky et al., 2022; Qin et al., 2020). Overall, the best-case scenario for such human-AI interactions is for people to have fruitful experiences with an AI-system and trust it just enough that they neither over-nor under-rely on the system’s decisions (Parasuraman and Riley, 1997).
In this vein, the concept of AI literacy (Ng et al., 2021) becomes important: To use an AI-system effectively, users need to understand the system, but this is difficult because the capabilities and functionalities of AI have developed faster than users’ comprehension of them (Zawacki-Richter et al., 2019). Since the ability to comprehend an AI-system is crucial for increasing trust (Nazaretsky et al., 2022; Qin et al., 2020), researchers have suggested that transparency and explainable AI (xAI) be employed (Cai et al., 2023; Khosravi et al., 2022; Memarian and Doleck, 2023), thereby improving trust and understanding and, in turn, interactions between humans and AI.
In (peer) feedback processes, anonymity is often considered beneficial to avoid social biases, but it does not always have the desired effects (e.g., Lu and Bol, 2007; Panadero and Alqassab, 2019). When working with educators or AI-systems, implementing anonymity is rather unrealistic: Learners know whose classes they take, and data protection laws require at least minimal information about algorithms. Furthermore, as mentioned above, users often want information about AI-systems for transparency reasons (Khosravi et al., 2022; Memarian and Doleck, 2023). Therefore, one approach might be to investigate the opposite of anonymity, namely whether giving additional information about a feedback provider actually promotes feedback effectiveness.
In the context of AI-systems, having information about the feedback provider (the AI-system) can be seen as an external aspect that affects situational trust (Hoff and Bashir, 2015) and is comparable to explainable AI (xAI). xAI aims at supporting users ‘to understand how, when, and why predictions are made’ (Kamath and Liu, 2021, p. 2), such that these explanations can establish trust and understanding as well as promote the use of AI-systems (Hoff and Bashir, 2015; Khosravi et al., 2022; Memarian and Doleck, 2023; Vössing et al., 2022). Therefore, the information about an AI-system can potentially influence whether and how the output of the system, e.g., feedback, is actually used by the user, e.g., the learner. However, providing information that is overwhelming or confusing reduces users’ understanding and can, thus, hamper transparency (Khosravi et al., 2022). One promising approach might be to offer users non-technical, global explanations about the general functioning, i.e., the competence, of the AI-system (Brdnik et al., 2023; Khosravi et al., 2022), such that learners can determine how the AI works, how it may affect them, and whether it is trustworthy (Holmes et al., 2021), even though there is no one-size-fits-all explanation in educational settings (Conijn et al., 2023).
Not only does having information about (the characteristics of) an AI-system increase trust in it, having information about human experts and their individual characteristics also increases trust in them and the information they provide, particularly in online contexts (Harris, 2012; Metzger, 2007; Flanagin et al., 2020). As outlined above, this is true for educators, whose perceived expertise (among other characteristics) influences the effectiveness of feedback (see 2.2 and 2.3.2).
In the context of this study, feedback literacy might play an important role because feedback literacy is said to diminish biases in learners’ reception of feedback (Carless and Boud, 2018). Additionally, feedback literacy involves strong critical thinking and reflection skills, and these skills are also essential for effectively dealing with the challenges brought by modern technologies like AI-systems (Alqahtani et al., 2023; Casal-Otero et al., 2023; Federal Ministry of Education and Research, 2023; Ng et al., 2021). According to Carless and Boud (2018), feedback literacy encompasses appreciating feedback, making judgments based on feedback, managing affect resulting from feedback, and taking action from feedback. Thus, one could assume that feedback-literate learners might manage their affective reaction to the feedback provider and prevent negative influences from getting in the way of engaging with the feedback information.
Building on the literature outlined above, we aimed to investigate the effect of providing provider-information about feedback providers as a way to avoid the pitfall of negative perceptions (in terms of feedback message and feedback provider perceptions) hindering feedback effectiveness, and we did this while also considering learners’ feedback literacy. We are thus asking whether providing background information about the feedback provider (i.e., educator vs. AI-system) influences learners’ perceptions of the feedback as well as the feedback provider, as these perceptions are known to influence the effectiveness of feedback. The framework underlying the study is illustrated in Figure 1. The study was pre-registered (see: https://aspredicted.org/N3H_JQ8).
We outlined (see 2.4) that having information about feedback providers influences not only the learner’s perceived trustworthiness of the feedback provider, but it also influences their perception of the feedback information itself. Those arguments led to our first hypothesis:
H1: We assume there will be a main effect of having provider-information about the feedback provider on learners’ perceptions of (a) the feedback and (b) the feedback provider (i.e., trustworthiness) compared to when learners have no information about the providers.
In 2.3.2, we briefly outlined the differences and similarities between humans and AI-systems regarding trustworthiness. We assume that even though educators are the traditional sources of feedback and hold a certain status, AI-systems still benefit from positivity bias. Accordingly, we derived our second hypothesis:
H2: We assume that (a) feedback from an LLM will be perceived more positively than feedback from a human and that (b) learners’ perceptions of the LLM as a feedback provider will be more positive than their perceptions of a humans as a feedback provider.
Since certain characteristics of the feedback provider also play a role (see 2.2), our third hypothesis involves the interaction between the feedback provider and their corresponding provider-information:
H3: We assume that having provider-information will more positively influence learners’ perceptions of (a) the feedback and (b) the feedback provider when this information is provided for the human than when it is provided for the LLM.
Lastly, some learner attributes influence whether and how much learners engage with the feedback process, such as feedback literacy (see 2.5). Because feedback literacy encompasses competences that allow a less biased evaluation of feedback, we explored whether feedback literacy affected learners’ reactions to the feedback to the and feedback provider.
Using G*Power (Faul et al., 2009) for MANOVA Special Effects/Interactions with α = 0.05, β = 0.95, and a medium-sized effect, a total sample of 65 participants was estimated. Participants were recruited via different channels (e.g., postings in the learning management system, using networks). In total, N = 462 German-speaking students (studying to be teachers) began participation in the study; N = 169 students finished the study, and their data were included in the analysis (included sample was 68.6% female, 0.18% diverse/not specified; Mage = 24.85, SDage = 6.74; 54.4% at bachelor’s degree level; 95.9% German native speakers).
The 2×2 between-subjects study with the factors feedback provider (educator vs. AI-system) and provider-information (yes vs. no) was implemented online (unipark.com by Questback EFS Survey). Participants were randomly assigned to one of the four experimental conditions.
Participants’ perceptions of the feedback providers’ trustworthiness were assessed with the Muenster Epistemic Trustworthiness Inventory (METI; Hendriks et al., 2015). The 16 items of the METI can be summarized in three subscales (i.e., expertise (seven items, α ≥ 0.94), integrity (five items, α ≥ 0.82), benevolence (four items, α ≥ 0.80)). The items were assessed on a seven-point semantic differential scale (using antonyms, e.g., 1 = professional vs. 7 = unprofessional (expertise), 1 = honest vs. 7 = dishonest (integrity), 1 = responsible vs. 7 = irresponsible (benevolence)).
To assess how participants evaluated the feedback, the Feedback Perceptions Questionnaire (FPQ; Strijbos et al., 2021) was applied. The 18 items were assessed on a 10-point bipolar scale ranging from 1 = fully disagree to 10 = fully agree. They are distributed across five subscales: fairness, α ≥ 0.87 (e.g., I would be satisfied with this feedback), usefulness, α ≥ 0.91 (e.g., I would consider this feedback useful), acceptance, α ≥ 0.83 (e.g., I would accept this feedback), willingness to improve, α ≥ 0.87 (e.g., I would be willing to improve my performance), and affect, α ≥ 0.85 (e.g., I would feel satisfied/content if I received this feedback on my revision).
For assessing participants’ feedback literacy, we employed five subscales of Zhan’s (2022) student feedback literacy scale: processing (e.g., ‘I am good at comprehending others’ comments’, α ≥ 0.76), enacting (e.g., ‘I am good at managing time to implement the useful suggestions of others’, α ≥ 0.68), appreciation (e.g., ‘I have realized that feedback from other people can make me recognize my learning strengths and weaknesses’, α ≥ 0.76), readiness (e.g., ‘I am always ready to receive hypercritical comments from others’, α ≥ 0.81), and commitment (e.g., ‘I am always willing to overcome hesitation to make revisions according to the comments I get’, α ≥ 0.70). The 20 items (four items each) were assessed on positively packed six-point Likert-type scales ranging from 1 (= strongly disagree) to 6 (= strongly agree).
To control for potential further influences of the participants’ characteristics (particularly on perceptions of the AI-system), we included demographic variables and collected information about participants’ experience with AI-systems (Kaplan et al., 2023). We had participants estimate their competence, experience, expertise, performance, and previous interactions with AI-systems in educational contexts (e.g., ‘I have sufficient competences to use an AI-system in educational contexts’) on a five-point Likert scale ranging from 1 = completely disagree to 5 = completely agree.
Furthermore, we wanted to gain insights into a potential positivity bias on the participants’ side. Therefore, we developed four items in accordance with Hoff and Bashir’s (2015) model to assess whether participants have dispositional, learned, internal or external situational trust in AI-systems (α ≥ 0.79).
After providing their informed consent and demographic information, participants received an introduction to the scenario: They saw a screenshot of a seemingly realistic interaction between an educator or an LLM and a learner depending on their experimental group (see Figures 2, 3). The screenshots in Figures 2, 3 show the educator and LLM conditions with provider-information. This information (on top of the screenshot) was missing in the no provider-information conditions. The human-human interactions were oriented on a common learning management system, while the human-AI interactions were oriented on ChatGPT (OpenAI, 2023). The feedback solely referred to structural, not contextual issues, and the feedback was designed to be neither positive nor negative. The fit between the text and the feedback was pilot tested (see below).
Building on research on xAI (see 2.4), the provider-information for the LLM was solely textual (Conijn et al., 2023) and addressed the LLM’s performance while not being too overwhelming (Khosravi et al., 2022). The provider-information was oriented on Conijn et al.’s (2023) global explanations but shortened and adapted. The provider-information about the human feedback provider described their expertise and was designed to align with the provider-information of the LLM.
After reviewing the screen with the feedback (and, for the corresponding conditions, the provider-information), participants were then asked to quantitatively and qualitatively give their perceptions of the feedback and the feedback provider as well as estimate their feedback literacy. More details on the procedure can be seen in Figure 4.
Prior to the experiment, we pilot tested the materials and the study with five people with educational backgrounds (60% female, Mage = 43.20, SDage = 22.05). The pilot tests verified the comprehensibility and design of the study and all of its materials.
The study complied with APA ethical standards for research with human subjects as well as the EC’s data protection act. All participants provided their informed consent and were debriefed about the purpose at the end of the survey. Dropping out was possible at any time without having to provide a reason. Participants were reimbursed with 10 €.
Using the lavaan package (Rosseel, 2012) in R studio (R Core Team, 2022), we built saturated path models (equivalent to regression analyses) to test our theoretically grounded model as outlined before and illustrated in Figure 5. We included dummy variables for our independent variables, using educators and no provider-information as the reference categories. Furthermore, we used a robust estimator (MLM) to account for minimal skewness in our data (Rosseel, 2012). We built one model with the overall means and one with the subscales for each hypothesis and set an α level of 0.05. The descriptive values of the dependent variables across the experimental groups can be found in Figures 6, 7.
Figure 5. Illustration of the theoretical background of the path models based on the literature review.
Figure 6. Overview over descriptive values of trustworthiness across all experimental groups. + Info, meta-information provided; − Info, no meta-information provided; METI, Muenster epistemic trustworthiness inventory; Exp, expertise; Ben, benevolence; Int, integrity.
Figure 7. Overview over descriptive values of feedback message perceptions across all experimental groups. FPQ, feedback perceptions questionnaire; FA, fairness; AF, affect, AC, acceptance; WI, willingness to improve; US, usefulness.
Accordingly, we built three models to test our hypotheses as stated above (see 2.6): Model A included the feedback provider and the provider information as independent variables and the overall values of feedback message and feedback provider perceptions as the dependent variables (see Supplementary Appendix Table A1). Instead of looking at the overall values, model B included all subscales as dependent variables (see Supplementary Appendix Table A2). These subscales remained the dependent variables in model C while including the feedback provider as independent variable and adding provider information as moderating variable (see Supplementary Appendix Table A3).
We assessed several other variables to get more insights into our setting and the experimental manipulations. The participants evaluated the setting as realistic (M = 2.36,1 SD = 1.38), reasonable (M = 2.50, SD = 1.32), appropriate (M = 2.76, SD = 1.42), and suitable (M = 2.87, SD = 1.38). The majority of participants (82.1%) did not think about a specific or known person or AI-system. Participants who received the provider-information agreed that they found it rather helpful (M = 4.542, SD = 1.59), clear (M = 4.44, SD = 1.58), and adequate (M = 4.29, SD = 1.48); They rather disagreed that the provider-information was overwhelming (M = 2.90, SD = 1.45), confusing (M = 2.92, SD = 1.64), and distracting (M = 2.78, SD = 1.43). This indicates a good quality of the explanations (Brdnik et al., 2023; Conijn et al., 2023; Khosravi et al., 2022).
Finally, we assessed participants’ content knowledge and their experience with, attitude toward, and trust in AI-systems in feedback contexts. Participants’ knowledge about the topic of the argumentative text was low (M = 1.61, SD = 0.84). According to participants’ self-evaluations, their competence (M = 2.82, SD = 1.73), knowledge (M = 3.15, SD = 1.86), and experience (M = 2.24, SD = 1.70) with AI-systems in feedback contexts were rather low, but they estimated their performance in such a setting as average (M = 3.93, SD = 1.34). Their expectations toward an AI-system in such a setting were rather high (M = 4.08, SD = 1.75).
There were no differences between the experimental groups except for experience [F(1, 167) = 4.19, p ≤ 0.05, R2 = 0.02], where the educator group (M = 2.50) reported more experience with AI-systems in feedback settings than the AI group (M = 1.96). There were no differences between the experimental groups regarding their attitudes toward AI-systems in feedback settings, which were rather positive (M = 4.31, SD = 0.91). This could indicate a slight positivity bias toward AI-systems in feedback contexts.
While there was no effect on trustworthiness, the availability of provider-information had a direct association with feedback message perceptions. We found that having provider-information improved participants’ feedback message perceptions overall (β = 0.53, p ≤ 0.05, model A, Figure 8) and positively influenced participants’ affective reactions to the feedback (β = 0.79, p ≤ 0.01, model B, Figure 9). The effect of provider-information and feedback providers was small according to Cohen (1988)3 (see Figures 8, 9).
Figure 9. Illustration of model B. Only significant associations are displayed. Covariances Shown in Table 1.
There was no evidence of significant associations between the feedback provider and feedback message perceptions. Nonetheless, the feedback provider was associated with trustworthiness: The LLM was perceived as more trustworthy than the educator overall (β = 0.58, p ≤ 0.001, model A, Figure 8) as well as on all three subscales (model B, Figure 9) expertise (β = 0.74, p ≤ 0.001), benevolence (β = 0.62, p ≤ 0.001), and integrity (β = 0.39, p ≤ 0.05).
We did not find a significant interaction effect of feedback provider and provider-information on the overall scores of feedback message perceptions and trustworthiness. Looking at the subscales, we found that the effect of the feedback provider on fairness changed when adding provider-information (see model C, Figure 10): The interaction of feedback provider and provider-information was significant regarding fairness (β = 1.24, p ≤ 0.05) and expertise (β = 1.24, p ≤ 0.05). Follow-up t-tests revealed that without provider information, there was a significant difference between LLMs (M = 7.07, SD = 2.13) and educators (M = 8.06, SD = 2.10) regarding fairness (t = 2.52, p ≤ 0.05, d = 0.53). When adding provider-information, this effect vanished (MLLM = 8.25, MEducator = 8.06, t = − 0.44, p = 0.66, d = 0.09). In addition, there was a significant difference in participants’ perceptions of the feedback providers’ expertise without provider-information (MLLM = 3.34, MEducator = 2.19, t = − 4.46, p ≤ 0.001, d = 0.94), and this disappeared when provider-information was provided (MLLM = 2.62, MEducator = 2.31, t = −1.29, p = 0.201, d = 0.28).
Overall, the models illustrating the interaction of the feedback provider and provider-information explain more variance than those excluding the interaction: When including the moderation, 2.4% more variance of fairness could be explained, and 2.9% more variance of expertise could be explained. These indicate small effects (Cohen, 1988).
Finally, we explored potential effects of feedback literacy on the associations under investigation. While neither model showed that feedback literacy significantly moderated the effects of feedback provider and provider-information on feedback message perceptions and trustworthiness, after running MANOVAs we found significant effects on overall feedback message perceptions and trustworthiness as well as on some subscales. Accordingly, with increases in feedback literacy, we found increases in feedback message perceptions [Figure 11; F(1, 167) = 10.61, p ≤ 0.01, R2 = 0.05] but decreases in trustworthiness [Figure 12; F(1, 167) = 8.22, p ≤ 0.01, R2 = 0.04]. A closer look at the subscales revealed the same pattern as in Figures 11, 12. The effects on expertise [F(1, 167) = 3.83, p = 0.052] and acceptance [F(1, 167) = 3.81, p = 0.053] were not significant though, meaning that feedback literacy did not influence these two subscales. Overall, the data show that feedback literacy does play a role in explaining learners’ perceptions of feedback and of feedback providers.
Figure 11. Plots of relationships between feedback literacy and feedback message perceptions. FPQ, feedback perceptions questionnaire; FA, fairness; AF, affect; AC, acceptance; US, usefulness; WI, willingness to improve.
To summarize, we found evidence that the type of feedback provider and the presence of provider-information about the feedback provider influence the effectiveness of the feedback, which was operationalized as learners’ perceptions of the feedback and the feedback provider. Furthermore, we found evidence that feedback literacy plays an important role for feedback effectiveness. First, feedback providers and corresponding provider-information were directly associated with learners’ perceptions of the feedback and feedback providers. While an LLM was perceived as more trustworthy than educators, the presence of provider-information improved participants’ perceptions of the feedback. Second, the presence of provider-information influenced the effect of the feedback provider: Without provider-information, feedback from an educator was perceived as fairer than from an LLM, but when the provider-information was present, AI-feedback was perceived as fairer. Similarly, there was a significant difference in trustworthiness regarding expertise without provider-information, such that an LLM were perceived as more trustworthy. Yet, when provider-information was present, the LLM and educator were perceived as similarly trustworthy regarding their expertise. Finally, we explored the role of feedback literacy and found that it did influence the way learners reacted to the feedback process. We found that with increases in feedback literacy, participants’ perceptions of the feedback improved, while the perceived trustworthiness of the feedback providers decreased.
The first hypothesis was confirmed with respect to the effect of having provider-information on learners’ perceptions of the feedback message (H1a). In line with the literature, the type of feedback provider as well as having knowledge about them influenced how learners reacted to feedback and, thus, how they perceived it. In the complex feedback process, various elements influence the effectiveness of feedback, including having access to any information that improves the relationship between the learner and the feedback provider (Panadero and Lipnevich, 2022; Winstone et al., 2017). Another reason that feedback message perceptions were positively influenced by provider-information could be that the presence of the provider-information improved transparency. As a whole, provider-information might help learners understand the feedback message (see 2.4).
In contrast, the second part of the first hypothesis (H1b) was not confirmed: There was no effect of provider-information on participants’ perceptions of the feedback provider, i.e., trustworthiness. This is a surprising finding, particularly because provider-information moderated the effect of the feedback provider on trustworthiness (see Hypothesis 3). Supposedly, provider-information that gives insights into the expertise and competence of the feedback provider should be beneficial for how the provider is perceived. Possibly, the provider-information presented in this study did not sufficiently emphasize the characteristics of the feedback provider that informed participants about their trustworthiness, such as expertise, experience, and status (Hoff and Bashir, 2015; Lechermeier and Fassnacht, 2018; Lucassen and Schraagen, 2011; Van De Ridder et al., 2015). In this vein, regarding provider-information, there is no one-size-fits-all (Conijn et al., 2023) – which also becomes evident below in the discussion on Hypothesis 3. Conclusively, the rather global information we provided may not be suitable to increase trustworthiness. In addition, participants’ individual characteristics could help explain the lack of an effect. These characteristics are crucial in context with trustworthiness (Kaplan et al., 2023). Keeping in mind the trust trajectories (see 2.3.2), the positivity bias we found (see Hypothesis 2), could be undermined by the additional information. With the additional information about the LLM as provider, trust in it decreases while this information adds to the trustworthiness of educators. The perceptions of the providers are thus approximating.
Overall, we can conclude that provider-information about the feedback provider influenced participants’ reactions to the feedback process, i.e., the effectiveness of feedback.
In line with our assumption, the type of feedback provider influenced participants’ perceptions of them (H2b). More specifically, the LLM was perceived as more trustworthy than the educator, a finding that confirms the results of one of our previous studies (Ruwe and Mayweg-Paus, 2023). Overall, the literature agrees that the feedback provider influences the effectiveness of the feedback (Panadero and Lipnevich, 2022; Winstone et al., 2017). The fact that an LLM was again perceived as more trustworthy than a human can be explained by our sample’s characteristics: The participants in this study did not have much experience with AI-systems, but they had high expectations of them and rather positive attitudes. This could indicate a potential positivity bias, which our data confirms. According to the positivity bias, people have high initial trust in AI-systems due to various prejudices, and this decreases after repeated interactions with the system (see 2.3.2).
On the other hand, our assumption that the feedback provider influenced participants’ perceptions of the feedback (H2a) was not confirmed. In previous studies (e.g., Dijks et al., 2018; Strijbos et al., 2010) the expertise of the feedback provider, i.e., a key characteristic of the feedback provider, was found to influence feedback message perceptions. Even though a key characteristic of the feedback provider is closely related to the feedback provider themself, they are not the same thing, which could explain the absence of a significant association here. Particularly, against the background of provider-information having a moderating effect (H3a), this explanation might be true, as the purpose of the provider-information was to emphasize the characteristics of the feedback provider.
In conclusion, feedback providers influence the effectiveness of feedback in terms of learners’ reactions to the feedback process, and this finding agrees with the literature on characterizing the feedback provider as an important influence on feedback effectiveness (e.g., Panadero and Lipnevich, 2022; Winstone et al., 2017).
For fairness, we found evidence against the assumed relationship (H3a): The educator’s feedback was perceived as less fair than feedback generated by an alleged LLM when provider-information was present. Without provider-information, feedback from the educator was perceived as fairer than that of the LLM. As outlined above, information about the specific characteristics of a feedback provider seems to influence how learners perceive the feedback (Dijks et al., 2018; Strijbos et al., 2010). Furthermore, this finding is in line with literature about xAI, which aims at increasing transparency and fairness (see 2.4). Still, it is interesting that the educator did not benefit from the presence of provider-information regarding the perceived fairness of their feedback. Possibly, the content of the provider-information could have affected whether the educator was seen as objective.
The assumed relationship of provider-information on trustworthiness (H3b) was confirmed: Trustworthiness regarding the educator’s expertise increased when provider-information was present and, thus, approached the same level as trust in the LLM’s expertise. Thus, the presence of provider-information benefited the educator’s trustworthiness. This finding may be related to the positivity bias and the varying trust trajectories between humans and AI-systems: People are predisposed to trust an AI-system initially, but once they interact with it more, their trust decreases. On the contrary, trust in humans increases with repeated interactions. In this vein, providing information about the educator could have sped up the process of getting to know the feedback provider and, subsequently, led to higher trust. For AI-systems, it would be interesting to compare different explanations and different systems, because the characteristics of the system, its training data, and its developers can influence how users perceive the system (Kaur et al., 2022).
Our exploratory investigation of whether participants’ feedback literacy affected their perceptions revealed interesting insights. Potentially, feedback literacy might allow learners to neither over-nor under-rely on feedback (providers) and accurately receive and engage with the feedback.
Increases in feedback literacy went hand in hand with increases in feedback message perceptions. Thus, feedback literacy did support learners in evaluating the feedback and planning their engagement with it. On the other hand, increases in feedback literacy also went hand in hand with decreases in trustworthiness of the feedback provider. This decrease in trustworthiness along with the increase in feedback message perceptions could mean that learners with higher feedback literacy engage with feedback in a way that is more independent of the feedback provider. Thus, these learners may rely less on potential biases related to the feedback provider and are more focused on the feedback itself.
Building on these findings, here we draw theoretical implications. Overall, the study confirmed that feedback processes are complex, and their elements are intertwined. This complexity does not disappear when AI-systems like LLMs are involved, and such systems bring their own complex backgrounds. It is worth diving deeper into feedback processes involving AI-systems and looking at the various relations in more detail.
Regarding provider-information, the results showed that its effect depends on the feedback provider. In this context, more research on what types of provider-information work for whom is important. We thus agree with authors like Conijn et al. (2023), who have stated that there is no one-size-fits-all explanation. In this vein, provider-information is closely related to the feedback provider, leading to interesting questions: Why do the effects of provider-information and feedback providers differ regarding feedback effectiveness? For future research, it would be interesting to investigate the underlying mechanisms and the value that learners place on the different elements of the feedback process.
Finally, we point toward the importance of feedback literacy. Further research should investigate how to promote feedback literacy or how to activate it in feedback processes to avoid biased feedback interactions. In this context, research on further provider-competences that allow reflective engagement with feedback (processes) seems promising and inevitable.
Our findings can also be used to infer practical implications. First, giving provider-information about feedback providers should be considered, as this information improves learners’ perceptions of the feedback and also influences the effect of the feedback provider. In this vein, it is important to consider that the effects of provider-information might differ with different content and for different feedback providers. Another aspect to strongly consider is how personal factors, e.g., the personal relationship between the learner and the feedback provider, influence trustworthiness. Second, positivity biases and stereotypes toward AI-systems should be considered when implementing them in feedback processes. No learner should blindly trust AI-systems. This leads directly to our third implication, which is that feedback literacy should be promoted to encourage learners to engage with the feedback (process) in a reflective manner. Conclusively, the importance of a trustworthy relationship between students and feedback providers should be emphasized. Such relationships should be based on trust and enable an open and critical engagement with the feedback process.
For various reasons, the results and implications derived from this study should be interpreted with caution. The sample is quite specific and encompassed only German (speaking) students training to be teachers. These participants had varying individual characteristics (like different levels of feedback literacy) that influenced their responses, and their culture also influenced their trustworthiness assessments, particularly in the context of AI-systems (Kaplan et al., 2023). The transferability of the findings to other feedback scenarios is further limited by the feedback message that was specifically related to the argumentative structure and not to the content. Furthermore, we mentioned that personal relationships are critically important in feedback processes, and their power should not be underestimated. Yet, since the data were collected in a fictional study where personal relationships were not relevant, field studies could derive different results. In this vein, another limitation related to the methodology is that the subscale ‘Enacting’ used for assessing feedback literacy may not have been reliable. Furthermore, this study only covered a small snippet of the feedback process, including the design of the process as well as the effectiveness of feedback. Since feedback environments are (increasingly) complex, it is important to look at effects of the specific aspects of individual feedback interactions.
To conclude, our study found a difference in participants’ perceptions of the feedback and the feedback provider, and these differences at least partly depend on the feedback provider and whether provider-information was present. While provider-information was associated with increases in feedback message perceptions, we found a link between feedback providers and their trustworthiness in a way that benefitted LLMs. Furthermore, provider-information moderated the effect of the feedback provider on the effectiveness of feedback. Finally, our findings suggest that feedback literacy might play an important role in supporting learners when they reflectively engage with the feedback process. Based on these findings, we outlined many potential starting points for future research and also offered recommendations for practitioners regarding the design of feedback processes.
The datasets presented in this article are not readily available because participants were informed their data remains confidential. Requests to access the datasets should be directed to dGhlcmVzYS5ydXdlQGh1LWJlcmxpbi5kZQ==.
The studies involving humans were approved by Humboldt-Universität zu Berlin. The studies were conducted in accordance with the local legislation and institutional requirements. The participants provided their written informed consent to participate in this study.
TR: Conceptualization, Formal analysis, Investigation, Methodology, Writing – original draft, Writing – review & editing. EM-P: Conceptualization, Funding acquisition, Project administration, Supervision, Writing – original draft, Writing – review & editing.
The author(s) declare that financial support was received for the research, authorship, and/or publication of this article. This study was funded by the Einstein Centre Digital Future (ECDF).
We thank our colleagues for their support. We would also like to thank the reviewers for their valuable input which helped improve our manuscript.
The authors declare that the research was conducted in the absence of any commercial or financial relationships that could be construed as a potential conflict of interest.
All claims expressed in this article are solely those of the authors and do not necessarily represent those of their affiliated organizations, or those of the publisher, the editors and the reviewers. Any product that may be evaluated in this article, or claim that may be made by its manufacturer, is not guaranteed or endorsed by the publisher.
The Supplementary material for this article can be found online at: https://www.frontiersin.org/articles/10.3389/feduc.2024.1461362/full#supplementary-material
1. ^The items were assessed as antonyms ranging from 1 = positive manifestation to 7 = negative manifestation.
2. ^The items were assessed on a scale from 1 = completely disagree to 7 = completely agree.
3. ^The benchmarks for R2 are small effect = 0.02, medium effect = 0.13, large effect = 0.26.
Ajjawi, R., and Boud, D. (2017). Researching feedback dialogue: An interactional analysis approach. Assess. Eval. High. Educ. 42, 252–265. doi: 10.1080/02602938.2015.1102863
Alqahtani, T., Badreldin, H. A., Alrashed, M., Alshaya, A. I., Alghamdi, S. S., Bin Saleh, K., et al. (2023). The emergent role of artificial intelligence, natural learning processing, and large language models in higher education and research. Res. Soc. Adm. Pharm. 19, 1236–1242. doi: 10.1016/j.sapharm.2023.05.016
Azevedo, R., and Bernard, R. M. (1995). A meta-analysis of the effects of feedback in computer-based instruction. J. Educ. Comput. Res. 13, 111–127. doi: 10.2190/9LMD-3U28-3A0G-FTQT
Boud, D., and Molloy, E. (2013). Rethinking models of feedback for learning: The challenge of design. Assess. Eval. High. Educ. 38, 698–712. doi: 10.1080/02602938.2012.691462
Bozkurt, A. (2023). Generative artificial intelligence (AI) powered conversational educational agents: The inevitable paradigm shift. Asian J. Distance Educ. 18. doi: 10.5281/zenodo.7716416
Brdnik, S., Podgorelec, V., and Šumak, B. (2023). Assessing Perceived Trust and Satisfaction with Multiple Explanation Techniques in XAI-Enhanced Learning Analytics. Electronics 12:2594. doi: 10.3390/electronics12122594
Brown, T., Mann, B., Ryder, N., Subbiah, M., Kaplan, J. D., Dhariwal, P., et al. (2020). Language Models are Few-Shot Learners, arXiv.org. Available at: https://arxiv.org/abs/2005.14165
Cai, Q., Lin, Y., and Yu, Z. (2023). Factors Influencing Learner Attitudes Towards ChatGPT-Assisted Language Learning in Higher Education. Int. J. Hum. Comp. Interact. doi: 10.1080/10447318.2023.2261725
Carless, D. (2006). Differing perceptions in the feedback process. Stud. High. Educ. 31, 219–233. doi: 10.1080/03075070600572132
Carless, D. (2012). “Trust and its role in facilitating dialogic feedback” in Feedback in higher and professional education. eds. I. D. Boud and E. Molloy (Routledge), 90–103.
Carless, D., and Boud, D. (2018). The development of student feedback literacy: Enabling uptake of feedback. Assess. Eval. High. Educ. 43, 1315–1325. doi: 10.1080/02602938.2018.1463354
Casal-Otero, L., Catala, A., Fernández-Morante, C., Taboada, M., Cebreiro, B., and Barro, S. (2023). AI literacy in K-12: a systematic literature review. Int. J. STEM Educ. 10:29. doi: 10.1186/s40594-023-00418-7
Cavalcanti, M. C., Ferreira, J. M., and Gomes, I. (2021). Automatic feedback in online learning environments: A systematic literature review. Comp. Educ. 2:100027. doi: 10.1016/j.caeai.2021.100027
Chang, D. H., Lin, M. P.-C., Hajian, S., and Wang, Q. Q. (2023). Educational Design Principles of Using AI Chatbot That Supports Self-Regulated Learning in Education: Goal Setting, Feedback, and Personalization. Sustainability, 15:12921. doi: 10.3390/su151712921
Chiu, T., Xia, Q., Zhou, X., Chai, C. S., and Cheng, M. (2023). Systematic literature review on opportunities, challenges, and future research recommendations of artificial intelligence in education. Comp. Educ. 4:100118. doi: 10.1016/j.caeai.2022.100118
Clark-Gordon, C. V., Bowman, N. D., Hadden, A. A., and Frisby, B. N. (2019). College instructors and the digital red pen: An exploratory study of factors influencing the adoption and non-adoption of digital written feedback technologies. Comput. Educ. 128, 414–426. doi: 10.1016/j.compedu.2018.10.002
Conijn, R., Kahr, P., and Snijders, C. (2023). The Effects of Explanations in Automated Essay Scoring Systems on Student Trust and Motivation. J. Learn. Anal. 10, 37–53. doi: 10.18608/jla.2023.7801
Dai, W., Lin, J., Jin, F., Li, T., Tsai, Y. S., Gašević, D., et al. (2023). Can Large Language Models Provide Feedback to Students? A Case Study on ChatGPT. doi: 10.35542/osf.io/hcgzj
Davis, S. E., and Dargusch, J. M. (2015). Feedback, Iterative Processing and Academic Trust–Teacher Education Students' Perceptions of Assessment Feedback. Aust. J. Teach. Educ. 40. doi: 10.14221/ajte.2015v40n1.10
Dijks, M., Brummer, L., and Kostons, D. (2018). The anonymous reviewer: the relationship between perceived expertise and the perceptions of peer feedback in higher education. Assess. Eval. High. Educ. 43, 1258–1271. doi: 10.1080/02602938.2018.1447645
Faul, F., Erdfelder, E., Buchner, A., and Lang, A.-G. (2009). Statistical power analyses using G*Power 3.1: Tests for correlation and regression analyses. Behav. Res. Methods 41, 1149–1160. doi: 10.3758/BRM.41.4.1149
Federal Ministry of Education and Research. Bundesministerium für Bildung und Forschung (2023). BMBF-Aktionsplan Künstliche Intelligenz. Neue Herausforderungen chancenorientiert angehen. Bundesministerium für Bildung und Forschung, Referat Künstliche Intelligenz.
Flanagin, A. J., Winter, S., and Metzger, M. J. (2020). Making sense of credibility in complex information environments: the role of message sidedness, information source, and thinking styles in credibility evaluation online. Inf. Commun. Soc. 23, 1038–1056. doi: 10.1080/1369118X.2018.1547411
Fleckenstein, J., Liebenow, L. W., and Meyer, J. (2023). Automated feedback and writing: a multi-level meta-analysis of effects on students’ performance. Front. Artif. Intell. 6:1162454. doi: 10.3389/frai.2023.1162454
Grassini, S. (2023). Shaping the Future of Education: Exploring the Potential and Consequences of AI and ChatGPT in Educational Settings. Educ. Sci. 13:692. doi: 10.3390/educsci13070692
Hattie, J., and Timperley, H. (2007). The power of feedback. Rev. Educ. Res. 77, 81–112. doi: 10.3102/003465430298487
Henderson, M., Ryan, T., and Phillips, M. (2019). The challenges of feedback in higher education. Assess. Eval. High. Educ. 44, 1237–1252. doi: 10.1080/02602938.2019.1599815
Hendriks, F., Kienhues, D., and Bromme, R. (2015). Measuring Laypeople's Trust in Experts in a Digital Age: The Muenster Epistemic Trustworthiness Inventory (METI). PLoS One 10:e0139309. doi: 10.1371/journal.pone.0139309
Hoff, K. A., and Bashir, M. (2015). Trust in automation: Integrating empirical evidence on factors that influence trust. Hum. Factors 57, 407–434. doi: 10.1177/0018720814547570
Holmes, K., and Papageorgiou, G. (2009). Good, bad and insufficient: Students’ expectations, perceptions and uses of feedback. J. Hosp. Leis. Sport Tour. Educ. 8, 85–96. doi: 10.3794/johlste.81.183
Holmes, W., Porayska-Pomsta, K., Holstein, K., Sutherland, E., Baker, T., Buckingham Shum, S., et al. (2021). Ethics of AI in education: Towards a community-wide framework. Int. J. Artif. Intell. Educ. 32, 504–526. doi: 10.1007/s40593-021-00239-1
Ilgen, D. R., Fisher, C. D., and Taylor, M. S. (1979). Consequences of individual feedback on behavior in organizations. J. Appl. Psychol. 64, 349–371. doi: 10.1037/0021-9010.64.4.349
Kamath, U., and Liu, J. (2021). Explainable artificial intelligence: An introduction to interpretable machine learning : Springer.
Kaplan, A. D., Kessler, T. T., Brill, J. C., and Hancock, P. A. (2023). Trust in Artificial Intelligence: Meta-Analytic Findings. Hum. Factors 65, 337–359. doi: 10.1177/00187208211013988
Kasneci, E., Sessler, K., Küchemann, S., Bannert, M., Dementieva, D., Fischer, F., et al. (2023). ChatGPT for good? On opportunities ans challenges of large language models for education. Learning and Individual Differences, 103:102274. doi: 10.1016/j.lindif.2023.102274
Kaur, D., Uslu, S., Rittichier, K., and Durresi, A. (2022). Trustworthy Artificial Intelligence: A Review. ACM Comput. Surv. 55, 1–38. doi: 10.1145/3491209
Khosravi, H., Buckingham Shum, S., Chen, G., Conati, C., Tsai, Y.-S., Kay, J., et al. (2022). Explainable Artificial Intelligence in education. Comp. Educ. 3:100074. doi: 10.1016/j.caeai.2022.100074
Kluger, A. N., and DeNisi, A. (1996). The Effects of Feedback Interventions on Performance: A Historical Review, a Meta-Analysis, and a Preliminary Feedback Intervention Theory. Psychol. Bull. II9, 254–284. doi: 10.1037/0033-2909.119.2.254
Knight, S., Shibani, A., Abel, S., Gibson, A., Ryan, P., Sutton, N., et al. (2020). AcaWriter: A learning analytics tool for formative feedback on academic writing. J. Writing Res. 12, 141–186. doi: 10.17239/jowr-2020.12.01.06
Langer, M., Hunsicker, T., Feldkamp, T., König, C. J., and Grgić-Hlača, N. (2022). Look! It’s a Computer Program! It’s an Algorithm! It’s AI!”: Does Terminology Affect Human Perceptions and Evaluations of Algorithmic Decision-Making Systems? CHI '22 :581. doi: 10.1145/3491102.3517527
Latifi, S., Noroozi, O., Hatami, J., and Biemans, H. J. A. (2019). How does online peer feedback improve argumentative essay writing and learning? Innov. Educ. Teach. Int. 58, 195–206. doi: 10.1080/14703297.2019.1687005
Lechermeier, J., and Fassnacht, M. (2018). How do performance feedback characteristics influence recipients’ reactions? A state-of-the-art review on feedback source, timing, and valence effects. Manag. Rev. Quart. 68, 145–193. doi: 10.1007/s11301-018-0136-8
Lipnevich, A. A., Berg, D., and Smith, J. K. (2016). “Toward a Model of Student Response to Feedback” in Human Factors And Social Conditions In Assessment. eds. G. T. L. Brown and L. Harris (Routledge), 169–185.
Lipnevich, A. A., Gjicali, K., Asil, M., and Smith, J. K. (2021). Development of a measure of receptivity to instructional feedback and examination of its links to personality. Personal. Individ. Differ. 169:110086. doi: 10.1016/j.paid.2020.110086
Lipnevich, A. A., and Panadero, E. (2021). A Review of Feedback Models and Theories: Descriptions, Definitions, and Conclusions. Front. Educ. 6. doi: 10.3389/feduc.2021.720195
Long, D., and Magerko, B. (2020). What is AI literacy? Competencies and design considerations. In Proceedings of the 2020 CHI conference on human factors in computing systems. 1–16. doi: 10.1145/3313831.3376727
Lu, R., and Bol, L. (2007). A Comparison of Anonymous versus Identifiable E-Peer Review on College Student Writing Performance and the Extent of Critical Feedback. J. Interact. Online Learn. 6, 100–115.
Lucassen, T., and Schraagen, J. M. (2011). Factual accuracy and trust in information: The role of expertise. J. Am. Soc. Inf. Sci. Technol. 62, 1232–1242. doi: 10.1002/asi.21545
Madhavan, P., and Wiegmann, D. A. (2007). Similarities and differences between human–human and human–automation trust: an integrative review. Theor. Issues Ergon. Sci. 8, 277–301. doi: 10.1080/14639220500337708
Memarian, B., and Doleck, T. (2023). Fairness, Accountability, Transparency, and Ethics (FATE) in Artificial Intelligence (AI) and higher education: A systematic review. Comp. Educ. 5:100152. doi: 10.1016/j.caeai.2023.100152
Metzger, M. (2007). Making sense of credibility on the Web: Models for evaluating online information and recommendations for future research. J. Am. Soc. Inf. Sci. Technol. 58, 2078–2091. doi: 10.1002/asi.20672
Metzger, M., Flanagin, A., Eyal, K., Lemus, D., and Mccann, R. (2016). Credibility for the 21st Century: Integrating Perspectives on Source, Message, and Media Credibility in the Contemporary Media Environment. Ann. Int. Commun. Assoc. 27, 293–335. doi: 10.1080/23808985.2003.11679029
Nazaretsky, T., Cukurova, M., and Alexandron, G. (2022). “An instrument for measuring teachers’ trust in AI-based educational technology” in Proceedings of the 12th International Conference on Learning Analytics and Knowledge (LAK’22) (ACM Press), 56–66.
Ng, D. T. K., Leung, J. K. L., Chu, S. K. W., and Qiao, M. S. (2021). Conceptualizing AI literacy: An exploratory review. Comput. Educ. 2:100041. doi: 10.1016/j.caeai.2021.100041
OpenAI (2023). GPT-3: Language Models for Text Generation. OpenAI. Available at: https://openai.com/research/gpt-3
Panadero, E., and Alqassab, M. (2019). An empirical review of anonymity effects in peer assessment, peer feedback, peer review, peer evaluation and peer grading. Assess. Eval. High. Educ. 44, 1253–1278. doi: 10.1080/02602938.2019.1600186
Panadero, E., and Lipnevich, A. A. (2022). A Review of Feedback Models and Typologies: Towards an Integrative Model of Feedback Elements. Educ. Res. Rev. 35:100416. doi: 10.1016/j.edurev.2021.100416
Parasuraman, R., and Riley, V. (1997). Humans and automation: Use, misuse, disuse, abuse. Hum. Factors 39, 230–253. doi: 10.1518/001872097778543886
Qin, F., Li, K., and Yan, J. (2020). Understanding user trust in artificial intelligence-based educational systems: Evidence from China. Br. J. Educ. Technol. 51, 1693–1710. doi: 10.1111/bjet.12994
R Core Team (2022). R: A language and environment for statistical computing. Vienna, Austria: R Foundation for Statistical Computing.
Redecker, C. (2017). European framework for the digital competence of educators: DigCompEdu (No. JRC107466). Joint Research Centre (Seville site).
Reeves, B., and Nass, C. (1996). The media equation: How people treat computers, television, and new media like real people and places : Cambridge University Press.
Rosseel, Y. (2012). lavaan: An R package for structural equation modeling. Amer. Stat. Assoc. 48, 1–36.
Ruwe, T., and Mayweg-Paus, E. (2023). Your Argumentation is Good, says the AI vs Humans - The Role of Feedback Providers and Personalized Language for Feedback Effectiveness. Computers \u0026amp; Education: Artificial Intelligence, 5. doi: 10.1016/j.caeai.2023.100189
Shin, D., Zhong, B., and Biocca, F. (2020). Beyond user experience: What constitutes algorithmic experiences. Int. J. Inf. Manag. 52, 102061–102011. doi: 10.1016/j.ijinfomgt.2019.102061
Strijbos, J.-W., Narciss, S., and Dünnebier, K. (2010). Peer feedback content and sender’s competence level in academic writing revision tasks: Are they critical for feedback message perceptions and efficiency? Unravel. Peer Assess. 20, 291–303. doi: 10.1016/j.learninstruc.2009.08.008
Strijbos, J. W., Pat-El, R., and Narciss, S. (2021). Structural validity and invariance of the feedback message perceptions questionnaire. Stud. Educ. Eval. 68:100980. doi: 10.1016/j.stueduc.2021.100980
Strzelecki, A. (2023). To use or not to use ChatGPT in higher education? Study Students’ acceptance and use of technology. Interact. Learn. Environ., 1–14. doi: 10.1080/10494820.2023.2209881
Swiecki, Z., Khosravi, H., Chen, G., Martinez-Maldonado, R., Lodge, J. M., Milligan, S., et al. (2022). Assessment in the age of artificial intelligence. Comp. Educ. 3:100075. doi: 10.1016/j.caeai.2022.100075
Tsai, Y. (2022). “Why feedback literacy matters for learning analytics” in Proceedings of the 16th International Conference of the Learning Sciences-ICLS 2022 (pp. 27–34). International Society of the Learning Sciences. eds. C. Chinn, E. Tan, C. Chan, and Y. Kali. doi: 10.48550/arXiv.2209.00879
Van De Ridder, J. M. M., Berk, F. C. J., Stokking, K. M., and Ten Cate, O. T. J. (2015). Feedback providers' credibility impacts students' satisfaction with feedback and delayed performance. Med. Teach. 37, 767–774. doi: 10.3109/0142159x.2014.970617
Van der Kleij, F. M., and Lipnevich, A. A. (2021). Student perceptions of assessment feedback: a critical scoping review and call for research. Educ. Assess. Eval. Account. 33, 345–373. doi: 10.1007/s11092-020-09331-x
Van der Kleij, F. M., Timmers, C. F., and Eggen, T. (2011). The effectiveness of methods for providing written feedback through a computer-based assessment for learning: a systematic review. CADMO 1, 21–38. doi: 10.3280/CAD2011-001004
Vössing, M., Kühl, N., Lind, M., and Satzger, G. (2022). Designing Transparency for Effective Human-AI Collaboration. Inf. Syst. Front. 24, 877–895. doi: 10.1007/s10796-022-10284-3
Wambsganss, T., Kueng, T., Soellner, M., and Leimeister, J. M. (2021). “ArgueTutor: An adaptive dialog-based learning system for argumentation skills” in Proceedings of the 2021 CHI conference on human factors in computing systems, 1–13. doi: 10.1145/3411764.3445781
Wilson, J., Ahrendt, C., Fudge, E. A., Raiche, A., Beard, G., and MacArthur, C. (2021). Elementary teachers’ perceptions of automated feedback and automated scoring: Transforming the teaching and learning of writing using automated writing evaluation. Comput. Educ. 168:104208. doi: 10.1016/j.compedu.2021.104208
Winstone, N. E., Nash, R. A., Parker, M., and Rowntree, J. (2017). Supporting learners’ agentic engagement with feedback: A systematic review and a taxonomy of recipience processes. Educ. Psychol. 52, 17–37. doi: 10.1080/00461520.2016.1207538
Wisniewski, B., Zierer, K., and Hattie, J. (2020). The Power of Feedback Revisited: A Meta-Analysis of Educational Feedback Research. Front. Psychol. 10. doi: 10.3389/fpsyg.2019.03087
Yang, B., Nam, S., and Huang, Y. (2023). ““Why My Essay Received a 4?”: A Natural Language Processing Based Argumentative Essay Structure Analysis” in Lecture Notes in Computer Science: Vol. 13916. Artificial Intelligence in Education. AIED 2023. eds. N. Wang, G. Rebolledo-Mendez, N. Matsuda, O. C. Santos, and V. Dimitrova (Springer).
Zawacki-Richter, O., Marín, V. I., Bond, M., and Gouverneur, F. (2019). Systematic Review of Research on Artificial Intelligence Applications in Higher Education—Where are the Educators? Int. J. Educ. Technol. High. Educ. 16:39. doi: 10.1186/s41239-019-0171-0
Keywords: provider-information, feedback provider, trustworthiness, feedback message perceptions, artificial intelligence in education, large language models
Citation: Ruwe T and Mayweg-Paus E (2024) Embracing LLM Feedback: the role of feedback providers and provider information for feedback effectiveness. Front. Educ. 9:1461362. doi: 10.3389/feduc.2024.1461362
Received: 08 July 2024; Accepted: 30 September 2024;
Published: 16 October 2024.
Edited by:
Julie A. Delello, University of Texas at Tyler, United StatesReviewed by:
Nashwa Ismail, University of Liverpool, United KingdomCopyright © 2024 Ruwe and Mayweg-Paus. This is an open-access article distributed under the terms of the Creative Commons Attribution License (CC BY). The use, distribution or reproduction in other forums is permitted, provided the original author(s) and the copyright owner(s) are credited and that the original publication in this journal is cited, in accordance with accepted academic practice. No use, distribution or reproduction is permitted which does not comply with these terms.
*Correspondence: Theresa Ruwe, VGhlcmVzYS5SdXdlQGh1LWJlcmxpbi5kZQ==
Disclaimer: All claims expressed in this article are solely those of the authors and do not necessarily represent those of their affiliated organizations, or those of the publisher, the editors and the reviewers. Any product that may be evaluated in this article or claim that may be made by its manufacturer is not guaranteed or endorsed by the publisher.
Research integrity at Frontiers
Learn more about the work of our research integrity team to safeguard the quality of each article we publish.