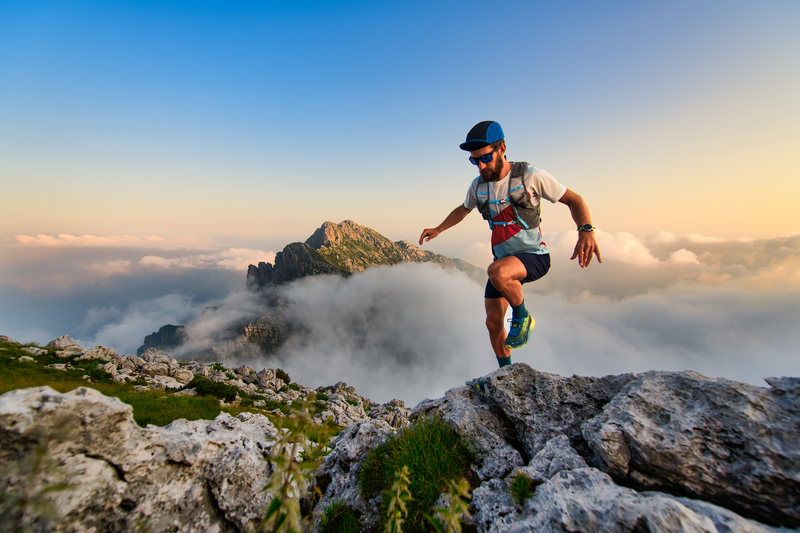
95% of researchers rate our articles as excellent or good
Learn more about the work of our research integrity team to safeguard the quality of each article we publish.
Find out more
ORIGINAL RESEARCH article
Front. Educ. , 25 November 2024
Sec. Assessment, Testing and Applied Measurement
Volume 9 - 2024 | https://doi.org/10.3389/feduc.2024.1440760
This article is part of the Research Topic Educational Evaluation in the Age of Artificial Intelligence: Challenges and Innovations View all 7 articles
Automated assessing prosody of oral reading fluency presents challenges due to the inherent difficulty of quantifying prosody. This study proposed and evaluated an approach focusing on specific prosodic features using a deep-learning neural network. The current work focuses on cross-domain performance, researching how generalizable the prosody scoring is across students and text passages. The results demonstrated that the model with selected prosodic features had better cross-domain performance with an accuracy of 62.5% compared to 57% from the previous research. Our findings also indicate that students’ reading patterns influence cross-domain performance more than specific text passage patterns. In other words, letting the student read at least one passage is more important than having others read all passage texts. The specific prosodic features had a high generalization to capture the typical prosody characteristics for achieving a satisfactorily high accuracy and classification agreement rate. This result provides valuable information for developing future automated scoring algorithms of prosody. This study is an essential demonstration of estimating the prosody score using fewer selected features, which would be more efficient and interpretable.
While there are various definitions of oral reading fluency among researchers, with various emphasis on its components, the agreement exists that oral reading fluency (ORF) is a multidimensional component consisting of accuracy, automaticity, and prosody. Fluent readers have a higher accuracy rate for word decoding in a text. Compared with these, poor readers with poor word-reading accuracy have reduced fluency and poor reading comprehension. Readers who misread words, therefore, tend not to comprehend the author’s intended message, which may cause misinterpretations of the text when word reading is inaccurate (Hudson et al., 2005, 2008; Paige, 2020; Paige et al., 2012; Rasinski, 2004). Prosody is one dimension of oral reading fluency, which is the ability to read smoothly with expressions, representing the meaning of the text by different stresses, pitch variations, intonations, rates, phrasings, and meaningful pausing (Rasinski, 2004). Studies have found that prosody seems closely associated with reading comprehension, and the relationship is substantial; overall, good oral reading prosody improves young readers’ comprehension with more contribution over reading accuracy and automaticity (Álvarez-Cañizo et al., 2015; Benjamin and Schwanenflugel, 2010; Binder et al., 2013; Klauda and Guthrie, 2008; Kuhn and Schwanenflugel, 2019; Miller and Schwanenflugel, 2008; Paige et al., 2012; Pinnell et al., 1995; Rasinski et al., 2009; Schwanenflugel and Benjamin, 2017).
In practical terms, the dimensions of accuracy and automaticity are easily quantified. Because these dimensions are highly intercorrelated, they are combined into the single measure of Words Correct Per Minute, or WCPM. This has been a single metric in measuring students’ oral reading proficiency using Curriculum-Based Measurement (CBM) (Deno, 1985) and its commercial variants, such as Dynamic Indicators of Basic Early Literacy Skills (DIBELS) (Good and Kaminski, 2002; University of Oregon, 2021), easyCBM (Alonzo et al., 2006; Riverside Assessments, 2018), and AIMSweb (Howe and Shinn, 2002).
However, unlike measuring oral reading fluency with WCPM, researchers have yet to have a consensus on measuring prosody in ORF. The most commonly used tool for human raters measuring prosody in traditional ORF assessments is rating rubrics, including the Allington (1983) scale, the National Assessment of Educational Progress (NAEP) scale (Pinnell et al., 1995), the Multidimensional Fluency Scale (MDFS) designed by Rasinski and colleagues (Rasinski, 2004; Zutell and Rasinski, 1991), and the Comprehensive Oral Reading Fluency Scale (CORFS) (Benjamin, 2012; Benjamin et al., 2013). These rubrics provide a framework with scales to quantify and evaluate prosodic features, such as expression and volume, phrasing, smoothness, and pacing. The disadvantage of using rubrics is that they are time-consuming, labor-intensive, and depend on the rater’s knowledge, skill, experience, and personal biases (Black et al., 2011).
ORF-related factors include lexicon and phoneme, sentence and punctuation, audio signals, and prosodic features. In automated scoring prosody, the researcher must convert the reading audio (i.e., speech sound waves of oral reading) to acoustic features for analyzing and studying oral reading fluency with computational algorithms. The popular tools among researchers include PRAAT (Boersma and van Heuven, 2001; Boersma and Weenink, 2021) and openSMILE (Eyben et al., 2010; Schuller et al., 2016). Researchers classified these features into different groups: prosodic features, spectral features, cepstral features, and sound quality features (Weninger et al., 2013). The critical features related to reading prosody are pitch, duration, pause, and stress (or intensity) (Kuhn et al., 2010; Schuller et al., 2016; Schwanenflugel et al., 2004). Some attempts have been made to score prosody for ORF assessments automatically (Black et al., 2007, 2011; Bolaños et al., 2013; Mostow and Duong, 2009; Sabu and Rao, 2018, 2024). Since the prosody of ORF is related to different features with complicated prosody characteristics, it is still a challenge for researchers to train a model with a machine-learning approach to mimic human judgment in evaluating prosody with improved accuracy. Therefore, in this paper, we proposed and evaluated a strategy to improve the accuracy rate for automated scoring for prosody, specifically for cross-domain contexts.
The use of specific prosodic features is satisfactory for emotion recognition in the literature (Eyben et al., 2016; Schuller et al., 2010; Weninger et al., 2013). Thus, we focused on combining selected specific prosodic and spectral features with the deep learning neural network structure to improve cross-domain performances. Also, we evaluated the effect of including features extracted from text-to-speech (TTS) audio to the deep learning neural network structure, as Sammit et al. (2022) suggested as a possible strategy to improve cross-domain performances.
A computer-based ORF assessment system will significantly reduce the assessment cost and administration effort, and some attempts to automatically score prosody have been made in this domain. As an earlier attempt, LISTEN (Literacy Innovation that Speech Technology ENables), started at the beginning of the 1990s at Carnegie Mellon University, was a project that aimed to develop an automated Reading Tutor system for children in oral reading by listening to them read aloud and helping them learn to read (Mostow et al., 2003). In their later research, Duong and others incorporated prosodic contours by focusing on prosodic features, such as latency, duration, mean pitch, and mean intensity (Duong et al., 2011; Mostow and Duong, 2009). It used pre-existing adult narration of the same sentences as a template model or a corpus of adults’ narrations to generalize models to estimate children’s readings. However, LISTEN concentrates on a single sentence read word by word. Lexical (word) level disfluency cannot measure fluency within a sentence; such methods are not good enough to rate ORF (Sabu and Rao, 2018). Since LISTEN’s pausing feature is extracted from speech that is read word by word, using the sentence level method to measure fluency between sentences is challenging. It is insufficient to rate reliable smoothness and meaningful pauses among sentences.
Bolaños et al. (2011) 2013 investigated an approach incorporating lexical and prosodic features. In 2011, the researchers introduced FLuent Oral Reading Assessment (FLORA), a web-based system with automatic speech recognition (ASR) technology, which provided an estimate for WCPM scores for first through fourth-grade students with 738 1-min reading samples from a text passage presented on the screen of a laptop. A year later, Bolaños et al. (2013) extended the functionality of FLORA into a fully automated assessment of WCPM and expressive reading according to a standard and recognized the 4-point NAEP scale. The study investigated five lexical and 15 prosodic features based on the same dataset. Five lexical features are L1-L5: WCPM, number of words spoken, number of word repetitions, number of trigrams back-offs, and variance of sentence reading rate. The prosodic features include features P1-P4 related to whether the child is paying attention to punctuation. Features P5-P6 about the number and duration of pauses made during reading. Features P9-P11 related to the number and duration of filled pauses correlated with decoding ability. Features P12-P15 related to syllable length and correlations between certain syllables on average pitch and duration within a sentence. A linear kernel was used to train three Support Vector Machine (SVM; James et al., 2013) classifiers with above 20 features and extracted 12 Mel frequency cepstral coefficients (MFCCs) and energy, and their delta and delta-delta coefficients (total of 39 features) from speech data. The results showed 73.24% for lexical features, 69.73% for prosodic features, and 76.05% for all features on overall classification accuracy with the NAEP-4 scale. The analysis of the relevance of features to classification indicates that features L1 and L2 correlate negatively with non-fluent reading, while L4 and L5 correlate positively with non-fluent reading. Silence and filled-pause features both correlate positively with non-fluent reading.
Sabu and Rao (2018) designed the assessment system to automatically measure lexical miscues evaluated in terms of insertions, deletions, and substitutions detected and prosodic miscues identified in terms of phrasal break detection and prominent word detection while working on developing an oral reading tutor, which provides automatic feedback. Two hundred readings were collected from 20 students aged between 10 and 14 with English as a second language by having each student read stories printed on paper with ten sentences each. Performance measured using precision and recall metrics is 73.2 and 73% for prominent word detection and 59.2 and 80% for phrasal break detection. In their recent study (Sabu and Rao, 2024), the researchers used a new data set with 1,447 recordings by 165 students (grades 5–8 with ages 10–14 years) reading from a pool of 148 unique passages (from 85 short English stories). A total of 144 features are extracted, which include nine lexical miscue features, four speech rate features related to accuracy and rate, 12 pauses, 50 prosodic miscues, and 69 Acoustic-Prosodic contours. Among the models with different features, the best-performed model has six features related to silence and pitch, a total of 21 features. Adjacent agreement (i.e., the percentage of scores that were only one level different) and Exact agreement rate (i.e., the percentage of scores that were precisely the same as the ground truth), two metrics used in White et al. (2021), were 88.3 and 37.7%, respectively.
Sammit et al. (2022) presented an exploration for automatically estimating prosody classification using a deep convolutional neural network. The model structure used X-vectors (Snyder et al., 2018) and self-attention (Okabe et al., 2018), two technologies in ASR and natural language processing (NLP) (Jurafsky and Martin, 2023). X-vectors aim to map the input speech features to a fixed-length vector representation (X-vector) to maximize the discrimination between different speakers while minimizing the variation within the same speaker. Self-attention enables the model to capture long-range dependencies and context within speech signals from past and future frames, improving speech recognition’s accuracy and robustness across various domains and conditions. Its best model with eight frequency features achieved classification accuracy in-domain, using known phrases in the training set, and was high at a classification rate of 86.4%. However, this declines to 57% when applied in cross-domain contexts, whereby phrases and/or students are unknown to the training algorithm. This could be suspected to be a gap between in- and cross-domain performance, probably because of the overfitting of the model since this study had resampled the data to balance the samples between classes.
On the other hand, speaker recognition and ASR with feature extraction have been studied actively for several decades (Bai and Zhang, 2021; Jurafsky and Martin, 2023; Kinnunen and Li, 2010). Feature extraction is a process that transforms the raw audio signal into acoustic feature vectors. Each vector represents the information in a small-time window of the signal, where the signal is broken down in short frames of about 20–30 ms in duration, often with at least 10 ms overlap to avoid losing information. The features can be separated into different categories based on their physical interpretations. Acoustic features, such as energy-related, spectral, and voicing-related, have been extensively used for studying in the speaker verification field (Ananthakrishnan and Narayanan, 2009; Dehak et al., 2007; Ferrer et al., 2010; Kockmann et al., 2010, 2011; Martínez et al., 2012, 2013). Prosodic features refer to non-segmental aspects of speech, including syllable stress, pitch, intonation patterns, durations, speaking rate, and rhythm (Kinnunen and Li, 2010). Typical prosodic features include loudness, the fundamental frequency or F0 closely related to pitch, zero-crossing rate related to fluency, etc. Researchers also found that prosodic features highly correlated to automatic emotion recognition in sound, speech, and music fields (Eyben et al., 2010, 2016; Schuller et al., 2010; Weninger et al., 2013).
Like some studies in the literature, our research utilizes acoustic and prosodic features and is based on a supervised model. We use the NAEP scale, a rating scheme for measuring expressive reading about whether the student is non-fluent (scores 1 and 2) or fluent (scores 3 and 4). Various studies have used this 4-class scale (Bolaños et al., 2013; Kuhn, 2005; Pinnell et al., 1995; Sammit et al., 2022; Valencia et al., 2010). Our model focused primarily on using specific features and comparing cross-domain performance among different feature sets. This work aims to enhance the model’s generalization with prosodic features and improve cross-domain performance in automated scoring of prosody in ORF assessments.
The data used in this study is audio-recorded reading data collected in the Computerized Oral Reading Evaluation (CORE) project by Nese, Kamata, and Alonzo (2014–2018) (Nese et al., 2014) and augmented by Sammit et al. (2022). This dataset includes a total of 5,841 recordings [4,128 (70.7%) in the training/validation sample and 1,713 (29.3%) in the testing cross-domain sample] on 30 reading passages (approximately 50–100 words in length) by 1,811 2nd through 4th-grade students in the U.S. The sample sizes for the three grades are roughly identical. However, the scores are imbalanced among the four classes, with score 3 dominating the classes with 49.9% samples and score four only having 3.4%. In contrast, the samples of score one and score two consist of 17.2 and 29.5%, respectively. The demographic information of the samples is shown in Figure 1. The range from 0 to 400 indicates the number of students in each ethnic category within the dataset at three grades. Most of the students in the dataset are White (83.68% in second grade, 79.10% in third grade, and 78.76% in fourth grade). Also, English was the first language for most students, with 74.63%, while English learners, with 25.37%, comprised about a quarter of all students.
We used specific prosodic features extracted with an open-source features extractor tool called openSMILE (The Munich open-Source Media Interpretation by Large feature-space Extraction) in ComParE_2016 (INTERSPEECH 2016 Computational Paralinguistics Challenge) and eGeMAPS (Geneva Minimalistic Acoustic Parameter Set) v02 format (Eyben et al., 2010, 2016; Schuller et al., 2016). ComParE_2016 is the dataset with 65 Low-Level Descriptor (LLD) features (acoustic features) and the largest with more than 6k functional features (acoustic features and their comprehensive statistically summarized information). eGeMAPS is an extension of GeMAPS that contains non-time series parameters with prosodic, excitation, vocal tract, and spectral descriptors. We focused on their LLD features, which included 65 features under the ComParE_2016 set (five energy-related features, 55 spectral-related features, and six voicing-related features) and 25 features under the eGeMAPS v02 set (three energy-related features, eight frequency-related features, and 14 spectral related features).
Based on the literature review, we chose nine specific prosodic features under ComParE_2016 LLD (shown in Table 1, along with their feature names and feature groups). These features have been reported to be relevant for speech and emotion recognition in the literature (e.g., Eyben et al., 2010; Weninger et al., 2013). The nine specific features were chosen as a small set of prosodic features, including five prosodic features (e.g., energy features that represent smoothness and loudness, and voicing features that represent fundamental frequency and pitch) and four spectral features (e.g., spectral energy at two different frequency ranges and psychoacoustic sharpness of acoustic signals).
Our well-targeted approach to feature selection differs from that of Sammit et al.’s (2022) best model, which uses only the eGeMAPS LLD subset with eight frequency-related acoustic features. We incorporated features extracted from standard text-to-speech (TTS) reading audio into the train data set, which included 300 readings (30 passages each with ten narrators, five male and five female). The purpose of doing this is to first regard these TTS data as standard with four scores to increase four score samples. Second, since kids tend to mimic the adult reading style of fluency, TTS data might help the model have better generalization.
We selected features with five different feature sets:
(1) ComParE_2016 (65 features): This feature set includes all 65 features from the ComParE_2016 dataset.
(2) ComParE_2016 (56 selected features): This set contains 56 features from ComParE_2016, excluding voicing features such as jitter, shimmer, logHNR, and spectral roll-off point features.
(3) Nine selected features: These are the nine specific features we discussed earlier.
(4) eGeMAPS v02 (seven frequency features): This set includes seven frequency-related features from the eGeMAPS v02 set, excluding jitterLocal from the original eight LLD frequency features. It includes features such as F0semitoneFrom27.5Hz and frequencies and bandwidths for F1 to F3.
(5) Sixteen combined features: This set combines nine selected features from (3) and seven frequency features from (4).
To improve the automated scoring algorithm, we first utilized the model structures used by Sammit et al. (2022). The key features of model architecture include: (1) Input layer, the input data has a time sequence of shape (sample size, 12,000, acoustic features size) with acoustic features extracted from every 2 min long embedded reading sample of audio with 20 ms frame with 10 ms overlap using openSMILE tool kit. (2) Multiple stacked SeparableConv1D layers perform efficient feature extraction while preserving computational resources and reducing the overall parameter count. Each SeparableConv1D block applies filters of increasing size (32, 64, 128, 256) to progressively capture more complex patterns in the data. (3) Downsampling and Residual Connections. Conv1D layers between blocks of SeparableConv1D with stride four continually reduce the sequence length from 12,000 to 3,000, 750, and 12 finally. Residual connections between blocks of SeparableConv1D help in flowing the gradient and prevent the vanishing gradient problem. (4) The X-vectors layer uses traditional μ and σ2 pooling. Or the weighted X-vectors layer uses processing gate blocks to multiply the μ and σ2 before pooling. (5) Self-attention layer. Or the Self-attention weighted X-vectors layer that gates the attention layer output to calculate the weighted X-vectors, providing a refined summary of the input sequence. This work trained the models using either categorical cross entropy (CCE) or quadratic weighted kappa (QWK; Shermis, 2014, 2015) as the loss function.
We wanted to examine how effective these smaller, selected feature sets were. For each feature set, we ran models using either X-Vectors or self-attention model structures, with CCE or QWK as the loss function. We also tested the models both with and without TTS samples. As a result, we trained 40 models (five feature sets × two model structures × two loss function types × two TTS conditions) and evaluated their performance. The total parameters of the seven features model are 1,006,159, with trainable parameters as 1,003,887, whereas the 65 features model is up to 1,105,540 and 1,103,268, respectively.
The study evaluated the performance of the 40 models with the classification agreements and human raters. As the dataset was imbalanced in classes (i.e., score categories) and using metrics that strike a balance between classifier performance and the uneven distribution among classes is a preferable approach, we evaluated various indices, including Micro Accuracy (which equals to micro average Precision, micro average Recall, and micro average F1-score), QWK, Micro-avg OvR ROC AUC Score, and Macro-avg OvR ROC AUC Score. All analyses ran with Python 3.10.5 (Van Rossum and Drake, 1995) and the scikit-learn package 1.1.2 (Pedregosa et al., 2011). Regarding cross-domain samples, we separated the samples into three cross-domain groups based on whether passages and/or students appeared in the training process (see Table 2).
We compared all 40 models based on the metrics with testing results of all 1,713 test samples and each cross-domain group (see Tables 3, 4). Overall test results for all 1,713 cross-domain data showed that the model with TTS data and selected 56 features of ComArE_2016 set had the best performance with 57.3% Micro-accuracy, 0.34 QWK, 0.82 Micro-avg OvR ROC AUC, and 0.74 Macro-avg OvR ROC AUC. On the other hand, the model without TTS data and the same structure had 59.0%, 0,35, 0.82, and 0.65 for the four indices.
The model with TTS data and selected nine prosodic features of ComParE_2016 with the cross-domain group that both passages and students were not in the training process showed the best performance with 59.2% Micro-accuracy, 0.33 QWK, 0.81 Micro-avg OvR ROC AUC, and 0.67 Macro-avg OvR ROC AUC. Even though this performance was not better than the model without TTS data with 56 features that had 62.5%, 0.38, 0.87, and 0.65, for the four indices, respectively, given that the model with only nine prosodic features, the result implies a potential impact of prosodic features for improving estimating performance. This might mean that even a small number of prosodic features could help the neural network to generalize the patterns. Through all models and cross-domain groups, the model with selected nine prosodic features of ComParE_2016 plus seven frequency features of eGeMAPS (Table 3), the model with selected nine prosodic features of ComParE_2016 (Table 4), and the model with seven frequency features of eGeMAPS (Table 4) showed the highest QWK score 0.44. The results in Tables 3, 4 confirm that adding TTS data further improves cross-domain performance, especially for models using selected prosodic features. This also suggests the prosodic features are cardinal for providing good generalization of prosody estimation across different reading passages and students, pushing the area further with more effective and interpretable automated scoring systems.
First, the train data set needed to be more balanced, with fewer observations in scoring class 4 compared to scoring classes 1–3. Therefore, it influenced the overall classification performance measured by QWK. We also tested by adding 300 standard TTS audios, scored in scoring class 4, to train samples. However, compared to the models without standard TTS audio data, the results did not show that they improved the model performance more than we expected. The reason might be that the sample size was still tiny in terms of our deep neural network structure. Another possible reason is that TTS audios were adult narrators, unlike our data with 2nd to 4th-grade students.
Second, when we considered the cross-domain conditions, there were two aspects: passage and student. That is, passage, student, or both might have yet to be discovered for a trained model. Initially, we suspected that passages would have more weight than students in model performance for score classifications. However, our results revealed that the data with students known in the training process showed overall better performance than those with students unknown in the training process. In other words, letting the student read at least one passage is more important than having others read all passage texts.
Third, the two models with selected nine prosodic features, testing with data with which both passages and students were unknown in the training process, showed the best performance by QWK compared to the model with more features. This result may imply that the model with specific prosodic features had a higher generalization to capture the typical characteristics of the prosody. This study can be an essential demonstration for achieving a satisfactorily high accuracy and classification agreement rate with fewer selected features, which would be more efficient and potentially more interpretable.
We also noted some limitations in this study. First, the training sample included readings from 1,811 students. Although it was seemingly a large sample for a deep learning neural network, it needed to be more significant to have the neural network learn scoring prosody with desirable accuracy. Second, most of the students in our sample were White students with English as their first language. Therefore, the model has limited generalizability when used for different racial groups of students and students whose first language is not English. Additional investigations are warranted to determine whether the model performance depends on English level, cross-domain groups, and grade levels. Also, the impact of specific types of features on classification performance needs to be investigated.
In sum, this work found the features that impact the performance of estimating the prosody score of ORF. However, we needed more research to answer the question of what kind of features and magnitude the features worked on. The current study only directly used acoustic and prosodic features with two-dimensional sequence data extracted from reading audio. We understand that the functional output by openSMILE takes statistical summaries over the sequence, so each two-dimensional sequence data becomes a vector with features information. Working on such data with an extensive machine learning approach will help us understand more about features impacting automated scoring prosody. Such exploration might support the researchers with better feature selection since prosody is more complex than simple acoustic features like pitch and energy.
This study explored adding TTS audio data to train a neural network to learn the “ideal” prosody patterns of readings. Future exploration can be done by training an encoder-decoder network using TTS as a prosody reference, audio from student oral reading, and textual features of reading text. Preprocessed student speech can subsequently be aligned to such “ideal” reference to estimate prosody scores. Prosody requires capturing relationships across longer time scales (intonation over a sentence, pauses, rhythm, etc.).
On the other hand, pausing is purposeful or accidental silence time during reading and is meaningfully related to prosody (Benjamin et al., 2013; Benjamin and Schwanenflugel, 2010). All related work we mentioned in this paper, except Sammit et al. (2022) included pause features in their estimating model to enhance performance. Information about pauses calculated with word-level silence time was also an essential prosodic feature, which can be obtained with the output of the ASR system. Researchers found that fluent/less-fluent readers have different pausing patterns, whereas no-fluent readers have more random and irrelevant pauses (Benjamin and Schwanenflugel, 2010; Miller and Schwanenflugel, 2008). Future research that seeks to understand how the pause patterns related to reading and text influence estimating prosody performance would be a meaningful try.
The data analyzed in this study is subject to the following licenses/restrictions: data analyzed in this study cannot be publicly available due to IRB restrictions. Requests to access these datasets should be directed to JN, am5lc2VAdW9yZWdvbi5lZHU=.
KW: Conceptualization, Methodology, Software, Writing – original draft, Writing – review and editing. XQ: Data curation, Writing – original draft. GS: Data curation, Software, Writing – review and editing. EL: Conceptualization, Writing – review and editing. JN: Data curation, Writing – review and editing. AK: Data curation, Project administration, Writing – review and editing.
The author(s) declare financial support was received for this article’s research, authorship, and/or publication. The research reported here was supported, in whole or in part, by the Institute of Education Science, U.S. Department of Education, through Grant R305D200018 to the University of Oregon.
The authors declare that the research was conducted without any commercial or financial relationships that could potentially create a conflict of interest.
All claims expressed in this article are solely those of the authors and do not necessarily represent those of their affiliated organizations, or those of the publisher, the editors and the reviewers. Any product that may be evaluated in this article, or claim that may be made by its manufacturer, is not guaranteed or endorsed by the publisher.
The opinions expressed are those of the authors and do not represent the views of the Institute or the U.S. Department of Education.
Alonzo, J., Tindal, G., Ulmer, K., and Glasgow, A. (2006). easyCBM© Online Progress Monitoring Assessment System. Eugene, OR: Behavioral Research and Teaching.
Álvarez-Cañizo, M., Suárez-Coalla, P., and Cuetos, F. (2015). The role of reading fluency in children’s text comprehension. Front. Psychol. 6:1810. doi: 10.3389/fpsyg.2015.01810
Ananthakrishnan, S., and Narayanan, S. (2009). Unsupervised adaptation of categorical prosody models for prosody labeling and speech recognition. IEEE Trans. Audio Speech Lang. Process. 17, 138–149. doi: 10.1109/TASL.2008.2005347
Bai, Z., and Zhang, X.-L. (2021). Speaker recognition based on deep learning: An overview. Neural Netw. 140, 65–99. doi: 10.1016/j.neunet.2021.03.004
Benjamin, R. G. (2012). Development and Validation of the Comprehensive Oral Reading Fluency Scale. [Unpublished Doctoral Dissertation]. Athens, GA: University of Georgia., 177.
Benjamin, R. G., and Schwanenflugel, P. J. (2010). Text complexity and oral reading prosody in young readers. Read. Res. Q. 45, 388–404. doi: 10.1598/RRQ.45.4.2
Benjamin, R. G., Schwanenflugel, P. J., Meisinger, E. B., Groff, C., Kuhn, M. R., and Steiner, L. (2013). A spectrographically grounded scale for evaluating reading expressiveness. Read. Res. Q. 48, 105–133. doi: 10.1002/rrq.43
Binder, K. S., Tighe, E., Jiang, Y., Kaftanski, K., Qi, C., and Ardoin, S. P. (2013). Reading expressively and understanding thoroughly: An examination of prosody in adults with low literacy skills. Read. Writ. 26, 665–680. doi: 10.1007/s11145-012-9382-7
Black, M., Tepperman, J., and Narayanan, S. S. (2011). Automatic prediction of children’s reading ability for high-level literacy assessment. IEEE Trans. Audio Speech Lang. Process. 19, 1015–1028. doi: 10.1109/TASL.2010.2076389
Black, M., Tepperman, J., Lee, S., Price, P., and Narayanan, S. S. (2007). “Automatic detection and classification of disfluent reading miscues in young children’s speech for the purpose of assessment,” in Proceedings of the Interspeech, Antwerp, 206–209. doi: 10.21437/Interspeech.2007-87
Boersma, P., and Weenink, D. (2021). Praat: Doing phonetics by computer [Computer program], version 6.1.53. Available online at: http://www.praat.org/ (accessed September 08, 2021).
Bolaños, D., Cole, R. A., Ward, W. H., Tindal, G. A., Schwanenflugel, P. J., and Kuhn, M. R. (2013). Automatic assessment of expressive oral reading. Speech Commun. 55, 221–236. doi: 10.1016/j.specom.2012.08.002
Bolaños, D., Cole, R. A., Ward, W., Borts, E., and Svirsky, E. (2011). FLORA: Fluent oral reading assessment of children’s speech. ACM Trans. Speech Lang. Process. 7:19. doi: 10.1145/1998384.1998390
Dehak, N., Dumouchel, P., and Kenny, P. (2007). Modeling prosodic features with joint factor analysis for speaker verification. IEEE Trans. Audio Speech Lang. Process. 15, 2095–2103. doi: 10.1109/TASL.2007.902758
Deno, S. L. (1985). Curriculum-based measurement: The emerging alternative. Except. Children 52, 219–232.
Duong, M., Mostow, J., and Sitaram, S. (2011). Two methods for assessing oral reading prosody. ACM Trans. Speech Lang. Process. 7:14. doi: 10.1145/1998384.1998388
Eyben, F., Scherer, K. R., Schuller, B. W., Sundberg, J., Andre, E., Busso, C., et al. (2016). The Geneva minimalistic acoustic parameter set (GeMAPs) for voice research and affective computing. IEEE Trans. Affect. Comput. 7, 190–202. doi: 10.1109/TAFFC.2015.2457417
Eyben, F., Wöllmer, M., and Schuller, B. (2010). “Opensmile: The Munich versatile and fast open-source audio feature extractor,” in Proceedings of the 18th International Conference on Multimedia MM ’10, Firenze. 1459–1462. doi: 10.1145/1873951.1874246
Ferrer, L., Scheffer, N., and Shriberg, E. (2010). “A comparison of approaches for modeling prosodic features in speaker recognition,” in Proceedings of the 2010 IEEE International Conference on Acoustics, Speech and Signal Processing, Dallas, TX, 4414–4417. doi: 10.1109/ICASSP.2010.5495632
Good, R. H., and Kaminski, R. A. (2002). DIBELS Oral Reading Fluency Passages for First through Third Grades (Technical Report No.10). Eugene, OR: University of Oregon, 12.
Howe, K. B., and Shinn, M. M. (2002). Standard Reading Assessment Passages (raps) for Use in General Outcome Measurement. Eden Prairie, MN: edformation.
Hudson, R. F., Lane, H. B., and Pullen, P. C. (2005). Reading fluency assessment and instruction: What, why, and how? Read. Teach. 58, 702–714. doi: 10.1598/RT.58.8.1
Hudson, R. F., Pullen, P. C., Lane, H. B., and Torgesen, J. K. (2008). The complex nature of reading fluency: A multidimensional view. Read. Writ. Q. 25, 4–32. doi: 10.1080/10573560802491208
James, G., Witten, D., Hastie, T., and Tibshirani, R. (2013). An Introduction to Statistical Learning, Vol. 103. New York, NY: Springer. doi: 10.1007/978-1-4614-7138-7
Jurafsky, D., and Martin, J. H. (2023). Speech and Language Processing (Third). Available online at: https://web.stanford.edu/~jurafsky/slp3/ (accessed October 23, 2023).
Kinnunen, T., and Li, H. (2010). An overview of text-independent speaker recognition: From features to supervectors. Speech Commun. 52, 12–40. doi: 10.1016/j.specom.2009.08.009
Klauda, S. L., and Guthrie, J. T. (2008). Relationships of three components of reading fluency to reading comprehension. J. Educ. Psychol. 100, 310–321. doi: 10.1037/0022-0663.100.2.310
Kockmann, M., Burget, L., and Èernockı, J. (2010). “Investigations into prosodic syllable contour features for speaker recognition,” in Proceedings of the 2010 IEEE International Conference on Acoustics, Speech and Signal Processing, Dallas, TX. 4418–4421. doi: 10.1109/ICASSP.2010.5495616
Kockmann, M., Ferrer, L., Burget, L., and Cernocky, J. (2011). “iVector fusion of prosodic and cepstral features for speaker verification,” in Proceedings of the 12th Annual Conference of the International Speech Communication Association, Florence, 265–268. doi: 10.21437/Interspeech.2011-57
Kuhn, M. R. (2005). A comparative study of small group fluency instruction. Read. Psychol. 26, 127–146. doi: 10.1080/02702710590930492
Kuhn, M. R., and Schwanenflugel, P. J. (2019). Prosody, pacing, and situational fluency (or why fluency matters for older readers). J. Adolesc. Adult Literacy 62, 363–368.
Kuhn, M. R., Schwanenflugel, P. J., and Meisinger, E. B. (2010). Aligning theory and assessment of reading fluency: Automaticity, prosody, and definitions of fluency. Read. Res. Q. 45, 230–251.
Martínez, D., Burget, L., Ferrer, L., and Scheffer, N. (2012). “iVector-based prosodic system for language identification,” in Proceedings of the 2012 IEEE International Conference on Acoustics, Speech and Signal Processing (ICASSP), Kyoto, 4861–4864. doi: 10.1109/ICASSP.2012.6289008
Martínez, D., Lleida, E., Ortega, A., and Miguel, A. (2013). “Prosodic features and formant modeling for an ivector-based language recognition system,” in Proceedings of the 2013 IEEE International Conference on Acoustics, Speech and Signal Processing, Vancouver, BC, 6847–6851. doi: 10.1109/ICASSP.2013.6638988
Miller, J., and Schwanenflugel, P. J. (2008). A longitudinal study of the development of reading prosody as a dimension of oral reading fluency in early elementary school children. Read. Res. Q. 43, 336–355.
Mostow, J., Aist, G., Burkhead, P., Corbett, A., Cuneo, A., Eitelman, S., et al. (2003). Evaluation of an automated reading tutor that listens: Comparison to human tutoring and classroom instruction. J. Educ. Comput. Res. 29, 61–117. doi: 10.2190/06AX-QW99-EQ5G-RDCF
Mostow, J., and Duong, M. (2009). “Automated assessment of oral reading prosody,” in Proceedings of the 2009 Conference on Artificial Intelligence in Education: Building Learning Systems That Care: From Knowledge Representation to Affective Modelling, Brighton. 189–196. doi: 10.3233/978-1-60750-028-5-189
Nese, J. F. T., Kamata, A., and Alonzo, J. (2014). “Measuring oral reading fluency: Computerized oral reading evaluation (CORE),” in Funded by institute of educational science—U.S. Department of Education to University of Oregon. R305a140203. Eugene, OR: University of Oregon.
Okabe, K., Koshinaka, T., and Shinoda, K. (2018). “Attentive statistics pooling for deep speaker embedding,” in Proceedings of the Interspeech 2018, Hyderabad. 2252–2256. doi: 10.21437/Interspeech.2018-993
Paige, D. D. (2020). Reading Fluency: A Brief History, the Importance of Supporting Processes, and the Role of Assessment. Report from Northern Illinois University retrieved from an ERIC Search.
Paige, D. D., Rasinski, T. V., and Magpuri-Lavell, T. (2012). Is fluent, expressive reading important for high school readers? J. Adolesc. Adult Literacy 56, 67–76. doi: 10.1002/JAAL.00103
Pedregosa, F., Varoquaux, G., Gramfort, A., Michel, V., Thirion, B., Grisel, O., et al. (2011). Scikit-learn: Machine learning in Python. J. Mach. Learn. Res. 12, 2825–2830.
Pinnell, G. S., Pikuiski, J. J., Wixson, K. K., Campbell, J. R., Gough, P. B., and Beatty, A. S. (1995). Listening to Children Read Aloud: Data from NAEP’s Integrated Reading Performance Record (IRPR) at Grade 4. Available online at: https://eric.ed.gov/?id=ED378550 (accessed July 27, 2021).
Rasinski, T. (2004). Assessing Reading Fluency. Honolulu, HI: Pacific Resources for Education and Learning (PREL).
Rasinski, T., Rikli, A., and Johnston, S. (2009). Reading fluency: More than automaticity? More than a concern for the primary grades? Literacy Res. Instruct. 48, 350–361. doi: 10.1080/19388070802468715
Sabu, K., and Rao, P. (2018). Automatic assessment of children’s oral reading using speech recognition and prosody modeling. CSI Trans. ICT 6, 221–225. doi: 10.1007/s40012-018-0202-3
Sabu, K., and Rao, P. (2024). Predicting children’s perceived reading proficiency with prosody modeling. Comput. Speech Lang. 84:101557. doi: 10.1016/j.csl.2023.101557
Sammit, G., Wu, Z., Wang, Y., Wu, Z., Kamata, A., Nese, J., et al. (2022). “Automated prosody classification for oral reading fluency with quadratic kappa loss and attentive x-vectors,” in Proceedings of the ICASSP 2022 - 2022 IEEE International Conference on Acoustics, Speech and Signal Processing (ICASSP), Singapore, 3613–3617. doi: 10.1109/ICASSP43922.2022.9747391
Schuller, B., Steidl, S., Batliner, A., Hirschberg, J., Burgoon, J. K., Baird, A., et al. (2016). The INTERSPEECH 2016 computational paralinguistics challenge: Deception, sincerity & native language,” in Proceedings of the 17th Annual Conference of the International Speech Communication Association, San Francisco, CA, 2001–2005. doi: 10.21437/Interspeech.2016-129
Schuller, B., Vlasenko, B., Eyben, F., Wöllmer, M., Stuhlsatz, A., Wendemuth, A., et al. (2010). Cross-corpus acoustic emotion recognition: Variances and strategies. IEEE Trans. Affect. Comput. 1, 119–131. doi: 10.1109/T-AFFC.2010.8
Schwanenflugel, P. J., and Benjamin, R. G. (2017). Lexical prosody as an aspect of oral reading fluency. Read. Writ. 30, 143–162. doi: 10.1007/s11145-016-9667-3
Schwanenflugel, P. J., Hamilton, A. M., Kuhn, M. R., Wisenbaker, J. M., and Stahl, S. A. (2004). Becoming a fluent reader: Reading skill and prosodic features in the oral reading of young readers. J. Educ. Psychol. 96, 119–129. doi: 10.1037/0022-0663.96.1.119
Shermis, M. D. (2014). State-of-the-art automated essay scoring: Competition, results, and future directions from a United States demonstration. Assess. Writ. 20, 53–76. doi: 10.1016/j.asw.2013.04.001
Shermis, M. D. (2015). Contrasting state-of-the-art in the machine scoring of short-form constructed responses. Educ. Assess. 20, 46–65. doi: 10.1080/10627197.2015.997617
Snyder, D., Garcia-Romero, D., Sell, G., Povey, D., and Khudanpur, S. (2018). “X-vectors: Robust dnn embeddings for speaker recognition,” in Proceedings of the 2018 IEEE International Conference on Acoustics, Speech and Signal Processing (ICASSP), Calgary, AB, 5329–5333. doi: 10.1109/ICASSP.2018.8461375
University of Oregon (2021). DIBELS 8 administration and scoring guide 2021. Eugene, OR: University of Oregon.
Valencia, S. W., Smith, A. T., Reece, A. M., Li, M., Wixson, K. K., and Newman, H. (2010). Oral reading fluency assessment: Issues of construct, criterion, and consequential validity. Read. Res. Q. 45, 270–292. doi: 10.1598/RRQ.45.3.1
Van Rossum, G., and Drake, F. L. Jr. (1995). Python Tutorial. Amsterdam: Centrum voor Wiskunde en Informatica.
Weninger, F., Eyben, F., Schuller, B., Mortillaro, M., and Scherer, K. (2013). On the acoustics of emotion in audio: What speech, music, and sound have in common. Front. Psychol. 4:292. doi: 10.3389/fpsyg.2013.00292
White, S., Sabatini, J., Park, B. J., Chen, J., Bernstein, J., and Li, M. (2021). The 2018 NAEP Oral Reading Fluency Study. NCES 2021-025. Washington, DC: National Center for Education Statistics.
Keywords: automated scoring, oral reading fluency, prosody, reading assessment, cross-domain test, deep learning, feature selection, speech
Citation: Wang K, Qiao X, Sammit G, Larson EC, Nese J and Kamata A (2024) Improving automated scoring of prosody in oral reading fluency using deep learning algorithm. Front. Educ. 9:1440760. doi: 10.3389/feduc.2024.1440760
Received: 30 May 2024; Accepted: 11 November 2024;
Published: 25 November 2024.
Edited by:
Raman Grover, Consultant, Vancouver, BC, CanadaReviewed by:
Soo Lee, American Institutes for Research, United StatesCopyright © 2024 Wang, Qiao, Sammit, Larson, Nese and Kamata. This is an open-access article distributed under the terms of the Creative Commons Attribution License (CC BY). The use, distribution or reproduction in other forums is permitted, provided the original author(s) and the copyright owner(s) are credited and that the original publication in this journal is cited, in accordance with accepted academic practice. No use, distribution or reproduction is permitted which does not comply with these terms.
*Correspondence: Kuo Wang, d2FuZ3BAc211LmVkdQ==
Disclaimer: All claims expressed in this article are solely those of the authors and do not necessarily represent those of their affiliated organizations, or those of the publisher, the editors and the reviewers. Any product that may be evaluated in this article or claim that may be made by its manufacturer is not guaranteed or endorsed by the publisher.
Research integrity at Frontiers
Learn more about the work of our research integrity team to safeguard the quality of each article we publish.