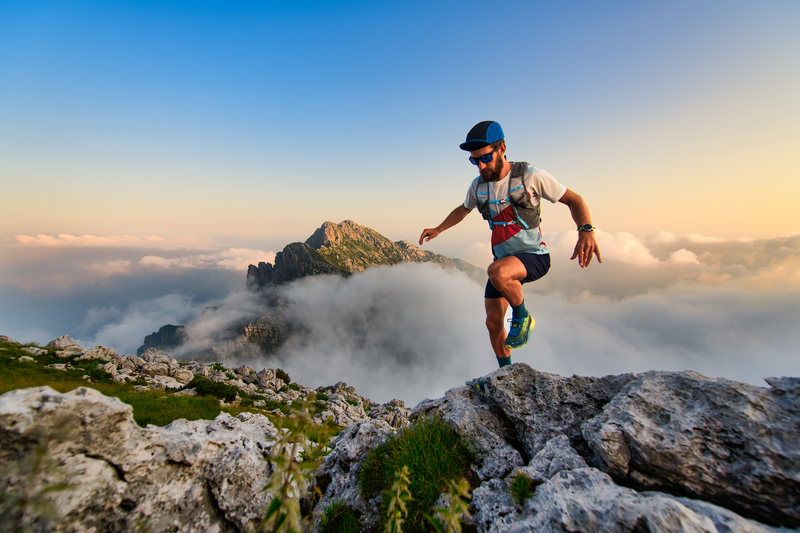
94% of researchers rate our articles as excellent or good
Learn more about the work of our research integrity team to safeguard the quality of each article we publish.
Find out more
ORIGINAL RESEARCH article
Front. Educ. , 30 October 2024
Sec. Assessment, Testing and Applied Measurement
Volume 9 - 2024 | https://doi.org/10.3389/feduc.2024.1248770
This article is part of the Research Topic Insights in Assessment, Testing, and Applied Measurement: 2022 View all 21 articles
This note discusses the radical technical inflation in error variance and the related standard error of test scores from both conceptual and empirical viewpoints. This technical inflation arises as a direct consequence of the technical underestimation of item-score correlation by the product–moment coefficient of correlation (PMC), which is embedded in the traditional estimators of reliability such as coefficients alpha, theta, omega, or rho (maximal reliability). Specifically, in educational settings where compilations usually include both easy and difficult items, the estimate by PMC may substantially deviate from the true association between an item and the score. Consequently, the use of traditional estimators of reliability leads to technically inflated estimates of standard errors, as the error variance related to these traditional measurement models is significantly inflated, resulting in deflated reliability estimates. In educational testing, employing deflation-corrected standard errors, calculated using deflation-corrected reliability estimators, would provide a more accurate measure of the test score’s true precision.
This note focuses on a consequential outcome concerning significant deflation observed in the primary reliability estimators used within classical test theory, namely, coefficients alpha, theta, omega, and rho (maximal reliability), as previously discussed by researchers such as Zumbo and colleagues (e.g., Zumbo et al., 2007; Gadermann et al., 2012) and more recently by Metsämuuronen (2022a,b,c,d,e,f, 2023). The reader is led to the concepts and literature from four perspectives. Section 1.1 discusses the general phenomena of deflation in reliability and inflation in error variance. Section 1.2 explores the phenomenon where correlation estimates serve as the primary cause of deflation in reliability estimates and inflation in error variance. Section 1.3 briefly examines conceptual aspects related to error variance inflation. Section 1.4 provides a hypothetical example illustrating the magnitude of error variance, inflation, and standard error. The empirical section investigates the extent of error variance, inflation, and standard errors, aiming to elucidate the circumstances under which notable effects are expected.
In certain kinds of tests, which typically include items of extreme difficulty levels, as is common in educational testing settings (see discussion in Metsämuuronen, 2023), the technical deflation in the estimates of reliability has been reported to range from 0.40 to 0.70 units of the reliability coefficient. In these types of tests, the standard errors related to the score are significantly inflated. For extremely easy or difficult tests, standard errors can be more than ten times higher when using traditional reliability estimators compared to deflation-corrected reliability estimators (DCER) (Metsämuuronen and Ukkola, 2019; Metsämuuronen, 2022b; for DCER details, see Metsämuuronen, 2022c,d,e). When tests include easy, medium, and difficult items, the standard errors can be two to three times higher with traditional estimators (Metsämuuronen, 2022f). This indicates that the estimated error variance related to the measurement model is radically inflated.
The deflation of 0.40–0.70 units of reliability discussed above related to the artificial technical or mechanical errors in the estimation of correlation needs to be separated from attenuation related to the violations against the measurement model. The attenuation related to estimators of reliability and, consequently, in the estimated standard errors has been discussed widely, especially the challenges related to coefficient alpha (Kuder and Richardson, 1937; Jackson and Ferguson, 1941; Guttman, 1945; Cronbach, 1951), which are well known (see discussions and literature in, e.g., Sijtsma, 2009; Cho and Kim, 2015; Hoekstra et al., 2019; Metsämuuronen, 2022b,d).
Guttman (1945) was the first to show that the coefficient we know today as coefficient alpha always gives estimates that are lower in magnitude than true population reliability. The magnitude of the attenuation related to the violations against the assumption related to coefficient alpha has been reported to vary from 1% (Raykov, 1997) to 11% (Green and Yang, 2009). However, it is commonly accepted that if the assumptions for the coefficient alpha are met, the items are (essentially) tau-equivalent, the phenomenon is unidimensional, and the measurement errors related to test items do not correlate. Alpha would give unattenuated estimates (see Novick and Lewis, 1967; Raykov and Marcoulides, 2017; see the discussion also in, e.g., Green and Yang, 2009, 2015; Davenport et al., 2015, 2016; Trizano-Hermosilla and Alvarado, 2016; McNeish, 2017). However, there is an ongoing debate among scholars about whether we could continue to use coefficient alpha as one of the lower boundaries of reliability or not at all (see a positive view in, e.g., Bentler, 2009; Falk and Savalei, 2011; Raykov et al., 2015; Metsämuuronen, 2017; Raykov and Marcoulides, 2017; and a negative view in, e.g., Sijtsma, 2009; Yang and Green, 2011; Dunn et al., 2013; Trizano-Hermosilla and Alvarado, 2016; McNeish, 2017).
Notably, the underestimation in the estimates of reliability is only partly related to attenuation, as discussed above. Metsämuuronen (2016) shows algebraically that the radical deflation in the estimates of reliability is directly related to technical or mechanical errors in the estimates of correlation by item-score correlation (Rit). This issue affects not only coefficient alpha but also other reliability coefficients such as theta, omega, and rho, which also incorporate item-score correlation in some form (see Metsämuuronen, 2022b,c,d). Metsämuuronen (2022b,d) identifies several other estimators of reliability with the same challenge. The role of Rit in the deflation is discussed later.
Deflation in reliability has a direct effect on the traditional standard error of measurement (S.E.m) related to the test score (see Metsämuuronen, 2023). The standard error is a concept used in quantifying the average amount of random measurement error in a score variable generated by a compilation of multiple test items; the technicalities are discussed in Section 1.3. Notably, in large-scale testing settings such as Program of International Student Assessment (PISA) and Trends in International Mathematics and Science Study (TIMSS), the focus is mainly on the standard errors across different parts of the ability scale, referred to as conditional standard errors, rather than the average S.E.m. (see, e.g., Schult and Sparfeldt, 2016; Foy and LaRoche, 2019). In this note, however, the traditional S.E.m. is discussed because it has a direct relationship with the traditional estimate of reliability (REL), that is, (e.g., Gulliksen, 1950), based on the classical test theory definition of reliability:
where , , and refer to the variances of the observed score variable (X), unobserved true score (T), and error element (E) related to the profound idea in measurement modeling: X = T + E.
Because of the simplicity of the definition of reliability in Equation (1), the technical reason for the observed radical deflation in the estimates of reliability can be traced to two sources: either the population variance ( ) is deflated, or the error variance ( ) is inflated—or both may happen at the same time. Metsämuuronen (2022h) specifically studies the magnitude and limits of the deflation in the population variance. The deflation in the population variance is an obvious reason for the deflation in the estimates by coefficients alpha and theta and related coefficients from the extended family (see the discussion in, e.g., Metsämuuronen, 2016, 2022b,d). The reason is obvious because the element embedded in the reliability formulae embeds the item–score correlation (Rit = ; ; see also Equation 8), and Rit is known to give radical underestimates when the scales of two variables differ. This is always the case with an item and a score. This is deepened and illustrated in Section 1.2.
In the more advanced estimators of reliability, such as coefficients omega and rho, the reason for the deflation is partly the overestimated error variance observed in the form of in the formulae (see later Equation 4), where refers to factor loading. Notably, factor loadings are essentially correlations between an item and a factor score (e.g., Cramer and Howitt, 2004; Yang, 2010). The consequences and magnitude of the deflation in reliability are discussed in the empirical part of this article.
Due to being the oldest estimator of association still in use—with over a century of research on and with the product–moment coefficient of correlation (PMC)—most of its weaknesses are well-known. General challenges are extensively covered in standard textbooks (e.g., Salkind, 2010; Tabachnick and Fidell, 2021). Two specific challenges strictly related to the topic of the article are discussed here.
First, scholars have extensively discussed a particular challenge of the product–moment coefficient of correlation (PMC) under the topic of “restriction of range” or “range restriction” (RR) for over a century, starting from the works of Pearson (1903) and Spearman (1904) onward. More recent discussions are summarized by Sackett and Yang (2000), Sackett et al. (2007), Meade (2010), Walk and Rupp (2010) and Metsämuuronen (2022d). This phenomenon refers to situations where only a portion of the range of values of a variable is realized in the sample, leading to inaccurate correlation estimates by PMC. These estimates are attenuated, meaning they are lower than the true correlation (see various patterns of RR in Sackett and Yang, 2000). Pearson (1903) and Spearman (1904) proposed initial solutions to correct this attenuation, and numerous solutions have been suggested since then (see typologies in Mendoza and Mumford, 1987; Sackett et al., 2007). This characteristic of PMC has been investigated and addressed, particularly within meta-analytic studies (see, e.g., Schmidt and Hunter, 2003, 2015; Schmidt et al., 2008).
The other challenge the PMC poses, closely related to the inflation in error variance, is its inaccurate estimation in item analysis settings. This is considered the primary reason for the deflation of reliability because PMC is embedded in the most widely used reliability estimators (see compiled in Metsämuuronen, 2022d). Through simulations, Metsämuuronen (2021a, 2022a); also partly observed in simulations by Martin (1973, 1978) and Olsson (1980) has identified seven cumulative and partly interrelated conditions where deflation in estimates by PMC is anticipated.
Based on these simulations, the item–score correlation (Rit) tends to consistently and systematically underestimate the true association between an item and a score variable under the following conditions:
1. The deflation approximates 100% the greater the extremity of the item difficulty is.
2. Scale discrepancy: The greater the discrepancy between the item’s scale and the score.
3. Fewer item categories: The fewer categories present in the item.
4. Fewer score categories: The fewer categories present in the score.
5. Number of items: The smaller the number of items comprising the score. This is closely linked to the number of categories in the score’s scale.
6. Non-uniform tied cases: The greater the presence of non-uniformly distributed tied cases in the score. This a consequence of having a small number of items.
7. Distribution: If the distribution of the latent variable (and score) deviates from a uniform distribution.
Consequently, if the test contains items with extreme difficulty levels, a small number of items, and items with a narrow scale, resulting in a score with a narrow scale, we anticipate obtaining significantly deflated item-total correlations. This leads to markedly inflated measurement errors, substantially deflated reliability estimates, and inflated standard errors. The extent of this inflation is illustrated in Section 1.4 with a numerical example.
The phenomenon of technical or mechanical deflation in the estimates of correlation can be easily illustrated with two identical (latent) variables that have an obvious perfect correlation = 1. If one of these identical variables is dichotomized (item) and the other polytomized into several categories (score), Rit cannot reach the perfect (latent) correlation. This is unlike other measures, such as polychoric correlation (RPC; Pearson, 1900, 1913), Goodman–Kruskal gamma (G; Goodman and Kruskal, 1954), dimension-corrected G (G2; Metsämuuronen, 2021a), and attenuation-corrected Rit and eta (RAC and EAC; Metsämuuronen, 2022e,g) (see simulations in Metsämuuronen, 2021a, 2022a). Some estimators, such as r-bireg and r-polyreg correlation (RREG; Livingston and Dorans, 2004; Moses, 2017), Somers delta directed so that “score dependent” (D; Somers, 1962), and dimension-corrected D (D2; Metsämuuronen, 2020b, 2021a) come close to a deflation-free outcome.
As an example of the radical technical deflation in PMC, let us take the vector of n = 1,000 cases from a normally distributed population and double it. Of these identical variables, one (item g) is divided into a binary form [df(g) = 1] by using a cut-off of p = 0.90; that is, 90% of the hypothetical test-takers give the correct answer, and the other (score X) is divided into seven categories [df(X) = 6] with an average difficulty level of [p(X) = 0.50]; this could be a latent reflection of a short subtest (e.g., “geometry”) amid a longer test (“mathematical achievement”). The difference between the latent correlation ( = 1) and the observed correlation ( = = Rit) indicates the magnitude of technical deflation in the estimates, even without attenuation, which may add some additional deflation to the outcome. Figure 1 illustrates the magnitudes of the technical deflation in selected estimators of association.
Figure 1. Magnitude of deflation in the selected estimators of association.
Rir, Henrysson item–rest correlation (= PMC); Tau-b, Kendall tau-b; RRank, Spearman rank-order correlation (= PMC); Rit, Item-total correlation (= PMC); eta, Coefficient eta (X dependent) (= PMC in the binary case); D, Somers delta (X dependent); D2, Dimension-corrected D; RReg, r-bireg correlation; RPC, Polychoric correlation; G, Goodman-Kruskal gamma; G2, Dimension-corrected G; RAC, Attenuation-corrected Rit; EAC, Attenuation-corrected eta.
Notably, the estimates by such known estimators of item-score association based on the mechanics of PMC as Henrysson’s item–rest correlation Rir (Henrysson, 1963), Spearman’s rank-order correlation RRank (Spearman, 1904), Rit, and eta cannot detect the obvious perfect latent correlation, and the magnitude of deflation is notable (> 0.47 units of correlation).1 Moreover, Kendall’s tau-b (Kendall, 1948) gives a deflated estimate because the values are always lower than those by PMC (see the reasons in, e.g., Metsämuuronen, 2021b). Such estimators as RPC, G, G2, RA, C, and EAC are found deflation-free in this kind of comparison. However, they may have some other challenges in fully reaching the true association (see Metsämuuronen, 2022a). RREG is almost deflation-free, and, in D and D2, the magnitude of deflation may be nominal, depending on the number of tied pairs in the items and score as well as the widths of the scales in item and score (see Metsämuuronen, 2021a). Hence, based on an analysis of 11 sources of deflation, Metsämuuronen (2022a) lifts coefficients RPC, RREG, G, D, G2, D2, RAC, and EAC as superior options for Rit to be used in estimators of reliability to reach deflation-corrected estimates of reliability. Some of these estimators are used as benchmarks in the numerical example and empirical section to assess the magnitude of the inflation in error variance and standard errors.
Section 1.4 gives a practical, hypothetical example of the phenomenon of inflation. Some concepts are needed to understand the notation in that section. However, the conceptual discussion is minimal in this section (see in detail in Supplementary Appendix 1).
Let us assume a congeneric measurement model with one latent variable (θ):
where xi denotes the observed values of an item gi and wi denotes a weight factor that links θ with xi (e.g., Metsämuuronen, 2022a,c,d). This congeneric measurement model is generalized from the traditional model (e.g., McDonald, 1999; Cheng et al., 2012). In the traditional model, the weight factor wi is usually assumed to be a factor loading (λi), and the factor score variable is assumed to reflect the most accurately the latent variable. In the general model, the weight factor is a coefficient of association in some form, also including principal component and factor loadings. The unobservable θ may manifest as a varying type of relevantly formed compilation of items such as a raw score (θX), standardized raw score (θXSDT), principal component score (θPC), factor score (θFA), theta2 score formed by the item response theory (IRT) or Rasch modeling (θIRT), or various non-linear combinations of the items (θNon-Linear).
If we assume that errors in the individual items do not correlate with each other, the error variance related to the compilation of the items is as follows:
In practical terms, the traditional measurement model takes the factor loading as the weight factor, and this leads to the following error variance related to the score variable:
Notably, the traditional model assumes that the weight factor wi, i.e., factor loading being a correlation coefficient, always gives accurate estimates. This assumption is too optimistic, as observed above, and the deflation in the estimate may be remarkable. However, if we select the correlation w wisely so that the magnitude of the mechanical error is as small as possible, that is, if we use some of the deflation-free or deflation-corrected estimators of correlation ( ), the outcome is deflation-free or near. The magnitude of the error component related to deflation may be near zero. This leads us to a deflation-corrected measurement model and, consequently, to deflation-corrected error variance as follows:
In practical terms, if using RPC, G, and D as the deflation-corrected estimators of association between an item i and the (undefined) latent variable θ, a theoretical deflation-corrected error variance based on RPC is as follows (Metsämuuronen, 2023):
and, based on G, it is as follows:
and based on D, it is as follows:
These estimators are used later in the hypothetical example and in the empirical section—except that instead of G and D, G2 and D2 are used in Equations (7) and (8) because they suit better polytomous settings; their computing is discussed later. Of these better-behaving estimators of association, RPC refers to a theoretical association in that it refers to theoretical (latent) items and scores that a researcher is not privy to (see the critique in Chalmers, 2017). G and D and the derivatives G2 and D2 refer to observed items and scores with a practical interpretation: the estimates strictly indicate the proportion of the test takers that are logically (ascending) ordered after they are ordered by the score variable; and (see Metsämuuronen, 2022i based on Metsämuuronen, 2021b). Of G and D, the estimates by D are more conservative in comparison with G because G omits the tied pairs in the computing proportions while D uses them (see Metsämuuronen, 2021b). In polytomous settings, the magnitude of the estimates by G2 tends to follow close to those by RPC and the estimates by D2 close to those by RREG (Metsämuuronen, 2022i).
Assume a hypothetical dataset, as in Table 1 with k = 5 items and incremental difficulty levels in items (p = 0.083–0.917) and n = 12 test takers. This could be a short subtest of “Sets” amid a larger mathematics achievement test given to a small group of students. Relevant indicators related to the traditional and deflation-corrected error variances are collected in Table 1. Four score variables are used: a raw score (θX), a standardized raw score (θXSTD), a factor score (θFA), and a theta score formed by the one-parameter logistic item response theory (1PL IRT) modeling or, factually, Rasch modeling (θIRT). The ML estimate is not optimal for the score variables because of the small sample size. However, it serves as an example of the computing process.
As an indicator of reliability, the coefficient omega total (ρω; later, just omega) based on the works of Heise and Bohrnstedt (1970) and McDonald (1970, 1999) also known as McDonald’s omega, is used. Omegas can be expressed as follows:
In the example of the possible outperforming estimators of correlation, RPC, G, and D and related deflation-corrected estimates of error variance are used as benchmarks for traditional factor loadings. Using G and D is justified because the items are binary (Metsämuuronen, 2020a,b, 2021a). From the viewpoint of the benchmarking estimators (Equations 6–8), using the raw score, standardized raw score, and 1PL model in IRT modeling leads to identical results because the order of the test takers does not change in the standardization and logistic transformation.
From the viewpoint of reliability estimates, the estimate by the traditional coefficient omega (Equation 8) is notably deflated as being = = 0.380. It appears that the factor score is not the best reflection of the true ability in the case. Namely, the related deflation-corrected estimate is based on the form of omega, and using RPC gives a deflated estimate of = = 0.483. In the hypothetical example, the estimates related to the raw score (and IRT score) appear more credible in comparison with the factor score, because items g1, g4, and g5 can deterministically distinguish test takers from each other when tied cases are not considered (RPC ≈ G = 1). The estimates are quite close when the tied cases are considered (D = 0.889–0.909). Factor analysis can detect this phenomenon only in g5 ( = 0.999) but fails notably in g1 ( = 0.091) and g4 ( = 0.522). Hence, we obtain the deflation in reliability by omega and inflation in the error variance.
If the raw score, standardized raw score, or IRT score are used as a justified reflection of the latent ability, the estimates of reliability would be notably higher by using RPC as the weight factor, = = 0.923, mildly higher if G was used ( = 0.932), and mildly lower if D was used ( = 0.869).
When comparing the standardized score variables in the hypothetical example, the deflation in the estimate by the traditional omega is 56% [=(0.869–0.380)/0.869 × 100] if the conservative D is taken as the benchmarking weight factor and 59% if RPC or G are taken as the benchmarks. The inflation in the traditional error variance based on the factor loadings is 30–41% when the factor score is considered and up to 76–182%, depending on the weight factor when the standardized raw score is considered. The magnitude of both deflation and inflation is notable and worth further investigation.
More in-depth analysis is discussed in Section 5, where a set of 1,440 real-life tests with various characteristics is used to explore the boundaries of the inflation in error variance.
From earlier studies, it is known that the traditional estimates of reliability tend to be deflated. The deflation may be radical (up to 0.40–0.70 units of reliability), and the reason for this deflation is the poor behavior of the product–moment coefficient of correlation in the case that the widths of the scales of the variables are far from each other. This is always the case in measurement modeling settings, and it is often exacerbated in achievement testing, where we are willing to use both very easy, medium, and very demanding tasks to cover the full range of ability scales in one test. In these types of tests, the standard errors related to the score are radically inflated; in some extremely easy or difficult tests, the standard errors have been reported to be more than 10 times higher than they should be.
Because the relationship between reliability and error variance and the standard error of the score can be easily observed from the formulae, the technical reasons for the observed radical deflation in the estimates of reliability can be traced to three sources: either the population variance ( ) is deflated, or the error variance ( ) is inflated—or both may happen at the same time. This article focuses on error variance, which is strictly embedded in widely used reliability estimators such as omega and maximal reliability (see Supplementary Appendix 1). In some empirical settings, it has been noted that the estimates of reliability may be deflated by 0.40–0.70 units, and this can be directly connected to mechanical errors in the estimation of correlation, which needs to be separated from attenuation related to violations against the measurement model. From this viewpoint, it appears that the phenomenon of radical inflation in error variance and measurement error caused by technical error during the estimation process is discussed sparsely in literature, if at all, considering its possible consequences (see, however, discussion in Metsämuuronen, 2023 related to achievement testing, and Metsämuuronen, 2022b,f, related to inflation in conditional standard errors). Hence, it seems justified to further discuss the reasons, mechanisms, and consequences of the deflation observed in the estimates of reliability and the inflation in error variance.
This note examines the magnitude and consequences of inflation in error variance estimates. The conceptual matters and reasons behind them are discussed in Section 1.3. The inflation in the error variance begs three key questions: (1) What is the magnitude of the inflation in the estimated error variance and the related standard errors in real-life testing settings? (2) How can the magnitude of inflation be predicted? and (3) How do deflation-corrected estimators of error variance and standard errors compare to traditional ones in real-life datasets? These questions are studied and discussed in the empirical section (Section 4) using a simulation dataset based on real-life settings.
A dataset of 4,023 nationally representative test-takers of a mathematics test with 30 binary items (FINEEC, 2018) is used as the “population.” From the original dataset, 10 samples with finite sample sizes of n = 25, 50, 100, and 200 test-takers were drawn. These samples imitate different real-life sample sizes, ranging from tests for a large student group (n = 200) to classroom settings (n = 25). In each of the 10 × 4 datasets, 36 tests were produced by varying the number of items in the tests, the difficulty levels of the items, and the length of the scales of the score [df(X) = number of categories in the score scale – 1] and the item [df(g) = number of categories in the item scale – 1]. Polytomous items were produced as a combination of binary items. In the final dataset, both the tests with the original items and the tests with fewer items but wider scales are mixed. Datasets comprising the traditional and deflation-corrected estimates of reliability, the estimates of error variance and standard errors and estimated population variances, and related derivatives and background information of the 1,440 tests are available at doi: 10.13140/RG.2.2.25390.79687 in CSV format and at doi: 10.13140/RG.2.2.33779.40481 in IBM SPSS format.
Because we are using both binary and polytomous items, instead of G and D, their dimension-corrected modifications (G2 and D2) are used. It is known that when the number of categories in the item exceeds 3 (D) or 4 (G), G and D tend to underestimate the item-score association (see, e.g., Metsämuuronen, 2020a,b, 2021a). Hence, Metsämuuronen (2021a) suggests modifications specific to the measurement modeling settings as follows: and , where G and D are the observed values of G and D and . With binary items, df(g) = 1, and A = 0, and, hence, G2 = G and D2 = D. Moreover, when G = D = 1, G2 = G, and D2 = D.
For the note, the estimates by G2 and D2 were computed manually from the values of G and D being standard outputs of a statistical software package (in the case of IBM SPSS; see syntaxes with some generally known packages in Supplementary Appendix 2).
In assessing the magnitude of the inflation in the estimates of error variance, a simple statistic is used: the difference between the traditional estimate and the deflation-corrected estimates. The traditional estimates are denoted or “VAR(E)_LFA” as an abbreviation of “error variance based on factor loadings as the linking factor and the factor score variable as the manifestation of the latent score estimated by using the maximum likelihood extraction method.” Correspondingly, the deflation-corrected estimators are denoted , , or “VAR(E)_RPCX,” “VAR(E)_G2X,” and “VAR(E)_D2X,” respectively as abbreviations of “error variance based on RPC/G2/D2 as the linking factor and the raw score as the manifestation of the latent score.” The “written” version is seen, specifically in Figures to come. While traditional estimates are based on factor score variables, the latter estimates are based on raw scores. We may also note that the result would be equal if the standardized raw scores or IRT scores were used because the estimates of the item–score association by the deflation-corrected estimators of correlation are equal with the raw scores, standardized scores, and IRT scores because the order of the test takers does not change in these transformations.
A difference (“d”) between the sample estimates of the traditional estimate of error variance and the deflation-corrected estimate reflects the magnitude of inflation. This difference is noted as follows: or “dVAR(E)_RPCX” refers to inflation in when is used as the benchmark. Similarly, “dVAR(E)_G2X” or “dVAR(E)_D2X” refers to the inflation in the case G2 or D2 have been used as the deflation-corrected estimator of weight factor wi. Technically,
Then, if the magnitude of is positive, the traditional estimated error variance of the score is overestimated. In some cases, this is expressed as percentages, which are notated using “dp” such as in . The percentages are computed so that the deflation-corrected estimate is the base; the percentage indicates the deflation in the traditional estimate, assuming that the deflation-corrected estimate represents the true value.
However, using the percentages is not necessarily wise to connect to the phenomenon because the magnitude of the error variance appears to vary radically depending on the number of items in the compilation. With two or three items, the magnitude of the error variances could be 0.2 and 0.6, leading to = 200; that is, the error variance seems to be inflated by 200%. If the difference is notably greater, such as 20 or 30, inflation would be only 50%.
The magnitude of the inflation is illustrated by using visual tools. The explaining factors are studied using standard linear regression modeling, and linear and non-linear graphical modeling is used with two variables. Decision tree analysis (DTA; IBM, 2017), a data mining tool with the CHAID algorithm (Chi-square Automatic Interaction Detector; Kass, 1980), explores the variables and groups the categories. In DTA, the outcome is a non-linear hierarchical model based on maximizing the F-test (or χ2) statistics; all possible combinations of the explaining factors are computed, and the statistically best combination is selected. This tool is used when the number of items in the compilation explaining the error variance is of interest. A paired-sample t-test is used to compare mean differences in standard errors, and Cohen’s d (Cohen, 1988) is used to indicate effect size.
Four lifts are made regarding the magnitude of the error variance in real-life settings. First, with the smallest sample size in the simulation (n = 25), it was not possible to produce all the factor models. While it was possible to produce 360 estimates of error variance related to the deflation-corrected correlation estimators, the factor solution was found only in 314 out of 360 tests. This loss of 12.5% in the group of the smallest sample size is systematic in that the error variances were missing with binary items and tests with more than 24 items; that is, in the settings where the inflation was the greatest (see Figure 2). Hence, in Figure 2, only estimates with sample sizes of n ≥ 50 (n = 1,080 tests out of 1,440) can be observed. Later, all possible estimates are used, that is, n = 1,394 for the traditional estimates and n = 1,440 for the deflation-corrected estimates. In the pairwise comparisons, only 1,394 pairs are available.
Figure 2. Magnitude of the error variance by the number of items in the compilation (sample sizes n = 50, 100, and 200) in groups suggested by DTA.
VAR(E)_LFA = error variance based on factor loading (L) as the linking factor and a one-factor factor score variable (FA) estimated by using maximum likelihood estimation as the manifestation of the latent score. VAR(E)_RPCX, VAR(E)_G2X, and VAR(E)_D2X = error variance based on RPC/G2/D2 as the linking factor and the raw score (X) as the manifestation of the latent score.
Second, the dataset used in the simulation did not include tests with 16–19 items or tests longer than 30 items. Technicalities in forming the dataset used in simulation led to practicalities such as the test with 20–30 items being based on binary items and the test with 2–15 items being polytomous items (Figure 2). Notably, some categories of k in Figure 2 are combined, as suggested by DTA with the CHAID algorithm; using these groups, the difference between the categories is the most statistically significant [for the traditional estimates, F(13, 1,066) = 14,768.58, p < 0.001]. In Figure 2, n refers to the number of tests; n = 60 indicates that the dataset consists of 60 tests compiled of 28–30 items.
Third, the magnitude of the error variance increases systematically with the number of items comprising the test (k). This is known from Eqs. (3) to (5), and it [i.e., the phenomenon in Eqs 3−5], is understandable because the error variance of the test is a cumulative sum of error variances of the single items. While the traditional error variance (“VAR(E)_LFA”) is approximately 0.5–2.2 units with tests with few items (k = 2–5), with 20–30 binary items, it is 15.9–23.4 units. The number of items in the compilation explains almost all error variance variability; using the linear regression model, R2 > 0.99 for the traditional error variance, and if using the deflation-corrected estimators of association, R2 > 0.98 (Figure 3).
Figure 3. Models of the magnitude of the error variance by the number of items in the compilation (all tests).
VAR(E)_LFA = the traditional error variance based on factor loading (L) as the weight factor and factor score variable (FA) from a one-factor solution estimated by using maximum likelihood estimation as the manifestation of the latent ability. VAR(E)_RPCX, Deflation-corrected error variance based on RPC as the weight factor and the raw score (X) as the manifestation of the latent ability.
Fourth, all the estimators of deflation-corrected error variance give estimates that are systematically smaller in magnitude than traditional estimates. While, in the given dataset, the traditional estimates related to the factor score variable and factor loadings tend to range from 0.5 to 23.4 units, depending on the number of items in the compilation, the deflation-corrected estimates related to the raw score range from 0.2 to 17.8 units. It may be possible that the lower magnitude of the error variance related to the deflation-corrected estimates could be partly explained by the difference in the score variable; after all, the score variables differ between the estimators. However, traditionally, factor score variable has been taken as one of the “optimal linear combinations” discussed over years by, chronologically, e.g., Thompson (1940), Guttman (1941), Stouffer (1950), Lord (1958), and Bentler (1968) and later, for example, Li et al. (1996) and Li (1997); the “optimal” combination should be, logically, better than the raw score and, hence, it should include less error in comparison with the raw score. However, the studies with deflation-corrected estimators of reliability have shown that the reason for the deflation is mainly in estimates of the association between the item and score variable (see the discussion above) rather than in the difference between the score variables (see Metsämuuronen, 2022b of the effects of different sources of underestimation of reliability).
As shown in Figures 2 and 3, the inflation in the error variance tends to become greater the more items there are in the compilation. Figures 4A,B and 5 further exploit the same finding: Figures 4A,B use factual estimates, and Figure 5 uses the means of error variance in the compiled groups of the number of items in the compilation suggested by DTA. Three major points are highlighted.
Figure 4. (A) Magnitude of the inflation in the error variance based on RPC (linear and non-linear models); all tests (left) and separated by the type of the tests (right). dVAR(E)_RPCX = VAR(E)_RPCX − VAR(E)_LFA, i.e., the difference (d) between the error variance based on the deflation-corrected and traditional estimates of error variance, that is, error variance based on RPC as the weight factor and raw sum (X) as the manifestation of the latent ability and error variance based on the factor loading (L) as the weight factor and factor score variable (FA) from a one-factor solution as the manifestation of the latent ability. (B) The magnitude of the inflation in the error variance based on G2 and D2 (linear and non-linear models); all tests. dVAR(E)_G2X = VAR(E)_G2X − VAR(E)_LFA, i.e., the difference (d) between the error variance based on the deflation-corrected and traditional estimates of error variance, that is, error variance based on G2 as the weight factor and raw sum (X) as the manifestation of the latent ability and error variance based on the factor loading (L) as the weight factor and factor score variable (FA) from a one-factor solution as the manifestation of the latent ability. Similarly, dVAR(E)_D2X = VAR(E)_D2X − VAR(E)_LFA based on D2 as the weight factor.
Figure 5. Magnitude of the inflation in the error variance by the number of items (n refers to the number of tests).
dVAR(E)_RPCX = VAR(E)_RPCX − VAR(E)_LFA, dVAR(E)_G2X = VAR(E)_G2X − VAR(E)_LFA, and dVAR(E)_D2X = VAR(E)_D2X − VAR(E)_LFA, i.e., the difference (d) between the error variance based on the deflation-corrected and traditional estimates of error variance, that is, error variance based on RPC/G2/D2 as the weight factor and raw sum (X) as the manifestation of the latent ability and error variance based on the factor loading (L) as the weight factor and factor score variable (FA) from a one-factor solution as the manifestation of the latent ability.
First, the models of the magnitude of the inflation may be different for binary items and polytomous items. This is illustrated in Figure 4A with RPC: the magnitude of the slope parameter with binary items is 0.209, and with the polytomous items, it is 0.155. In the dataset used in the simulation, the polytomous items were dependent on the binary items; after all, the polytomous items were formed as combinations of binary items. Systematic studies with independent polytomous and binary items would be valuable in confirming this phenomenon.
Second, in the simulation dataset, a linear model of inflation = 0.2 × k – 0.3 explains well the magnitude of inflation when the benchmarking estimator is based on RPC and inflation = 0.2 × k – 0.2 when G2 is the benchmark; that is, the estimates tend to be somewhat higher when using G2 than RPC (Figure 4B). The model for the conservative estimates by D2 have a smaller magnitudes in the slope parameter and constant (inflation = 0.17 × k – 0.25). In all cases, the explaining power for a linear model is high (R2 = 0.94–0.97), although the models with a second power give slightly better explaining powers (R2 = 0.96–0.98) (Figure 4B).
Third, not only is the error variance cumulative by the number of items (see Figures 4A,B), but the inflation in the error variance is also cumulative by the number of items. With 2–4 items, the error variance ranges from 0.3 to 0.5 regardless of the benchmarking deflation-corrected estimator, while with 30 binary items, the inflation in the error variance ranges 4.7–5.4 units depending on the benchmarking estimator (Figure 5). The technical reason for the phenomenon is that they tend to give estimates with a higher magnitude than PMC because of the better behavior of the deflation-corrected estimators of association. This is understood by the common characteristics of the deflation-corrected estimators of correlation, which give higher estimates than the traditional deflation-prone estimators. Because the error variance is cumulative, the more items we have in the compilation, the more cumulative error we obtain.
The magnitude of inflation was studied with linear regression analysis by using five factors related to the tests: the sample size (n), the test difficulty assessed by the average item difficulty ( ), the number of items (k), the number of categories in the score [df(g)], and the score [df(X)]. However, because the number of items alone explains 96.4–97.5% of the variability in inflation by the quadratic model ( = 0.964–0.975), the other factors cannot add much information to the model. Factually, in all conjoint models, different combinations of other elements increase the explaining power statistically significantly, but the final explaining power of the more complicated linear model after Wherry’s adjustment is lower ( = 0.952–0.974) than in the models with only one explaining factor without a need for the adjustment. Hence, these models are not included in this note. However, Table 2 condenses an example of the impact of different factors in a conjoint linear model where RPC is used as a deflation-corrected estimator of association. By using RPC in the correction, a number of items alone explain 97.7% (by quadratic model) or 96.8% (by linear model) of the variability in inflation. The whole linear model explains 97.4%. Notably, the relationship is not linear (see Figure 5).
Table 2. Conjoint model of relevant factors explaining the inflation on error variance; dependent variable: dVAR(E)_RPCX.
As discussed above, inflation in the error variance is strictly linked to the deflation of reliability. Another direct consequence is that the estimated standard errors are inflated. The more the item–score correlations are deflated, the more the reliability estimates are deflated, and, consequently, because of Equation (1), the more the standard errors are inflated (see the discussion in Metsämuuronen, 2023). The relation between the inflated error variance and inflated standard errors is somewhat more complicated than the inflation in error variance itself.
Taking the form of coefficient omega (Equation 9) as an example, the deflation in reliability depends not only on the inflated error variance but also on the other component related to “true variance” , which is deflated when the traditional factor loadings are considered; these two elements are intertwined. If using the basic formula for the S.E.m. (based on Equation 1) with deflation-corrected estimators of correlation in estimation (e.g., and ) and related deflation-corrected estimators of reliability (DCER; Metsämuuronen, 2022c,d,e), we obtain deflation-corrected standard errors (S.E.m._DC).
The inflation in the standard errors is studied by using the coefficient omega as an example of an estimator of reliability. The traditional omega is used to estimate the reliability of a factor score, which is a standardized variable with = 1, and, hence, the traditional estimator of S.E.m using omega is . The corresponding DCERs, “OmegaRPC,” “OmegaG2,” and “OmegaD2,” use the form of Equation (6) as the base and RPC, G2, and D2 as the weight factors (see, e.g., Metsämuuronen, 2022d). However, the score variable in the datasets used in the simulation was originally the raw score. To compare estimated standard errors, without losing generalizability, we can assume that the raw scores were standardized; a correlation between an item and a raw score is identical to the correlation between an unstandardized item and a standardized raw score. Then, the deflation-corrected standard errors based on RPC are computed as follows: , which is abbreviated in the figures to come as “SEM_RPC_STD,” referring to “standard error based on the formula of omega and using RPC as the weight factor and standardized raw score (STD) as the manifestation of the latent ability.”
Similarly, the deflation-corrected standard errors based on G2 are computed as follows: . It is abbreviated as “SEM_G2_STD.”
The deflation-corrected standard errors based on D2 are computed as follows: . It is abbreviated as “SEM_G2_STD.” The notations (“OmegaRPCSTD”), (“OmegaG2STD”), and (“OmegaD2STD”) indicate that the base of the estimator of reliability is omega (Equation 9), the weight factor wi is operationalized as RPC, G2, or D2, and the latent score variable is manifested as the standardized raw score (θXSTD). Hence, the standard errors related to the factor score variables and the standard errors of standardized raw scores are compared. Understandably, the outcome is not exact, but it gives us a rough idea of the magnitude of the inflation in standard errors.
In the datasets used in the simulation, the average S.E.m by using the traditional omega is 0.38 standard units, while the deflation-corrected standard errors using the deflation-corrected estimators of association with the formula of omega vary by 0.26–0.28, depending on the weight factor. Hence, on average, the traditional standard errors are inflated by 35–48% (Figure 6). The difference is statistically significant (paired-samples t-test, t = 112.39–128.40; p < 0.001) and remarkable or “huge” (Cohen’s d = 3.20–3.40; see Sawilowsky, 2009). The modest inflation in comparison with the datasets by Metsämuuronen (2022b,f) is caused by the fact that the dataset used does not contain many items with extreme difficulty levels, and, hence, the deflation in the estimates of reliability is modest: ρω = 0.85 by using the traditional omega vs. ρω_wiθ = 0.92–0.93 by using DCERs, that is, 7–8%. Notably, in the extremely easy dataset discussed by Metsämuuronen (2022b) (originally in Metsämuuronen and Ukkola, 2019), the deflation in the estimates by omega was 53–57% (ρω = 0.42 by omega vs. ρω_wiθ = 0.87–0.97 by DCERs). In a real-life setting by Metsämuuronen (2022f), the deflation in reliability with easy items was 68–69% (ρω = 0.29 by omega vs. 0.86–0.90 by DCERs using G and D).
Figure 6. General tendencies of the traditional and deflation-corrected standard errors.
SEM omega_L_FA traditional, Traditional standard errors based on the estimates of reliability by coefficient omega using factor loadings (L) as weight factors in estimation. SEM omega_RPC_STD deflation-corrected, Deflation-corrected standard errors based on the estimates of reliability by coefficient omega using RPC between items and the standardized raw score as weight factors in estimation; SEM omega_G2_STD deflation-corrected, Deflation-corrected standard errors based on the estimates of reliability by coefficient omega using G2 between items and the standardized raw score as weight factors in estimation; SEM omega_D2_STD deflation-corrected, Deflation-corrected standard errors based on the estimates of reliability by coefficient omega using D2 between items and the standardized raw score as weight factors in estimation.
Even though the error variance by Equations (4, 6–8) is directly related to the number of items in the compilation, the magnitude of the standard error by Equation (1) is not systematically related to the number of items in the compilation, although it tends to become smaller the wider the scales of the item and score (Figure 7). In Figure 7, the abbreviations “SEM L_FA,” “SEM RPC_STD,” “SEM G2_STD,” and “SEM D2_STD” refer to standard errors (SEM) estimated either by the traditional way by using coefficient omega with factor loadings (L_FA) or by using the formula of omega with deflation-corrected estimators of association (RPC/G2/D2) and standardized raw scores (STD). Formally, the DCERs are , , and , where the base of the estimator of reliability is omega (Equation 9), the weight factor wi is operationalized as RPC, G2, or D2, and the latent score variable is manifested as the standardized raw score (θXSTD).
Figure 7. Selected factors explaining the inflation in standard errors.
SEM L_FA, Traditional standard errors (SEM) estimated using coefficient omega with factor loadings (L_FA). SEM RPC_STD, SEM G2_STD, SEM D2_STD, Deflation-corrected standard errors (SEM) estimated using the formula of omega with deflation-corrected estimators of association (RPC/G2/D2) and standardized raw scores (STD).
We have presented two approaches to computing the average standard error. On the one hand, we have the traditional S.E.m. based on the definition of reliability of the score (Equation 1), that is,
This implies and determines that the standard error cannot exceed the magnitude of the standard deviation ( ) related to the score because REL ≤ 1. With a standardized score with = 1, according to Equation (11), the variance of the score can be divided into reliable variance (reliability, REL) and unreliable variance ( ), which together do not exceed the value 1, that is, REL + = 1.3
On the other hand, we can compute the “standard errors” based on the measurement model related to factor models by using Equation (3), that is,
Even if this statistic is based on standardized score variables, it does not produce “standard errors” in the same metric as does the traditional formula (Equation 1), and the outcomes may differ radically from each other (see Figure 7). For example, with 100 items of wi = 0.4 in each, the latter form leads to = 7.7 regardless of the reliability. The standard errors by Equation (11) and the “standard errors” by Equation (12) do not speak of the same thing.
The starting point of this note was the deflation in the reliability estimates. The term error variance related to the general one-factor measurement model ( ) is embedded in classical reliability estimators such as coefficient omega and rho (maximal reliability). The traditional measurement model assumes that the weight factor wi does not include technical or mechanical error. However, previous studies related to deflation in correlation estimates indicate that this is not true. If factor loadings are used as the weight coefficient wi as they are with the traditional omega and rho, the error variance is always overestimated because factor loading is essentially a product–moment coefficient of correlation between the item and the score, and PMC is one of those estimators of correlation that are especially prone to deflation. Deflation-corrected estimators are obtained when, instead of PMC, some alternative, a better-behaving correlation estimator, such as polychoric correlation, Goodman–Kruskal gamma, or Somers delta, is used in the estimation.
Under the assumption of the one-factor measurement model, the error variance tends to be overestimated as the number of items on the test increases. This can also be derived from the error variance formula. Moreover, the inflation in the traditional error variance tends to grow by the number of items in relation to deflation-corrected estimators of error variance. The technical reason for the phenomenon is that, because of the better behavior of the deflation-corrected estimators of association, they tend to give estimates with a higher magnitude than PMC. The common characteristic of the deflation-corrected correlation estimators is that they give higher estimates than the traditional deflation-prone estimators. Because the error variance is cumulative, the more items we have in the compilation, the more cumulative error we obtain.
An obvious consequence of the inflated error variance is that the standard errors of the measurement are also inflated when the traditional reliability estimators are used. If the deflation-corrected reliability estimators are used, the consequent deflation-corrected standard errors may be notably lower. In the dataset used in the empirical section, the inflation was 35–48%, depending on the benchmarking coefficient of association. However, the deflation may be radically greater in magnitude if the difficulty levels of the items were extreme. This is typical in the tests within educational settings with achievement testing because, usually, the tests include both easy, medium, and difficult items.
An obvious limitation in the empirical section is that the treatment was based on one real-world dataset with certain limitations: the latent reliability was not controlled, only small sample sizes were used, tests with more than 30 and less than 10 categories in the score were missing, and no tests with extreme difficulty levels or very short tests were included in the dataset used in the simulation. Systematic studies of the phenomenon would enrich our understanding of the nature of inflation in terms of error variance and standard error.
The theoretical basis for the deflation-corrected standard errors is somewhat underdeveloped. The estimators discussed in this article are mainly short-cuts where the poorly behaved Rit is replaced by better-behaving coefficients. However, these deflation-corrected estimators are theoretical because no such factor analysis routine currently exists that would yield some of the deflation-corrected estimators of association between an item and a score instead of the traditional product–moment coefficient of correlation (PMC). Of the alternative estimators of association, using RPC and RREG leads to theoretical standard errors because the outcome of deflation-corrected reliability by using RPC or RREG instead of the traditional estimator would lead us to infer something from the theoretical score that researchers do not have access to (see Chalmers, 2017; Metsämuuronen, 2022d). The other alternatives suggested by Metsämuuronen (2022a), G, G2, D, D2, RAC, and EAC, refer to observed scores and items.
This note is restricted to classical estimators of reliability. Consequently, we do not know much about how applicable the results would be with estimators of reliability within generalizability theory, confirmatory factor analysis (CFA), structural equation modeling (SEM), or IRT and Rasch modeling (see related discussion and literature in Metsämuuronen, 2022d).
Finally, this article aims to explore the reasons for, implications of, and factors related to the empirical finding discussed by Metsämuuronen (2023) that certain types of test settings, common in educational testing settings with widely varying levels of item difficulty, are prone to producing standard errors that may be vastly overestimated. The results of this note enrich our understanding of the factors associated with this phenomenon. Since inflation in the standard error tends to increase with the number of items and the traditional tenet in testing settings is that reliability increases with the number of items, there may be an apparent tension between these tendencies. Since the deflation-corrected estimates of reliability could be used to assess the magnitude of inflation, it is strongly suggested that the estimates of the DCERs be reported alongside traditional reliability estimates for a more comprehensive evaluation.
The datasets presented in this study can be found in online repositories. The names of the repository/repositories and accession number(s) can be found below: datasets comprising the traditional and deflation-corrected estimates of reliability, the estimates of error variance and standard errors and estimated population variances, and related derivatives and background information of the 1,440 tests are available at http://dx.doi.org/10.13140/RG.2.2.25390.79687 in CSV format and at http://dx.doi.org/10.13140/RG.2.2.33779.40481 in IBM SPSS format.
Ethical approval was not required for the studies involving humans because according to the law in Finland, the national achievement test results can be used in research purposes by application. The datasets are always anonymized. Part of the simulation dataset is based on old dataset. The studies were conducted in accordance with the local legislation and institutional requirements. Written informed consent for participation was not required from the participants or the participants’ legal guardians/next of kin in accordance with the national legislation and institutional requirements.
The author confirms being the sole contributor of this work and has approved it for publication.
The author declares that the research was conducted in the absence of any commercial or financial relationships that could be construed as a potential conflict of interest.
All claims expressed in this article are solely those of the authors and do not necessarily represent those of their affiliated organizations, or those of the publisher, the editors and the reviewers. Any product that may be evaluated in this article, or claim that may be made by its manufacturer, is not guaranteed or endorsed by the publisher.
The Supplementary material for this article can be found online at: https://www.frontiersin.org/articles/10.3389/feduc.2024.1248770/full#supplementary-material
1. ^Notably, although coefficient eta uses different information in comparison with Rit, in the binary case, their formulae are identical (see Wherry and Taylor, 1946; see also Metsämuuronen, 2022g).
2. ^It may cause some confusion that the tradition within IRT and Rasch modeling uses “theta” as a general name for the observed score variable. While logically consistent, it creates a tension between the notation used within the article, where “theta” refers to the latent variable rather than the observed variable. To resolve this tension, “theta” is written with a subscript when referring to the manifestation of the latent variable (e.g., θX or θIRT), while the latent variable itself is denoted by the Greek letter θ.
3. ^Sincere thanks to PhD Christian Geiser from QuantFish LLC for reminding me of this in a private discussion concerning the matter.
Bentler, P. M. (1968). Alpha-maximized factor analysis (Alphamax): its relation to alpha and canonical factor analysis. Psychometrika 33, 335–345. doi: 10.1007/BF02289328
Bentler, P. M. (2009). Alpha, dimension-free, and model-based internal consistency reliability. Psychometrika 74, 137–143. doi: 10.1007/s11336-008-9100-1
Chalmers, R. P. (2017). On misconceptions and the limited usefulness of ordinal alpha. Educ. Psychol. Meas. 78, 1056–1071. doi: 10.1177/0013164417727036
Cheng, Y., Yuan, K.-H., and Liu, C. (2012). Comparison of reliability measures under factor analysis and item response theory. Educ. Psychol. Meas. 72, 52–67. doi: 10.1177/0013164411407315
Cho, E., and Kim, S. (2015). Cronbach’s coefficient alpha: well known but poorly understood. Organ. Res. Methods 18, 207–230. doi: 10.1177/1094428114555994
Cramer, D., and Howitt, D. (2004). The Sage Dictionary of Statistics. A Practical Resource for Students. London: SAGE Publications, Inc.
Cronbach, L. J. (1951). Coefficient alpha and the internal structure of tests. Psychometrika 16, 297–334. doi: 10.1007/BF02310555
Davenport, E. C., Davison, M. L., Liou, P.-Y., and Love, Q. U. (2015). Reliability, dimensionality, and internal consistency as defined by Cronbach: distinct albeit related concepts. Educ. Meas. Issues Pract. 34, 4–9. doi: 10.1111/emip.12095
Davenport, E. C., Davison, M. L., Liou, P.-Y., and Love, Q. U. (2016). Easier said than done: rejoinder on Sijtsma and on Green and Yang. Educ. Meas. Issues Pract. 35, 6–10. doi: 10.1111/emip.12106
Dunn, T. J., Baguley, T., and Brunsden, V. (2013). From alpha to omega: a practical solution to the pervasive problem of internal consistency estimation. Br. J. Psychol. 105, 399–412. doi: 10.1111/bjop.12046
Falk, C. F., and Savalei, V. (2011). The relationship between unstandardized and standardized alpha, true reliability, and the underlying measurement model. J. Pers. Assess. 93, 445–453. doi: 10.1080/00223891.2011.594129
FINEEC (2018). National assessment of learning outcomes in mathematics at grade 9 in 2002 (Unpublished dataset opened for the re-analysis 18.2.2018). Finnish education evaluation centre.
Foy, P., and LaRoche, S. (2019). Estimating standard errors in the TIMSS 2019 results. Ch. 14 in TIMSS 2019 Technical Report. eds. M. O. Martin, M. von Davier, & I.V.S. Mullis, Available at: https://timssandpirls.bc.edu/timss2019/methods/chapter-14.html (Accessed September 4, 2022).
Gadermann, A. M., Guhn, M., and Zumbo, B. D. (2012). Estimating ordinal reliability for Likert-type and ordinal item response data: a conceptual, empirical, and practical guide. Pract. Assess. Res. Eval. 17, 1–13. doi: 10.7275/n560-j767
Goodman, L. A., and Kruskal, W. H. (1954). Measures of association for cross classifications. J. Am. Stat. Assoc. 49, 732–764. doi: 10.1080/01621459.1954.10501231
Green, S. B., and Yang, Y. (2009). Commentary on coefficient alpha: a cautionary tale. Psychometrika 74, 121–135. doi: 10.1007/s11336-008-9098-4
Green, S. B., and Yang, Y. (2015). Evaluation of dimensionality in the assessment of internal consistency reliability: coefficient alpha and omega coefficients. Educ. Meas. Issues Pract. 34, 14–20. doi: 10.1111/emip.12100
Guttman, L. (1941). “The qualifications of a class of attributes: a theory and method of scale construction” in The prediction of personal adjustment. Social Science Research Council, Bulletin 48. (ed.) P. Horst, 321–345.
Guttman, L. (1945). A basis for analyzing test-retest reliability. Psychometrika 10, 255–282. doi: 10.1007/BF02288892
Heise, D., and Bohrnstedt, G. (1970). Validity, invalidity, and reliability. Sociol. Methodol. 2, 104–129. doi: 10.2307/270785
Henrysson, S. (1963). Correction of item–total correlations in item analysis. Psychometrika 28, 211–218. doi: 10.1007/BF02289618
Hoekstra, R., Vugteveen, J., Warrens, M. J., and Kruyen, P. M. (2019). An empirical analysis of alleged misunderstandings of coefficient alpha. Int. J. Soc. Res. Methodol. 22, 351–364. doi: 10.1080/13645579.2018.1547523
IBM (2017). IBM SPSS Decision Trees 25. IBM. Available at: https://www.ibm.com/docs/en/SSLVMB_25.0.0/pdf/en/IBM_SPSS_Decision_Trees.pdf (Accessed September 4, 2022).
Jackson, R. W. B., and Ferguson, G. A. (1941). Studies on the reliability of tests. Department of Educational Research, University of Toronto.
Kass, G. (1980). An exploratory technique for investigating large quantities of categorical data. Appl. Stat. 29, 119–127. doi: 10.2307/2986296
Kuder, G. F., and Richardson, M. W. (1937). The theory of the estimation of test reliability. Psychometrika 2, 151–160. doi: 10.1007/BF02288391
Li, H. (1997). A unifying expression for the maximal reliability of a linear composite. Psychometrika 62, 245–249. doi: 10.1007/BF02295278
Li, H., Rosenthal, R., and Rubin, D. B. (1996). Reliability of measurement in psychology: from Spearman-Brown to maximal reliability. Psychol. Methods 1, 98–107. doi: 10.1037/1082-989X.1.1.98
Livingston, S. A., and Dorans, N. J. (2004). A graphical approach to item analysis. Research Report No. RR-04-10. Educational Testing Service. doi: 10.1002/j.2333-8504.2004.tb01937.x
Lord, F. M. (1958). Some relations between Guttman’s principal component scale analysis and other psychometric theory. Psychometrika 23, 291–296. doi: 10.1007/BF02289779
Martin, W. S. (1973). The effects of scaling on the correlation coefficient: a test of validity. J. Mark. Res. 10, 316–318. doi: 10.1177/002224377301000315
Martin, W. S. (1978). Effects of scaling on the correlation coefficient: additional considerations. J. Mark. Res. 15, 304–308. doi: 10.1177/002224377801500219
McDonald, R. P. (1970). Theoretical canonical foundations of principal factor analysis, canonical factor analysis, and alpha factor analysis. Br. J. Math. Stat. Psychol. 23, 1–21. doi: 10.1111/j.2044-8317.1970.tb00432.x
McNeish, D. (2017). Thanks coefficient alpha, we’ll take it from here. Psychol. Methods 23, 412–433. doi: 10.1037/met0000144
Meade, A. W. (2010). “Restriction of range” in Encyclopedia of Research Design. ed. N. J. Salkind (London: SAGE Publications, Inc.), 1278–1280.
Mendoza, J. L., and Mumford, M. (1987). Corrections for attenuation and range restriction on the predictor. J. Educ. Stat. 12, 282–293. doi: 10.3102/10769986012003282
Metsämuuronen, J. (2016). Item–total correlation as the cause for the underestimation of the alpha estimate for the reliability of the scale. Glob. J. Res. Analy. 5, 471–477.
Metsämuuronen, J. (2017). Essentials of Research Methods in Human Sciences. New Delhi: SAGE Publications, Inc.
Metsämuuronen, J. (2020a). Somers’ D as an alternative for the item–test and item–rest correlation coefficients in the educational measurement settings. Int. J. Educ. Methodol. 6, 207–221. doi: 10.12973/ijem.6.1.207
Metsämuuronen, J. (2020b). Dimension-corrected Somers’ D for the item analysis settings. Int. J. Educ. Methodol. 6, 297–317. doi: 10.12973/ijem.6.2.297
Metsämuuronen, J. (2021a). Goodman–Kruskal gamma and dimension-corrected gamma in educational measurement settings. Int. J. Educ. Methodol. 7, 95–118. doi: 10.12973/ijem.7.1.95
Metsämuuronen, J. (2021b). Directional nature of Goodman-Kruskal gamma and some consequences. Identity of Goodman-Kruskal gamma and Somers delta, and their connection to Jonckheere-Terpstra test statistic. Behaviormetrika 48:283–307. doi: 10.1007/s41237-021-00138-8
Metsämuuronen, J. (2022a). Effect of various simultaneous sources of mechanical error in the estimators of correlation causing deflation in reliability. Seeking the best options of correlation for deflation-corrected reliability. Behaviormetrika 49, 91–130. doi: 10.1007/s41237-022-00158-y
Metsämuuronen, J. (2022b). How to obtain the most error-free estimate of reliability? Eight sources of underestimation of reliability. Pract. Assess. Res. Eval. 27:1–27. doi: 10.7275/7nkb-j673
Metsämuuronen, J. (2022c). Deflation-corrected estimators of reliability. Front. Psychol. 12:748672. doi: 10.3389/fpsyg.2021.748672
Metsämuuronen, J. (2022d). Typology of deflation-corrected estimators of reliability. Front. Psychol. 13:891959. doi: 10.3389/fpsyg.2022.891959
Metsämuuronen, J. (2022e). Attenuation-corrected reliability and some other MEC-corrected estimators of reliability. Appl. Psychol. Meas. 46:720–737. doi: 10.1177/01466216221108131
Metsämuuronen, J. (2022f). Reliability for a score compiled from multiple booklets with equated scores. ResearchGate [Preprint]. doi: 10.13140/RG.2.2.20880.69120/2
Metsämuuronen, J. (2022g). Artificial systematic attenuation in eta squared and some related consequences. Attenuation-corrected eta and eta squared, negative values of eta, and their relation to Pearson correlation. Behaviormetrika, 50:27–61. doi: 10.1007/s41237-022-00162-2
Metsämuuronen, J. (2022h). Note on the deflation in population variance in the measurement modelling settings. ResearchGate [Preprint]. doi: 10.13140/RG.2.2.31887.87202
Metsämuuronen, J. (2022i). Rank–polyserial correlation: quest for a “missing” coefficient of correlation. Front. Appl. Math. Stat. 8:914932. doi: 10.3389/fams.2022.914932
Metsämuuronen, J. (2023). Seeking the real reliability. Why the traditional estimators of reliability usually fail in achievement testing and why the deflation-corrected coefficients could be better options. Pract. Assess. Res. Eval. 28:10. doi: 10.7275/pare.1264
Metsämuuronen, J., and Ukkola, A. (2019). Methodological solutions of zero level assessment. Publications 18: 2019. Finnish education evaluation Centre. [in Finnish, abstract in English] Available at: https://www.karvi.fi/sites/default/files/sites/default/files/documents/KARVI_1819.pdf
Moses, T. (2017). A review of developments and applications in item analysis in Advancing Human Assessment. The Methodological, Psychological and Policy Contributions of ETS. (eds.) R. Bennett and von Davier M. (USA: Springer Open), 19–46
Novick, M. R., and Lewis, C. (1967). Coefficient alpha and the reliability of composite measurement. Psychometrika 32, 1–13. doi: 10.1007/BF02289400
Olsson, U. (1980). Measuring correlation in ordered two-way contingency tables. J. Mark. Res. 17, 391–394. doi: 10.1177/002224378001700315
Pearson, K. (1900). I. Mathematical contributions to the theory of evolution. VII. On the correlation of characters not quantitatively measurable. Philos. Trans. R. Soc. A Math. Phys. Eng. Sci. 195, 1–47. doi: 10.1098/rsta.1900.0022
Pearson, K. (1903). I. Mathematical contributions to the theory of evolution. XI. On the influence of natural selection on the variability and correlation of organs. Philos. Trans. R. Soc. A Math. Phys. Eng. Sci. 200, 1–66. doi: 10.1098/rsta.1903.0001
Pearson, K. (1913). On the measurement of the influence of “broad categories” on correlation. Biometrika 9, 116–139. doi: 10.1093/biomet/9.1-2.116
Raykov, T. (1997). Scale reliability, Cronbach's coefficient alpha, and violations of essential tau-equivalence for fixed congeneric components. Multivar. Behav. Res. 32, 329–354. doi: 10.1207/s15327906mbr3204_2
Raykov, T., and Marcoulides, G. A. (2017). Thanks coefficient alpha, we still need you! Educ. Psychol. Meas. 79, 200–210. doi: 10.1177/0013164417725127
Raykov, T., West, B. T., and Traynor, A. (2015). Evaluation of coefficient alpha for multiple component measuring instruments in complex sample designs. Struct. Equ. Model. 22, 429–438. doi: 10.1080/10705511.2014.936081
Sackett, P. R., Lievens, F., Berry, C. M., and Landers, R. N. (2007). A cautionary note on the effect of range restriction on predictor intercorrelations. J. Appl. Psychol. 92, 538–544. doi: 10.1037/0021-9010.92.2.538
Sackett, P. R., and Yang, H. (2000). Correction for range restriction: an expanded typology. J. Appl. Psychol. 85, 112–118. doi: 10.1037/0021-9010.85.1.112
Sawilowsky, S. (2009). New effect size rules of thumb. J. Mod. Appl. Stat. Methods 8, 467–474. doi: 10.22237/jmasm/1257035100
Schmidt, F. L., and Hunter, J. E. (2003). “History, development, evolution, and impact of validity generalization and meta-analysis methods, 1975–2001” in Validity Generalization: A Critical Review. ed. K. R. Murphy (USA: Erlbaum), 31–66.
Schmidt, F. L., and Hunter, J. E. (2015). Methods of Meta-Analysis: Correcting Error and Bias in Research Findings. 3rd Edn: London: SAGE Publications, Inc.
Schmidt, F. L., Shaffer, J. A., and Oh, I.-S. (2008). Increased accuracy for range restriction corrections: implications for the role of personality and general mental ability in job and training performance. Pers. Psychol. 61, 827–868. doi: 10.1111/j.1744-6570.2008.00132.x
Schult, J., and Sparfeldt, J. R. (2016). Reliability and validity of PIRLS and TIMSS. Eur. J. Psychol. Assess. 34, 258–269. doi: 10.1027/1015-5759/a000338
Sijtsma, K. (2009). On the use, the misuse, and the very limited usefulness of Cronbach’s alpha. Psychometrika 74, 107–120. doi: 10.1007/s11336-008-9101-0
Somers, R. H. (1962). A new asymmetric measure of association for ordinal variables. Am. Sociol. Rev. 27, 799–811. doi: 10.2307/2090408
Spearman, C. (1904). The proof and measurement of association between two things. Am. J. Psychol. 15, 72–101. doi: 10.2307/1412159
Stouffer, S. A., and Princeton, N. J. (Ed.) (1950). “Measurement and prediction” in Studies in Social Psychology in World War II, vol. IV (Princeton, N.J: Princeton university press).
Tabachnick, B. G., and Fidell, L. S. (2021). Using Multivariate Statistics. 6th Edn. India: Pearson Education.
Thompson, G. H. (1940). Weighting for battery reliability and prediction. Br. J. Math. Stat. Psychol. 30, 357–360. doi: 10.1111/j.2044-8295.1940.tb00968.x
Trizano-Hermosilla, I., and Alvarado, J. M. (2016). Best alternatives to Cronbach's alpha reliability in realistic conditions: congeneric and asymmetrical measurements. Front. Psychol. 7:769. doi: 10.3389/fpsyg.2016.00769
Walk, M. J., and Rupp, A. A. (2010). “Pearson product-moment correlation coefficient” in Encyclopedia of Research Design. ed. N. J. Salkind (London: SAGE Publications, Inc.), 1022–1026.
Wherry, R. J., and Taylor, E. K. (1946). The relation of multiserial eta to other measures of correlation. Psychometrika 11, 155–161. doi: 10.1007/BF02289296
Yang, H. (2010). “Factor loadings” in Encyclopedia of Research Design. ed. N. J. Salkind (London: SAGE Publications, Inc.), 480–483.
Yang, Y., and Green, S. B. (2011). Coefficient alpha: a reliability coefficient for the 21st century? J. Psychoeduc. Assess. 29, 377–392. doi: 10.1177/0734282911406668
Keywords: item-score correlation, error variance, standard errors, deflation-corrected error variance, deflation-corrected standard errors
Citation: Metsämuuronen J (2024) Note on the radical inflation in the estimates of error variance in measurement models. Front. Educ. 9:1248770. doi: 10.3389/feduc.2024.1248770
Received: 27 June 2023; Accepted: 09 May 2024;
Published: 30 October 2024.
Edited by:
Gavin T. L. Brown, University of Auckland, New ZealandReviewed by:
Yi-Fang Wu, Cambium Assessment, Inc., United StatesCopyright © 2024 Metsämuuronen. This is an open-access article distributed under the terms of the Creative Commons Attribution License (CC BY). The use, distribution or reproduction in other forums is permitted, provided the original author(s) and the copyright owner(s) are credited and that the original publication in this journal is cited, in accordance with accepted academic practice. No use, distribution or reproduction is permitted which does not comply with these terms.
*Correspondence: Jari Metsämuuronen, amFyaS5tZXRzYW11dXJvbmVuQGdtYWlsLmNvbQ==
Disclaimer: All claims expressed in this article are solely those of the authors and do not necessarily represent those of their affiliated organizations, or those of the publisher, the editors and the reviewers. Any product that may be evaluated in this article or claim that may be made by its manufacturer is not guaranteed or endorsed by the publisher.
Research integrity at Frontiers
Learn more about the work of our research integrity team to safeguard the quality of each article we publish.