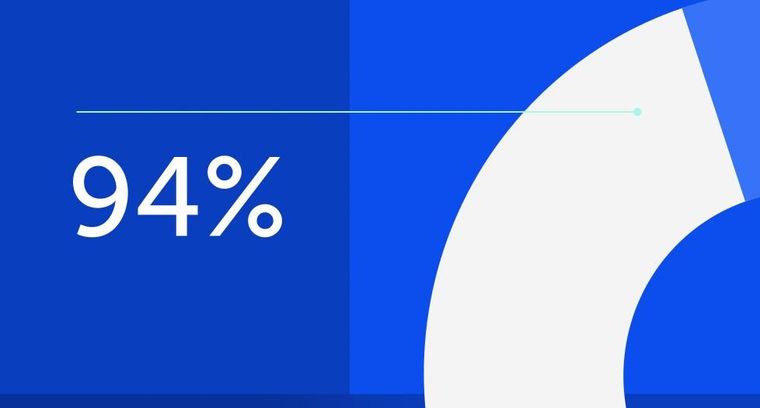
94% of researchers rate our articles as excellent or good
Learn more about the work of our research integrity team to safeguard the quality of each article we publish.
Find out more
ORIGINAL RESEARCH article
Front. Comput. Sci., 06 March 2025
Sec. Computer Security
Volume 7 - 2025 | https://doi.org/10.3389/fcomp.2025.1539519
This article is part of the Research TopicCyber Security Prevention, Defenses Driven by AI, and Mathematical Modelling and Simulation ToolsView all 5 articles
Cybersecurity has become a significant concern in recent decades. Enhancing cybersecurity and safeguarding important information systems are essential in today’s world. It is now one of the most important challenges in the realm of IT. Malware has become a significant issue in the modern digital age. The primary objectives of malware are to disrupt, harm, or impair computer systems and information systems without the user’s consent or awareness. Currently, malwares are viewed as some of the most prevalent cyber threats. The prevalence of Windows operating system has made it a prime target for malware attacks. PE (Portable Executable) is the standard file format for executable files and DLLs on Windows systems, with PE malware being the most common form of malicious software. Static analysis, which is mainly a signature-based method for detecting malware, can only identify already known malware. The main weakness of this approach is its struggle with obfuscation, such as encryption and packing. The use of machine learning methods has demonstrated significant potential in the field of malware detection and is an emerging field with many opportunities. Most previous works focus on binary classification, limited number of ML algorithms and even a single dataset. In this paper, we present both a binary and multiclass PE malware classification using four classic machine learning algorithms and four deep learning algorithms. We have applied this algorithm on three publicly available datasets and deduced the best algorithm depending on the number of features and dataset size.
Protecting computer systems and information systems from malware has become one of the most challenging and complex tasks in cyber security. With the proliferation of the Internet, the impact of malware has become dangerous and cannot be ignored. Attackers use malware to compromise computers, steal confidential information and can cause inflict substantial financial harm. The Windows operating system is the most popular operating system globally, making it a prime target for malicious software. AV-TEST Institute reported that by the end of May 2024, there were 49,582,654 newly discovered malwares, with over 85% of them designed to attack the Windows operating system as opposed to Android, Linux, or MacOS. Moreover, almost 90% of these malwares were specifically targeting executable files instead of archive, data, HTML, or other file formats (AV-TEST, 2024). The file format used for executables, DLLs, object code, and other files in the Windows operating system is known as the portable executable (PE) format. This format is used in both 32-bit and 64-bit versions of Windows. The PE file format serves as a data structure that provides the necessary information for the Windows OS loader to handle the enclosed executable code. A PE file infector is a type of malware that spreads by adding or enclosing malicious code within other PE files on a compromised system. Malware detection techniques are mainly categorized into two types: signature-based (static) and behavior-based (dynamic) methods. Signature-based detection involves comparing code signatures against a database of known malicious signatures without executing the code, providing efficient detection of known malware. However, this approach is limited in detecting zero-day malware and polymorphic malwares, as well as facing issues with the exponential increase in the size of the signatures database affecting matching time. On the other hand, dynamic malware analysis requires executing malware code in a controlled environment to observe its system interactions. Despite being computationally expensive, dynamic analysis does not cover all possible execution paths. In recent years, there has been a significant focus on utilizing machine learning for detecting malware, particularly the supervised learning technique. Machine learning algorithms leverage the efficiency and speed of static malware analysis, requiring fewer computational resources than dynamic analysis. These algorithms are able to differentiate between malware and legitimate files by analyzing the properties of PE headers.
Several ML-based methods for detecting malware have been introduced, but some of them only utilized binary classification (malware or benign). These methods typically employed either classic ML algorithms or deep learning algorithms, but rarely compared the outcomes of both. Furthermore, they did not test the algorithms across various datasets. In this paper, we present a machine learning-based method for detecting malware in PE files. We utilized binary and multiclass classification, employing a variety of classic machine learning algorithms such as random forest, SVM, ANN, and Naive Bayes, as well as deep learning algorithms like CNN, RNN, LSTM, and CNN-LSTM. Our approach involved testing these algorithms on multiple publicly available datasets with different characteristics related to the number of features, dimensionality and to evaluate the outcomes.
The rest of this paper is structured in the following manner: a review of previous work in section two. Section three provides an overview of the PE file format. Sections four and five explain the different stages of the ML-based malware detection process used for both binary and multiclass classification. Section six present a discussion about the obtained results and in the final section seven, we present our conclusion and future work.
The use of machine learning techniques for PE malware detection is not a new concept as several approaches and methods have been proposed in the literature. Merabet and Hajraoui (2019) examined and reviewed several methods for feature extraction and selection used in previous machine learning based malware detectors and evaluated classification methods used. They were interested in the accuracy rate of each one of the previous classifiers. They concluded that a deep learning approach could lead to a smart anti-malware program. Yanfang et al. (2017) provided a complete survey on malware detection techniques using machine learning. They mentioned that the performance of a malware detection system depends greatly on the extracted features and the methods for classification/clustering. For the task of feature extraction, they suggested using static analysis as first step, since over 80% of the file sample collection can be well-represented by static features. Then use dynamic analysis. In addition, they discussed issues and challenges of malware detection using machine learning techniques and forecast the trends of malware development.
Raff et al. (2017) applied two different types of neural networks for malware detection and feature learning. They restricted to the minimum domain knowledge for the extraction a portion of the PE header without explicit feature construction. An automatic feature extraction was done based using n-grams features vector which used an Elastic-Net regularized Logistic Regression classifier. They claimed that their model was able to match and even surpass the performance of a domain knowledge approach. Kumar et al. (2017) proposed a static analysis technique for the detection of malicious PE file. Their approach consists of using an integrated feature set created from raw and derived features, based on different header fields’ values of PE file. They claimed that their method could improve the classification accuracy of machine learning classifiers. They used various machine learning algorithms and provided a performance comparison of each classifier. Gibert et al. (2020) presented an interesting review of the most recent machine learning techniques used in malware classification. Their paper provided a basic background in malware analysis with a brief description of the process and tools used in malware detection and classification.
Radwan (2019) used a static analysis method for malware detection by deriving some new features to increase the accuracy and improve the robustness of their classifier. He has used a dataset containing 5,184 samples with 2,683 malware and 2,501 benign to test his classifier. He applied seven machine learning algorithms for the classification and found that the performance of Random Forest is the highest among them. Varma and Narasimharao (2022) used a dataset of 138,047 sample of malware and benign files each has 57 PE attributes where they selected 13 most significant features. They used six different classic Machine learning algorithms and come up with a classification accuracy of 99.4% as they claimed in their paper. Rakesh Kumar (2022) selected a dataset containing 138,047 samples of PE files of which 27,610 were classified as benign and 110,432 as malicious. He used four classic machine learning algorithms namely AdaBoost, Random Forest, Gradient Boosting and Ensemble Algorithm and two deep learning algorithms: CNN and a combination of CNN and LSTM algorithms. He found that gradient boosting performed the best of classic ML and that CNN + LSTM gave better results than using just CNN. Yousuf et al. (2023) proposed a static Portable Executable (PE) malware analysis system based on seven classic machine learning models, three ensemble learning techniques and two dimensionality reduction techniques. They used a dataset of 27,920 samples divided into six categories and 1878 benign files. They concluded that combining the PE Header and the PE Section provides the highest accuracy and lowest error rate.
Azmee et al. (2020) compared accuracy of nine classic machine learning classifiers for the detection of PE malware files and showed that XG- Boost achieved the highest accuracy. They used a dataset of 19,611 sample collected from Microsoft Kaggle where ach sample has 79 features. They also applied the PCA (Principal Component Analysis) dimension reduction technique and used standard scalar for dataset standardization. Connors and Sarkar (2022) used the well-known large dataset EMBER [The Elastic Malware Benchmark for Empowering Researchers dataset (AV-TEST, 2024)] to evaluate features of PE files by applying common classic machine learning techniques for malware detection. They concluded that ANN-based classifier outperformed other used classifiers. Jhansi Priya et al. (2024) combined CNN and LSTM networks with YARA rule-based for PE malware detection. They used the windows malware dataset containing PE Header of malware samples having different features and a second dataset of grayscale images of only malware PE files (25 families of malware images) and a benign dataset consisting of 3,000 benign images. Sumit and Amol (2022) proposed a static analysis of malware by extracting the features from the PE files using a deep learning algorithm. They emphasized the importance of feature extraction and reducing the feature space and applied their approach to the EMBER dataset Hyrum and Phil (2018) with nearly 600,000 samples of PE files. They claimed that their model achieved 98.85% accuracy.
Ayofe et al. (2021) proposed an ensemble learning-based framework fully connected dense ANN and 1-D CNN as first-stage classifiers and employed five classic machine learning algorithms as end-stage classification. They also used principal component analysis (PCA) for dimensionality reduction. They used a publicly available dataset containing malicious and benign program data from Windows Portable Executable (PE) files having 19,611 samples each has 77 features. They found that ExtraTrees classifier achieved the best accuracy when used as a final stage. Manavi and Hamzeh (2021) used the long short-term memory (LSTM) network to detect one type of malware, namely ransomware by analyzing the header of executable files and they achieved a detection accuracy of 93.25%. Al-Khshali et al. (2024) proposed the use of adapting subspace learning-based One-Class Classification (OCC) methods for detecting malware namely One-Class Support Vector Machine (OCSVM) and Support Vector Data Description (SVDD). They employed three publicly available datasets from Kaggle where the first contains 19,611 sample each with 77 features, the second contains 5,910 samples each with 69 samples and the last contains 43,293 samples each with 4 features. They come up with a True Positive Rate (TPR) of 100%.
Louk and Bayu (2022) compared and evaluated the performance of various tree-based classifiers in detecting PE malware. They used four tree-based ensemble techniques namely XGBoost, CatBoost, GBM, and LightGBM and trained their models on three different datasets. They concluded that their approach outperforms existing ML-based PE malware detectors. Ayofe et al. (2021) proposed an ensemble learning-based method for PE malware detection where the first-stage classifiers are composed of neural networks and examined 15 machine learning models as a final-stage classifier. They used five different machine learning algorithms as base models for comparison. The publicly available data set used was composed of 19,611 samples each with 77 features. They also applied principal component analysis (PCA) for dimensionality reduction. They found that fully connected dense ANN and 1-D CNN models with ExtraTrees as a final-stage classifier achieved the best accuracy.
In summary, previous works did not use a variety of data sets with different number of features and different dimensionalities. They used either classic machine learning algorithms or deep learning algorithms but not both to investigate which approach gave better results. Most previous works used one kind of classic ML algorithms or deep learning algorithms. In addition, the malware classification was restricted to a binary classification or limited to one type of malwares.
PE (Portable Executable) file format is a data structure used in 32-bits and 64-bits Windows operating systems that encapsulates the information needed by the Windows OS loader to be able to load that executable into memory and execute it. It is a file format for basically executables (.exe) and dynamic link library (.dll). This includes dynamic library references for linking, API export, import tables, resource management data, and thread-local storage (TLS) data.
The basic structure of PE file contains two main parts: the header and the sections as shown in Figure 1. The header part includes the following sections: (1) Dos header and Dos stub for compatibility purpose only, the former checks whether the file is valid PE file or not and the letter prints an error message saying that the program cannot be run on windows. (2) The PE header section which contains information about the file and made-up of three main components: the signature which identifies the file as a PE file, the file header which contains relevant information about the file and the optional section which provides important information to the OS loader. The last part of the header is the section table which contains information about the section it refers to. The second part of a PE file is the sections part which contains several sections, each one with its own purpose. These sections are where the actual contents of the file are stored. Among these sections we can find.text,.data,.rdata,.pdate,.rsrc,.reloc etc. Analyzing and studying features of PE header files leads to better comprehension of the file type and helps a lot in differentiating benign files from malware ones.
For binary classification, we used two publicly available datasets that have different sizes and different number of features to check the accuracy of our model on different datasets. Table 1 shows the characteristics of each dataset.
We followed the steps included in a typical machine learning project. Figure 2 depicts the stages involved in our malware detection framework.
The first step of the cycle consists of data preprocessing to ensure the dataset is balanced, we need to make sure that the number of samples in each class (benign and malware) is approximately equal. This is important for training machine learning models. Then, we need to drop the useless features we do not such as the hash, name, etc. For the feature selection step, we need to calculate the importance of each feature and select the most important features and for this end we have used the tree-base feature selection algorithm. We then split the dataset into training (80%) and testing (20%) sets. The training set will be used to train the model, while the testing set will assess its performance on unseen data. This is an essential step in machine learning, as it helps us evaluate the performance of our model. During the next step, we have chosen eight machine learning algorithms for the file classification among them four classic machine learning algorithms namely SVM, Naive Bayes, Random Forest and Neural network and four deep learning algorithms namely CNN, RNN, LSTM and CNN + LSTM.
Finally, we evaluated our model using most known metrics such as:
• Accuracy = (TP + TN)/(TP + TN + FP + FN)
• Precision = TP/(TP + FP)
• Recall = TP/(TP + FN)
• F1-Score = 2*(precision*recall)/(precision + recall)
Where.
TP: True positive, TN: True negative, FP: False positive and FN: False negative.
In addition, we used the confusion matrix to visualize and summarizes the performance of each classification algorithm.
Table 2 summarizes the evaluation of malware detection models using both classic machine learning algorithms and deep learning algorithms on the two datasets and Figure 3 shows the accuracy of each algorithm on dataset 1, dataset 2 and dataset 3. Figure 4 shows the confusion matrices of the best classic machine learning algorithm (Random Forest) and Figure 5 shows the confusion matrices of the best deep learning algorithm (LSTM).
For multiclass classification, we used one publicly available dataset containing 6 types of malwares in addition to benign ones, each has 54 features. The total size of the dataset is 29,807 samples. Table 3 shows the characteristics of the dataset.
We followed the same steps as the classic machine learning approach except the phase of feature selection which is omitted because deep learning algorithms achieve such task automatically.
Table 4 summarizes the evaluation of malware detection models using both classic machine learning algorithms and deep learning algorithms on the selected dataset and Figure 6 shows the accuracy of each algorithm on dataset 3. Figure 7 shows the confusion matrices of the best classic machine learning algorithm (Random Forest) and Figure 8 shows the confusion matrices of the best deep learning algorithms (CNN + LSTM).
The aim of this work is the classification of windows PE files into malwares or benign ones using machine learning techniques. In addition to the variety of algorithms used for the classification, we tested our approach on three publicly available datasets having different characteristics. The two datasets used in binary classification have different number of features (high, low) and different size (high, low) as shown in Figure 9. The dataset used for testing the multiclass classification has six classes of malwares and one benign as in Figure 10.
In the case of binary classification, the number of features is very important in the classification process and the higher dimensionality introduces more noise and complexity, making it harder for the model to discern relevant patterns. Random forest gave the best results among classic machine learning algorithms. In contrast, the high dimensionality of the dataset has a greater impact on the classification accuracy compared to the number of features in case of deep learning. The best results were obtained using CNN and CNN-LSTM algorithms. Both types of ML techniques achieve good results in the case of binary classification, but the deep learning algorithms perform better in general.
In the case of multiclass classification, having fewer classes generally simplifies the classification task, as the model needs to differentiate between fewer categories. When using classic machine learning algorithms, the best results were obtained using either random forest or ANN while the Naive Bayes and SVM classifiers achieved the worst results. All the deep learning algorithms perform good with almost similar results however the CNN-LSTM algorithm provided the best classification accuracy. None of the two types of ML algorithms was able to achieve more than 90% of accuracy.
Dataset 1 was used by Varma and Narasimharao (2022) for detection of malware using seven supervised ML algorithms (Decision Trees, Random Forest, GradientBoosting, AdaBoost, Gaussian Naive Bayes, Linear Regression) where two of them are present in our work: random forest with achieved accuracy 0.99 same as in our work and Gaussian Naive Bayes with achieved accuracy 0.7 (in our work it is 0.5 after data balancing and selection of important features). It was also used in Siregar et al. (2023) with just one algorithm namely Back Propagation Neural Network (BPNN) with Hyperparameter Variations not really similar to our work. The best configuration achieved 0.98 of accuracy. Another use of the dataset 1 use was by Del Aguila et al. (2024) where authors used it on three supervised ML algorithms: artificial neural networks (ANN), support vector machines (SVMs), and gradient boosting machines (GBMs) where two of them are present in our work: ANN with achieved accuracy 0.94 (in our work it is 0.95 after data balancing and selection of important features) and SVM with achieved accuracy 0.91 (in our work it is 0.49 after data balancing and selection of important features).
Dataset 2 was used previously in Alqahtani et al. (2023) where authors made use of 1D-CNN with different hyperparameters, and authors achieved almost the same results as our work. Another use of this dataset can be found in Paza et al. (2022) where the dataset was used mainly with the Gradient Boosting algorithm.
Dataset 3 was used in Yousuf et al. (2023) with seven classic machine learning models: gradient boosting, decision tree, random forest, support vector machine, K-nearest neighbor, naive Bayes, and nearest centroid, and three ensemble learning techniques including Majority Voting, Stack Generalization, and AdaBoost to classify the malware. Three of these algorithms were used in our work and achieved better results than ours. This dataset was also used in Baghirov (2024) where seven ML models are evaluated on both binary and multiclass classification tasks before and after applying Principal Component Analysis (PCA). They achieved better results for binary classification and lower results for multiclass classification.
Due to the increase in the types of malwares and the number of malicious activities, the demand and need for effective malware detectors to protect against zero-day attacks has increased. Our main goal was to develop and analyze a machine learning system that can detect as many malwares as possible using binary and multiclass classification. We applied both classic machine learning and deep learning algorithms to different publicly available datasets. In the case of binary classification, both machine learning techniques performed good and the best results were obtained when using random forest, CNN and CNN-LSTM. In the case of multiclass classification, the deep learning algorithms performed generally better than classic machine learning algorithms however with a good configuration of classic machine learning hyper-parameters, these algorithms can achieve better results than deep learning. A final word would be to encourage the use of classic machine learning algorithms when detecting windows PE malware files and there is no raison to use deep learning in such a task as the same result or better can be obtained with less computational resources.
Our future work consists of focusing on the algorithms that gave the best classification results and try to refine the process further or combine the best algorithms to obtain better results and detect zero-day attacks.
The datasets presented in this study can be found in online repositories. The names of the repository/repositories and accession number(s) can be found at: https://www.kaggle.com/.
MM: Conceptualization, Formal analysis, Methodology, Writing – review & editing. MB: Data curation, Investigation, Visualization, Writing – review & editing.
The author(s) declare that no financial support was received for the research, authorship, and/or publication of this article.
The authors declare that the research was conducted in the absence of any commercial or financial relationships that could be construed as a potential conflict of interest.
The authors declare that no Generative AI was used in the creation of this manuscript.
All claims expressed in this article are solely those of the authors and do not necessarily represent those of their affiliated organizations, or those of the publisher, the editors and the reviewers. Any product that may be evaluated in this article, or claim that may be made by its manufacturer, is not guaranteed or endorsed by the publisher.
Al-Khshali, H. H., Ilyas, M., Sohrab, F., and Gabbouj, M. (2024). Malware detection with subspace learning-based one-class classification. IEEE Access 12, 81017–81029. doi: 10.1109/ACCESS.2024.3409937
Alqahtani, A., Azzony, S., Alsharafi, L., and Alaseri, M. (2023). Web-based malware detection system using convolutional neural network. Digital 3, 273–285. doi: 10.3390/digital3030017
AV-TEST. (2024). Available online at: https://portal.av-atlas.org/malware/statistics (Accessed May 30, 2024).
Ayofe, A. N., Odufuwa, O. E., Misra, S., Oluranti, J., and Damaševicˇius, R. (2021). Windows PE malware detection using ensemble learning. Informatics 8:10. doi: 10.3390/informatics8010010
Azmee, A. B. M. A., Choudhury, P. P., Alam, M. D., Dutta, O., and Hossain, M. (2020). I: performance analysis of machine learning classifiers for detecting PE malware. Int. J. Adv. Comput. Sci. Appl. 11, 510–517. doi: 10.14569/IJACSA.2020.0110163
Baghirov, E. (2024). Advanced machine learning and interpretability for windows malware detection. Journal of Modern Technology and Engineering 9, 165–177. doi: 10.62476/jmte93165
Connors, C., and Sarkar, D. (2022). Machine learning for detecting malware in PE files. doi: 10.48550/arXiv.2212.13988
Del Aguila, B. R., Pérez, C. D. C., Silva-Trujillo, A., Cuevas-Tello, G. C., and Nunez-Varela, J. (2024). Static malware analysis using low-parameter machine learning models. Computers 13:59. doi: 10.3390/computers13030059
Gibert, D., Mateu, C., and Planes, J. (2020). The rise of machine learning for detection and classification of malware: research developments, trends and challenges. J. Netw. Comput. Appl. 153:102526. doi: 10.1016/j.jnca.2019.102526
Hyrum, S.A., and Phil, R. (2018), EMBER: an open dataset for training static PE malware machine learning models. arXiv:1804.04637.
Jhansi Priya, S., Sadiq, A., Akanksh, Pn., Dhruva, S. K., and Sudhamani, M. V. (2024). Malware Detec- tion and classification in portable executable files using deep learning methods. Int. J. Eng. Res. Technol. 13. doi: 10.17577/IJERTV13IS040270
Jayanth, D. (2024). Available online at: https://www.kaggle.com/datasets/dasarijayanth/pe-header-data (Accessed April 15, 2024).
Joe Beach, C. (2024). Available online at: https://www.kaggle.com/datasets/joebeachcapital/windowsmalwares?select=PE_Header.csv (Accessed May 20, 2024).
Kumar, B. S. R. (2022). Detection of PE malware files using machine learning and deep learning techniques. Int. J. All Res. Educ. Scientific Methods 10, 1269–1277.
Kumar, A., Kuppusamy, K.S., and Aghila, G. (2017), “A learning model to detect ma- liciousness of portable executable using integrated feature set”, Journal of King Saud University - Computer and Information Sciences.
Louk, M. H. L., and Bayu, A. T. (2022). Tree-based classifier ensembles for PE malware analysis: a performance revisit. Algorithms 15:332. doi: 10.3390/a15090332
Manavi, F., and Hamzeh, A. (2021). Static detection of ransomware using LSTM network and PE header. Proc. 26th Int. Comput. Conf., Comput. Soc. Iran, 1–5.
Mauricio, A. (2024). Available online at: https://www.kaggle.com/datasets/amauricio/pe-files-malwares/data (Accessed May 15, 2024).
Merabet, H. E., and Hajraoui, A. (2019). “A survey of malware detection techniques based on machine learning”, IJACSA. Int. J. Adv. Comput. Sci. Appl. 10, 366–373. doi: 10.14569/IJACSA.2019.0100148
Paza, S. L., Pudjiantoro, T. H., and Hadiana, A. (2022). “Malware detection using portable executable header and gradient boosting classification algorithm” in 3rd Asia Pacific international conference on industrial engineering and operations management, vol. 2022. doi: 10.46254/AP03.20220174
Radwan, A.M. (2019), “Machine learning techniques to detect maliciousness of portable executable files, 2019 international conference on promising electronic technologies (icpet) ”.
Raff, E., Sylvester, J., and Nicholas, C. (2017). “Learning the PE header, malware Detec- tion with minimal domain knowledge” in Proceedings of the 10th ACM workshop on artificial intelligence and security - AISec ‘17.
Siregar, A. A., Soim, S., and Fadhli, M. (2023). Optimizing Malware Detection Using Back Propagation Neural Network and Hyperparameter Tuning. J. Artificial Intelligence Data Mining 6, 220–230. doi: 10.24014/ijaidm.v6i2.24731
Sumit, S. L., and Amol, C. A. (2022). Improved deep learning model for static PE files malware detection and classification. Int. J. Computer Network Information Security 14, 14–26. doi: 10.5815/ijcnis.2022.02.02
Varma, S., and Narasimharao, J. (2022). Malware analysis with machine learning: classifying malware based on PE header. Int. J. Res. Applied Sci. Eng. Technol. 10, 3583–3590. doi: 10.22214/ijraset.2022.44668
Yanfang, Y., Tao, L., Donald, A., and Sitharama, L. S. (2017). A survey on malware detection using data mining techniques. ACM Comput. Surv. 50, 1–40. doi: 10.1145/3073559
Keywords: malware, PE file, machine learning, deep learning, classification
Citation: Miraoui M and Belgacem MB (2025) Binary and multiclass malware classification of windows portable executable using classic machine learning and deep learning. Front. Comput. Sci. 7:1539519. doi: 10.3389/fcomp.2025.1539519
Received: 04 December 2024; Accepted: 20 February 2025;
Published: 06 March 2025.
Edited by:
Eduard Babulak, National Science Foundation (NSF), United StatesReviewed by:
Jorge E. López De Vergara, Autonomous University of Madrid, SpainCopyright © 2025 Miraoui and Belgacem. This is an open-access article distributed under the terms of the Creative Commons Attribution License (CC BY). The use, distribution or reproduction in other forums is permitted, provided the original author(s) and the copyright owner(s) are credited and that the original publication in this journal is cited, in accordance with accepted academic practice. No use, distribution or reproduction is permitted which does not comply with these terms.
*Correspondence: Moeiz Miraoui, bS5taXJhb3VpQGFyYWJvdS5lZHUuc2E=
Disclaimer: All claims expressed in this article are solely those of the authors and do not necessarily represent those of their affiliated organizations, or those of the publisher, the editors and the reviewers. Any product that may be evaluated in this article or claim that may be made by its manufacturer is not guaranteed or endorsed by the publisher.
Research integrity at Frontiers
Learn more about the work of our research integrity team to safeguard the quality of each article we publish.