- Department of Learning and Instruction, Institute of Psychology and Education, Ulm University, Ulm, Germany
Learning with desktop virtual reality learning environments (DVR) can be highly visual and present many visual stimuli simultaneously. This can be distracting and require instructional support to help learners in their learning processes. The signaling principle could be a promising approach to support these processes, as signals can guide learners’ attention to the relevant information (Mayer, 2005). The present study investigated the effects of signals in a 360° DVR on learning outcomes and cognitive load. In our between-subjects design, we examined a total of N = 96 participants who were randomly assigned to the signaling or non-signaling group. We hypothesized that the signaling group would achieve higher recall, comprehension, and transfer performance than the non-signaling group. We also expected that the signaling group would experience less extraneous cognitive load and higher germane cognitive load than the non-signaling group. The results show that learners who received signals in a DVR achieved significantly higher recall and comprehension scores than learners who did not receive signals. Transfer performance did not differ between groups. Participants in the signals group also experienced significantly lower extraneous cognitive load than participants in the non-signaling group. However, no differences in germane cognitive load were found between groups. These results suggest that learners in a DVR can be supported by signals in their learning processes while simultaneously helping to reduce unnecessary cognitive load.
Introduction and Theoretical Background
In recent years, virtual reality learning environments (VRLE) have become increasingly popular. They are also increasingly used as a learning medium in educational institutions to enhance learning (Radianti et al., 2020; Wu et al., 2020). VRLE makes it possible for learners to be immersed in a wide variety of scenarios while feeling as if they are actually present in that environment. With VRLE, learning scenarios can be created that would be difficult or impossible to implement in the real world. But what exactly is virtual reality (VR)? VR is defined as a realistic computer-generated environment that can engage multiple human senses (Burdea and Coiffet, 2003), leading to the immersive and sensory illusion of actually being present in the VR environment (Biocca and Delaney, 1995). One of the most important features that distinguishes classical learning environments from VR environments is immersion. On one hand, immersion can be defined as a psychological state of how much learners feel mentally involved in the learning environment (Li et al., 2020). While, this definition is also often used for presence, in this article we will limit ourselves to this definition of immersion. On the other hand, immersion can be defined as objectively measurable by the characteristics of the technology used, such as its degrees of freedom, which relate to the user’s freedom of movement in a three-dimensional space (Slater and Wilbur, 1997). Depending on the VR technology, the extent of immersion may differ (Radianti et al., 2020). According to both definitions, so-called head-mounted displays (HMD) usually lead to a high degree of immersion (Radianti et al., 2020). These are displays worn in front of the eyes, the display of which adapts according to the movement of the head and thus enables a view of the virtual environment of up to 360° (Rolland and Hua, 2005). However, in a learning scenario, the drawbacks of HMD technology include its high acquisition cost and difficult scalability in real learning environments (Richards and Taylor, 2015; Radianti et al., 2020).
A widely used alternative to HMDs is a desktop virtual reality. These are virtual environments that run on inexpensive computer systems and can be operated with a mouse, keyboard or touchscreen (Lee and Wong, 2014). They can even be used in an online classroom setting (Dodd and Antonenko, 2012). While, desktop virtual reality learning environments (DVR) are technically classified as low immersive, they still contain certain immersive aspects to it (Robertson et al., 1997; Radianti et al., 2020). The learner is in control by determining the temporal and visual sequence of the learning environment, which allows the learner to be drawn into the virtual environment (Robertson et al., 1997). There is also a form of navigation and search through the operation of the 360° field of view, as textual coherent learning information, depending on the learner’s point of view, can be discovered in the virtual space. The main advantage over HMDs is the lower acquisition cost, since only an Internet-enabled device with a browser is needed to present the VRLE. This is also associated with greater scalability in difficult learning settings.
In theory, VRLE seem to bring great opportunities for learning. However, the empirical evidence on whether VRLE lead to better learning outcomes is heterogeneous. Some studies indicate that learning in VR improves learning outcomes (Dalgarno and Lee, 2010; Tüysüz, 2010). In contrast, other studies have shown that learners learn less in a VRLE, regardless of the level of immersion (Parong and Mayer, 2018; Makransky et al., 2019b). However, there are also studies that find no differences regarding learning outcomes (Stepan et al., 2017). Recent meta-analyses show that learners using VRLE can show higher learning outcomes than learners in control groups, but further empirical research is needed to investigate the levels of processing in more detail (Radianti et al., 2020; Wu et al., 2020). A common classification for these levels of processing is described in Bloom’s (1956) Taxonomy. The first three levels are recall, comprehension, and transfer. While, recall tasks only aim at reproducing memorized information, comprehension questions test a deeper understanding of the content, e.g., by asking for explanations or relations. The transfer level describes how the acquired knowledge can be transferred to new situations. The majority of the presented studies only examine recall performance for learning outcomes in VR, with some study results suggesting an advantage in retention performance (Pulijala et al., 2018). Other studies find a lower recall performance in VR compared to a less immersive presentation of the learning material (Parong and Mayer, 2018; Makransky et al., 2019b). A few studies also measure transfer performance and also show heterogeneous results for learning in VR (Chittaro et al., 2018; Makransky et al., 2019b). In summary, it is important to consider the learning outcome in a DVR in a differentiated way, otherwise possible effects can be overlooked or overestimated.
Challenges of Learning With DVR
Although we examine a 360° DVR in this study, the challenges presented below are also relevant to VRLE. The learning material was developed to be presented in both DVR and a VRLE. That is why we also draw conclusions from and for VRLE. Characteristic of immersive DVR is the particularly large number of 3D models, which are often displayed with high resolution and a high level of detail. With realistic textures and sounds, a DVR can look convincingly real and thus promote immersion (Jensen and Konradsen, 2018). However, when so much visual information is presented graphically at the same time, learners can easily become overwhelmed in filtering out the relevant learning content (Mayer, 2005; Jensen and Konradsen, 2018). In the Cognitive Theory of Multimedia Learning (CTML) this process is called selection (Mayer, 2005). An impaired selection process can result in either important information being missed or irrelevant information being selected for further processing. Since the selection process is the basis for further processes of learning, all subsequent levels of learning outcomes can be impaired (Moreno and Mayer, 2000). If information is incompletely selected, then only incomplete connections can be made between the information in the working memory. When irrelevant information is selected, not only is it more difficult to make correct connections between relevant stimuli, but unnecessary prior knowledge may be activated, requiring additional working memory capacity (Moreno and Mayer, 2000). In addition, the visual complexity in a DVR can also actively distract the learner from the actual learning subject (Jensen and Konradsen, 2018). In this complex representation, there are often stimuli that are not related to the actual learning content. These so-called seductive details are stimuli that are not relevant for the understanding of the learning material and can distract from what is actually important (Sundararajan and Adesope, 2020). In a DVR, this would mean that anything that is not coherent with the learning objective can be counted as seductive details. de Koning et al. (2009) have shown that in a dynamic and transient learning environment, salient but not learning-relevant information is more likely to be selected and processed, and therefore the capacity of working memory may not be sufficient to cognitively process the learning-relevant information (de Koning et al., 2009). Due to the many visual stimuli and thus possible seductive details, learners in a DVR may experience increased cognitive load by placing additional demands on working memory capacity simply by presenting the information in DVR (Parong and Mayer, 2018; Frederiksen et al., 2019). This unnecessary load for learning is called extraneous cognitive load (ECL) in the Cognitive Load Theory (CLT; Sweller, 2005).
Another challenge in the selection of information in a DVR is that the relevant information is not always placed directly in front of the learner, but only becomes visible when the learner actively looks around in the learning environment. This additional navigation effort must also be invested by the learner and takes up capacity in limited working memory, which can lead to higher ECL (Parong and Mayer, 2018; Frederiksen et al., 2019).
When the actual learning content, distractions and navigation effort must be processed simultaneously in working memory, there may not be enough capacity left for the germane cognitive load (Sweller, 2005). The germane cognitive load results from the effort a learner must invest in the learning task and thus contributes directly to learning outcomes (Sweller, 2005).
The cognitive process of organization involves making connections between selected elements of the learning material. If irrelevant information is selected for learning, further processing of that information would not be useful for learning and could result in irrelevant or incorrect connections being made, leading to impaired comprehension performance. In order to select relevant information for better comprehension performance, it might be necessary for the learner to return to an earlier processing step. Another challenge in the process of organization can occur when auditory information is presented in addition to visual information. In a DVR, the learner must then independently search for the matching visual information to the auditory information and determine whether the information belongs together. If the information does not match, the visual search continues. However, a narrated audio track will continue regardless of whether the learner has already formed enough connections, which can affect comprehension performance. Therefore, it is important to assist the learner by means of instructional design in how to focus attention on what is relevant, be less distracted, and reduce navigation effort in a DVR.
Guiding Attention by Using Signals
To help leaners avoid getting distracted by seductive details and focus their attention on the relevant parts of the learning material, highlighting these sections could be beneficial for learning in DVR. A multimedia design principle that derived from the CTML and particularly addresses this problem is the signaling principle. The signaling principle refers to the idea that learners can process the learning material more deeply when learning-relevant information is highlighted. The learner’s attention is thus directed to the relevant parts of the learning material (Mayer, 2005; Van Gog, 2014). In classical multimedia learning environments, signaling has already proven to be an effective instructional aid to support selection processes (Van Gog, 2014; Alpizar et al., 2020). To understand whether signals can also support deeper learning processes, a differentiated view of learning outcomes is necessary (Xie et al., 2017). In Xie et al.’s (2017) meta-analysis, they distinguished between three types of learning outcomes according to the levels of Bloom mentioned above (Bloom, 1956). They found that signaling not only increased learning outcome for recall and comprehension but also for transfer scores. Schneider et al. (2018) found in their meta-analyses that signaling can improve recall and transfer performance, when compared to non-signaling groups. Another meta-analysis by Richter et al. (2016) also found effects for comprehension and transfer when signaling was used in the multimedia learning environment. This effect was moderated by prior knowledge, making it even more pronounced for learners with low prior knowledge (Richter et al., 2016). Overall, it can be assumed that signals can be used as an effective instructional aid in a DVR, just as in classical multimedia environments. This is because signals directing attention to relevant learning material, making it salient to the learner, so that it can stand out from irrelevant material (Lorch, 1989). This so-called guiding attention hypothesis was supported by Ozcelik et al. (2010) in an eye tracking study in which learners in a signaling group outperformed the non-signaling group in transfer and matching tests.
Directing the learner’s attention with signals in a visually complex DVR can reduce unnecessary search and orientation processes, which can lead to less seductive details being selected, ultimately reducing extraneous cognitive load (Dodd and Antonenko, 2012; Alpizar et al., 2020). The freed-up capacity in the limited working memory, could then be used for germane processes (de Koning et al., 2009). In a DVR, the learning material may have relevant information outside the current field of view. To navigate to the relevant information, the learner must decide what might be relevant to the learning (Neumann, 1996). Especially for learners without prior knowledge, signals could help to reduce this navigation effort (Richter et al., 2016).
In conclusion, signaling in a DVR could help to focus on relevant information, help to not be distracted by seductive details and help to orientate in the learning environment. Therefore, signaling represents a promising approach to support the visually and cognitively demanding learning processes in a DVR, thus enhancing the user’s learning performance and helping them manage the different types of cognitive load.
Recent Studies
While, different types of signals in DVR have been explored, there are still research gaps on how these may affect learning (Horst et al., 2019). Initial research investigating the effects of signals on learning outcomes and cognitive load in a VR context appears promising. Albus et al. (2021) revealed that signaling in the form of textual annotations can improve learning outcomes in a VRLE compared to a group without signaling on recall performance. In addition, they also found that the use of signaling in VR can also increase germane cognitive load (Albus et al., 2021). Another study that looked at signals in a VRLE showed an interaction effect on learning outcome with motivation as a moderator. They found that less motivated learners supported by signals achieved a similar learning outcome as intrinsically motivated learners (Vogt et al., 2021a). Chin et al. (2015) found similar results in VR lab safety training. They showed that the signaling group achieved better learning outcomes than the group without signals when prior knowledge was taken into account as a covariate (Chin et al., 2015).
Research Question and Hypothesis
In this study, we will examine the effects of signals in a 360° DVR on the topic of the German forest and its animals on learning outcomes and cognitive load. In order to examine learning outcome and the underlying levels of processing in a DVR more precisely, the learning outcome were differentiated into recall, comprehension and transfer according to Bloom’s (1956) Taxonomy. We also measured cognitive load differentiated into extraneous cognitive load and germane cognitive load (Sweller, 2005). In addition, variables relevant to the learning process are considered in the data analysis, including motivation (Goodman et al., 2011) and prior knowledge of the subjects (Seufert, 2003) to shed more light on their influence of signaling when learning with DVR.
We hypothesize, that the use of signals in a DVR can lead to better recall (H1), comprehension (H2), and transfer (H3) outcomes, than learning without signals. Concerning cognitive load, we hypothesize that extraneous cognitive load should be significantly lower in the signals condition than in the non-signaling condition (H4). We also hypothesize that germane cognitive load should be significantly higher in the signal condition than in the non-signaling condition (H5).
Materials and Methods
A priori Power Analysis
For the estimation of the required sample size for this study, an a priori power analysis was performed. Mayer’s (2017) review concerning the effect size of signaling on learning outcomes was considered as reference of the effect size. Applying α = 0.05, a power level of (1–β) = 0.95 and reference effect size of d = 0.46, the required sample size for our study was estimated to be approximately N = 64 [G*Power 3.1; Faul et al. (2009)]. In total, we collected more participants than we initially calculated. We decided to collect additional subjects in order to counteract the expected drop out of an online study. We could not identify which subjects had to be excluded due to technical problems or early termination of the study until the end of the data collection.
Participants and Study Design
A total of 113 participants completed the study, of which 17 were excluded from the study due to technical problems with the learning environment. The analyses presented therefore refer to a total sample of N = 96 subjects (72.9% female). The age of the participants varied from 18 to 64 years (M = 28.29; SD = 12.48). The study was conducted as an online survey with the DVR built in. Participation was 95.8% via desktop devices and 4.2% via tablets or smartphones. In our one-factorial between-subjects design with two groups participants were randomly assigned to either the signaling group (n = 48) or the non-signaling group (n = 48). Informed consent was obtained from participants prior to participation in the study. The study was conducted as a browser-based online study. Within the survey, the subjects were redirected to an external website where the integrated 360° DVR started automatically. As dependent variables, we measured learning outcomes (recall, comprehension, and transfer) and cognitive load (ECL and GCL). As potential covariates we assessed prior knowledge, motivation, previous contact with VR and immersion.
Materials and Instruments
The learning material in the 360° DVR was about native animals in German forests. While, learners could click and drag their way around the visually rich environment, additional auditive information about the animals was played. In total, there were 14 interactive segments that were presented to the learners in a linear progression. In the first segment, the use and navigation of the learning environment was explained to the learners. At this point, they were instructed to work through the learning environment conscientiously and to listen to each audio track once. They were able to move on to the next learning content in a self-paced manner, but could not return to the previous content. Also, the audio track could be activated in each segment by clicking on the corresponding icon. The audio tracks were on average about 60 s long and could not be paused once activated. Only the signaling group contained static signals in the form of light green circles around learning relevant information (e.g., the feet of the deer are highlighted when it is explained that they belong to the cloven-hoofed genus; see Figure 1). Both groups spent the same amount of time in the DVR, approximately 14 min. The questionnaires and constructs measured in the present study can be found below. In addition, basic demographic data such as age, gender, student status and previous contact with VR of participants were collected.
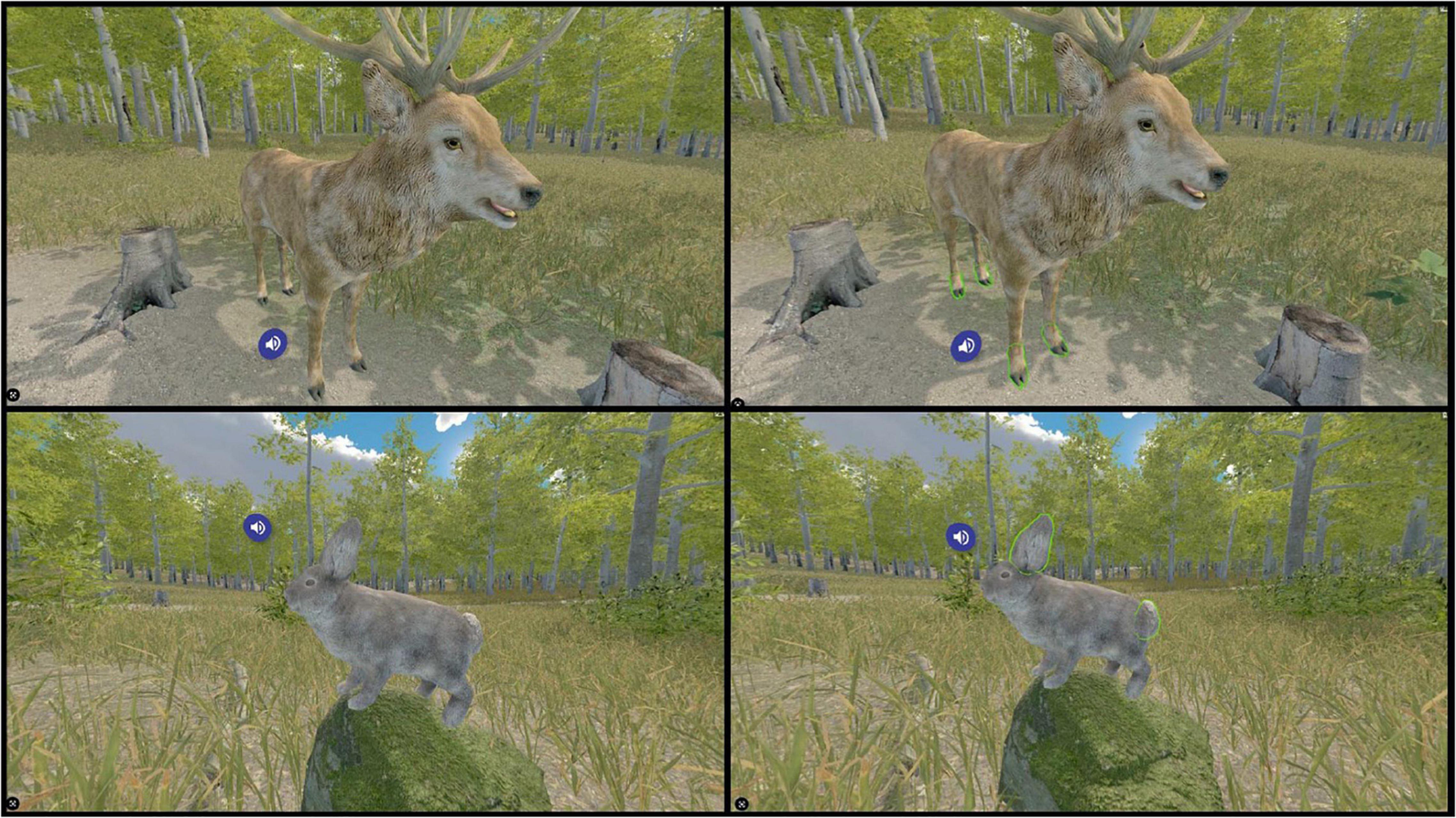
Figure 1. Desktop virtual learning environment with signals and without signals in comparison. The learning environment was displayed in full screen and the participants could turn 360° in any direction.
Prior Knowledge
At the beginning of the study, the participants’ prior knowledge was determined with a total of 15 questions. Domain-specific knowledge about the German forest and its native animals was assessed. These questions were created with experts on the topic to ensure content validity. The test consisted of five open questions (e.g., “Name five animal species that live in our forests.”) and ten closed multiple-choice questions that had between three and five possible answers. The maximum score achievable was 18 points, and participants could earn half points for partially correct answers. No points were deducted for incorrect answers. Two independent raters scored the answers using a coding scheme and achieved very high inter rater reliability (ICC = 0.98, 95% CI [0.95–0,98] p < 0.001).
Motivation
The Questionnaire on Current Motivation (QCM; Rheinberg et al., 2001) was used to measure motivation. The 18 items of the questionnaire (e.g., “I would also work on such a task in my spare time.”) are rated on a 7-point Likert scale (1 “strongly disagree” to 7 “strongly agree”). For further analyses, a mean was calculated across all items. Internal consistency was Cronbach’s α = 0.72 (95% CI [0.63–0.79]) indicating acceptable reliability (Cohen, 1988).
Cognitive Load
Cognitive load was assessed differentiated by ECL and GCL using the Cognitive Load Questionnaire (Klepsch et al., 2017). Two items each for ECL (e.g., “During this task, it was difficult to recognize and link the crucial information.”) and GCL (e.g., “For this task, I had to think intensively about what things meant.”) were rated using a 7-point Likert scale (1 “absolutely not true” to 7 “completely true”). Internal consistency for ECL was Cronbach’s α = 0.65, 95% CI [0.48–0.77] and for GCL α = 0.70, 95% CI [0.58–0.79].
Learning Outcomes
To measure the participants’ learning outcome, a knowledge test was conducted that was related to the content of the 360° learning unit. The posttest consisted of 13 open-ended questions that were differentiated into recall, comprehension, and transfer according to Bloom’s taxonomy (Bloom, 1956). With five recall questions (e.g., “Name the two most important body parts of the fox, which are used to detect prey and enemies.”), four comprehension questions (e.g., “Explain why there is only one male deer in a rutting pack.”), and four transfer questions (e.g., “Briefly justify whether these molars belong to a younger or older deer.”), a maximum total score of 16.5 could be achieved. The maximum score for recall was six points, five points for comprehension, and 5.5 points for transfer. Partial points were awarded for partially correct answers and no points were deducted. Interrater reliability between two independent raters was very high for all learning outcomes (recall: ICC = 0.86, 95% CI [0.79–0.91], p < 0.001; comprehension: ICC = 0.90, 95% CI [0.85–0.93], p < 0.001; transfer: ICC = 0.97, 95% CI [0.96–0.98], p < 0.001).
Immersion
Immersion was assessed using a subscale of the Technology Usage Inventory (TUI; Kothgassner et al., 2013). A total of four items (e.g., “During the virtual simulation, I completely forgot about the world around me.”) were rated on a 7-point Likert scale (1 “I disagree” to 7 “I agree”).
Results
All data analysis was performed with IBM SPSS (Version 28) with an α-error = 0.05 for all calculations. The interpretation of partial η2 as a measure of effect size, was appropriately categorized according to Cohen (1988) as η2 = 0.01 (small), η2 = 0.06 (medium), and η2 = 0.14 (large).
Descriptive Analysis
Descriptive analysis revealed no significant differences between the signaling and non-signaling groups in terms of gender [χ2(1, N = 96) = 0.21, p = 0.646], age [t(94) = 1.86, p = 0.067], motivation [t(94) = 0.65, p = 0.519], prior exposure contact to VR [t(94) = 0.20, p = 0.846], immersion [t(94) = 0.84, p = 0.403], and time-on-task [t(86) = −1.10, p = 0.273]. However, there was a significant difference in prior knowledge between the signaling and non-signaling groups [t(94) = 2.34, p = 0.022]. Since prior knowledge was also significantly correlated with recall (r = 0.29, p = 0.004) and comprehension (r = 0.45, p > 0.001), it was included as a covariate in subsequent analyses.
To identify other potential control variables, we tested whether motivation, prior contact to VR, immersion, or time spent in the learning unit had an influence on learning outcomes or cognitive load. There was a significant correlation between motivation and GCL (r = 0.45, p < 0.001). Motivation was therefore included as a covariate in the analyses of GCL. Beyond that, there were no other correlations between control variables and dependent variables. Descriptive statistics of demographic and other relevant variables, separately for the signaling and non-signaling conditions, are presented in Table 1.
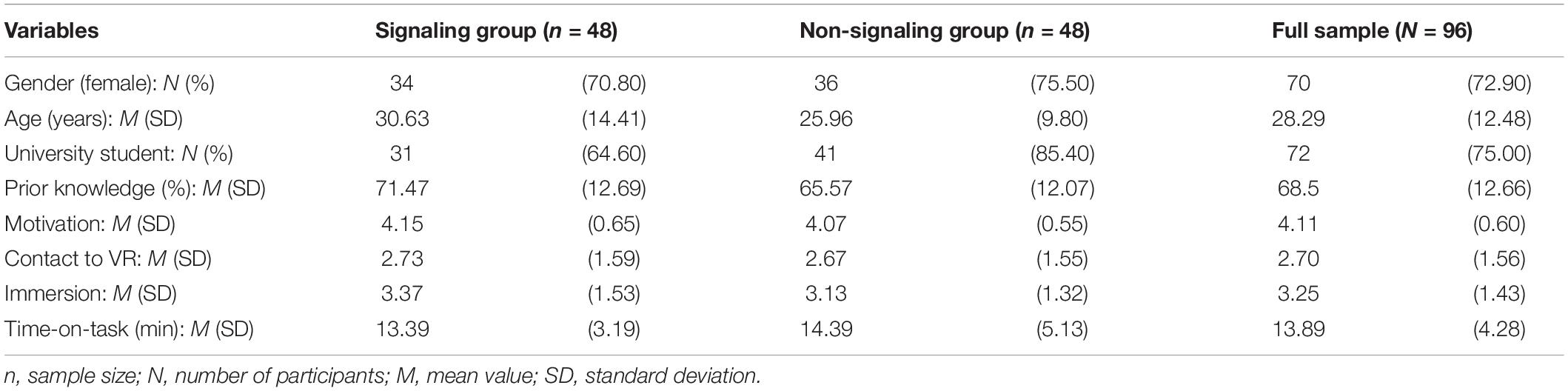
Table 1. Descriptive data of relevant variables, separately for the signaling and non-signaling conditions.
The descriptive statistics of the results for learning outcomes and cognitive load can be found in Table 2.
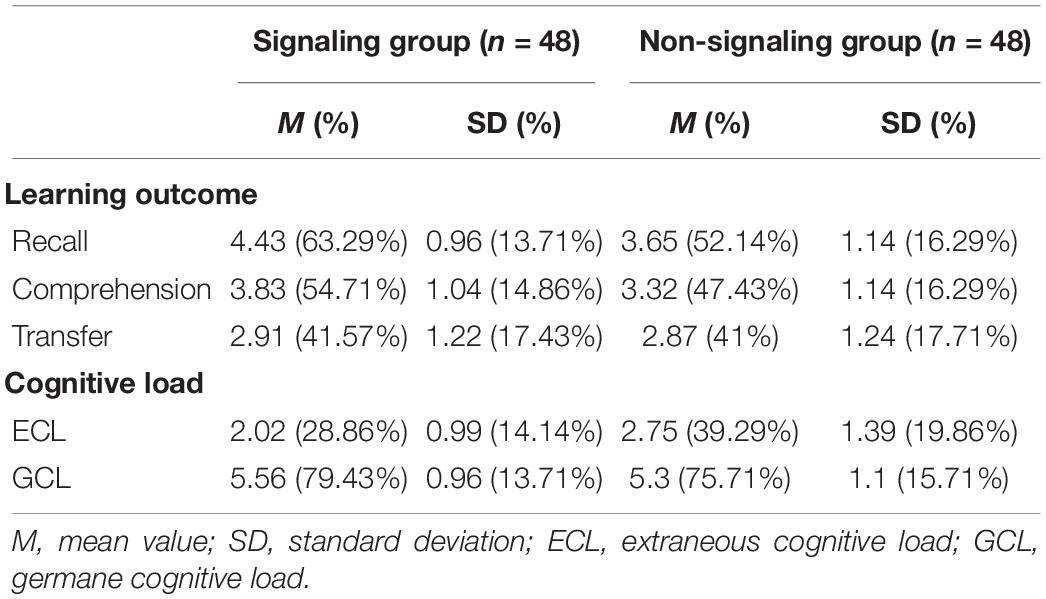
Table 2. Means, standard deviations, and ANOVA/ANCOVA results, separately for the signaling and non-signaling condition.
Learning Outcomes
The ANCOVAs with prior knowledge as covariate for all three learning outcomes revealed significant differences between the signaling and non-signaling groups for recall [H1: F(1,93) = 9.58, p = 0.002, η2 = 0.093] with a medium to high effect size. Participants in the signaling group achieved higher recall scores than participants in the non-signaling group.
In addition, there was a significant between-group difference for comprehension [H2: F(1,93) = 3.06, p = 0.042, η2 = 0.032] with a medium effect size. Participants in the signaling group scored again higher on comprehension than participants in the non-signaling group.
Regarding transfer, the data showed no significant difference between groups [H3: F(1,93) = 0.58, p = 0.405, η2 = 0.001]. These results are visualized in Figure 2.
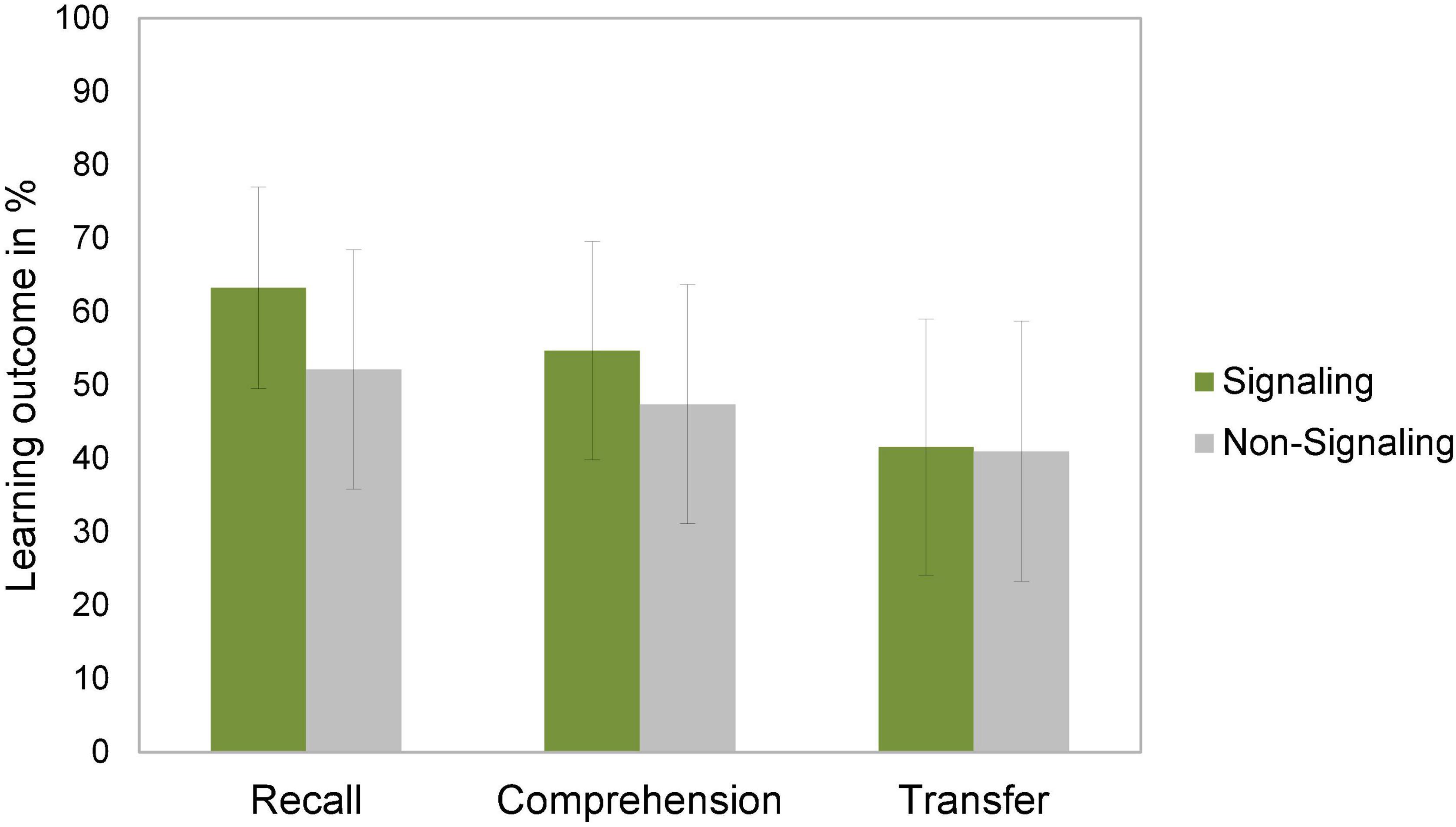
Figure 2. Mean values (in percent) for the learning outcomes recall, comprehension and transfer, separately for the signaling and non-signaling group. The vertical error bars indicate the standard error of the mean.
Cognitive Load
Results of the ANCOVA with prior knowledge as a covariate showed a significant difference in ECL between the signaling and non-signaling group [H4: F(1,93) = 5.97, p = 0.008, η2 = 0.060] with a medium effect size. Participants in the signaling group experienced less ECL than those in the non-signaling group. However, contrary to our hypothesis, there was no significant difference between groups on GCL when controlling for motivation and prior knowledge [H5: F(1,92) = 0.62, p = 0.217, η2 = 0.007]. The graphical representation of these results can be seen in Figure 3.
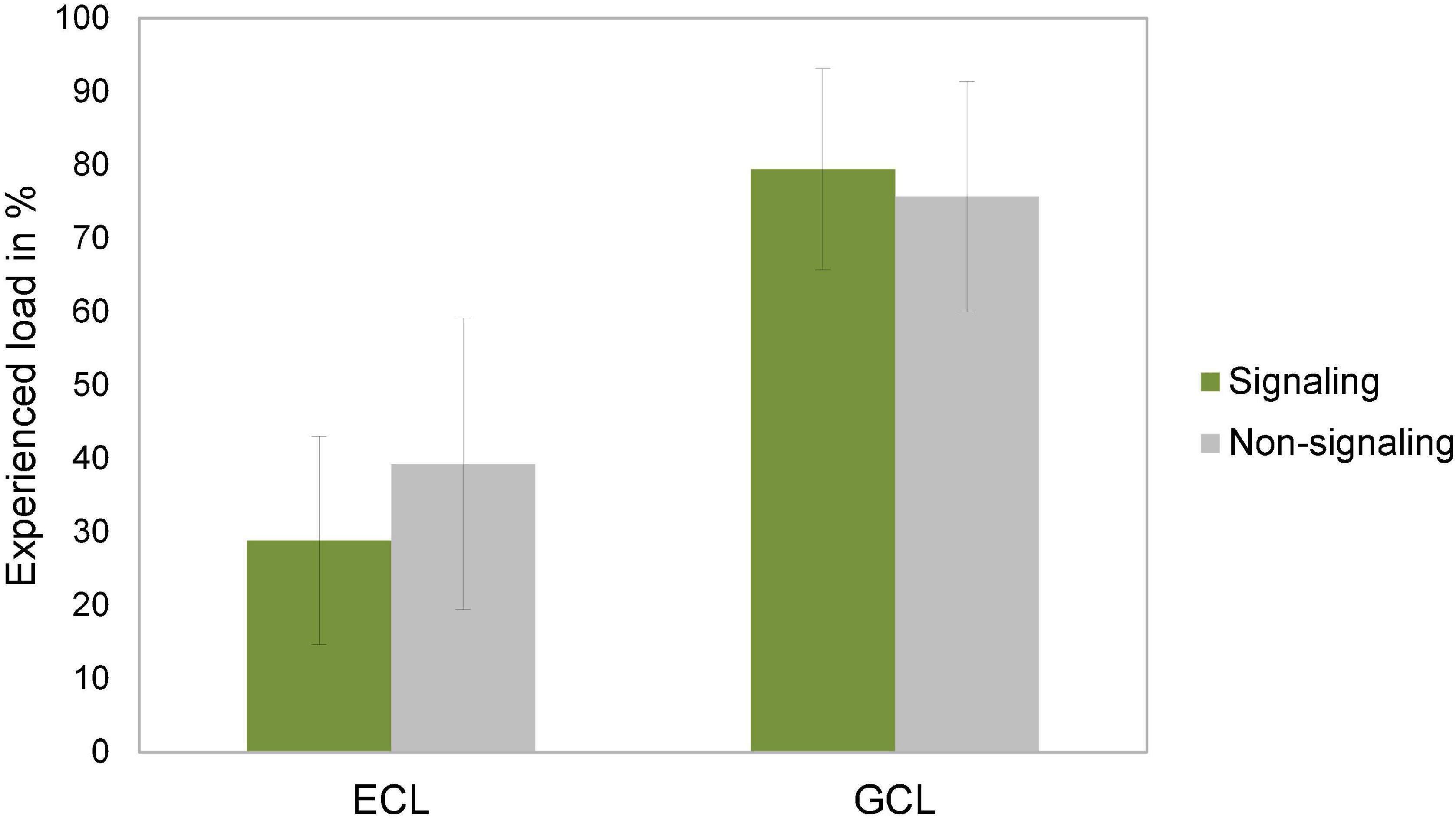
Figure 3. Mean values (in percent) for ECL, and GCL, separately for the signaling and non-signaling group. The vertical error bars indicate the standard error of the mean; ECL, extraneous cognitive load; GCL, germane cognitive load.
Discussion
The aim of this study was to investigate the effects of signaling in a 360° DVR on learning outcome differentiated by recall, comprehension, and transfer, as well as on cognitive load differentiated by ECL and GCL.
Effects of Signaling on Learning Outcomes
In line with our hypotheses, learning with signaling led to significantly higher learning outcomes for both recall (H1) and comprehension (H2) than learning without signaling in a DVR. However, we found no differences between the groups in terms of their transfer performance (H3). These results indicate that signals in a DVR can be used to support learners in their selection and organization processes.
We infer that learners were supported by signals in a DVR in their selection process by directing their attention to the relevant information (Ozcelik et al., 2010; Dodd and Antonenko, 2012). Other studies on signals in classical multimedia settings have also shown that the selection process can be supported, which is reflected in a higher recall performance of the highlighted information (Lorch, 1989; Mautone and Mayer, 2001). We were thus able to show that the effects of signals in classical learning environments can be transferred to DVR, since the underlying learning processes involved remain identical. Our results are also partially supported by the findings of previous research in a VRLE. Albus et al. (2021) who examined signals in a VRLE in the form of textual annotations also found higher recall scores for the signaling group compared to the non-signaling group, but no effects for comprehension or transfer. This is an indication that textual annotations can support the selection processes, but not the deeper organization or integration processes (Albus et al., 2021). However, in this study we were able to show that signals in a DVR could promote comprehension performance. This could be an indicator that the signals also supported the learners in their organizational processes. The signals might have enabled the learners to process the relevant visual and auditory information simultaneously in working memory. This allows logical mental connections to be made between the information, which has translated into higher comprehension performance. When the auditory information is processed, the learner searches for the corresponding matching visual information in the DVR. The signals make it clear that it belongs together with the auditory information. They help learners identify matching information in working memory as being related and encourage them to want to comprehend how this information fits together. Auditory information is also transient and learners need to associate the information together in working memory in time for understanding processes to happen. The signals help reduce the visual search time for the relevant information within the DVR (Xie et al., 2017). Signals help learners to be less distracted by seductive details, allowing more time to be spent on comprehension processes.
However, we did not measure visual search time in this study, but it could be measured by eye movements in future studies. Furthermore, it is not only relevant to link auditory and visual information, but also several visual information among each other. Although the signals were only simple outlines of the objects, they seem to be able to help highlight the connections between the individual visual information that learners would have to conclude themselves without signals (Lorch, 1989; Mautone and Mayer, 2001).
Contrary to our hypothesis (H3), we did not find a significant effect of the signals in a DVR on the transfer performance. A possible explanation for this could be that our signals were solely visual in the form of green outlines. While, these could draw the learner’s attention to the relevant information and even possibly reduce visual search time, they did not help to stimulate learners to deeper processing at the transfer level. Here, for example, prompts could be helpful in the future to get the learner to invest in deeper processing of the information (Vogt et al., 2021b). Signals also do not seem to have had an effect on immersion. The signals used here are visually coherently integrated into the DVR and therefore should not interfere with the learners’ experience of immersion. However, it would be interesting to find out which features of the signals, such as size relative to the image or animations could influence the feeling of immersion.
Effects of Signaling on Cognitive Load
Our hypotheses on cognitive load could only be partially confirmed. We could show that signals in a DVR can significantly reduce ECL compared to when learners are not supported by signals (H4). DVR are often visually complex and can contain many seductive details. While, on the one hand these are important for immersion, on the other hand they can also be distracting and occupy cognitive resources (Van Merrienboer and Sweller, 2005). Our results suggest that these non-relevant visual stimuli in a DVR can be perceived as distracting to learners and occupy working memory capacity important for learning (Parong and Mayer, 2020). Signals, on the other hand, could make the essential elements salient to learners through their attention-guiding function, which reduces unnecessary visual search time (Mayer and Fiorella, 2014). Thus, fewer seductive details are also processed cognitively, which can reduce the associated ECL (Sweller, 2005; Noetel et al., 2021). If less seductive details are cognitively processed, the selection process can also be supported (Parong and Mayer, 2020).
If the ECL can be reduced by signals, there should be more cognitive capacity left in working memory for germane processes (Sweller, 2005). Our hypothesis about GCL could not be supported by the results we found (H5). Learners in the signaling group reported no significant difference in terms of their invested GCL compared to the non-signaling group. One possible explanation for why the signals in the DVR did not encourage learners to invest more GCL is that we measured high GCL values in both groups. Learners thus already invested high levels of GCL regardless of the signals. This may be due to our DVR, because learners may have been motivated by the interesting topic or the visually appealing learning environment to invest enough cognitive capacity for it. For many learners, DVR are still a new technological experience. This may be a factor that can motivate learners to learn in a DVR (Huang et al., 2021). In studies that compared classical learning environments with VRLE, it was found that learners showed higher motivation and interest when learning in with VRLE (Makransky et al., 2019a; Parong and Mayer, 2020). Since we also identified motivation as a covariate for GCL, this could be an indicator of why a possible effect of signals on GCL does not become sufficiently pronounced.
Strengths and Limitations
The present study used a controlled randomized trial design investigated the effects of signaling in a DVR, differentially on learning outcomes and cognitive load. The DVR used in this study was chosen to reflect realistic educational settings. This is primarily due to its accessibility and low-cost implementation, as learners only need an Internet-enabled device to immerse themselves in the DVR. Another strength of this study is the differentiated perspective on learning outcomes on different levels of processing as well as on cognitive load. In doing so, we also meet the demands of recent meta-analyses and articles on learning in VRLE that criticized the lack of theory-driven research and application development in VRLE (Radianti et al., 2020; Makransky and Petersen, 2021). However, there are also limitations that need to be considered. Despite proper randomization to both groups, there was a significant difference in prior knowledge. To avoid a possible bias in the results, we statistically controlled for prior knowledge in the analysis of the hypothesis. There were also limitations within the DVR. Learners could look around independently and start the audio track to the image in a self-directed manner. However, we do not have objective data on whether subjects listened to each audio track in its entirety. It could also be that learners, against instruction, listened to the audio track multiple times. Nevertheless, we measured how much time learners spent in total in the DVR. Since both groups spent on average the same amount of time in the DVR, any possible influence on the results should be negligible. Furthermore, learners were also able to self-navigate to the next content, but not jump back to the previous one. It could be possible that participants jumped to the next topic by mistake or too fast, which could possibly influence the post-test results. However, participants were able to indicate in the post-test if they experienced any problems while completing the learning environment. That said, participants who experienced problems were excluded from the analysis, as explained in the methods section.
Since the participants could look around 360° in the learning unit at any time, it is possible that the relevant learning content that the audio track is currently talking about is not in the field of view of the learners at this time. This might have affected the integration of text and pictures, leading to poorer learning outcome performance (Seufert, 2003). To further empirically investigate the attention-guiding function of signals, future eye tracking studies in DVR would be highly desirable and informative.
Conclusion
Our study was able to show that signaling can improve learners’ recall and comprehension performance in a DVR. At the same time, signaling can also reduce unnecessary load in working memory. Our results also demonstrated that established theories from multimedia research, such as the CTML or the CLT can be well-suited as a foundation for studies in a DVR (Mayer, 2005; Sweller, 2005). Although DVR can often be more visually complex and also involve a higher degree of immersion, it is these features that can be perceived as distracting. Nevertheless, the learning processes are the same in both classical and immersive learning environments and can benefit from instructional aids (Parisi, 2015).
It is also interesting to consider that even quite simple visual signals can have positive effects on learning outcomes and the cognitive load. However, such simple signals do not seem to be sufficient to stimulate deeper levels of processing. Here, future studies would be interesting to investigate signals specifically aimed at supporting deeper levels of processing or how signals work in combination with prompts (Vogt et al., 2021b). While, different types of signals have been studied in DVR, their effectiveness in learning environments has not yet been investigated (Horst et al., 2019). It would also be desirable to investigate different types of signals (e.g., auditory signals, adaptive or dynamic signals) to find out how they can affect different levels of processes in learning. In addition, it is also important to investigate further instructional aids in DVR. A possible starting point could be further multimedia design principles originating from the CTML.
Data Availability Statement
The raw data supporting the conclusions of this article will be made available by the authors, without undue reservation.
Ethics Statement
Ethical review and approval was not required for the study on human participants in accordance with the local legislation and institutional requirements. The patients/participants provided their written informed consent to participate in this study.
Author Contributions
PA contributed to the conception and design of the study, developed the used questionnaires, led data collection, performed the statistical analysis, and interpreted the data. PA wrote the first draft of the manuscript with support from TS. TS supervised this manuscript. Both authors contributed to the final version of the manuscript.
Funding
This study was funded by the Federal Ministry of Education and Research Germany (grant number: 16OH22032).
Conflict of Interest
The authors declare that the research was conducted in the absence of any commercial or financial relationships that could be construed as a potential conflict of interest.
Publisher’s Note
All claims expressed in this article are solely those of the authors and do not necessarily represent those of their affiliated organizations, or those of the publisher, the editors and the reviewers. Any product that may be evaluated in this article, or claim that may be made by its manufacturer, is not guaranteed or endorsed by the publisher.
References
Albus, P., Vogt, A., and Seufert, T. (2021). Signaling in virtual reality influences learning outcome and cognitive load. Comput. Educ. 166:104154. doi: 10.1016/j.compedu.2021.104154
Alpizar, D., Adesope, O. O., and Wong, R. M. (2020). A meta-analysis of signaling principle in multimedia learning environments. Educ. Technol. Res Dev. 68, 2095–2119. doi: 10.1007/s11423-020-09748-7
Biocca, F., and Delaney, B. (1995). “Immersive virtual reality technology,” in Communication in the Age of Virtual Reality, eds F. Biocca and M. R. Levy (Mahwah, NJ: Lawrence Erlbaum Associates), 57–125.
Bloom, B. S. (1956). Taxonomy of Educational Objectives, Handbook I: The Cognitive Domain. New York, NY: David McKay.
Burdea, G. C., and Coiffet, P. (2003). Virtual Reality Technology, 2nd Edn. Hoboken, NJ: John Wiley & Sons, Inc.
Chin, W., Yahaya, W., and Muniandy, B. (2015). “Virtual Science Laboratory (ViSLab): a pilot study on signaling principles towards science laboratory safety training,” in Proceedings of the 3rd International Conference on Language, Literature, Culture and Education 2015, Kuala Lumpur. doi: 10.13140/RG.2.1.4311.3041
Chittaro, L., Corbett, C. L., McLean, G. A., and Zangrando, N. (2018). Safety knowledge transfer through mobile virtual reality: a study of aviation life preserver donning. Saf. Sci. 102, 159–168. doi: 10.1016/j.ssci.2017.10.012
Cohen, J. (1988). Statistical Power Analysis for the Behavioral Sciences, 2nd Edn. Hillsdale, NJ: Lawrence Erlbaum Associates.
Dalgarno, B., and Lee, M. J. (2010). What are the learning affordances of 3-D virtual environments? Br. J. Educ. Technol. 41, 10–32. doi: 10.1111/j.1467-8535.2009.01038.x
de Koning, B. B., Tabbers, H. K., Rikers, R. M., and Paas, F. (2009). Towards a framework for attention cueing in instructional animations: guidelines for research and design. Educ. Psychol. Rev. 21, 113–140. doi: 10.1007/s10648-009-9098-7
Dodd, B. J., and Antonenko, P. D. (2012). Use of signaling to integrate desktop virtual reality and online learning management systems. Comput. Educ. 59, 1099–1108. doi: 10.1016/j.compedu.2012.05.016
Faul, F., Erdfelder, E., Buchner, A., and Lang, A.-G. (2009). Statistical power analyses using G*Power 3.1: tests for correlation and regression analyses. Behav. Res. Methods 41, 1149–1160. doi: 10.3758/BRM.41.4.1149
Frederiksen, J. G., Sørensen, S. M., Konge, L., Svendsen, M. B., Nobel-Jørgensen, M., Bjerrum, F., et al. (2019). Cognitive load and performance in immersive virtual reality versus conventional virtual reality simulation training of laparoscopic surgery: a randomized trial. Surg. Endosc. 34, 1244–1252. doi: 10.1007/s00464-019-06887-8
Goodman, S., Jaffer, T., Keresztesi, M., Mamdani, F., Mokgatle, D., Musariri, M., et al. (2011). An investigation of the relationship between students’ motivation and academic performance as mediated by effort. S. Afr J. Psychol. 41, 373–385. doi: 10.1348/000709907X189118
Horst, R., Diez, S., and Dörner, R. (2019). “Highlighting techniques for 360 Video Virtual Reality and their immersive authoring,” in Advances in Visual Computing. 14th International Symposium on Visual Computing, eds G. Bebis, R. Boyle, B. Parvin, D. Koracin, D. Ushizima, S. Chai, et al. (Heidelberg: Springer), 515–526. doi: 10.1007/978-3-030-33720-9_40
Huang, W., Roscoe, R. D. Johnson-Glenberg, M. C., and Craig, S. D. (2021). Motivation, engagement, and performance across multiple virtual reality sessions and levels of immersion. J. Comput. Assist. Learn. 37, 745–758. doi: 10.1111/jcal.12520
Jensen, L., and Konradsen, F. (2018). A review of the use of virtual reality head-mounted displays in education and training. Educ. Inf. Technol. 23, 1515–1529. doi: 10.1007/s10639-017-9676-0
Klepsch, M., Schmitz, F., and Seufert, T. (2017). Development and validation of two instruments measuring intrinsic, extraneous, and germane cognitive load. Front. Psychol. 8:1997. doi: 10.3389/fpsyg.2017.01997
Kothgassner, O. D., Felnhofer, A., Hauk, N., Kastenhofer, E., Gomm, J., and Kryspin-Exner, I. (2013). Technology Usage Inventory (TUI). Vienna: ICARUS Research Team.
Lee, E. A.-L., and Wong, K. W. (2014). Learning with desktop virtual reality: low spatial ability learners are more positively affected. Comput. Educ. 79, 49–58. doi: 10.1016/j.compedu.2014.07.010
Li, P., Legault, J., Klippel, A., and Zhao, J. (2020). Virtual reality for student learning: understanding individual differences. Hum. Behav. Brain 1, 28–36. doi: 10.37716/HBAB.2020010105
Lorch, R. F. (1989). Text-signaling devices and their effects on reading and memory processes. Educ. Psychol. Rev. 1, 209–234. doi: 10.1007/BF01320135
Makransky, G., Terkildsen, T. S., and Mayer, R. E. (2019b). Adding immersive virtual reality to a science lab simulation causes more presence but less learning. Learn. Instr. 60, 225–236. doi: 10.1016/j.learninstruc.2017.12.007
Makransky, G., Borre-Gude, S., and Mayer, R. E. (2019a). Motivational and cognitive benefits of training in immersive virtual reality based on multiple assessments. J. Comput. Assist. Learn. 35, 691–707. doi: 10.1111/jcal.12375
Makransky, G., and Petersen, G. B. (2021). The Cognitive Affective Model of Immersive Learning (CAMIL): a theoretical research-based model of learning in immersive Virtual Reality. Educ. Psychol. Rev. 33, 937–958. doi: 10.1007/s10648-020-09586-2
Mautone, P. D., and Mayer, R. E. (2001). Signaling as a cognitive guide in multimedia learning. J. Educ. Psychol. 93, 377–389. doi: 10.1037/0022-0663.93.2.377
Mayer, R. E. (2005). The Cambridge Handbook of Multimedia Learning. Cambridge: Cambridge University Press.
Mayer, R. E. (2017). Using multimedia for e-learning. J. Comput. Assist. Learn. 33, 403–423. doi: 10.1111/jcal.12197
Mayer, R. E., and Fiorella, L. (2014). “Principles for reducing extraneous processing in multimedia learning: Coherence, signaling, redundancy, spatial contiguity, and temporal contiguity principles,” in The Cambridge Handbook of Multimedia Learning, 2nd Edn, ed. R. E. Mayer (Cambridge: Cambridge University Press), 279–315.
Moreno, R., and Mayer, R. E. (2000). A learner-centered approach to multimedia explanations: deriving instructional design principles from cognitive theory. Interactive Multimedia Electronic. J. Comput. Enhanc. Learn. 2, 12–20.
Neumann, O. (1996). “Theories of attention,” in Handbook of Perception and Action, Vol. 3, eds O. Neumann and A. F. Sanders (Amsterdam: Elsevier), 389–446. doi: 10.1016/S1874-5822(96)80027-2
Noetel, M., Griffith, S., Delaney, O., Harris, N. R., Sanders, T., Parker, P., et al. (2021). Multimedia design for learning: an overview of reviews with meta-meta-analysis. Rev Educ. Res. 92, 413–454. doi: 10.3102/00346543211052329
Ozcelik, E., Arslan-Ari, I., and Cagiltay, K. (2010). Why does signaling enhance multimedia learning? Evidence from eye movements. Comput. Hum. Behav. 26, 110–117. doi: 10.1016/j.chb.2009.09.001
Parisi, T. (2015). Learning Virtual Reality: Developing Immersive Experiences and Applications for Desktop, Web, and Mobile. Sebastopol, CA: O’Reilly Media, Inc.
Parong, J., and Mayer, R. E. (2018). Learning science in immersive virtual reality. J. Educ. Psychol. 110, 785–797. doi: 10.1037/edu0000241
Parong, J., and Mayer, R. E. (2020). Cognitive and affective processes for learning science in immersive virtual reality. J. Comput. Assist. Learn. 37, 226–241. doi: 10.1111/jcal.12482
Pulijala, Y., Ma, M., Pears, M., Peebles, D., and Ayoub, A. (2018). Effectiveness of immersive virtual reality in surgical training: a randomized control trial. J. Oral Maxillofac. Surg. 76, 1065–1072. doi: 10.1016/j.joms.2017.10.002
Radianti, J., Majchrzak, T. A., Fromm, J., and Wohlgenannt, I. (2020). A systematic review of immersive virtual reality applications for higher education: design elements, lessons learned, and research agenda. Comput. Educ. 147:103778. doi: 10.1016/j.compedu.2019.103778
Rheinberg, F., Vollmeyer, R., and Burns, B. D. (2001). FAM: ein Fragebogen zur Erfassung aktueller Motivation in Lern- und Leistungssituationen. Diagnostica. 47, 57–66. doi: 10.1026//0012-1924.47.2.57
Richards, D., and Taylor, M. (2015). A comparison of learning gains when using a 2D simulation tool versus a 3D virtual world: an experiment to find the right representation involving the Marginal Value Theorem. Comput. Educ. 86, 157–171. doi: 10.1016/j.compedu.2015.03.009
Richter, J., Scheiter, K., and Eitel, A. (2016). Signaling text-picture relations in multimedia learning: a comprehensive meta-analysis. Educ. Res. Rev. 17, 19–36. doi: 10.1016/j.edurev.2015.12.003
Robertson, G., Czerwinski, M., and van Dantzich, M. (1997). “Immersion in desktop virtual reality,” in Proceedings of the 10th annual ACM symposium on User Interface Software and Technology, (New York, NY: Association for Computing Machinery), 11–19. doi: 10.1145/263407.263409
Rolland, J. P., and Hua, H. (2005). “Head-mounted display systems,” in Encyclopedia of Optical Engineering, eds R. B. Johnson and R. G. Driggers (New York: Taylor and Francis), 1–13.
Schneider, S., Beege, M., Nebel, S., and Rey, G. D. (2018). A meta-analysis of how signaling affects learning with media. Educ. Res. Rev. 23, 1–24. doi: 10.1016/j.edurev.2017.11.001
Seufert, T. (2003). Supporting coherence formation in learning from multiple representations. Learn. Instr. 13, 227–237. doi: 10.1016/S0959-4752(02)00022-1
Slater, M., and Wilbur, S. (1997). A framework for immersive virtual environments (FIVE): speculations on the role of presence in virtual environments. Presence Teleoperators Virtual Environ. 6, 603–616. doi: 10.1162/pres.1997.6.6.603
Stepan, K., Zeiger, J., Hanchuk, S., Del Signore, A., Shrivastava, R., Govindaraj, S., et al. (2017). Immersive virtual reality as a teaching tool for neuroanatomy. Int. Forum Allergy Rhinol. 7, 1006–1013. doi: 10.1002/alr.21986
Sundararajan, N., and Adesope, O. (2020). Keep it coherent: a meta-analysis of the seductive details effect. Educ. Psychol. Rev. 32, 707–734. doi: 10.1007/s10648-020-09522-4
Sweller, J. (2005). “Implications of Cognitive Load Theory for Multimedia Learning,” in The Cambridge Handbook of Multimedia Learning, ed. R. Mayer (Cambridge: Cambridge University Press), 19–30. doi: 10.1017/CBO9780511816819.003
Tüysüz, C. (2010). The effect of the virtual laboratory on students’ achievement and attitude in chemistry. Int. Online J. Educ. Sci. 2, 37–53.
Van Gog, T. (2014). “The signaling (or cueing) principle in multimedia learning,” in The Cambridge Handbook of Multimedia Learning, 2nd Edn, ed. R. E. Mayer (Cambridge: Cambridge University Press), 263–278.
Van Merrienboer, J. J., and Sweller, J. (2005). Cognitive Load Theory and complex learning: recent developments and future directions. Educ. Psychol. Rev. 17, 147–177. doi: 10.1007/s10648-005-3951-0
Vogt, A., Albus, P., and Seufert, T. (2021a). Learning in virtual reality: bridging the motivation gap by adding annotations. Front. Psychol. 12:645032. doi: 10.3389/fpsyg.2021.645032
Vogt, A., Babel, F., Hock, P., Baumann, M., and Seufert, T. (2021b). Prompting in-depth learning in immersive virtual reality: impact of an elaboration prompt on developing a mental model. Comput. Educ. 171:104235. doi: 10.1016/j.compedu.2021.104235
Wu, B., Yu, X., and Gu, X. (2020). Effectiveness of immersive virtual reality using head-mounted displays on learning performance: a meta-analysis. Br. J. Educ. Technol. 51, 1991–2005. doi: 10.1111/bjet.13023
Keywords: virtual reality, signaling, desktop virtual reality, cognitive load, instructional design, learning outcome, media in education
Citation: Albus P and Seufert T (2022) Signaling in 360° Desktop Virtual Reality Influences Learning Outcome and Cognitive Load. Front. Educ. 7:916105. doi: 10.3389/feduc.2022.916105
Received: 08 April 2022; Accepted: 09 May 2022;
Published: 27 May 2022.
Edited by:
Bernhard Ertl, Universität der Bundeswehr München, GermanyReviewed by:
Sunawan Sunawan, State University of Semarang, IndonesiaJuliette C. Désiron, University of Zurich, Switzerland
Copyright © 2022 Albus and Seufert. This is an open-access article distributed under the terms of the Creative Commons Attribution License (CC BY). The use, distribution or reproduction in other forums is permitted, provided the original author(s) and the copyright owner(s) are credited and that the original publication in this journal is cited, in accordance with accepted academic practice. No use, distribution or reproduction is permitted which does not comply with these terms.
*Correspondence: Patrick Albus, cGF0cmljay5hbGJ1c0B1bmktdWxtLmRl