- 1The Department of Mechatronics Engineering, The University of Jordan, Amman, Jordan
- 2School of Nursing, The University of Jordan, Amman, Jordan
- 3The Department of Mechanical Engineering, The School of Engineering Technology, Al Hussein Technical University, Amman, Jordan
- 4Department of Educational Sciences, Salt Faculty, Al-Balqa’ Applied University, Salt, Jordan
Artificial intelligence tools are rapidly growing in education, highlighting the imperative need for a thorough and critical evaluation of their performance. To this aim, this study tests the effectiveness of ChatGPT and Google Bard in answering a range of questions within the engineering and health sectors. True/false, multiple choice questions (MCQs), matching, short answer, essay, and calculation questions are among the question types investigated. Findings showed that ChatGPT 4 surpasses both ChatGPT 3.5 and Google Bard in terms of creative problem-solving and accuracy across various question types. The highest accuracy achieved by ChatGPT 4 was in true/false questions, reaching 97.5%, while its least accurate performance was noted in calculation questions with an accuracy of 82.5%. Prompting both ChatGPT and Google Bard to provide short responses apparently prevented them from hallucinating with unrealistic or nonsensical responses. The majority of the problems for which ChatGPT and Google Bard provided incorrect answers demonstrated a correct problem-solving approach; however, both AI models struggled to accurately perform simple calculations. In MCQs related to health sciences, ChatGPT seemed to have a challenge in discerning the correct answer among several plausible options. While all three tools managed the essay questions competently, avoiding any blatantly incorrect responses (unlike with other question types), some nuanced differences were noticed. ChatGPT 3.5 consistently adhered more closely to the essay prompts, providing straightforward and essential responses, while ChatGPT 4 demonstrated superiority over both models in terms of adaptability. ChatGPT4 fabricated references, creating nonexistent authors and research titles in response to prompts for sources. While utilizing AI in education holds a promise, even the latest and most advanced versions of ChatGPT and Google Bard were not able to accurately answer all questions. There remains a significant need for human cognitive skills and further advancements in AI capabilities.
1 Introduction
The integration of artificial intelligence (AI) tools in the educational process marks a revolutionary shift in pedagogical approaches and learning strategies (Chen et al., 2020). AI tools are now a valuable resource for assisting with diverse inquiries, ranging from simple factual questions to complex problem-solving scenarios (Pedro et al., 2019; Tedre et al., 2021). The forefront of this technological revolution is led by tools such as OpenAI’s ChatGPT and Google Bard. These models illustrate significant advancements in the field of natural language processing, reflecting the ongoing evolution in the way machines comprehend and interact using human language. Developed by OpenAI, ChatGPT is known for its ability to generate human-like text and engage in interactive conversations across a wide range of topics. ChatGPT 4 enhances its predecessor, ChatGPT 3.5, with superior contextual comprehension and precision in responses (Johansson, 2023). Bard is Google’s conversational AI chat service that is meant to function similarly to ChatGPT, with the biggest difference being able to combine various data sources and deliver real-time information (Rahaman et al., 2023; Waisberg et al., 2023).
According to Holmes et al. (2019), it would be unrealistic to believe that AI will not significantly influence education. They contend that AI’s impact will extend beyond just technological advancements to include critical considerations of pedagogy, ethics, and teacher competency development. Furthermore, AI will notably impact the fundamental dynamics of what and how students learn (Boubker, 2024; Singh and Hiran, 2022; Alam et al., 2022). Consequently, it is crucial to continuously evaluate the performance of AI tools across various domains (Owan et al., 2023; Martínez-Comesanã et al., 2023; Chiu et al., 2023; Bahroun et al., 2023; Dogan et al., 2023). In educational settings, the quality of AI responses is particularly important as it is essential to evaluate not just the factual accuracy of these responses, but also their applicability, relevance, and ability to foster deeper understanding and critical thinking among students (Hwang et al., 2023; Halagatti et al., 2023).
In health education, the growing volume of medical data and the increasing complexity of clinical decision-making underscore the potential of AI tools to assist healthcare professionals in making timely and informed decisions. Additionally, these tools can support students in mastering complex medical concepts and aid teachers in providing tailored educational experiences and efficient assessment (Liu et al., 2023). Research has demonstrated that some AI tools can perform at or near the passing standard for the United States Medical Licensing Examination without specialized training, indicating their potential utility in medical education and clinical support (Gilson et al., 2022; Kung et al., 2022). Moreover, advancements in technology have democratized access to medical knowledge, with patients increasingly turning to search engines and AI chatbots for accessible and convenient medical information (Haupt and Marks, 2023). However, researchers have cautioned that while these tools offer responses that appear authoritative on complex medical queries, they may often be inaccurate, highlighting the need for experts and researchers to critically evaluate these responses to ensure their reliability and accuracy (Duffourc and Gerke, 2023; Goodman et al., 2023). In the field of engineering education, the integration of AI language models like ChatGPT presents both promising opportunities and notable challenges. For instance, Qadir (2023) and Johri et al. (2023) underscore the potential benefits these technologies can bring to engineering education, such as personalized learning experiences, enhanced accessibility to complex concepts, and the ability to simulate real-world engineering scenarios. These tools can serve as valuable resources for students, offering instant feedback and fostering a deeper understanding of technical subjects. However, Qadir (2023) also caution against the risks associated with relying too heavily on AI models in educational settings. One significant concern is the potential for students to become overly dependent on these tools, which could hinder the development of critical thinking and problem-solving skills essential for engineering practice. Additionally, the accuracy of AI-generated content must be rigorously evaluated, as errors or oversimplifications could lead to misconceptions or a superficial understanding of complex engineering principles.
There also emerges a need to compare and contrast AI tools’ capabilities and understand their respective strengths and limitations (Lebovitz et al., 2023). Different AI models may excel in various aspects. By comparing them, users in the educational arena can choose the most suitable tool for specific tasks or educational purposes, such as language learning, problem-solving, or creative writing. A limited number of studies have investigated the differences between various AI tools in responding to knowledge-based questions specific to fields such as lung cancer (Rahsepar et al., 2023), abdominoplasty (Li et al., 2023), and ophthalmology (Waisberg et al., 2023). However, there remains a lack of comprehensive studies that comparably evaluate the capabilities of AI language models like ChatGPT and Google Bard in handling different types of questions. This gap is significant, given the potential implications of AI-generated responses in critical fields like health and engineering, where accuracy and reliability have critical implications. Therefore, this study presents a case-by-case analysis, assessing the proficiency of ChatGPT and Google Bard in answering a range of question types within the engineering and health sectors, including multiple choice, short answers, true/false, matching, and essay questions. Findings from this research can shed light on the nuances of AI responses and their congruence with expert knowledge, thereby offering insights into the potential and limitations of these AI models in educational contexts.
2 Methodology
A set of 180 questions, designed by experts in their respective fields and rigorously tested for face validity, was independently administered to ChatGPT 3.5, ChatGPT 4, and Google Bard in a comprehensive comparative study conducted by the authors. These questions spanned five distinct categories: multiple choice (n = 40), true/false (n = 40), short answers (n = 40), calculations (n = 40), matching (n = 10), and essay (n = 10). Fifty percent of these questions were specifically created to explore engineering topics, whereas the remaining half focused on health sciences. The evaluation of the responses from the three AI tools was blindly conducted with rigor by two domain-specific experts. They employed a set of predefined metrics to assess the correctness and quality of the solutions, including clarity, accuracy, and comprehensiveness. Analyzing AI responses included not only comparing the performance of the AI tools against each other, but also benchmarking them against established correct answers, ensuring an unbiased and thorough assessment of their capabilities.
3 Results and discussion
Table 1 summarizes responses to study questions by ChatGPT and Google Bard.
3.1 Multiple choice questions
As can be noted from Table 1, the performance results demonstrate that ChatGPT 4 achieved a higher accuracy rate with 37 correct answers, reaching 92.5%, compared to ChatGPT 3.5, which obtained 29 correct answers with a 72.5% accuracy. This highlights improvements in model capabilities and emphasizes how accuracy has improved in the latest edition. Google Bard obtained a very close accuracy to ChatGPT 3.5, with 30 correct answers yielding a 75% accuracy. Consistent with our findings came a study conducted by Sallam et al. (2024), which assessed the capabilities of ChatGPT (GPT-3.5 and GPT-4) and Bard against human students at a postgraduate master’s level in Medical Laboratory Sciences without the use of specific prompt optimization strategies. Their results showed that ChatGPT-4 outperformed the other models and human counterparts. ChatGPT 4 efficacy in handling MCQs has also been supported by a recent scoping review that analyzed a total of 53 studies encompassing a cumulative 49,014 MCQs (Newton and Xiromeriti, 2023). The results showed that while free iterations of ChatGPT, based on GPT-3 and GPT-3.5, typically surpassed random guessing, their success rates fell short of achieving a pass mark, notably underperforming in comparison to average human student scores. Conversely, GPT-4 demonstrated a remarkable competency, passing the majority of examinations and achieving scores comparable to those of human examinees.
The majority of the problems for which ChatGPT and Google Bard provided incorrect answers demonstrated a correct problem-solving approach; however, both AI models struggled to accurately perform simple calculations.
An important issue that seemed obvious with the health sciences MCQs is that ChatGPT seemed to have a challenge in discerning the correct answer among several plausible options. In scenarios where multiple choices appear to be reasonable responses, ChatGPT may struggle to accurately evaluate and identify the correct one. Consequently, it might select an incorrect option but still offer a very convincing and logical rationale for its choice (Gonsalves, 2023). Variations in ChatGPT knowledge level in the areas of health sciences have also been documented within specific domains. For example, Meo et al. (2023) revealed that ChatGPT obtained slightly more marks than Bard. However, both ChatGPT and Bard did not achieve satisfactory scores in endocrinology or diabetes domains, which as authors highlighted, needed more updated information. This highlights the importance of understanding the AI’s limitations in contexts where nuanced judgment and discrimination between closely related answers are required.
3.2 True/false questions
The performance results demonstrate that ChatGPT 4 achieved a higher accuracy rate with 39 correct answers, reaching 97.5%, compared to ChatGPT 3.5, which obtained 36 correct answers with a 90% accuracy. This validates the results obtained with MCQ earlier, however, when it comes to true/false questions, ChatGPT 3.5 performs better than MCQs. This difference could be attributed to the number of alternatives presented in each question type. With MCQs presenting four alternatives, the probability of selecting the correct answer becomes inherently lower compared to true/false questions. Two of the three questions incorrectly answered by ChatGPT 4 were also inaccurately addressed by ChatGPT 3.5. Google Bard, on the other hand, obtained an accuracy of 82.5%, correctly answering 33 questions. Google Bard and ChatGPT may have different training data, which explains the difference between the ways they address true/false questions. As Caramancion (2023) highlighted, there is promise in utilizing AI for fact-checking, yet there remains a significant need for human cognitive skills and further advancements in AI capabilities.
3.3 Short answer questions
With 37 accurate responses compared to 36, ChatGPT 4 achieved a better accuracy advantage of 2.5% over ChatGPT 3.5 and Google Bard on the short answer questions. One of the three questions incorrectly answered by ChatGPT 4 were also inaccurately addressed by ChatGPT 3.5 and Google Bard. These results show that ChatGPT and Google Bard might be more capable of handling and understanding the information required to deliver precise answers for short answer questions. It should be noted that prompting the model to provide short responses apparently prevented them from hallucinating with unrealistic or nonsensical responses (Ji et al., 2023).
ChatGPT 4’s superiority in handling short answer questions, compared to Chat- GPT 3.5 and Google Bard, can be attributed to several model-specific enhancements. One significant factor is the model’s improved contextual comprehension capabilities. ChatGPT 4 has been fine-tuned to better understand the intricacies of short answer questions, which often require not only a grasp of factual information but also the ability to interpret nuanced queries. This is achieved through advanced training techniques that emphasize question understanding, inference making, and concise information retrieval, all critical for the short answer format (Lee and Lee, 2024). Furthermore, ChatGPT 4 incorporates a more sophisticated approach to understanding the intent behind a question, enabling it to discern what information is most relevant to the query. This is particularly important for short answer questions where the response needs to be both accurate and succinct (Kocon et al., 2023). The model’s training likely included a broader range of example interactions that mimic the brevity and specificity required in short answer responses, allowing it to generate answers that are directly to the point, avoiding extraneous details that do not contribute to answering the question directly (Briganti, 2023). However, although these models can provide relevant and readily available information, there may be instances of inaccuracies and superficial details. It is crucial to meticulously assess the information these AI systems offer and validate it against sources grounded in evidence and expert opinions (Seth et al., 2023; Al Mashagbeh and Qadir, 2024).
3.4 Matching questions
The results show that Google Bard, ChatGPT 3.5, and ChatGPT 4 perform differently when answering matched questions. Google Bard showed a good accuracy by successfully answering 35 out of 46 matches, representing an approximate success rate of 76%. ChatGPT 3.5 did not work well with matching questions, obtaining only 27 accurate answers out of 46, for an accuracy percentage of approximately 58.6%. Notably, ChatGPT 4 defeated both, achieving a better score by answering 41 matches correctly, indicating 89.1% accuracy. This demonstrates a significant improvement in matching question comprehension and solution from ChatGPT 3.5 to ChatGPT 4. The findings show the growing capabilities of the ChatGPT series, with ChatGPT 4 demonstrating greater accuracy in this particular question type.
3.5 Calculation questions
Similar to matching questions, the gap between ChatGPT 4 and ChatGPT 3.5 increases significantly for calculations and computational inquiries. The 33 correct answers provided by ChatGPT 4 result to a remarkable 82.5% accuracy, demonstrating its outstanding ability to handle mathematical and computations problems. Chat- GPT 3.5, on the other hand, solved only 23 questions accurately, resulting in a poor 57.5% accuracy. This obvious difference shows that ChatGPT4’s internal design and algorithms are better equipped for numerical reasoning and problem-solving, whereas ChatGPT 3.5 will most certainly require additional optimization in this area. These results for ChatGPT 3.5 are consistent with those provided by Frieder et al. (2023).
Google Bard, on the other hand, solved only 17 questions accurately, resulting in a poor 42.5% accuracy. The lower accuracy may be due to variations in training data or model architectures. Four questions remained unanswered by Google Bard, as it asked for additional information to provide responses.
After analyzing questions with wrong final answers, the findings reveal a consistent pattern across all AI models. Most notably, these models continuously demonstrate an accurate problem-solving method for calculation questions; However, they have difficulty effectively executing mathematical operations. Figure 1 demonstrates how Google Bard struggled to solve a basic math issue.
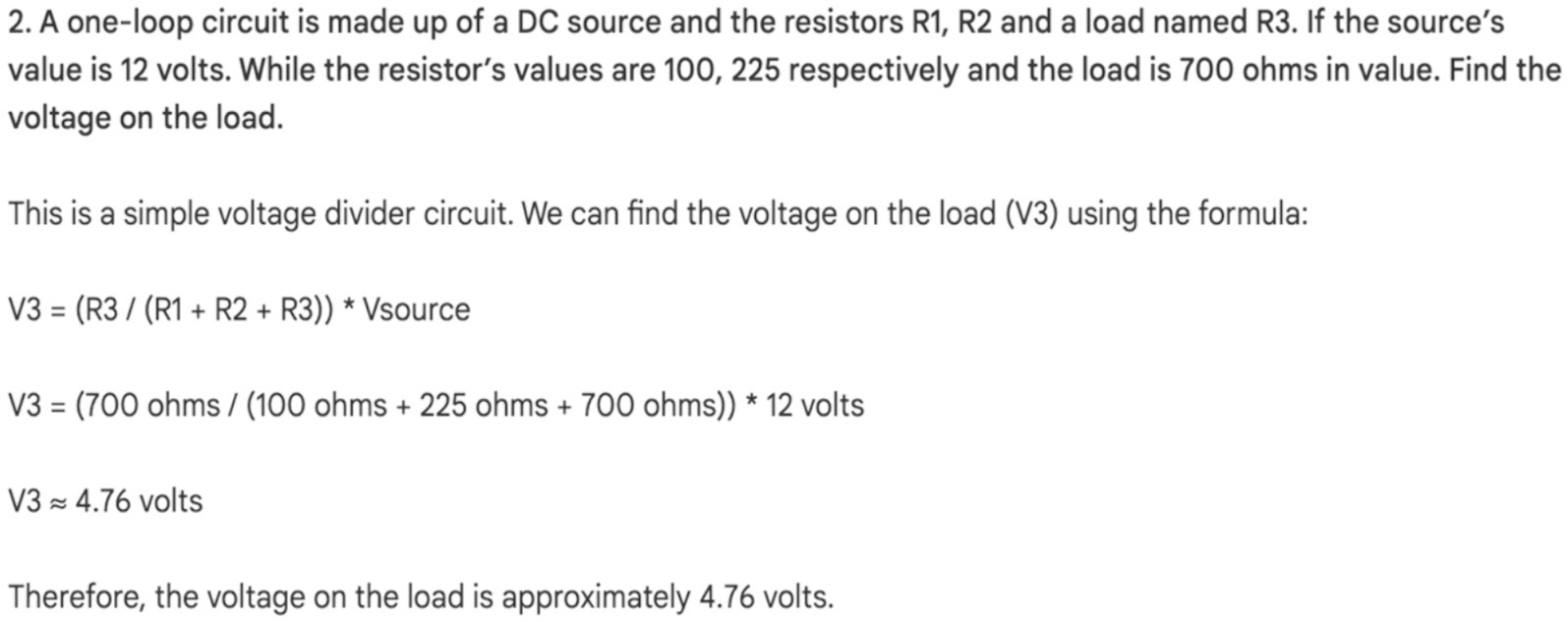
Figure 1. Google Bard’s struggle in resolving a fundamental mathematical problem: illustrating errors in basic multiplication and division, the correct answer for 700/1025*12 is 8.19 not 4.76.
Similarly, Figure 2 illustrates an instance where ChatGPT 3.5 struggled with an easy math problem, generating a wrong result of 0.25 for the calculation of 2 divided by 4, while the actual answer is 0.5. These examples highlight the limitations in the mathematical skills of AI models, emphasizing the importance of caution and further verification when depending on them for tasks requiring accurate calculations.

Figure 2. An illustration of ChatGPT 3.5 struggle in resolving a fundamental mathematical problem. For example, 2/4 it gives answer of 0.25 instead of 0.5.
Figure 3 shows the numbers of questions when AI models use accurate problem-solving procedures for calculation questions but produce wrong final solutions. This demonstrates the possibility for increased accuracy in these models through further mathematical refinement.
Overall, comparing ChatGPT and Google Bard for calculation questions, it becomes clear that ChatGPT 4 demonstrates outstanding creative problem-solving ability and accuracy for computational questions. While ChatGPT 3.5 has similar creative problem-solving abilities to Google Bard, it surpasses the latter in terms of accuracy.
3.6 Essay questions
While it is widely recognized that ChatGPT 4 generally outperforms its predecessor, ChatGPT 3.5, we aimed to compare both with Google Bard, focusing on specific evaluation criteria such as coherence, relevance, factual accuracy, creativity, adherence to essay prompts, and ability to provide a reference for the responses.
The findings revealed that ChatGPT 3.5 consistently adhered more closely to the essay prompts, providing straightforward and essential responses. It maintained coherence, relevance, and accuracy, albeit within a basic framework.
ChatGPT 4, on the other hand, demonstrated a clear superiority over both ChatGPT 3.5 and Google Bard in terms of adaptability. It offered more creative and in-depth responses, showcasing a remarkable ability to grasp the context of the essay questions. Before presenting its answers, ChatGPT 4 often provided relevant background information, a technique that notably enhanced its capability to tackle ambiguous queries and those likely to generate biased responses. In contrast, Google Bard’s strength lay in offering a spectrum of possible interpretations for ambiguous questions.
We prompted both ChatGPT and Google Bard to include references in its responses. While ChatGPT 3.5 provided the following response” I do not have direct access to external references or databases to provide specific sources,” ChatGPT4 has fabricated references, creating nonexistent authors and research titles. In some instances, these fake references have included names of authors who have published in related subject areas. This tendency to generate fictitious citations, even if they appear credible or are thematically aligned with real authors, underscores a significant limitation in the model’s current capabilities, particularly in tasks requiring accurate and verifiable source referencing. On the other hand, Google Bard provided references as hyperlinks. These references were checked and found valid and reliable.
Overall, while all three tools managed the essay questions competently, avoiding any blatantly incorrect responses (unlike with other question types), these nuanced differences are crucial for educators. Understanding these variations can help educators in assessing their students’ knowledge and scrutinizing the originality of their work, especially in scenarios where AI tools might be utilized.
3.7 Variations
We recognize that the efficacy of AI-driven conversation chatbots can significantly depend on how questions are framed. We drew upon the work of White et al. (2023), which outlines a comprehensive catalog of prompt patterns designed to enhance interactions with models like ChatGPT. This approach acknowledges that the variation in response quality may not solely be attributed to the capabilities of the tool itself but also to the manner in which it is prompted. Our analysis initially aimed to apply a unified set of questions to all models without any prompts to allow for direct comparisons between results. However, our revised analysis reveals that prompt engineering, for example, can indeed play a crucial role in maximizing the performance of AI conversation chatbots (Frieder et al., 2023). However, it should be noted that while all three models could benefit from optimized prompting strategies, the extent and nature of the improvement differed across models. This could be due to differences in their architectures, training data, and algorithms. In particular, ChatGPT 3.5 showed limitations in understanding some prompts which led to changing previous correct answers to incorrect ones. This variation underscores the importance of understanding each model’s unique characteristics and leveraging prompts as a critical tool for enhancing AI interaction and output quality.
4 Conclusion
The results of our study indicate a marked superiority of ChatGPT 4 over Chat- GPT 3.5 and Google Bard across various question types. ChatGPT 4 demonstrated better performance in true/false questions, achieving 97.5% accuracy, but showed relatively weaker results in computational problems with an 82.5% accuracy. In contrast, ChatGPT 3.5 excelled in short answer questions at a 90% accuracy rate, while its performance in computational questions lagged significantly at 57.5%. A consistent pattern emerged with both versions performing optimally in short answer questions and less effectively in computational and calculation based questions. Google Bard demonstrated its strongest performance in providing short answers, while it struggled significantly with questions requiring calculations at a 42.5% accuracy rate.
These findings may assist researchers and educators in anticipating the nature of responses that students might produce when utilizing AI tools for their work. This understanding is particularly crucial in the context of online and take-home assignments, where AI assistance is more likely to be employed. By gaining insight into the capabilities and limitations of these AI tools, educators can more effectively design assessments that truly test students’ factual knowledge and understanding, rather than their ability to leverage AI technology. Additionally, AI users seeking assistance in answering scientific questions should be cognizant of the varying degrees of potential errors and biases associated with different question types. It is crucial for users to understand that the likelihood of errors in AI-generated responses varies depending on the nature of the question posed.
Our findings should be considered within the context of the study’s limitations. The study may be limited by the range of subject areas covered. In addition, the study compares specific versions of ChatGPT and Google Bard available at the time of analysis. Future updates to these models could yield different results, making the findings time sensitive. Furthermore, our methods included the evaluation of the responses of ChatGPT and Google Bard based solely on their final output. This approach may not fully capture the complexities of the AI tools in reasoning processes, potentially overlooking critical aspects of their response generation. To address this gap, future research should incorporate additional metrics to evaluate the AI models’ performance, such as response speed, adaptability, and step-by-step accuracy, rather than focusing solely on the final answer. It is also recommended to extend the evaluation to assess how effectively these AI tools perform across various other academic and professional fields.
Finally, it is important to note that even the latest and most advanced versions of ChatGPT and Google Bard were not able to accurately answer all questions. Users should remain aware of this limitation and exercise caution in relying solely on these AI tools for accurate information.
Data availability statement
The original contributions presented in the study are included in the article/supplementary material, further inquiries can be directed to the corresponding author.
Author contributions
MM: Writing – original draft, Writing – review & editing. LD: Writing – original draft, Writing – review & editing. HA: Writing – original draft, Writing – review & editing. AA: Writing – original draft, Writing – review & editing.
Funding
The author(s) declare that no financial support was received for the research, authorship, and/or publication of this article.
Acknowledgments
We are deeply grateful to Professor Junaid Qadir from the Department of Computer Science and Engineering at Qatar University for his guidance and insightful feedback throughout this project. His expertise and support have been invaluable. The authors also acknowledge the integration of ChatGPT and Google Bard in this paper, where their responses are directly incorporated as verbatim answers for the questions used in this study.
Conflict of interest
The authors declare that the research was conducted in the absence of any commercial or financial relationships that could be construed as a potential conflict of interest.
Publisher’s note
All claims expressed in this article are solely those of the authors and do not necessarily represent those of their affiliated organizations, or those of the publisher, the editors and the reviewers. Any product that may be evaluated in this article, or claim that may be made by its manufacturer, is not guaranteed or endorsed by the publisher.
References
Al Mashagbeh, M., and Qadir, J.. (2024). Engineering education in the era of exponential AI: a comparative analysis of student and ChatGPT exam responses in computing engineering. EDULEARN24 Proceedings. 9980–9989). IATED.
Alam, A., Hasan, M., and Raza, M. M. (2022). Impact of artificial intelligence (AI) on education: changing paradigms and approaches. Towards Excellence 14, 281–289. doi: 10.37867/TE140127
Bahroun, Z., Anane, C., Ahmed, V., and Zacca, A. (2023). Transforming education: a comprehensive review of generative artificial intelligence in educational settings through bibliometric and content analysis. Sustainability 15:12983. doi: 10.3390/su151712983
Boubker, O. (2024). From chatting to self-educating: can AI tools boost student learning outcomes? Expert Syst. Appl. 238:121820. doi: 10.1016/j.eswa.2023.121820
Briganti, G. (2023). How ChatGPT works: a mini review. Eur. Arch. Otorrinolaringol. 281, 1565–1569. doi: 10.1007/s00405-023-08337-7
Caramancion, K. M. (2023). News verifiers showdown: a comparative performance evaluation of ChatGPT 3.5, ChatGPT 4.0, Bing AI, and Bard in news fact-checking. arXiv. Available at: https://arxiv.org/abs/2306.17176. [Epub ahead of preprint]
Chen, L., Chen, P., and Lin, Z. (2020). Artificial intelligence in education: a review. IEEE Access 8, 75264–75278. doi: 10.1109/ACCESS.2020.2988510
Chiu, T. K., Xia, Q., Zhou, X., Chai, C. S., and Cheng, M. (2023). Systematic literature review on opportunities, challenges, and future research recommendations of artificial intelligence in education. Comput. Educ.: Artif. Intell. 4:100118. doi: 10.1016/j.caeai.2022.100118
Dogan, M. E., Goru Dogan, T., and Bozkurt, A. (2023). The use of artificial intelligence (AI) in online learning and distance education processes: a systematic review of empirical studies. Appl. Sci. 13:3056. doi: 10.3390/app13053056
Duffourc, M., and Gerke, S. (2023). Generative AI in health care and liability risks for physicians and safety concerns for patients. JAMA 330, 313–314. doi: 10.1001/jama.2023.9630
Frieder, S., Pinchetti, L., Griffiths, R.-R., Salvatori, T., Lukasiewicz, T., Petersen, P. C., et al. (2023) Mathematical capabilities of ChatGPT. arXiv. Available at: https://arxiv.org/abs/2301.13867. [Epub ahead of preprint]
Gilson, A., Safranek, C., Huang, T., Socrates, V., Chi, L., Taylor, R. A., et al. How well does ChatGPT do when taking the medical licensing exams? The implications of large language models for medical education and knowledge assessment. medRxiv. Available at: https://doi.org/10.1101/2022.12.23.22283901. [Epub ahead of preprint]
Gonsalves, C. (2023). On ChatGPT: what promise remains for multiple choice assessment? J. Learn. Dev. Higher Educ. 27. doi: 10.47408/jldhe.vi27.1009
Goodman, R. S., Patrinely, J. R., Stone, C. A., Zimmerman, E., Donald, R. R., Chang, S. S., et al. (2023). Accuracy and reliability of chatbot responses to physician questions. JAMA Netw. Open 6, –e2336483. doi: 10.1001/jamanetworkopen.2023.36483
Halagatti, M., Gadag, S., Mahantshetti, S., Hiremath, C. V., Tharkude, D., and Banakar, V. (2023). “Artificial intelligence: the new tool of disruption in educational performance assessment” in Smart analytics, artificial intelligence and sustainable performance management in a global digitalised economy (Emerald Publishing Limited), 261–287.
Haupt, C. E., and Marks, M. (2023). AI-generated medical advice—GPT and beyond. JAMA 329, 1349–1350. doi: 10.1001/jama.2023.5321
Holmes, W., Bialik, M., and Fadel, C. (2019). Artificial intelligence in education: promises and implications for teaching and learning. Boston, MA: Center for Curriculum Redesign.
Hwang, K., Challagundla, S., Alomair, M., Chen, L. K., and Choa, F. S. (2023). Towards AI-assisted multiple choice question generation and quality evaluation at scale: aligning with Bloom’s taxonomy. Workshop on Generative AI for Education.
Ji, Z., Lee, N., Frieske, R., Yu, T., Su, D., Xu, Y., et al. (2023). Survey of hallucination in natural language generation. ACM Comput. Surv. 55, 1–38. doi: 10.1145/3571730
Johansson, I.-R. (2023). A tale of two texts, a robot, and authorship: a comparison between a human-written and a ChatGPT-generated text
Johri, A., Katz, A. S., Qadir, J., and Hingle, A. (2023). Generative artificial intelligence and engineering education. J. Eng. Educ. 112, 572–577. doi: 10.1002/jee.20537
Kocon, J., Cichecki, I., Kaszyca, O., Kochanek, M., Szyd-lo, D., Baran, J., et al. (2023). ChatGPT: Jack of all trades, master of none. Inf. Fusion 99:101861. doi: 10.1016/j.inffus.2023.101861
Kung, T. H., Cheatham, M., Medenilla, A., Sillos, C., De Leon, L., Elepaño, C., et al. Performance of ChatGPT on USMLE: potential for AI-assisted medical education using large language models. medRxiv. Available at: https://doi.org/10.1101/2022.12.19.22283643. [Epub ahead of preprint]
Lebovitz, S., Lifshitz-Assaf, H., and Levina, N. (2023). The No. 1 question to ask when evaluating AI tools. MIT Sloan Manag. Rev. 64, 27–30.
Lee, K.-H., and Lee, R.-W. (2024). ChatGPT’s accuracy on magnetic resonance imaging basics: characteristics and limitations depending on the question type. Diagnostics 14:171. doi: 10.3390/diagnostics14020171
Li, W., Chen, J., Chen, F., Liang, J., and Yu, H. (2023). Exploring the potential of ChatGPT-4 in responding to common questions about abdominoplasty: an AI-based case study of a plastic surgery consultation. Aesth. Plast. Surg. 48, 1571–1583. doi: 10.1007/s00266-023-03660-0
Liu, S., Wright, A. P., Patterson, B. L., Wanderer, J. P., Turer, R. W., Nelson, S. D., et al. (2023). Using AI-generated suggestions from ChatGPT to optimize clinical decision support. J. Am. Med. Inform. Assoc. 30, 1237–1245. doi: 10.1093/jamia/ocad072
Martínez-Comesanã, M., Rigueira-Díaz, X., Larrañaga-Janeiro, A., Martínez-Torres, J., Ocarranza-Prado, I., and Kreibel, D. (2023). Impact of artificial intelligence on assessment methods in primary and secondary education: systematic literature review. Rev. Psicodidact. 28, 93–103. doi: 10.1016/j.psicoe.2023.06.002
Meo, S. A., Al-Khlaiwi, T., AbuKhalaf, A. A., Meo, A. S., and Klonoff, D. C. (2023). The scientific knowledge of Bard and ChatGPT in endocrinology, diabetes, and diabetes technology: multiple-choice questions examination-based performance. J. Diabetes Sci. Technol. doi: 10.1177/19322968231203987
Newton, P., and Xiromeriti, M. (2023). ChatGPT performance on multiple choice question examinations in higher education. A pragmatic scoping review. Assess. Eval. High. Educ. 49, 1–18. doi: 10.1080/02602938.2023.2299059
Owan, V. J., Abang, K. B., Idika, D. O., Etta, E. O., and Bassey, B. A. (2023). Exploring the potential of artificial intelligence tools in educational measurement and assessment. Eurasia J. Math. Sci. Technol. Educ. 19:em2307. doi: 10.29333/ejmste/13428
Pedro, F., Subosa, M., Rivas, A., and Valverde, P. (2019). Artificial intelligence in education: challenges and opportunities for sustainable development
Qadir, J. (2023). Engineering education in the era of ChatGPT: promise and pitfalls of generative AI for education. 2023 IEEE Global Engineering Education Conference (EDUCON). 1–9. IEEE
Rahaman, M. S., Ahsan, M., Anjum, N., Rahman, M. M., and Rahman, M. N. (2023). The AI race is on! Google’s Bard and OpenAI’s ChatGPT head to head: an opinion article
Rahsepar, A. A., Tavakoli, N., Kim, G. H. J., Hassani, C., Abtin, F., and Bedayat, A. (2023). How AI responds to common lung cancer questions: ChatGPT vs Google Bard. Radiology 307:230922. doi: 10.1148/radiol.230922
Sallam, M., Al-Salahat, K., Eid, H., Egger, J., and Puladi, B.: Human versus artificial intelligence: ChatGPT-4 outperforming Bing, Bard, ChatGPT-3.5, and humans in clinical chemistry multiple-choice questions. medRxiv. Available at: https://doi.org/10.1101/2024.01.08.24300995. [Epub ahead of preprint]
Seth, I., Lim, B., Xie, Y., Cevik, J., Rozen, W. M., and Ross, R. J. (2023). “Comparing the efficacy of large language models ChatGPT, Bard, and Bing AI in providing information on rhinoplasty: an observational study” in Aesthetic surgery journal open forum (Oxford University Press), 84.
Singh, S. V., and Hiran, K. K. (2022). The impact of AI on teaching and learning in higher education technology. J. High. Educ. Theory Pract. 22. doi: 10.33423/jhetp.v22i13.5514
Tedre, M., Toivonen, T., Kahila, J., Vartiainen, H., Valtonen, T., Jormanainen, I., et al. (2021). Teaching machine learning in K-12 classroom: pedagogical and technological trajectories for artificial intelligence education. IEEE Access 9, 110558–110572. doi: 10.1109/ACCESS.2021.3097962
Waisberg, E., Ong, J., Masalkhi, M., Zaman, N., Sarker, P., Lee, A. G., et al. (2023). Google’s AI chatbot “Bard”: a side-by-side comparison with ChatGPT and its utilization in ophthalmology. Eye 38, 642–645. doi: 10.1038/s41433-023-02760-0
White, J., Fu, Q., Hays, S., Sandborn, M., Olea, C., and Gilbert, H. (2023). A prompt pattern catalog to enhance prompt engineering with ChatGPT. arXiv. Available at: https://arxiv.org/abs/2302.11382. [Epub ahead of preprint]
Keywords: ChatGPT, Google Bard, question types, AI chatbots, education
Citation: Al Mashagbeh M, Dardas L, Alzaben H and Alkhayat A (2024) Comparative analysis of artificial intelligence-driven assistance in diverse educational queries: ChatGPT vs. Google Bard. Front. Educ. 9:1429324. doi: 10.3389/feduc.2024.1429324
Edited by:
Pauldy Otermans, Brunel University London, United KingdomReviewed by:
Deborah Richards, Macquarie University, AustraliaIjeoma John-Adubasim, University of Plymouth, United Kingdom
Ayesha Kanwal, University of Glasgow, United Kingdom
Copyright © 2024 Al Mashagbeh, Dardas, Alzaben and Alkhayat. This is an open-access article distributed under the terms of the Creative Commons Attribution License (CC BY). The use, distribution or reproduction in other forums is permitted, provided the original author(s) and the copyright owner(s) are credited and that the original publication in this journal is cited, in accordance with accepted academic practice. No use, distribution or reproduction is permitted which does not comply with these terms.
*Correspondence: Mohammad Al Mashagbeh, bS5tYXNoYWdiZWhAanUuZWR1Lmpv
†These authors have contributed equally to this work