- 1College of Geography and Environmental Science, Henan University, Kaifeng, China
- 2Henan Key Laboratory of Earth System Observation and Modeling, Henan University, Kaifeng, China
- 3College of Resources and Environmental Sciences, China Agricultural University, Beijing, China
- 4Experimental Station of Quzhou, China Agricultural University, Beijing, China
- 5BNU-HKUST Laboratory for Green Innovation, Beijing Normal University, Zhuhai, China
The growth of agriculture led to indiscriminate use of synthetic pesticides or fertilizers and unsustainable crop management farming practices which can aggravate harmful impacts on the microbial population and physical and chemical characteristics of soil ecosystem. Based on this fact, the present study was planned to evaluate the effect of long run farming practices on different soil physico-chemical parameters and soil microbial abundance and diversity within different soil depth (0–20 cm and 20–40 cm) at Quzhou Experimental Station of China Agricultural University, Hebei, China during October and December, 2016. The effect of farming practices on soil microbial abundance and diversity was studied by phospho-lipid fatty acid (PLFA) and DNA high-throughput sequencing methods. The findings revealed that soil is neutral to slightly alkaline in nature with highest water content under organic farming (ORF) at 0–20 cm and least under conventional farming at 20–40 cm depth. It was found that the ORF significantly increased the contents of total organic carbon (TOC), total carbon (TC), ammonium nitrogen, available nitrogen (AN), total nitrogen (TN), total phosphorus (TP), and available phosphorus (AP) followed by low input and conventional farming modes in both October and December soil samples. The correlation analysis showed significantly (at p ≤ 0.05 and 0.01) strong positive relationship within different physical and chemical properties of the soil under study. ANOVA and MANOVA analysis indicated significant effect of interaction between soil depth and farming modes on soil parameters. PCA analysis showed the most significant correlation between most of the bacterial types (G + bacteria, G− bacteria, actinomycetes) and soil AP, total available nitrogen, TOC and soil WC. Pearson correlation analysis revealed a significant correlation between microbial phylum groups (Proteobacteria, Bacteroidetes, and Latescibacteria) and microbial class group (Alphaproteobacteria, Sphingobacteriia, Flavobacteriia) with most of the soil physicochemical properties.
Introduction
Soil acts as a key element for food production on which life sustains on this earth. Soil ecosystem provides various functional services, such as maintenance of soil fertility, promoting ecosystem stability, and regulating climate change (Medan et al., 2011; Jing et al., 2015). It is well recognized that anthropogenic disturbances like indiscriminate use of chemical fertilizers and agricultural farming regimes greatly affect soil physical and chemical properties, and biochemical activities. Farming practices can influence water use efficiency and soil moisture content due to indiscriminate fertilizer application, selection of crop type, irrigation management etc. (Niyogi et al., 2010). Continuous tillage can disrupt soil macro-aggregates formation which results in loss of organic carbon (OC) (Nandan et al., 2019), thereby reduction in CO2 concentration in an open atmosphere (Xu and Zhang, 2021). Total organic carbon (TOC) of soil can provide better indication of soil quality and carbon stock (Venkatesh et al., 2013). Nitrogen is another most important nutrient required for better crop production. It improves soil adaptation to environmental stresses like limited water availability and salinity (Hou et al., 2007). Soil TN and organic carbon concentration can show variation along the soil profile and generally decreases with increase in the soil depth. Another important nutrient, phosphorus (P) retention in the near-surface zone of soil shows fluctuation under different tillage methods (Carvalho et al., 2014) and only organic form of phosphorus remain available for plant uptake (Zhu et al., 2016). The available P (AP) in soil can be restricted by both availability and acquirement of P from plant root architecture and mycorrhizal association (Xomphoutheb et al., 2020).
Agricultural farming practices like conventional (COF), low-input (LOF), organic farming (ORF), and many more are commonly employed for management of agricultural fields. Conventional farming type practice can result in low soil fertility, food produce with traces of chemical residues and increasing market cost (Riedo et al., 2021). COF deteriorate physical status of soil, reduce its water and fertilizer use efficiency, and thereby results in growth stagnation and reduced grain yield (Zhou et al., 2014). However, COF was found to increase soil nitrogen and phosphorus accumulation in plants which generally remain unavailable to them (Shannon et al., 2002). The LOF farming system was found to increase storage of excess nitrogen in the soil environment (Clark et al., 1998). ORF is one of the most popularly used farming practices worldwide. It employs use of catch crops, animal manure, organic fertilizers, and recycling of crop residues, also it evades use of plowing in fields (Gajda et al., 2020). ORF strictly avoids use of crop protection chemicals (synthetic mineral fertilizers), which results in better soil biophysical environment, and qualitative agricultural products (Kwiatkowski et al., 2020). ORF is found to enhance soil organic matter and micronutrient accumulation which can improve its quality, structure and can increase soil biological activity; water capacity and infiltration rate (Morshedi et al., 2017; Sankaramoorthy and Rangasamy, 2019; Kwiatkowski and Harasim, 2020; Hietala et al., 2021). Over many advantages, organic type agriculture may lead to nitrogen deficiency in soil and unwanted weed growth which resulted in lower crop yields (Ponisio et al., 2015). ORF produce more nutritious and fewer pesticide residue containing foods as compared to other farming practices (Seufert and Ramankutty, 2017). Soil nutrients can be influenced by agriculture land type and farming method used, and can alter carbon and nitrogen biogeochemical cycles, thereby low soil carbon storage (Wang et al., 2011). Many previous studies reported effect of agricultural farming practices on soil microorganisms and their activities (Zhu et al., 2018). Continuous cropping is one of the factors which can lead to degradation and imbalance in the composition and structure of soil microbial community (Lu et al., 2013; Li Y. et al., 2014).
Soil quality, a comprehensive capacity to sustain better environmental quality as well as high biological productivity results in enhancement of plant health, which can be evaluated by soil indicators, i.e., physicochemical properties and biochemical processes (Bunemann et al., 2018; Ukalska-Jaruga et al., 2019). In soil, presence of different micro and macro-nutrients (Yang et al., 2020), content of nitrogen and some elements (Fe, K, Mg, Mn, Zn); water content, C: N ratio, mineralizable and permanganate oxidizable carbon, soil microbial composition, and soil enzymes activity (Gannett et al., 2019; Ozlu et al., 2019; Thomas et al., 2019) can act as important soil health/fertility indicators. Soil microorganisms contain plenty of fatty acids which vary among each individual organism dwelling in the soil (Willers et al., 2015). Assessment of these fatty acid profiles using phospho-lipid fatty-acid analysis (PLFA) can determine the presence or absence and relative abundance of any type of micro-organism (Gram– and Gram + bacteria, actinobacteria, actinomycetes, fungi etc.) in the soil (Balser et al., 2019; Brinton, 2020). Earlier, effect of agricultural farming practices on soil microbial communities reported use of gene libraries, PCR amplification and gel electrophoresis which can detect presence or absence of some specific microbial groups with high abundance (Chen et al., 2012). The use of next-generation sequencing (NGS) techniques like Illumina-based techniques and pyro-sequencing provides more information and identification of microbial taxa with low-abundance in the soil. It enables analysis of a huge number of taxonomic profiles of soil micro-organisms in detail (Uroz et al., 2013; Zhu et al., 2018).
The information based on agricultural management practices or fertilizer-based alterations in the agricultural soils is still poorly understood. In past, most of the studies were carried out on effect on soil physicochemical and microbiological properties under different farming practices, but effect on abundance and diversity of soil microorganisms was scarcely considered. Therefore, a comprehensive assessment of the influences of farming strategies on important soil parameters (i.e., C−, N−, P−), as well as abundance of soil microorganisms within different layers of soil are necessarily required from an agronomic and environmental point of view. Based on these facts, the present study was aimed to: (i) check the effects of three agricultural farming practices (conventional, low input and ORF systems) on soil physico-chemical parameters including pH, water content (WC) (%), total carbon (TC) (%), TOC (%), ammonium nitrogen (NH4N) (%), nitrate nitrogen (NO3N) (%), available nitrogen (AN) (%), total nitrogen (TN) (%), total phosphorus (TP) (%), and available phosphorus (AP) within different soil layers (15–20 and 20–40 cm) and sampling months (October and December); and (ii) investigate changes in microbial abundance and diversity in response to different farming practices. We hypothesized that three farming practices under study will differentially influence soil physico-chemical parameters and abundance of soil microorganisms within different soil depths.
Materials and methods
Study area
Present study was conducted at China Agricultural University, Quzhou (36°87′N, 115°02′E), Hebei, China. At Quzhou Experimental Station, the long-run greenhouse experiment was set up in March 2002 and has maintained production of vegetables since then. The temperature and humidity maintained in all three greenhouses was found to be 14.8–24.6°C (October), 8.2–17.5°C (December) and 60% while light intensity was 12-h light/dark cycle, respectively. Soil parameters like organic matter (COF- 1.893%; LOF- 1.525%; ORF- 1.663%), AN (COF- 0.136%; LOF- 0.119%; ORF- 0.117%), and AP (COF- 0.0188%; LOF- 0.00816%; ORF- 0.01391%) were measured before the start of experiment in 2002. The station generally remains warm with semi-humid climate which constitutes dry-cold winters and rainy summers. Mean annual temperature recorded is 13.2°C and with main soil type of silt fluvo-aquic texture (Li X. et al., 2014).
The present experiment considered three different greenhouse systems (52 m in length and 7 m in width) under three different farming modes viz., COF, LOF, and ORF (Wang et al., 2021). All the three greenhouses are organized in an integrated way as side by side plots at same place with same soil type (silt fluvo-aquic). The growing vegetable varieties, type of irrigation and tillage methods are same for all the greenhouses under same growing season. Vegetables like cucumber, celery, cauliflower, eggplant, fennel, and tomato are planted in rotation bases in different seasons. Vegetables like celery and cauliflower are grown in all the greenhouses during autumn season at the same time while eggplant is planted in spring season. In conventional type of farming, chemical fertilizers, pesticides, and chicken + cow manure generated from the local style of greenhouse vegetables was used while LOF farming used 50% chemical fertilizer and 50% compost fertilizer and also used low-toxic biological chemical pesticides for protection of plants. The ORF practice was carried out according to the International Federation of Organic Agriculture Movements (IFOAM) and employed chicken and cow manure compost, also used biological and physical methods to protect the crop plants.
Physico-chemical analysis
Soil samples were collected during the months of October (after colonization) and December (before harvesting/maturity), 2016 from all three greenhouses under celery vegetation. Samples were collected at a depth of 0–20 and 20–40 cm according to the model S within 5 points sampling method. A total of four samples were collected from each greenhouse for each farming system during October and December. Fresh soil samples brought to the laboratory were immediately used for analyzing soil microbial abundance and diversity while for physico-chemical analysis, soil samples were dried in the open, put into aseptic bags, properly labeled and stored in refrigerator at 4°C for further analysis.
Different physico-chemical parameters viz., pH, WC, TC, TOC, NH4N, NO3N, AN, TN, TP, and AP; and soil microbial abundance and diversity of soil samples collected from celery growing field were determined. pH (1:5 soil: water) was estimated by pH meter; water content was measured by drying the soil samples for 24 h at 105°C while total C and N contents were measured by dry combustion method (950°C) employing Vario Max CN Analyzer (Elementar; Hanau, Germany). Soil TOC was determined by TOC analyzer (Elementar, liquid TOC II, Germany) (Wang et al., 2021). Soil NH4N content was measured by adding 5 g of soil sample in 25 ml of 2 mol/l KCl in a 250 ml flask (Robertson et al., 1999). The flask was kept on shaker for 1 h and the solution was filtered. In a 50 ml volumetric flask, to 10 ml filtrate, 5 ml of 0.1 mol/l phenol solution and 5 ml of 0.1 mol/l NaClO were added and solution was kept undisturbed for 1 h. After 1 h ammonium nitrogen content of solution was determined by measuring absorbance at 625 nm using spectro-photometer (Shimazu UV-3600, Japan). Further, NO3N was determined by taking 1 ml of the filtered solution into a colorimetric tube to which 25 ml of distilled water was added and absorbance was recorded at 210 nm using spectro-photometer. AN was estimated by diffusion absorption method in which 2 g of air-dried soil was passed through 1 mm sieve and was hydrolyzed by 1 ml/l NaOH to release NH3 and then NH3 was absorbed by adding 20 g/l of H3BO3 (Griesheim and Mulvaney, 2019). The solution was titrated against 0.005 ml/l H2SO4. AP and TP were determined by Olsen et al. (1954) and Bowman (1988) method, respectively.
Soil microbial abundance and diversity
Soil microbial abundance and diversity was determined by phospho-lipid fatty acid (PLFA) and DNA high-throughput sequencing methods.
Phospho-lipid fatty acid technology
PLFAs in soil samples were separated and detected by gas chromatography following standard method of Bligh and Dyer (1959) with slight modifications. Citric acid buffer was used for extraction of phospho-lipids in soil samples. To 8 g of freeze-dried soil sample in white thick centrifuge tubes, single-phase extractant citric acid buffer solution [chloroform: methanol: citric acid ratio, 1:2:0.8 (v/v)] was added, the soil was repeatedly soaked with the extracting solution and shaken well. The extract was poured into a separatory funnel to which 12 ml of chloroform and phosphate buffer was added, shaken well for 2 min and kept undisturbed overnight. For purification of fatty acids, SPE column (3 ml chloroform activated) was used. To the extract, added 5 ml chloroform and later 10 ml acetone and finally rinsed with 5 ml methanol. The methanol phase was collected in test tubes, kept on water bath at 32°C and concentrated with N2.
For esterification, 1 ml of 1:1 toluene: methanol and 0.2 mol/l KOH solution was added to the test tubes, shaken well, incubated at 37°C for 1 h. After incubation, 0.3 ml of 1 mol/l acetic acid solution, 2 ml of n-hexane and 2 ml of ultra-Pure water was added to test tubes, shaken at low speed for 10 min (repeated shaken and leached once), the upper n-hexane solution was transferred into vials. Sample was dehydrated and dried on water bath under N2 and methyl esterified fatty acid samples were obtained. The methyl esterified fatty acid samples were dissolved in 200 μl of n-hexane and PLFAs in samples were identified and analyzed on a gas chromatography (Agilent 6890N, USA) using MIDI software system (MINDI, Newark, Delaware, USA) combined with SherlockMIS4.5 system (Sherlock Microbial Identification System). PLFAs were assigned with different biomarkers for different microbial groups according to method of Kandler and Konig (1998).
DNA high-throughput sequencing technology
High-throughput sequencing technique was used to study effect of farming practices on soil microbial relative abundance and diversity. FastDNA SPIN Kit (MP Biomedicals, LLC, USA; Protocol: #116560200-201908/#116560000-201908) was used to extract microbial DNA from soil samples according to the manufacturer’s protocol earlier used in many studies (Song et al., 2017; Hemkemeyer et al., 2018; Paul et al., 2018). SPIN kit can efficiently isolates PCR-ready genomic DNA directly from soil samples in less than 30 min. The DNA was extracted from 0.5 g of fresh soil by using MT and phosphate buffers and repeated centrifugation using SPIN™ filters. The collection tube containing eluted DNA was stored at −20 or −80°C for further processing (Niu et al., 2017). The Illumina Mi Seq 2 × 300 sequencing platform was used to perform paired-end sequencing analysis of PCR amplification products. The concentration of DNA samples was assessed using a Qubit 2.0 Fluorometer (Invitrogen, Carlsbad, CA) and sequencing libraries were created using the MetaVx™ Library Construction Kit (GENEWIZ, Inc., South Plainfield, NJ, USA). All the reactions were performed in duplicate. Two reads per individual library were taken. DNA was PCR amplified using 16S rRNA gene prokaryotic primers. The 515F (GTG CCA GCM GCC GCG G) and 907R (CCG TCA ATT CMT TTR AGT TT) primers were designed to amplify the hyper-variable regions (V3 and V4) of the 16S rRNA gene from bacteria (Roesch et al., 2007). Using 30–50 ng DNA as template, a series of PCR primers designed by GENEWIZ were used to amplify both the hyper-variable regions on prokaryotic 16S rRNA. Also, a linker with Index was added to the end of the PCR products of 16S rDNA for NGS sequencing. The sequencing was commissioned by GENEWIZ (Suzhou, China).
Statistical analyses
Results were analyzed and processed in Microsoft Excel 2007 using SPSS software (ver. 20.0). First, multivariate analysis of variance (MANOVA) analysis was carried out to check whether there was a significant effect of independent variables (sampling time, soil depth, and farming modes) on at least one of the soil physico-chemical parameters. Then, univariate analysis of variance (ANOVA) along with Tuckey’s and Duncan’s test for different soil parameters was performed to examine the significant effect of sampling time, soil depth and farming modes and differences in mean at p ≤ 0.05 were taken as significant. Correlation between different soil physico-chemical parameters and microbial abundance based on PLFA content was carried out using Canoco 4.5 software. Pearson’s correlation (at p ≤ 0.01 and p ≤ 0.05) analysis was also performed among different physico-chemical parameters of soil; soil microbial phylum with soil physico-chemical properties; and soil microbial class with soil physico-chemical properties. Also, On the basis of OTU clustering results, Alpha diversity indices including Chao index, ACE index, Simpson and Shannon index were also calculated.
MANOVA analysis was carried out to check the variance analysis of different soil parameters which can reflect the significance levels of different soil parameters differences at different sampling times, soil depths, and farming modes. Four MANOVA tests (Wilks’ Lambda, Lawley-Hotelling, Pillai’s Trace, and Roy’s Largest Root) values are selected, while the difference levels between groups correspond to F-values (Matheron, 1963).
Results
Physico-chemical parameters
The 14-year (2002–2016) long-run farming practices were observed to change soil physico-chemical properties to some extent (Table 1). It was observed that the soil under all three farming regimes is neutral to slightly alkaline (pH = 7.3–8.1) in nature. The differences were found to be insignificant (at p ≥ 0.05). The soil samples collected at 0–20 cm soil depth during October and December months showed lower pH than that of samples collected at 20–40 cm soil depth. At 0–20 cm soil depth, ORF showed increase in soil pH whereas, at 20–40 cm soil depth, conventional farming increased pH of the soil followed by organic and LOF farming modes. Overall results showed increase in soil pH with increase in soil depth i.e., low pH was observed in soil samples collected from 0 to 20 cm depth while high pH was recorded in samples taken from 20 to 40 cm soil depth.
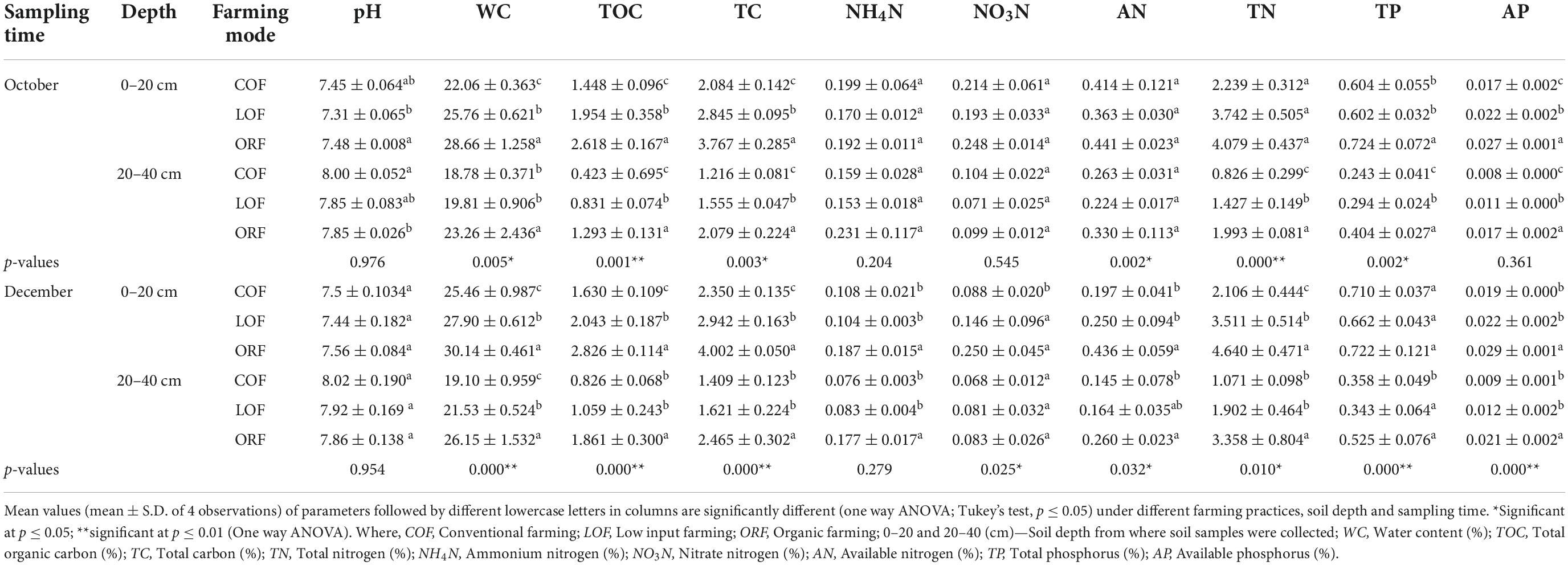
Table 1. Effect of different farming practices on soil parameters within different soil depths and sampling months.
The variation among water content (moisture content) was significant in both December and October soil samples. The water content recorded under organic, low input and conventional farming modes was found to be 30–43, 24–38, and 23–34%, respectively. WC was significantly lower under conventional farming as compared to other farming practices. The change in water content in 0–20 cm soil depth was significantly higher than that in 20–40 cm depth under same farming mode during same month of sampling with an increasing percentage range between 21 and 28%. Soil TOC and TC contents under low input and ORF modes increased significantly after long-term application of bio-compost. The contents of TOC and TC in the soil samples were significantly higher under ORF followed by low input and conventional farming modes. The contents of both forms of carbon were found higher in December soil samples collected from 0 to 20 cm soil depth while October soil samples collected within 0–20 cm depth showed low contents.
The soil ammonium nitrogen (NH4N) content was observed to be insignificantly higher under ORF, and lowest under conventional and low input modes, and the content in the 0–20 cm soil depth was higher than that in the 20–40 cm soil layer. It was found that NH4N content under conventional and low input farming modes was higher in October samples as compared to December ones. Under all three farming modes, change in soil NO3N content at 0–20 cm depth was higher than that of 20–40 cm soil depth. NO3N content of soil samples collected during December from 0 to 20 cm soil depth followed a significant trend as: ORF > low input farming > conventional farming, while from soil depth of 20–40 cm, the trend was observed to be: conventional farming > ORF > low input farming significant at p ≤ 0.05. The AN content of soil samples collected from 0 to 20 cm soil depth showed no significant difference under organic and low input modes; however, it was significantly higher than the conventional farming mode. October soil samples collected from 20 to 40 cm depth showed variation in trend of AN content in ORF which was significantly higher than that in conventional and low input modes. Overall results revealed that the variation in soil AN content at 0–20 cm soil depth was significantly higher than that in 20–40 cm soil depth and October soil samples showed higher content of AN as compared to soil samples collected during December month. The TN content in December soil samples was found to be higher than that in October samples. Within 0–20 cm soil depth, no significant differences in TN content were observed in organic and low input farming mode, however, content was higher than that of conventional type of farming. The variation of soil TN content under all three farming modes followed a trend as: ORF > low input farming > conventional farming mode. The change in soil TN content at 0–20 cm depth was higher than that in 20–40 cm soil depth and TN content was found to be significantly higher in December soil samples as compared to the October samples. The variation in total phosphorus (TP) content of soil was observed to be significantly higher at 0–20 cm soil depth as compared to 20–40 cm soil depth. The TP content of December soil samples was higher than that of October samples. The soil samples collected from 0 to 20 cm depth showed TP content in a trend as: ORF > conventional farming > low input farming while, soil samples collected from 20 to 40 cm depth revealed a trend as: ORF > low input farming > conventional farming mode. The variation in AP in soil collected from fields under three farming modes follow a trend as: ORF > low input farming > conventional farming mode. The content of AP content was significantly higher in October soil samples than in December one and at 0–20 cm soil depth AP was found to be higher than that at 20–40 cm soil depth. It can be observed that application of organic fertilizers significantly increased soil total and AP content under ORF mode.
MANOVA analysis showed the effect of interaction between independent variables (sampling time*soil depth; sampling time*farming modes; soil depth*farming modes; and sampling time*soil depth*farming modes) on the dependent variables (pH, WC, TC, TOC, NH4N, NO3N, AN, TN, TP, and AP). The descriptive results for MNOVA are given in Table 2. Pillai’s Trace, Hotelling’s Trace, and Roy’s Largest Root values were all significant at p ≤ 0.01 where Hotelling’s Trace value was almost equal to Pillai’s Trace value which indicates that there was a significant interaction of independent variables with some of the dependent variables. Hotelling’s Trace and Roy’s Largest Root values were also similar which also indicate significant interactive effect of independent variables with some of the dependent variables. The output generated reveals that there exists significant effect of interaction between soil depth and farming modes on soil WC (F = 8.800; p = 0.001), TC (F = 10.721; p = 0.000), TN (F = 7.303; p = 0.002), TP (F = 5.942; p = 0.006), and AP (F = 5.396; p = 0.009) whereas pH (F = 1.389; p = 0.262), TOC (F = 1.337; p = 0.275), NH4N (F = 1.280; p = 0.290), NO3N (F = 0.834; p = 0.443), and AN (F = 1.562; p = 0.223) were insignificantly effected by the interaction. Interaction effect of soil depth*sampling time showed significant effect on NO3N (F = 4.390; p = 0.043) content whereas, all other soil parameters showed insignificant effect. Also, there was no significant interaction effect of different farming modes*sampling time; and farming modes*sampling time*soil depth on any of the soil parameters.
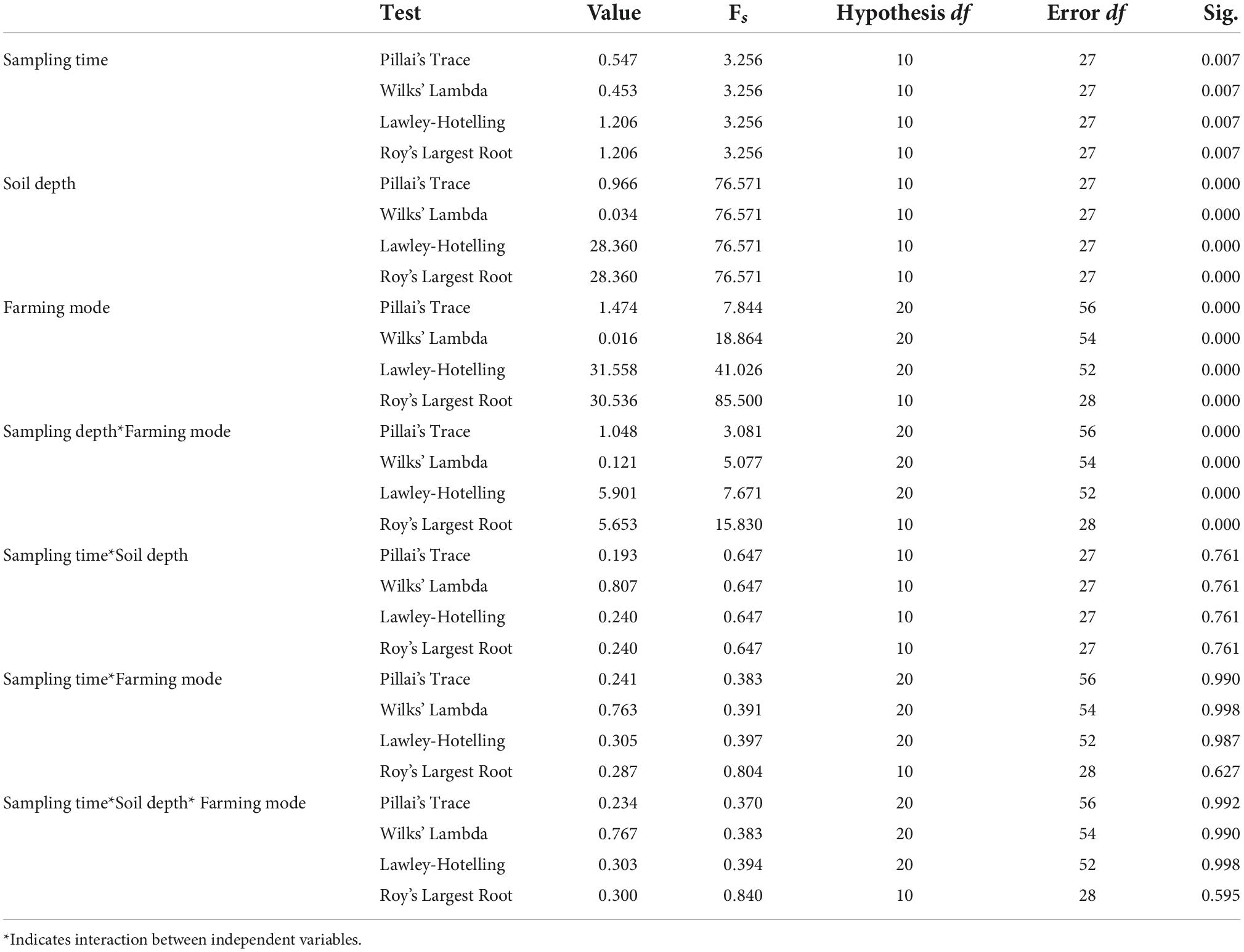
Table 2. MANOVA results for soil samples collected during different farming practices within different soil depths and sampling months.
Soil microbial abundance and diversity
Phospho-lipid fatty acids
PLFA analysis detected biomarkers for 33 microorganisms in the tested soil samples, out of which only 22 biomarkers greater than 0.1% were selected for the further study (Supplementary Tables 1, 2). It was observed that the PLFA markers of soil microorganisms under different farming modes were significantly different and were completely distributed within 0–20 and 20–40 cm soil depths (14:0, 16:0, 18:0, 15:0 anteiso, 15:0 iso, 16:0 10-methyl, 16:0 iso, 16:1 2OH, 17:0 anteiso, 17:0 cyclo, 17:0 iso, 17:1 iso w9c, and 19:0 cyclo w8c) etc. while, some biomarkers like 15:0, 17:0, 20:0, 16:1 w7c, 17:0 10-methyl, 18:0 10-methyl TBSA, 18:1 w9c, 18:3 w6c (6, 9, 12), and 20:4w6, 9c were not distributed. The content of the PLFA biomarkers in the 0–20 cm soil depth samples was significantly higher than that in the 20–40 cm depth soil samples. Soil samples collected during December showed more microorganisms with biomarkers of 16:1 w7c while October samples showed microorganisms with biomarkers 15:0, 17:0, 20:0, 18:0 10-methyl TBSA, 18:3 w6c (6,9,12), and 20:4w6.
The most abundant PLFA biomarkers whose content was greater than 5% were found to be 16:0 (gram-positive bacteria) followed by 19:0 cyclo w8c (gram-negative bacteria), 16:0 10-methy1 (Actinomycetes), 15:0 iso (gram-positive bacteria), and 17:1 iso w9c (gram-negative bacteria) (Figure 1). However, the PLFA content of reported five types of microorganisms showed variation under different farming modes and followed a trend as: ORF > LOF > COF. No fungal community was reported in 20–40 cm soil depth while in October soil samples, the PLFA content of Gram-positive bacteria and Actinomycetes was highest in LOF and ORF modes and lowest in COF mode. The PLFA content of various microorganisms was reported higher in ORF followed by COF and LOF mode in soil samples collected during December month. Overall results showed that the abundance of gram-positive and gram-negative bacteria was significantly higher under ORF mode followed by LOF and conventional mode. The total PLFA content of soil can indirectly represent the total amount of soil microorganisms. It can be seen that the changing pattern of soil total PLFA content under different farming modes followed a trend as: organic mode > LOF mode > conventional farming mode (Figure 2). The variation in soil total PLFA was reported to be highest at 0–20 cm soil depth as compared to 20–40 cm depth. ORF management practice showed significant increase in soil total PLFA content during October than in December. Under conventional farming mode, soil total PLFA content was higher during December month as compared to October while LOF farming mode revealed insignificant difference in total PLFA content of soil during both the sampling times.
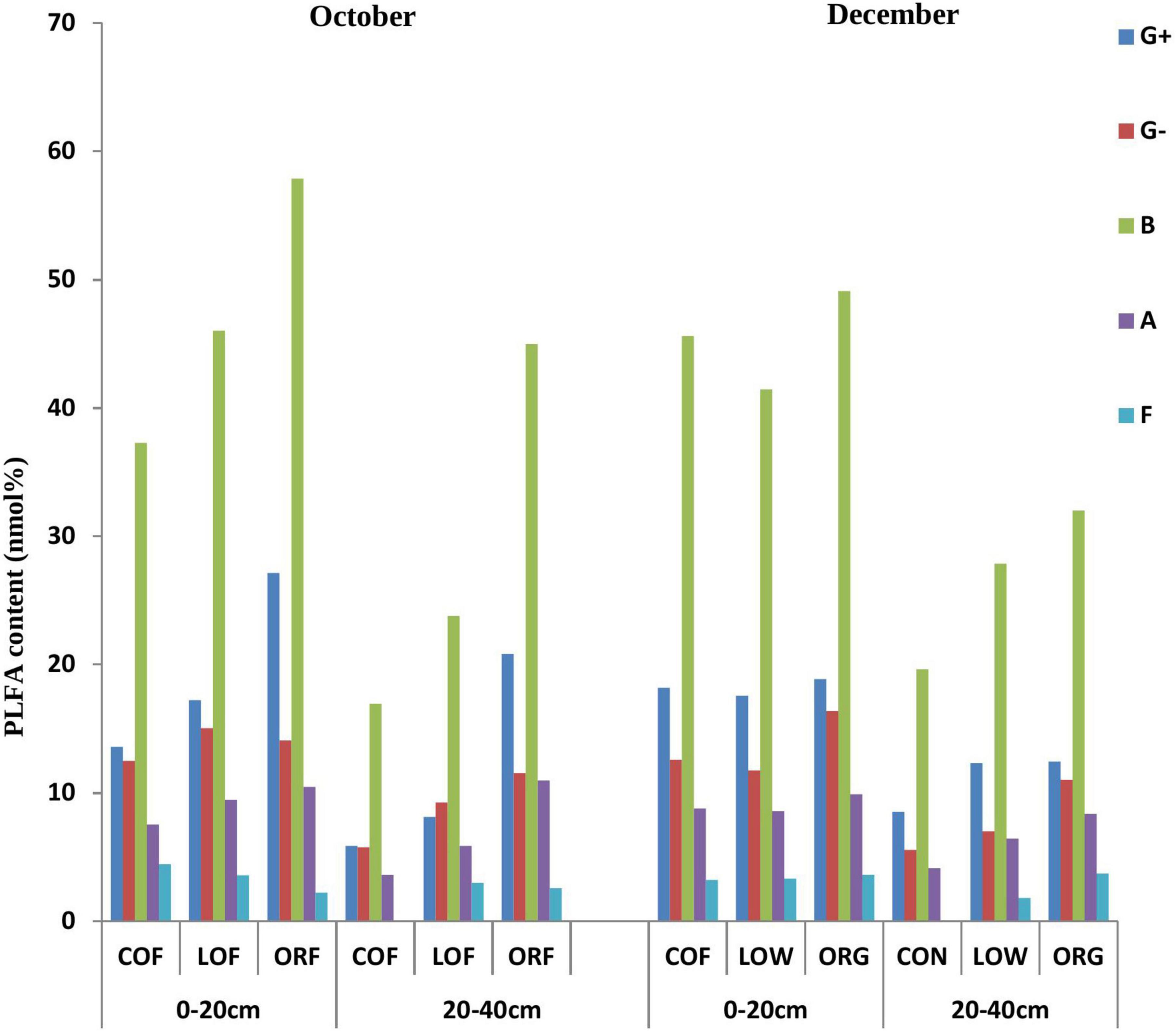
Figure 1. Effect of different farming practices on PLFA content of various soil microbial types within different soil depths during different sampling months. Where, G +, Gram-positive bacteria; G–, Gram-negative bacteria; B, Bacteria; A, Actinomycetes; F, Fungi; October and December- Months of soil sampling; PLFA, Phospho-lipid fatty acids; COF, Conventional farming; LOF, Low input farming; ORF, Organic farming; 0–20 and 20–40 (cm)—Soil depth from where soil samples were collected.
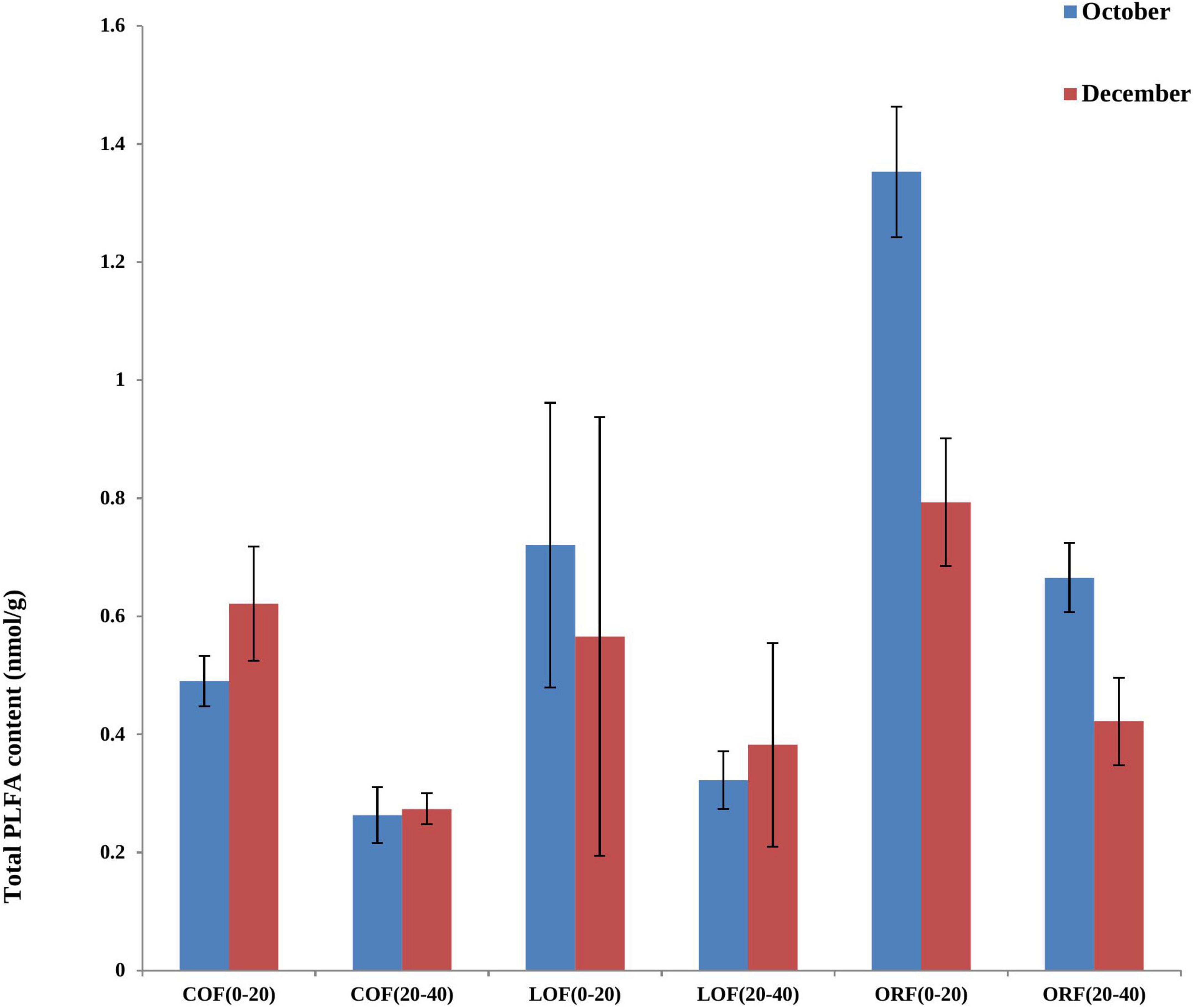
Figure 2. Effect of different farming practices on total PLFA content of soil within different soil depths during different sampling months. Where, October and December- Months of soil sampling; PLFA, Phospho-lipid fatty acids; COF, Conventional farming; LOF, Low input farming; ORF, Organic farming; 0–20 and 20–40 (cm)—Soil depth from where soil samples were collected.
DNA high-throughput sequencing
Sequences were obtained through DNA high-throughput sequencing and it extracted 29,689 sortable operational units (OTUs) with 97% sequence similarity. The OTUs revealed microorganisms from 2 kingdoms, 27–30 phyla, 62–67 classes, 72–81 orders, 129–143 families, 154–186 genera, and 15–20 species (Supplementary Table 3). It was observed that, under different farming modes, soil depths and sampling times there was a mild effect on the abundance and diversity of soil microorganisms at various taxonomic levels but differences in the structural distribution of microorganisms were reported. In the present study, the relative abundance and diversity of microorganisms was studied at phylum and class level of classification. At the phylum level of classification, 30 phyla were reported in the metagenomic test samples, and the unclassified phyla accounts for 0.01–0.08%. The relative abundance of more than 0.1% was selected (Figure 3). Figure 3 is drawn for 11 phyla which revealed Proteobacteria as the main dominant phyla followed by Acidobacteria, Bacteroidetes, Actinobacteria, Chloroflexi, Gemmatimonadetes, Firmicutes etc., each accounted for 24–44%, 19–30%, 6–23%, 3.8–6.2%, 3.8–8.3%, 0.9–4.3%, 0.7–2.2% of the total microbial content, respectively. Among them, Proteobacteria, Acidobacteria, and Bacteroidetes showed higher relative abundance of 95%, followed by Actinobacteria, Chloroflexbacteria etc. The relative abundance of various phyla was found to be insignificantly affected within different soil depths (0–20 and 20–40 cm), but significant differences were observed in microbial abundance in response to different farming modes and sampling months.
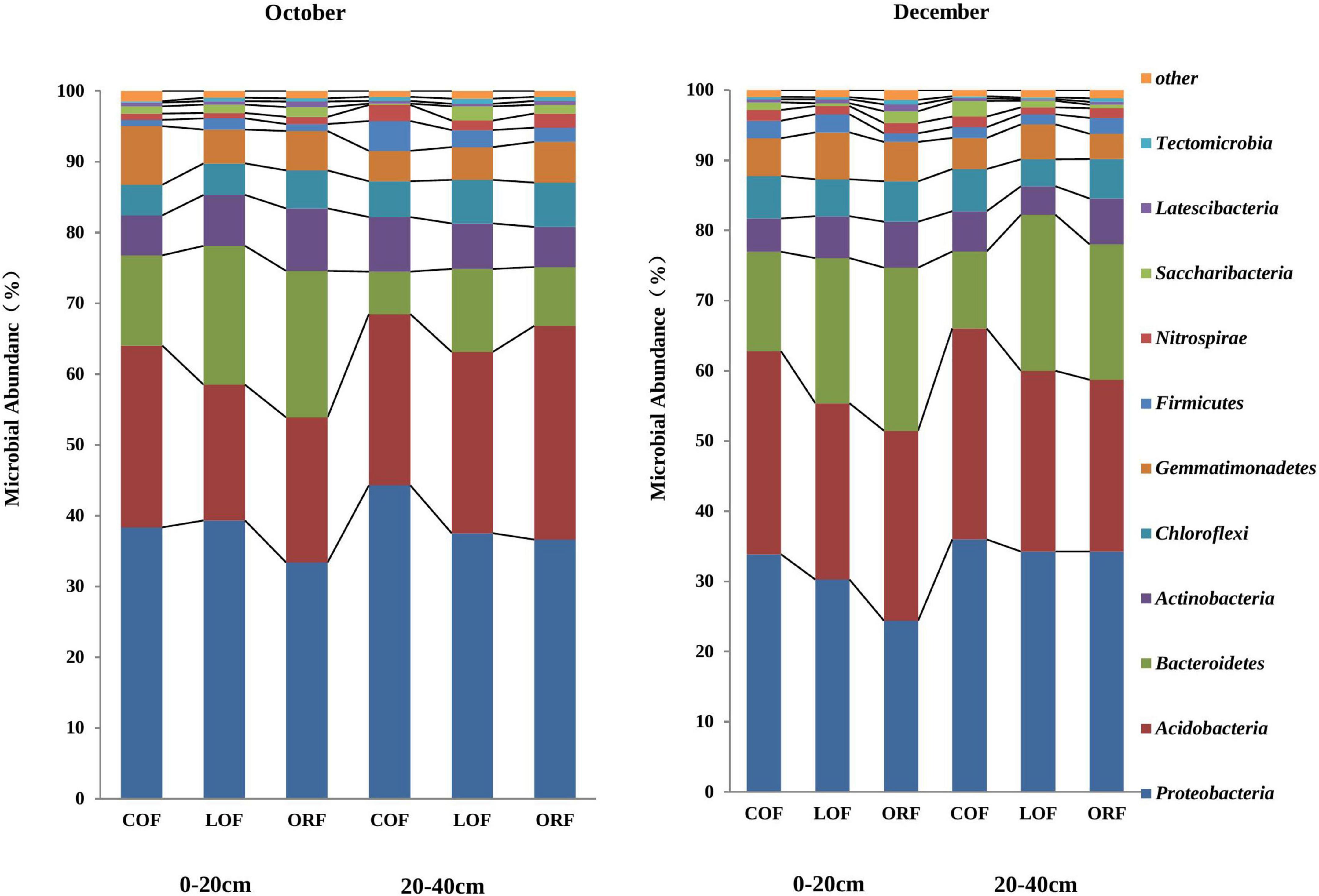
Figure 3. Effect of different farming practices on soil microbial abundance at class levels within different soil depths and sampling months. Where, October and December- Months of soil sampling; COF, Conventional farming; LOF, Low input farming; ORF, Organic farming; 0–20 and 20–40 (cm)—Soil depth from where soil samples were collected.
The relative abundance of Proteobacteria in October soil samples at 0–20 cm soil depth was observed to be significantly higher under LOF farming mode and slightly lower in the conventional and ORF modes whereas, the abundance of Acidobacteria was found significantly higher under conventional mode and lower under LOF and organic modes. The abundance of Bacteroidetes was significantly higher at 0–20 cm depth than that in the 20–40 cm soil depth. The relative abundance of Actinobacteria in soil samples collected from 0 to 20 cm soil depth showed a trend as: organic mode > LOF mode > conventional mode while at 20–40 cm soil depth, the trend was observed to be: conventional mode > LOF mode > organic mode. The relative abundance of Chloroflexbacteria at 20–40 cm depth was significantly higher than that at 0–20 cm soil depth, highest under conventional farming mode followed by organic and LOF farming modes. During December, the relative abundance of Proteobacteria and Acidobacteria was found to be highest under conventional mode followed by LOF and ORF modes. Bacteroidetes abundance at 0–20 cm was significantly higher under organic mode while at 20–40 cm, the relative abundance was highest in the LOF mode as compared to the organic and conventional farming modes. The abundance of Actinobacteria and Chloroflexbacteria in 20–40 cm soil depth showed insignificant difference between farming modes while at 0–20 cm depth, the abundance of Actinomycetes was reported to be highest in the organic mode followed by LOF and conventional modes. The abundance of Chloroflexi was not found to be affected under any farming modes while phylum Bacillus showed significantly higher abundance under LOF mode as compared to other two modes.
At the class level of classification, there were 67 classes of bacteria in the metagenomic testing samples and the relative abundance of unclassified bacterial classes was 11.91–22.59% (Figure 4). Figure 4 is plotted for 11 classes of microorganisms with an abundance of more than 0.1% and the main dominant classes included Alphaproteobacteria, Gammaproteobacteria, Sphingobacteriia, Blastocatellia, Betaproteobacter, Actinobacteria, Deltaproteobacteria, Gemmatimonadetes, Acidimicrobiia, and Nitrospira. Among them, the abundance of Alphaproteobacteria, Gammaproteobacteria, Sphingobacteria, and Blastocatellia was relatively high with the total microbial abundance of 40–60%. In addition, the relative abundance of fungi classes showed obvious differences under different farming modes within different soil depths during different sampling times. The relative abundance of Alphaproteobacteria and Blastocatellia was higher in conventional mode while, the presence of Sphingobacteria at 0–20 cm soil depth was higher in LOF mode while at 20–40 cm depth, the abundance was higher under conventional farming mode. Supplementary Table 4 showed that at 97% similarity level sequencing results, the coverage rate of the established microbial library in all soil samples was between 99.4 and 99.6%, which can truly reflect the OTU composition of the microbial community of samples and the information has high degree of confidence, which have high research significance and practical value.
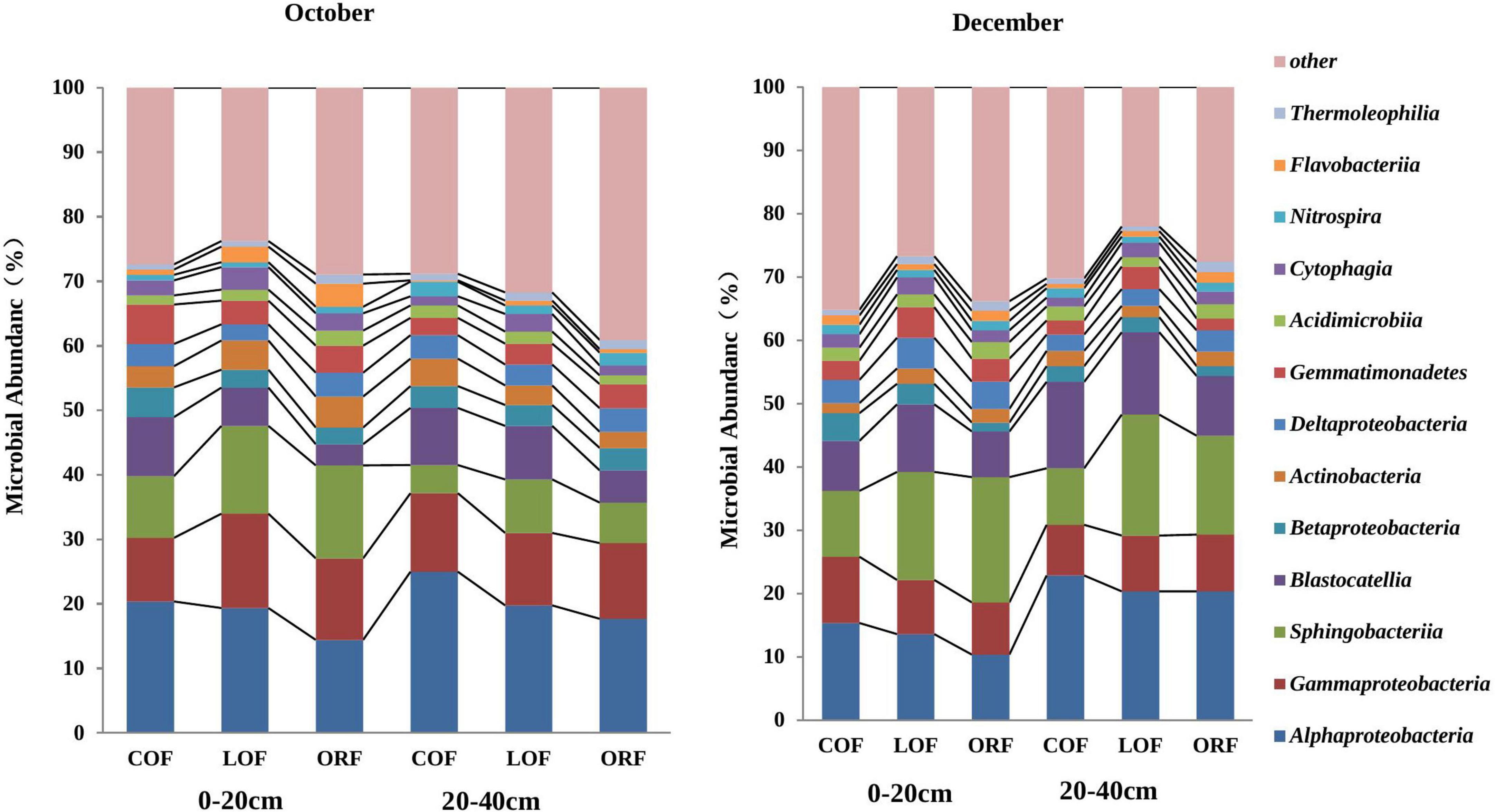
Figure 4. Effect of different farming practices on soil microbial abundance at phylum levels within different soil depths and sampling months. Where, October and December- Months of soil sampling; COF, Conventional farming; LOF, Low input farming; ORF, Organic farming; 0–20 and 20–40 (cm)—Soil depth from where soil samples were collected.
The ACE richness, Chao index and Shannon diversity indices of the microbial community were found to be affected by different farming modes during different sampling periods while Simpson index was found to be unaffected (Supplementary Table 4). In October, all three indices were observed to be higher under LOF mode while low in Chao and ACE richness index was observed in organic and conventional modes. The Shannon diversity index was higher in the ORF mode as compared to conventional mode. During late stage of celery production (December), the Chao and ACE richness index and Shannon diversity index of the microbial community did not show any effect of farming modes, which might be attributed to celery crop and its effect on soil microorganism’s abundance.
Pearson’s correlation analysis
Pearson’s correlation analysis revealed significant correlation among almost all of the physico-chemical parameters of the soil samples except for pH, NH4N, NO3N, and TP which showed insignificant correlation with other soil parameters. Significantly strong positive correlations were observed between AP and TOC (r = 0.969) and TC (r = 0.962) at p ≤ 0.01 while, TN content showed strong positive significant correlation with organic carbon (r = 0.913, p ≤ 0.01). Other parameters like TOC-WC (r = 0.941); TC-WC (r = 0.936); TC-TOC (r = 0.989); TN-TOC (r = 0.931); TN-TC (r = 0.905); AP-WC (r = 0.944); NO3N-AN (r = 0.919) also revealed strong significant positive correlation between each other at p ≤ 0.01 level of significance.
Also, correlation between different soil physico-chemical parameters and soil microorganisms groups based on PLFA content was performed using Canoco 4.5 software, RDA analysis (Figure 5). The total explained amount of the two principal components in the two periods reached 99.9%. Among them, the first principal component (PC1) explained 93.4 and 86.1% of the variables, and the second principal component (PC2) explained the variables were 6.5 and 13.8%. In October, the most significant correlation was observed between most of the bacterial types and soil AP, total available nitrogen, TOC and soil water content. The abundance of microbial population was negatively correlated with pH. There was a significant positive correlation among G + bacteria, G− bacteria, fungi, actinomycetes and total bacteria, and all were at the negative end of the second principal component and the correlations between various microbial populations reached at significant to highly significant level. In December, there was a significant positive correlation between G + and G− bacteria, fungi, actinomycetes and total bacteria, and they were also at the negative end of the first principal component and the second principal component and the correlations between various microbial populations reached to a significant and extremely significant levels. Bacterial population, G + bacteria, G− bacteria, actinomycetes, and fungi were observed to be positively correlated with soil TOC, TC, TN, nitrate nitrogen, total available nitrogen, TP, AP and water content, among which soil TN, TP, TOC correlation was the most significant. The ratio of G + bacteria to G− bacteria was observed to be extremely significant and negatively correlated with soil ammonium nitrogen and positively correlated with soil pH.
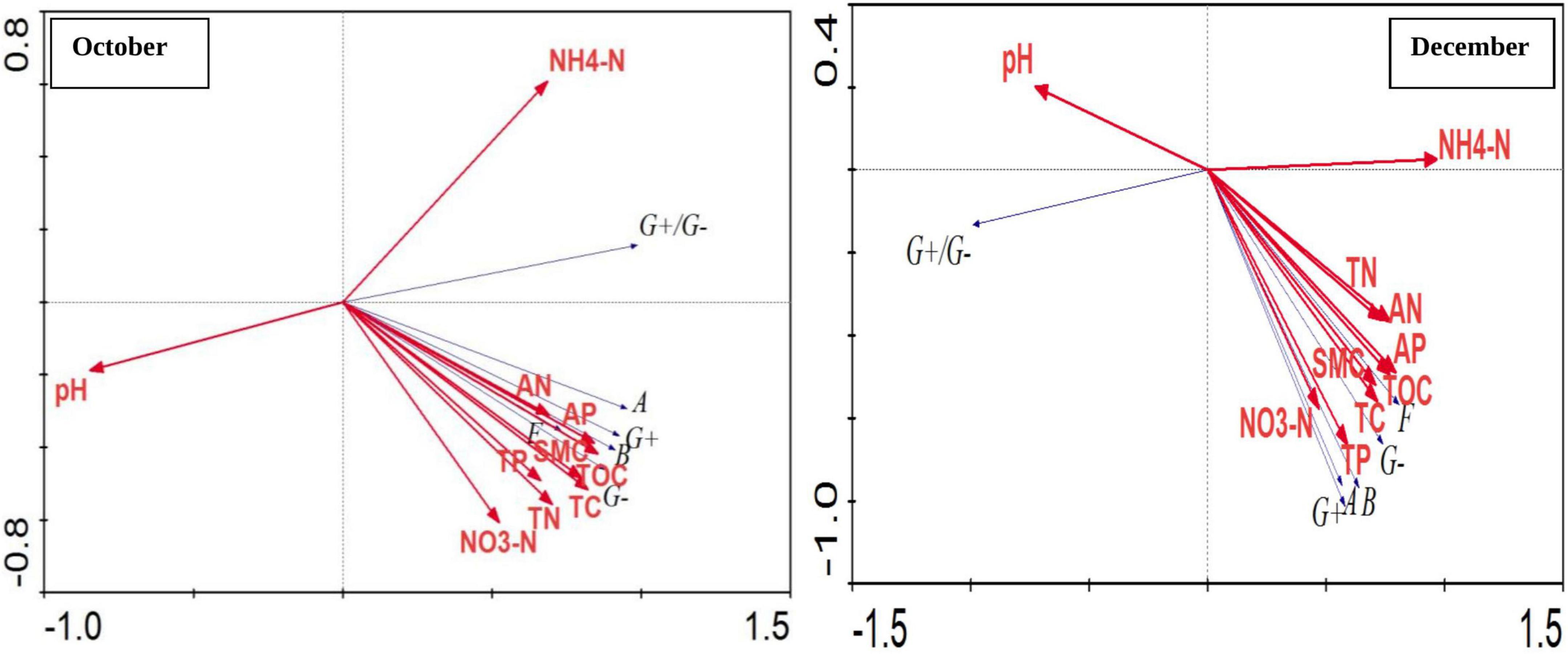
Figure 5. Correlation between different soil physico-chemical parameters and soil microbial groups based on PLFA content. Where, October and December- Months of soil sampling; G +, Gram-positive bacteria; G–, Gram-negative bacteria; B, Bacteria; A, Actinomycetes; F, Fungi; WC, Water content (%), TOC, Total organic carbon (%), TC, Total carbon (%), TN, Total nitrogen (%), NH4N, Ammonium nitrogen (%), NO3N, Nitrate nitrogen (%), AN, Available nitrogen (%), TP, Total phosphorus (%), AP, Available phosphorus (%).
Table 3 shows correlation analysis between different soil physicochemical properties and microbial phylum groups. Presence of Proteobacteria, Bacteroidetes, and Latescibacteria showed significant correlations with most of the soil physicochemical properties where Proteobacteria was negatively correlated with WC, TOC, TC, and AP at significance level of p ≤ 0.01 whereas TN, TP at p ≤ 0.05. Bacteroidetes community was found positively correlated with soil WC, TOC, TC, TN, and AP at a significant level of p ≤ 0.01 while TP at p ≤ 0.05. Actinobacteria population was found positively correlated with soil AN at a significance level of p ≤ 0.01 whereas presence of Gemmatimonadetes was observed to be negatively correlated with soil pH at p ≤ 0.05. Presence of Latescibacteria was positively correlated with WC, TOC, TC, TN NO3N, AP, and AN at p ≤ 0.01 whereas TP reached a significant level of p ≤ 0.05. Chao index was negatively correlated with presence of Latescibacteria presence. Table 4 represents correlation analysis between different soil physicochemical properties and microbial class groups. Presence of Alphaproteobacteria revealed significant negative correlation with WC, TOC, TC, TN, TP, and AP at significance level of p ≤ 0.01 and NO3N at p ≤ 0.05 while soil pH showed significant positive correlation at p ≤ 0.05. Sphingobacteriia population was found positively correlated with TN at a significance level p ≤ 0.01 while WC, TOC, TC, and AP at a level of p ≤ 0.05. Blastocatellia presence was found negatively correlated NO4N and AN at a significance level of p ≤ 0.01 while AP and Shannon index at a level of p ≤ 0.05. Deltaproteobacteria was found negatively correlated with Chao index of richness while Gemmatimonadetes presence was observed to be negatively correlated with soil pH at the significance level of at p ≤ 0.05. Presence of Cytophagia was negatively correlated with soil pH and Shannon index at p ≤ 0.01 whereas Nitrospira was found positively correlated with soil pH at p ≤ 0.05 level of significance. Flavobacteriia population was positively correlated with TOC, TC, TN, AP (at p ≤ 0.01), WC, NO3N, TP and Shannon index (at p ≤ 0.05) while negatively correlated with soil pH at p ≤ 0.05.
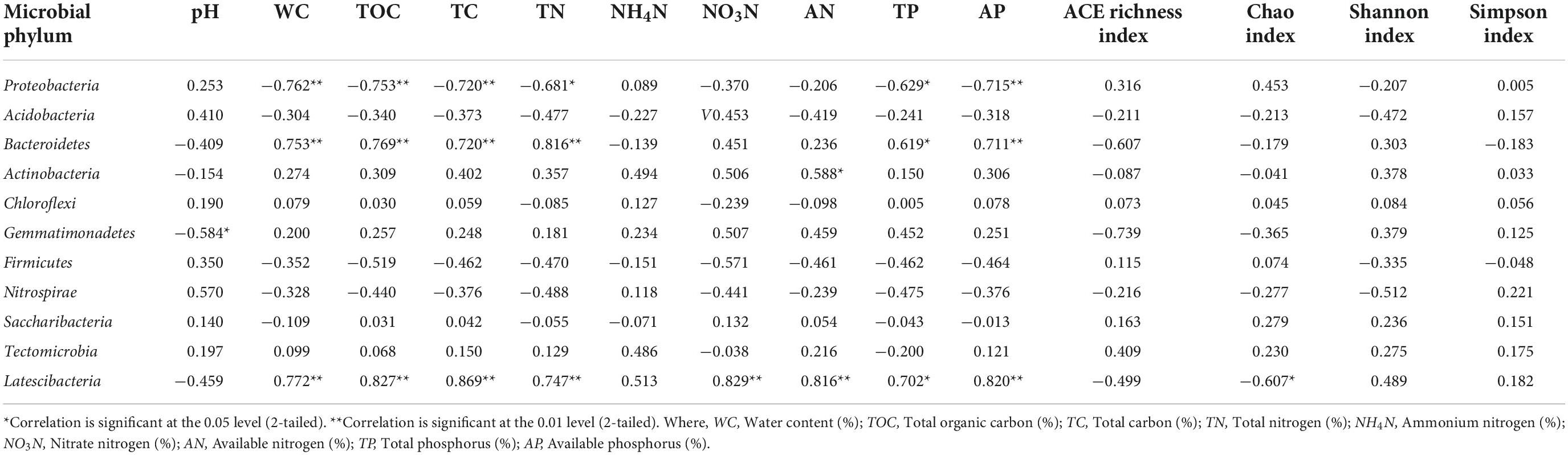
Table 3. Correlation between the soil microbial abundance (phylum level), soil physico-chemical properties and Alpha diversity indices.
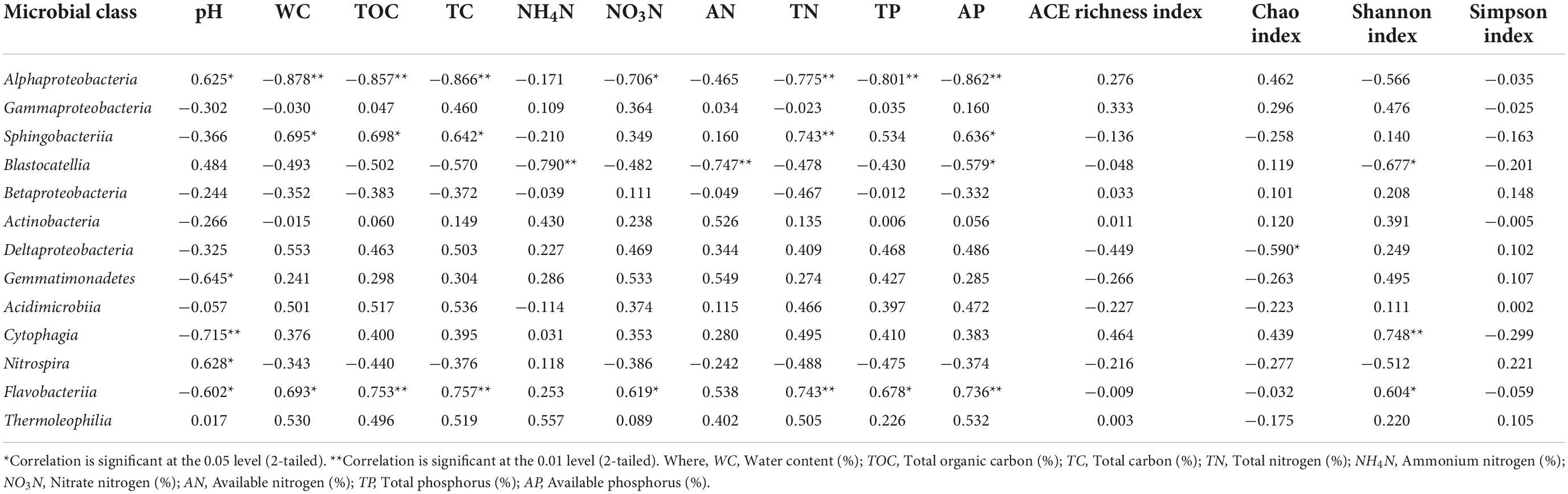
Table 4. Correlation between the soil microbial abundance (class level), soil physico-chemical properties and Alpha diversity indices.
Discussion
In the present study, soil pH was observed to be slightly alkaline in nature where alkalinity of soil increases with increase in the soil depth under ORF. Similarly, Kwiatkowski and Harasim (2020) reported high pH in organically managed soil as compared to other conventional farming modes. Organic material introduced in the soil can enhances the soil buffering capacity and Al3+ chelation which results in increased soil pH (Baolan et al., 2014). The variability in pH within different soil depths can be attributed to increase in downward solutes movement of soil bases within the soil profile due to leaching (Assen et al., 2005) and enhanced levels of carbonate ions and less weathering rates in soil (Malo et al., 2005). Soil moisture content can influence the pH by altering soil chemical equilibrium, and changing soil nutrient forms and their effectiveness (Morales et al., 2011). Soil pH can increase due to the application of organic residues in the soil (Manna et al., 2005; Huang et al., 2016) or due to retention of crop residues (Liu et al., 2010; Butterly et al., 2011). In organically farmed soil, the water content was found higher at the upper soil surface as compared to deep surface. Pimentel et al. (2005) also observed the application of organic fertilizer can increase soil water storing capacity. The presence of high organic matter in soil can provide a ground cover which can help in reducing soil temperature resulting in less water evaporation (Calvino and Monzon, 2009). Hathaway-Jenkins et al. (2011) in their study reported high infiltration rate and reduced runoff in agricultural soils of England managed under ORF mode. One of the recent studies by Durrer et al. (2021) observed that organic treatment of soil can maintain high water stock in the soil due to presence of high organic matter.
It was observed that organic fertilizer input rapidly increased the contents of soil carbon at the 0–20 cm soil depth which might be attributed to bio-compost application. For assessing productivity of any agricultural soil, organic carbon can be used as one of the most important indicators (Shukla et al., 2006). Similar to the present study, the relatively higher OC in upper surface (0–20 cm) of soil was observed by Selassie and Ayanna (2013) in their study and attributed this increase to relatively enhanced return of soil biomass at the surface due to microbial decomposition. Also, Wakene (2001) reported high OC and TOC in the surface soil which is the most biologically active layer in the whole soil profile. High carbon accumulation in agricultural soil can be credited to leaf litter fall on the surface and also through root deposition in deeper soil layers (Zhou and Wang, 2017). Present results were also supported by findings of Mandal et al. (2018) and Kwiatkowski and Harasim (2020). Contrary to the present results, Sharma et al. (2020) reported that intensive cultivation, inappropriate tillage and other management practices can increase soil organic carbon loss through high microbial activities and oxidation process. The other form of carbon, i.e., soil inorganic carbon (IC) contains compounds like carbonate ions, calcium and magnesium carbonate deposits in soil solution and CO2 in soil air. In the present study, soil inorganic carbon can be calculated by subtracting TOC from TC. It was observed that IC was relatively high in ORF system as compared to LOF and COF farming systems. Mostly arid and semiarid climate soils have high content of IC as compared to moist soils which have very low IC content due to long turnover period and occurrence of chemical processes which shows little effect on soil fertility (Kowalska et al., 2020).
Long-term ORF and vegetable cultivation increased soil ammonium nitrogen at upper surface (0–20 cm) and lowest in the conventional and low input modes (20–40 cm depth). Organic N soil stock increased due to decrease in nitrogen leaching (Kwiatkowski and Harasim, 2020). Soil NO3N, AN and TN contents was found to be highest at upper surface under ORF. The application of organic fertilizer can not only improve soil TN storage, but also have a certain effect on ammonium nitrogen in soil and AN components. Higher organic input (leaf litter) can enhance soil nitrogen mineralization (Zhou and Wang, 2017). Similar results were observed by Drinkwater et al. (1995) and Wang et al. (2011) who recorded higher total N content and high mineralization rate in organically managed soils as compared to conventional ones. Also, Kwiatkowski and Harasim (2020) in their study reported high TN and NH4N content in top layer of agriculture soil and attributed this to type of fertilizers used in ORF which are highly mineralized and can stimulate high soil enzymatic activity resulting in higher nitrogen release in the soil. They also reported low content of NO3N in organically managed field as compared to conventional one which showed significant increase with depth. In the present study, it was observed that availability of TN decreases consistently with increase in depth from 0 to 20 cm and 20 to 40 cm under all farming modes as earlier reported by Gong et al. (2005), Geissen and Guzman (2006), and Selassie and Ayanna (2013). Contradictory, Emiru and Gebrekidan (2013) reported that continuous organic cultivation can increase oxidation rate of organic matter which can result in reduced nitrogen availability to the crops.
TP and AP were found to be highest content under ORF mode at 0–20 cm depth as compared to 20–40 cm soil depth. In the present study, the amount of AP showed decline with increase in the soil depth. Similar results were observed by Selassie and Ayanna (2013) who credited decrease in P available forms with increasing soil depth to reduction in soil organic matter content in lower soil profile. Generally, P availability increases in organically managed soils due to increase in organic compounds which led to formation of organophosphate complex that can be easily assimilated by plants and also organic matter in soil can promote maximum dissolution of inorganic phosphorus by reducing its fixation and improving its availability in the soil (Selassie and Ayanna, 2013). It can be observed in the present study that AP at the surface soil (0–20 cm) was higher than that in sub soils (20–40 cm), similar results were reported earlier by many studies (Eshetu et al., 2004; Tuma, 2007). The long-term application of phosphate fertilizers and organic manures in soils revealed increased availability of P to the crop plants through decomposition of organic matter and release of certain acids helpful in calcium phosphates solubility (Mohammadi et al., 2009).
Soil microbial abundance and diversity was studied by obtaining biomarkers for different microbes through PLFA technique. In the present study, the most abundant PLFA biomarkers were found to be 16:0, 19:0 cyclo w8c etc. which indicated presence of gram-positive bacteria, gram-negative bacteria, Actinomycetes etc. Soil total PLFA content indicated highest microbial diversity under ORF. Durrer et al. (2021) reported that the presence of N-input as compost poultry and green manure may resulted in changes in bacterial community structure which can lead to improved nutrient cycling of phosphorus and carbon. Similarly, Neha et al. (2022) reported ORF as an effective farming technique which can increase total PLFA soil content and improve soil microbial biomass. Similar results were observed in some previous study of Zhong et al. (2010) who studied the long term effects of organic and inorganic fertilizers on PLFA profiles and microbial functional diversity and reported significant increase in the total PLFA amount of soil microorganisms like bacteria and Actinomycetes under application of both organic and inorganic type of fertilizers while Jingwei (2016) showed that application of organic and bio-fertilizers can improve the total PLFA content of bacteria and fungi present in the soil ecosystem. Contrary to the present study, Zhang et al. (2010) reported reduced relative content of PLFA in Actinomycetes and fungi under ORF mode.
We used DNA high-throughput sequencing of the 16S rRNA to evaluate diversity shifts and to identify the specific bacterial groups associated with soil under organic, conventional and LOF farming systems. Results revealed 97% sequence similarity of data and some effect on structural distribution of microorganisms under different farming modes within different soil depth and sampling time. Use of V3 and V4 regions for 16S rRNA gene sequencing is recommended as these provide accurate and adequate information for taxonomic classification of bacterial communities (Castelino et al., 2017). Many early studies demonstrated the effects of different farming methods on the soil microbial community, which may increase, decrease or remain stable (Reilly et al., 2013; Lupatini et al., 2017). In our study we observed increase in the content of C and N under ORF mode, which can led to increase in the microbial diversity indices, as reported earlier by Hartmann et al. (2015). Out of 30 phyla reported, Proteobacteria was found with high relative abundance followed by Acidobacteria, Bacteroidetes etc. and within 67 classes, Alphaproteobacteria, Gammaproteobacteria, Sphingobacteriia etc. were found dominant at the study area. It was found that 0–20 cm soil depth has significantly higher abundance of microorganisms under LOF mode followed by organic and conventional modes. Shen et al. (2010) also reported significant affect of different fertilization or farming modes on soil microbial abundance and diversity. Similar results were observed in many previous studies of Niu et al. (2017) where Illumina MiSeq high-throughput sequencing technology was used to study microbial community structure of plateu soil and found that the abundance of Proteobacteria (dominant bacterial phylum) was the highest followed by Actinobacteria, Bacteroidetes, and Acidobacteria. Similarly, Megyes et al. (2021) reported significant variations in different bacterial and archaeal community (mostly acidotolerant taxa including Acidobacteria, Solibacter, Bryobacter etc.) under long-term farming practices in maize growing fields using Illumina MiSeq technilogy. Also, Hao et al. (2008) used 16S rRNA pyro-sequencing or Illumina MiSeq to study the effect of different farming modes on the dominant bacterial community and showed high relative abundance of Acidobacteria followed by Proteobacteria, Actinobacteria, Bacteroidetes, and Gemmatimonadetes. Among them, Proteobacteria were more stable phyla in soil as compared to Acidobacteria and found to be unaffected under different soil treatments (Yin et al., 2010), which is consistent with the present study results. Fierer et al. (2007) showed high abundance of Acidobacteria in low organic carbon soil. Lukow et al. (2000) and Nacke et al. (2011) reported dominance of Actinobacteria, Gemmatimonadetes, and Bacteroidetes in agricultural soils while Ivanova et al. (2015) found higher number of Actinobacteria and Bacillus in soils applied with organic matter, which indicated positive effect of organic matter. Wang et al. (2017) used DNA high-throughput sequencing technology to detect the bacterial community structure in soil under different modes and reported increased abundance of oligotrophic bacteria, i.e., Bacteroidetes under application of inorganic fertilizers while organic fertilizer application increased large number of eutrophic bacteria (Proteus).
All the diversity based indices like Chao1, ACE richness and Shannon diversity indices were significantly affected due to different farming practices at the study area. It can be noticed that organic fertilizer application increased the relative abundance and diversity of soil microorganisms while combination of both organic and inorganic fertilizers had more significant effect on the improvement of soil microbial abundance and diversity. The results of Chao1 and ACE revealed that soil treatment with inorganic fertilizers can significantly reduce soil bacterial richness than that with the no soil treatment. Similarly, Liu et al. (2022) reported increase in Shannon diversity index and richness index of the microbial community of soil under application of biological compound fertilizers for 25 years. Kirchmann et al. (2013) found that the application of inorganic and organic fertilizers had no significant effect on the bacterial and fungal Shannon diversity index as compared to the control soil as reported in the present study, which can be attributed to adaptation of soil microorganisms to different farming regimes.
Pearson’s correlation analysis revealed significant positive association among all the soil parameters studied and similar significant positive association within soil physico-chemical parameters was observed by Gebreselassie (2002) in Adet Research Center soils of North-Western Ethiopia. Different microbial types and soil AP, TOC, total available nitrogen, and soil water content were found to have the most significant correlation between them. Soil with higher C and N contents can favor diverse microbial population which ultimately regulates biogeochemical cycles (Chen Q. et al., 2020; Han et al., 2021). Similar to the present study, Ma et al. (2010) revealed that soil organic matter, nitrogen and AP were the main nutrient sources for micro-organisms growth while Zhong et al. (2010) revealed the role of TN, AP and soil pH which can significantly affect PLFA profiles and microorganisms functional diversity. At the phylum level, the correlation between different microbial groups and soil physicochemical properties was observed to be significantly different where Proteobacteria, Bacteroidetes, and Latescibacteria showed significant correlations with most of the physicochemical properties of the soil. Proteobacteria and Bacteroidetes include many bacteria that can fix nitrogen (Farag et al., 2017) and Latescibacteria have a small abundance in soil, but can help in uptake and conversion of sugars, fatty acids and amino acids as carbon sources. The microbes under ORF mode undergo oxidation-reduction reaction and help in degradation of organic matter (He et al., 2017). The correlation between these phyla and soil carbon, nitrogen and phosphorus was reported significant (Jones et al., 2009). Similar to the present study, Sun et al. (2015) showed that bacterial abundance was positively correlated with soil nutrient status such as nitrate, TN, TC, and AP, however, bacterial diversity was mainly determined by soil pH. Also, Niu et al. (2017) showed that soil water content, pH, AN, TN and organic carbon had a greater impact on the composition of microbial communities. Most of the soil parameters are highly correlated with the changes in the microbial population of the area, similar to report of Chen X. et al. (2020). Contrary, a study based on large-scale analysis has shown that the diversity of soil bacteria and fungi mainly depends on soil pH or spatial distance (Green et al., 2004) while Acidobacteria showed negative correlation with soil pH (Dong et al., 2014). Our study positively confirmed that ORF can result in high nutrient availability to the soil fauna and flora, promote changes in microbial bacterial community and their functional aspects.
Conclusion
The results of the present study revealed soil depth and farming modes as key impacting factors on different soil parameters and microbial population. Manure and organic fertilizer application promoted significant soil fertility and improved nutrient status of soil by increasing nutrients like carbon, nitrogen, and phosphorus availability to plants.
The PCA analysis showed highly significant correlation between most of the bacterial types (G + bacteria, G− bacteria, actinomycetes) and soil AP, total available nitrogen, TOC and soil WC. Most of the soil properties revealed a significant relationship between microbial phylum and microbial classes.
Under long-term cultivation of organic vegetables at the studied area showed increase in soil organic nitrogen which may possibly act as a viable strategy for better vegetable production in the Northern China (study area). In conclusion, use of ecological friendly farming practices and application of more organic based fertilizers by the farmers should be encouraged by the Government. In future, more NGS based technologies should be explored in characterizing the microbial communities and suitable and environment friendly agricultural management practice should be used.
Data availability statement
The datasets presented in this study can be found in online repositories. The name of the repository and accession number can be found below: NCBI; PRJNA883513.
Author contributions
MK: writing—original draft, writing—review and editing, formal analysis, and data curation. JL and H-FY: conceptualization and visualization. PZ: investigation, formal analysis, and data curation. MX: conceptualization, visualization, and funding acquisition. LW: writing—original draft, conceptualization, investigation, formal analysis, visualization, data curation, and funding acquisition. All authors contributed to the article and approved the submitted version.
Funding
This research was funded by the National Natural Science Foundation of China (32071552).
Acknowledgments
We would like to acknowledge Experimental Station of Quzhou, China Agricultural University and College of Geography and Environmental Sciences (Henan University) for providing all help in completing this study. MK would also like to thank Dr. Parvinder Pal Singh for insights into data analysis and data interpretation.
Conflict of interest
The authors declare that the research was conducted in the absence of any commercial or financial relationships that could be construed as a potential conflict of interest.
Publisher’s note
All claims expressed in this article are solely those of the authors and do not necessarily represent those of their affiliated organizations, or those of the publisher, the editors and the reviewers. Any product that may be evaluated in this article, or claim that may be made by its manufacturer, is not guaranteed or endorsed by the publisher.
Supplementary material
The Supplementary Material for this article can be found online at: https://www.frontiersin.org/articles/10.3389/fevo.2022.1026771/full#supplementary-material
References
Assen, M., le Roux, A. L., Barker, C. H., and Heluf, G. (2005). Soils of jelo micro-catchment in the chercher highlands of eastern ethiopia: I. Morphological and physiochemical properties. Ethopian J. Nat. Res. 7, 55–81.
Balser, T. C., Liang, C., and Gutknecht, J. L. M. (2019). Linking microbial community analysis and ecosystem studies: A rapid lipid analysis protocol for high throughput. Soil Ecol. Lett. 1, 22–32. doi: 10.1007/s42832-019-0003-0
Baolan, H., Shuai, L., Wei, W., Lidong, S., Liping, L., Weiping, L., et al. (2014). pH dominated niche segregation of ammonia-oxidising microorganisms in Chinese agricultural soils. FEMS Microbiol. Ecol. 90, 290–299. doi: 10.1111/1574-6941.12391
Bligh, E. G., and Dyer, W. J. (1959). A rapid method of total lipid extraction and purification. Can. J. Biochem. Physiol. 37, 911–917. doi: 10.1139/o59-099
Bowman, R. A. (1988). The rapid method to determine total phosphorus in soils. Soil Sci. Soc. Am. J. 52, 1301–1304. doi: 10.2136/sssaj1988.03615995005200050016x
Brinton, W. F. (2020). Phospholipid fatty acid (PLFA) analysis: A robust indicator for soil health? Agri. Res. Tech. 24, 118–119. doi: 10.19080/ARTOAJ.2020.24.556281
Bunemann, E. K., Bongiorno, G. Z. G., Creamerb, R. E., Deynb, G. D., Goedeb, R. D., Fleskensd, L., et al. (2018). Soil quality – a critical review. Soil Biol. Biochem. 120, 105–125.
Butterly, C. R., Kaudal, B., Baldock, J. A., and Tang, C. (2011). Contribution of soluble and insoluble fractions of agricultural residues to short-term pH changes. Eur. J. Soil Sci. 62, 718–727. doi: 10.1111/j.1365-2389-2011-01387.x
Calvino, P., and Monzon, J. (2009). “Farming systems of Argentina: Yield constraints and risk management,” in Crop physiology, applications for genetic improvement and agronomy, eds V. O. Sadras and D. Calderini (Cambridge, MA: Academic Press), 55–70.
Carvalho, A. M. D., Bustamante, M. M. D. C., Almondes, Z. A. D. P., and Figueiredo, C. C. D. (2014). Forms of phosphorus in an oxisol under different soil tillage systems and cover plants in rotation with maize. Rev. Bras. Ciênc. Solo 38, 972–979. doi: 10.1590/S0100-06832014000300029
Castelino, M., Eyre, S., Moat, J., Fox, G., Martin, P., Hp, P., et al. (2017). Optimization of methods for bacterial skin microbiome investigation: Primer selection and comparison of the 454 versus MiSeq platform. BMC Microbiol. 17:23. doi: 10.1186/s12866-017-0927-4
Chen, M., Li, X., Yang, Q., Chi, X., Pan, L., Chen, N., et al. (2012). Soil eukaryotic microorganism succession as affected by continuous cropping of peanut pathogenic and beneficial fungi were selected. PLoS One 7:e40659. doi: 10.1371/journal.pone.0040659
Chen, Q., Ding, J., Zhu, D., Hu, H. W., Delgado-Baquerizo, M., Ma, Y. B., et al. (2020). Rare microbial taxa as the major drivers of ecosystem multifunctionality in long-term fertilized soils. Soil Biol. Biochem. 141:107686.
Chen, X., Henriksen, T. M., Svensson, K., and Korsaeth, A. (2020). Long-term effects of agricultural production systems on structure and function of the soil microbial community. Appl. Soil Ecol. 147:103387.
Clark, M. S., Horwath, W. R., Shennan, C., and Scow, K. M. (1998). Changes in soil chemical properties resulting from organic and low-input farming practices. Agron. J. 90, 662–671. doi: 10.2134/agronj1998.00021962009000050016x
Dong, W. Y., Zhang, X. Y., Dai, X. Q., Fu, X. L., Yang, F. T., Liu, X. Y., et al. (2014). Changes in soil microbial community composition in response to fertilization of paddy soils in subtropical China. Appl. Soil Ecol. 84, 140–147. doi: 10.1016/j.apsoil.2014.06.007
Drinkwater, L. E., Letourneau, D. K., Workneh, F., van Bruggen, A. H. C., and Shennan, C. (1995). Fundamental differences between conventional and organic tomato agroecosystems in California. Ecol. Appl. 5, 1098–1112. doi: 10.2307/2269357
Durrer, A., Gumiere, T., Rumenos, M., Zagatto, G., Feiler, H. P., Silva, A. M. M., et al. (2021). Organic farming practices change the soil bacteria community, improving soil quality and maize crop yield. PeerJ 9:e11985. doi: 10.7717/peerj.11985
Emiru, N., and Gebrekidan, H. (2013). Effect of land use changes and soil depth on soil organic matter, total nitrogen and available phosphorus contents of soils in Senbat Watershed, Western Ethiopia. Am. J. Agric. Biol. Sci. 8, 206–212.
Eshetu, Z., Giesler, R., and Högberg, P. (2004). Historical land use pattern affects the chemistry of forest soils in the Ethiopian highlands. Geoderma 118, 149–165.
Farag, I. F., Youssef, N. H., and Elshahed, M. S. (2017). Global distribution patterns and pangenomic diversity of the candidate phylum “Latescibacteria” (WS3). Appl. Environ. Microbiol. 83:e00521-17. doi: 10.1128/AEM.00521-17
Fierer, N., Bradford, M. A., and Jackson, R. B. (2007). Toward an ecological classification of soil bacteria. Ecology 88, 1354–1364. doi: 10.1890/05-1839
Gajda, A. M., Czyz, E. A., and Ukalska-Jaruga, A. (2020). Comparison of the effects of different crop production systems on soil physico-chemical properties and microbial activity under winter wheat. Agronomy 10:1130. doi: 10.3390/agronomy10081130
Gannett, M., Pritts, M. P., and Lehmann, J. (2019). Soil amendments affect soil health indicators and crop yield in perennial strawberry. HortTechnology 29, 179–188. doi: 10.21273/HORTTECH03959-18
Gebreselassie, Y. (2002). Selected chemical and physical characteristics of soils of Adet Research Center and its testing sites in north-western Ethiopia. Ethiopian J. Nat. Res. 4, 199–215.
Geissen, V., and Guzman, G. M. (2006). Fertility of tropical soils under different land use systems-a case study of soils in Tabasco, Mexico. Appl. Soil Ecol. 31, 169–178. doi: 10.1016/j.apsoil.2005.02.012
Gong, J. L., Chen, N. F., Huang, Y., Huang, Z., and Peng, H. (2005). Effect of land use on soil nutrients in the Loess hilly area of the Loess Plateau, China. Land Degrad. Dev. 17, 453–465. doi: 10.1002/ldr.701
Green, J. L., Holmes, A. J., Westoby, M., Oliver, I., Briscoe, D., Dangerfield, M., et al. (2004). Spatial scaling of microbial eukaryote diversity. Nature 432, 747–750. doi: 10.1038/nature03034
Griesheim, K. L., and Mulvaney, R. L. (2019). Improving the accuracy of diffusion for inorganic 15n analyses of soil extracts and water. Commun. Soil Sci. Plant Anal. 50, 1161–1169. doi: 10.1080/00103624.2019.1604734
Han, S., Delgado-Baquerizo, M., Luo, X. S., Liu, Y. R., Nostrand, J. D. V., Chen, W. L., et al. (2021). Soil aggregate size-dependent relationships between microbial functional diversity and multifunctionality. Soil Biol. Biochem. 154:108143.
Hao, D. C., Ge, G. B., and Yang, L. (2008). Bacterial diversity of Taxus rhizosphere: Culture-independent and culture-dependent approaches. FEMS Microbiol. Lett. 284, 204–212. doi: 10.1111/j.1574-6968.2008.01201.x
Hartmann, M., Frey, B., Mayer, J., Mader, P., and Widmer, F. (2015). Distinct soil microbial diversity under long-term organic and conventional farming. ISME J. 9, 1177–1194.
Hathaway-Jenkins, L. J., Sakrabani, R., Pearce, B., Whitmore, A. P., and Godwin, R. J. (2011). A comparison of soil and water properties in organic and conventional farming systems in England. Soil Use Manag. 27, 133–142. doi: 10.1111/j.1475-2743.2011.00335.x
He, X. L., Song, C., Zhang, L. P., Wang, N., Cao, L. H., and Wei, D. S. (2017). Effects of landfill leachate on microbial diversity in contaminated soil. J. Tianjin Polytechnic Univ. 36, 36–40. doi: 10.3969/j.issn.1671-024x.2017.01.007
Hemkemeyer, M., Dohrmann, A. B., Christensen, B. T., and Tebbe, C. C. (2018). Bacterial preferences for specific soil [article size fractions revealed by community analyses. Front. Microbiol. 9:149. doi: 10.3389/fmicb.2018.00149
Hietala, R., Liu, P., and Qi, S. (2021). Does small-scale organic farming contributes to the local environment—A case study in suburban Shanghai, China. Agronomy 11:1601. doi: 10.3390/agronomy11081601
Hou, Z., Li, P., Li, B., Gong, J., and Wang, Y. (2007). Effects of fertilization scheme on N uptake and N use efficiency in cotton. Plant Soil 290, 115–126. doi: 10.1007/s11104-006-9140-1
Huang, M., Zhou, X., Cao, F., and Zou, Y. (2016). Long-term effect of no-tillage on soil organic carbon and nitrogen in an irrigated rice-based cropping system. Paddy Water Environ. 14, 367–371. doi: 10.1007/s10333-015-0506-y
Ivanova, E. A., Kutovaya, O. V., Tkhakakhova, A. K., Chernov, T. I., Pershina, E. V., Markina, L. G., et al. (2015). The structure of microbial community in aggregates of a typical Chernozem aggregates under contrasting variants of its agricultural use. Eurasian Soil Sci. 48, 1242–1256. doi: 10.1134/S1064229315110083
Jing, X., Sanders, N. J., Shi, Y., Chu, H. Y., Classen, A. T., Zhao, K., et al. (2015). The links between ecosystem multifunctionality and above- and belowground biodiversity are mediated by climate. Nat. Commun. 6:8159. doi: 10.1038/ncomms9159
Jingwei, W. (2016). Different effects of fertilization treatment on microbial phospholipid fatty acids in reclaimed soil. Ph.D. thesis. Jinzhong: Shanxi Agricultural University.
Jones, R. T., Robeson, M. S., Lauber, C. L., Hamady, M., Knight, R., Fierer, N., et al. (2009). A comprehensive survey of soil acidobacterial diversity using pyrosequencing and clone library analyses. ISME J. 3, 442–453. doi: 10.1038/ismej.2008.127
Kandler, O., and Konig, H. (1998). Cell wall polymers in Archaea (Archaebacteria). Cell. Mol. Life Sci. 54, 305–308. doi: 10.1007/s000180050156
Kirchmann, H., Schan, M., Barjesson, G., Hamnér, K., and Kätterer, T. (2013). Properties of soils in the Swedish long-term fertility experiments: VII. Changes in topsoil and upper subsoil at Orja and Fors after 50 years of nitrogen fertilization and manure application. Acta Agric. Scand. B Soil Plant Sci. 63, 25–36. doi: 10.1080/09064710.2012.711352
Kowalska, A., Pawlewicz, A., Dusza, M., Jaskulak, M., and Grobelak, A. (2020). “Plant–soil interactions in soil organic carbon sequestration as a restoration tool,” in Climate change and soil interactions, eds M. N. V. Majeti Prasad and M. Pietrzykowski (Amsterdam: Elsevier), 663–688. doi: 10.1016/B978-0-12-818032-7-00023-0
Kwiatkowski, C. A., Haliniarz, M., and Harasim, E. (2020). Weed infestation and health of organically grown Chamomile (Chamomilla recutita (L.) Rausch.) depending on selected foliar sprays and row spacing. Agriculture 10:168. doi: 10.3390/agriculture10050168
Kwiatkowski, C. A., and Harasim, E. (2020). Chemical properties of soil in four-field crop rotations under organic and conventional farming systems. Agronomy 10:1045. doi: 10.3390/agronomy10071045
Li, X., Ding, C., Zhang, T., and Wang, X. (2014). Fungal pathogen accumulation at the expense of plant-beneficial fungi as a consequence of consecutive peanut monoculturing. Soil Biol. Biochem. 72, 11–18. doi: 10.1016/j.soilbio.2014.01.019
Li, Y., Cao, Z., Hu, C., Li, J., and Yang, H. (2014). Response of nematodes to agricultural input levels in various reclaimed and unreclaimed habitats. Eur. J. Soil Biol. 60, 120–129. doi: 10.1016/j.ejsobi.2013.12.001
Liu, E., Yan, C., Mei, X., He, W., Bing, S. H., Ding, L., et al. (2010). Long-term effect of chemical fertilizer, straw, and manure on soil chemical and biological properties in northwest China. Geoderma 158, 173–180. doi: 10.1016/j.geoderma.2010.04.029
Liu, X., Shi, Y., Kong, L., Tong, L., Cao, H., Zhou, H., et al. (2022). Long-term application of bio-compost increased soil microbial community diversity and altered its composition and network. Microorganisms 10:462. doi: 10.3390/microorganisms10020462
Lu, L., Yin, S., Liu, X., Zhang, W., Gu, T., Shen, Q., et al. (2013). Fungal networks in yield-invigorating and-debilitating soils induced by prolonged potato monoculture. Soil Biol. Biochem. 65, 186–194. doi: 10.1016/j.soilbio.2013.05.025
Lukow, T., Dunfield, P. F., and Liesack, W. (2000). Use of the T-RFLP technique to assess spatial and temporal changes in the bacterial community structure within an agricultural soil planted with transgenic and non-transgenic potato plants. FEMS Microbiol. Ecol. 32, 241–247. doi: 10.1111/j.1574-6941.2000.tb00717.x
Lupatini, M., Korthals, G. W., de Hollander, M., Janssens, T. K. S., and Kuramae, E. E. (2017). Soil microbiome is more heterogeneous in organic than in conventional farming system. Front. Microbiol. 7:2064. doi: 10.3389/fmicb.2016.02064
Ma, N.-N., Li, T.-L., Wu, C.-C., and Zhang, E.-P. (2010). Effects of long-term fertilization on soil enzyme activities and soil physicochemical properties of facility vegetable field. Chin. J. Appl. Ecol. 21, 1766–1771.
Malo, D. D., Schumacher, T. E., and Doolittle, J. J. (2005). Long-term cultivation impacts on selected soil properties in the Northern Great Plains. Soil Tillage Res. 81, 277–291. doi: 10.1016/j.still.2004.09.015
Mandal, A., Toor, A. S., and Dhaliwal, S. S. (2018). Effect of land-uses on physico-chemical properties and nutrient status of surface (0-15 cm) and sub-surface (15-30 cm) layers in soils of South-Western Punjab, India. Int. J. Curr. Microbiol. Appl. Sci. 7, 2659–2671. doi: 10.20546/ijcmas.2018.706.315
Manna, M. C., Swarup, A., Wanjari, R. H., Ravankar, H. N., Mishra, B., Saha, M. N., et al. (2005). Long-term effect of fertilizer and manure application on soil organic carbon storage, soil quality and yield sustainability under sub-humid and semi-arid tropical India. Field Crop Res. 93, 264–280.
Matheron, G. (1963). Principles of geostatistics. Econ. Geol. 58, 1246–1266. doi: 10.2113/gsecongeo.58.8.1246
Medan, D., Torretta, J. P., Hodara, K., dela Fuente, E. B., and Montaldo, N. H. (2011). Effects of agriculture expansion and intensification on the vertebrate and invertebrate diversity in the Pampas of Argentina. Biodivers. Conserv. 20, 3077–3100. doi: 10.1007/s10531-011-0118-9
Megyes, M., Borsodi, A. K., Arendas, T. M., and Marialigeti, K. (2021). Variations in the diversity of soil bacterial and archaeal communities in response to different long-term fertilization regimes in maize fields. Appl. Soil Ecol. 168:104120. doi: 10.1016/j.apsoil.2021.104120
Mohammadi, S., Kalbasi, M., and Shariatmadri, H. (2009). Cumulative and residual effects of organic fertilizer application on selected soil properties, water soluble P and phosphorus sorption index. J. Agric. Sci. Technol. 11, 487–497.
Morales, L. A., Vazquez, E. V., and Paz-Ferreiro, J. (2011). Spatial and temporal variability of Mehlich-1 extractable Fe, Mn and Zn over a rice field as a function of lime amendment. Stoch. Environ. Res. Risk Assess. 25, 1039–1048. doi: 10.1007/s00477-011-0490-8
Morshedi, L., Lashgarara, F., Hosseini, S. J. F., and Najafabadi, M. O. (2017). The role of organic farming for improving food security from the perspective of Fars farmers. Sustainability 9:2086. doi: 10.3390/su9112086
Nacke, H., Thürmer, A., Wollherr, A., Will, C., Hodac, L., Herold, N., et al. (2011). Pyrosequencing-based assessment of bacterial community structure along different management types in German forest and grassland soils. PLoS One 6:e17000. doi: 10.1371/journal.pone.0017000
Nandan, R., Singh, V., Singh, S. S., Kumar, V., Hazra, K. K., Nath, C. P., et al. (2019). Impact of conservation tillage in rice–based cropping systems on soil aggregation, carbon pools and nutrients. Geoderma 340, 104–114. doi: 10.1016/j.geoderma.2019.01.001
Neha, Bhardwaj, Y., Sharma, M. P., Pandey, J., and Dubey, S. K. (2022). Response of crop types and farming practices on soil microbial biomass and community structure in tropical agroecosystem by lipid biomarkers. J. Soil Sci. Plant Nutr. 22, doi: 10.1007/s42729-022-00758-3
Niu, S. Q., Long, Y., Li, H. Y., Da, W. Y., Hu, S., Li, W. J., et al. (2017). Microbial diversity in saline alkali soil from Hexi Corridor analyzed by Illumina MiSeq high-throughput sequencing system. Microbiol. China 44, 2067–2078. doi: 10.13344/j.microbiol.china.160824
Niyogi, D., Kishtawal, C., Tripathi, S., and Govindaraju, R. S. (2010). Observational evidence that agricultural intensification and land use change may be reducing the Indian summer monsoon rainfall. Water Resour. Res. 46:W03533. doi: 10.1029/2008WR007082
Olsen, S. R., Cole, C. V., Watanable, F. S., and Dean, L. A. (1954). Estimation of available phosphorus in soils by extraction with sodium bicarbonate. Circular, Vol. 939. Washington, DC: US Department of Agriculture.
Ozlu, E., Sandhu, S. S., Kumar, S., and Arriaga, F. J. (2019). Soil health indicators impacted by long-term cattle manure and inorganic fertilizer application in a corn soybean rotation of South Dakota. Sci. Rep. 9:11776. doi: 10.1038/s41598-019.48207-z
Paul, C., Bayrychenko, Z., Junier, T., Filippidou, S., Beck, K., Bueche, M., et al. (2018). Dissemination of antibiotic resistance genes associated with the sporobiota in sediments impacted by wastewater. PeerJ 6:e4989. doi: 10.7717/peerj.4989
Pimentel, D., Hepperly, P., Hanson, J., Douds, D., and Seide, R. (2005). Environmental, energetic, and economic comparisons of organic and conventional farming systems. BioScience 55, 573–582.
Ponisio, L. C., M’Gonigle, L. K., Mace, K. C., Palomino, J., de Valpine, P., and Kremen, C. (2015). Diversification practices reduce organic to conventional yield gap. Proc. R. Soc. B Biol. Sci. 282:20141396. doi: 10.1098/rspb.2014.1396
Reilly, K., Cullen, E., Lola-luz, T., Stone, D., Valverde, J., Gaffney, M., et al. (2013). Effect of organic, conventional and mixed cultivation practices on soil microbial community structure and nematode abundance in a cultivated onion crop. J. Sci. Food Agric. 93, 3700–3709. doi: 10.1002/jsfa.6206
Riedo, J., Wettstein, F. E., Rösch, A., Herzog, C., Banerjee, S., Büchi, L., et al. (2021). Widespread occurrence of pesticides in organically managed agricultural soils—the ghost of a conventional agricultural past? Environ. Sci. Technol. 55, 2919–2928. doi: 10.1021/acs.est.0c06405
Robertson, G. P., Sollins, P., Ellis, B. G., and Laijtha, K. (1999). “Exchangeable ions, pH, and cation exchange capacity,” in Standard soil methods for long term ecological research, eds G. P. Robertson, D. C. Coleman, C. S. Bledsoe, and P. Sollins (New York, NY: Oxford University Press).
Roesch, L. F. W., Fulthorpe, R. R., Riva, A., Casella, G., Hadwin, A. K. M., Kent, A. D., et al. (2007). Pyro-sequencing enumerates and contrasts soil microbial diversity. ISME J. 1, 283–290.
Sankaramoorthy, A., and Rangasamy, V. (2019). Impacts of different sources of organic manures on soil physico-chemical properties, nutrient balance and yield of rice-green gram cropping sequence under organic farming. SSRG Int. J. Agric. Environ. Sci. 6, 65–85. doi: 10.14445/23942568/IJAES-V6I4P112
Selassie, Y. G., and Ayanna, G. (2013). Effects of different land use systems on selected physico-chemical properties of soils in Northwestern Ethiopia. J. Agric. Sci. 5, 112–120. doi: 10.5539/jas.v5n4p112
Seufert, V., and Ramankutty, N. (2017). Many shades of Gray—the context dependent performance of organic agriculture. Sci. Adv. 3:e1602638. doi: 10.1126/sciadv.1602638
Shannon, D., Sen, A. M., and Johnson, D. B. (2002). A comparative study of the microbiology of soils managed under organic and conventional regimes. Soil Use Manag. 18(Suppl. S1), 274–283. doi: 10.1111/j.1475-2743.2002.tb00269.x
Sharma, S., Singh, P., and Sodhi, G. P. S. (2020). Soil organic carbon and biological indicators of uncultivated vis-a-vis intensively cultivated soils under rice-wheat and cotton –wheat cropping systems in South-Western Punjab. Carbon Manage. 11, 681–695. doi: 10.1080/17583004.2020.1840891
Shen, J. P., Zhang, L. M., Guo, J. F., Ray, J. L., and He, J. Z. (2010). Impact of long-term fertilization practices on the abundance and composition of soil bacterial communities in Northeast China. Appl. Soil Ecol. 46, 119–124. doi: 10.1016/j.apsoil.2010.06.015
Shukla, M. K., Lal, R., and Ebinger, M. (2006). Determining soil quality indicators by factor analysis. Soil Tillage Res. 87, 194–204. doi: 10.1016/j.still.2005.03.011
Song, Z.-Q., Cheng, J.-E., Cheng, F.-X., Zhang, D.-Y., and Liu, Y. (2017). Development and evaluation of loop-mediated isothermal amplification assay for rapid detection of Tylenchulus semipenetrans using DNA extracted from soil. Plant Pathol. J. 33, 184–192. doi: 10.5423/PPJ.OA.10.2016.0224
Sun, R., Zhang, X. X., Guo, X., Wang, D., and Chu, H. (2015). Bacterial diversity in soils subjected to long-term chemical fertilization can be more stably maintained with the addition of livestock manure than wheat straw. Soil Biol. Biochem. 88, 9–18. doi: 10.16/soilbio.2015.05.007
Thomas, B. W., Hunt, D., Bittman, S., Hannam, K. D., Messiga, A. J., Haak, D., et al. (2019). Soil health indicators after 21 yr of no-tillage in South Coastal British Columbia. Can. J. Soil Sci. 99, 222–225. doi: 10.1139/cjss-2018-0146
Tuma, A. (2007). Effects of fruit based land use systems on soil physicochemical properties: The case of smallholders farming systems in Gamo Gofa, Southern Ethiopia. M. Sc. thesis. Hawassa: Hawassa University.
Ukalska-Jaruga, A., Klimkowicz-Pawlas, A., and Smreczak, B. (2019). Characterization of organic matter fractions in the top layer of soils under different land uses in Central-Eastern Europe. Soil Use Manag. 35, 595–606. doi: 10.1111/sum.12514
Uroz, S., Ioannidis, P., Lengelle, J., CeÂbron, A., Morin, E., BueÂe, M., et al. (2013). Functional assays and metagenomic analyses reveals differences between the microbial communities inhabiting the soil horizons of a Norway spruce plantation. PLoS One 8:e55929. doi: 10.1371/journal.pone.0055929
Venkatesh, M. S., Hazra, K. K., Ghosh, P. K., Praharaj, C. S., and Kumar, N. (2013). Long–term effect of pulses and nutrient management on soil carbon sequestration in Indo–Gangetic plains of India. Can. J. Soil Sci. 93, 127–136. doi: 10.4141/CJSS2012-072
Wakene, N. (2001). Assessment of important physicochemical properties of Nitosols under different management systems in Bako Area, Western Ethiopia. M. Sc. thesis. Alemaya: Alemaya University.
Wang, J., Song, Y., Ma, T., Raza, W., Li, J., Howland, J. G., et al. (2017). Impacts of inorganic and organic fertilization treatments on bacterial and fungal communities in a paddy soil. Appl. Soil Ecol. 112, 42–50. doi: 10.1016/j.apsoil.2017.01.005
Wang, L., Kaur, M., Zhang, P., Li, J., and Xu, M. (2021). Effect of different agricultural farming practices on microbial biomass and enzyme activities of celery growing field soil. Int. J. Environ. Res. Public Health 18:12862. doi: 10.3390/ijerph182312862
Wang, Y., Tu, C., Cheng, L., Li, C., Gentry, L. F., Hoyt, G. D., et al. (2011). Long-term impact of farming practices on soil organic carbon and nitrogen pools and microbial biomass and activity. Soil Tillage Res. 117, 8–16. doi: 10.1016/j.still.2011.08.002
Willers, C., van Rensburg, P. J., and Claassens, S. (2015). Phospholipid fatty acid profiling of microbial communities–a review of interpretations and recent applications. J. Appl. Microbiol. 119, 1207–1218. doi: 10.1111/jam.12902
Xomphoutheb, T., Jiao, S., Guo, X., Mabagala, F. S., Sui, B., Wang, H., et al. (2020). The effect of tillage systems on phosphorus distribution and forms in rhizosphere and non-rhizosphere soil under maize (Zea mays L.) in Northeast China. Sci. Rep. 10:6574. doi: 10.1038/s41598-020-63567-7
Xu, H., and Zhang, C. (2021). Investigating spatially varying relationships between total organic carbon contents and pH values in European agricultural soil using geographically weighted regression. Sci. Total Environ. 752:141977. doi: 10.1016/j.scitotenv.2020.141977
Yang, T., Kadambot, H. M. S., and Liu, K. (2020). Cropping systems in agriculture and their impact on soil health-A review. Glob. Ecol. Conserv. 23:e01118. doi: 10.1016/j.gecco.2020.e01118
Yin, C., Jones, K. L., Peterson, D. E., Garrett, K. A., Hulbert, S. H., Paulitz, T. C., et al. (2010). Members of soil bacterial communities sensitive to tillage and crop rotation. Soil Biol. Biochem. 42, 2111–2118. doi: 10.1016/j.soilbio.2010.08.006
Zhang, Q. C., Wang, X. Q., Shi, Y. N., and Wang, G. H. (2010). Effects of different fertilizer treatments on ecological characteristics of microorganism in chemical fertilizer omission paddy soil. J. Plant Nutr. Fertil. 16, 118–123.
Zhong, W., Gu, T., Wang, W., Zhang, B., Lin, X., Huang, Q., et al. (2010). The effects of mineral fertilizer and organic manure on soil microbial community and diversity. Plant Soil 326, 511–522. doi: 10.1007/s11104-009-9988-y
Zhou, W., Lv, T.-F., Chen, Y., Westby, A. P., and Ren, W.-J. (2014). Soil physicochemical and biological properties of paddy-upland rotation: A review. Sci. World J. 2014:856352. doi: 10.1155/2014/856352
Zhou, Z., and Wang, C. (2017). Soil-microbe-mineralization carbon and nitrogen stoichiometry under different land-uses in the Maoershan region. Acta Ecol. Sin. 37, 2428–2436.
Zhu, S., Wang, Y., Xu, X., Liu, T., Wu, D., Zheng, X., et al. (2018). Potential use of high-throughput sequencing of soil microbial communities for estimating the adverse effects of continuous cropping on ramie (Boehmeria nivea L. Gaud). PLoS One 13:e0197095. doi: 10.1371/journal.pone.0197095
Keywords: agricultural soil, carbon, nitrogen, phosphorus, ammonium nitrogen, nitrate nitrogen, PLFA, DNA high-throughput sequencing
Citation: Kaur M, Li J, Zhang P, Yang H-f, Wang L and Xu M (2022) Agricultural soil physico-chemical parameters and microbial abundance and diversity under long-run farming practices: A greenhouse study. Front. Ecol. Evol. 10:1026771. doi: 10.3389/fevo.2022.1026771
Received: 24 August 2022; Accepted: 27 September 2022;
Published: 13 October 2022.
Edited by:
Anna Gałązka, Institute of Soil Science and Plant Cultivation, PolandReviewed by:
Artur Banach, The John Paul II Catholic University of Lublin, PolandEwa Maria Furmanczyk, Research Institute of Horticulture, Poland
Copyright © 2022 Kaur, Li, Zhang, Yang, Wang and Xu. This is an open-access article distributed under the terms of the Creative Commons Attribution License (CC BY). The use, distribution or reproduction in other forums is permitted, provided the original author(s) and the copyright owner(s) are credited and that the original publication in this journal is cited, in accordance with accepted academic practice. No use, distribution or reproduction is permitted which does not comply with these terms.
*Correspondence: Lin Wang, d2FuZ2xpbkBoZW51LmVkdS5jbg==; Ming Xu, bWluZ3h1QGhlbnUuZWR1LmNu