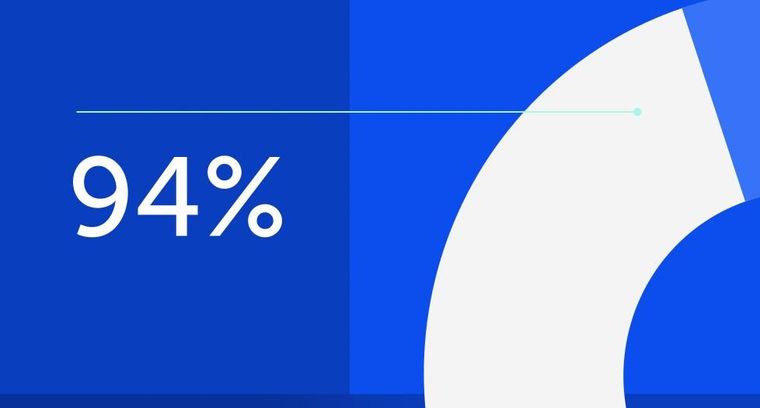
94% of researchers rate our articles as excellent or good
Learn more about the work of our research integrity team to safeguard the quality of each article we publish.
Find out more
PERSPECTIVE article
Front. Comput. Sci., 21 March 2025
Sec. Human-Media Interaction
Volume 7 - 2025 | https://doi.org/10.3389/fcomp.2025.1508004
This article is part of the Research TopicDigital Heritage FuturesView all 3 articles
This perspective article argues that not only humanities benefit and are transformed by recent AI developments but AI might also benefit from the humanities. This is demonstrated with regard to the symbol grounding problem in AI by considering that meaning is not the outcome of a two-way relation between an object and a brain (or AI) but of the negotiation of meaning in the triadic relation between objects, symbols, and human practices. This is common in the interpretive social research tradition of the humanities. We argue that AI benefits from embedding generative methods in interpretive social research methodologies. This can be achieved by using the example of the recently developed methodology of interpretive agent-based simulation (iABM). This methodology enables the generation of counterfactual narratives anchored in ethnographic evidence and hermeneutically interpreted, producing symbolically grounded and plausible futures. Criteria for plausibility correspond to contemporary guidelines for assessing trustworthy AI, namely human agency and oversight, transparency, and auditability.
AI is making unexpected rapid progress. Still, in 2019, a book on AI (Marcus and David, 2019) noticed that AI was still achieving success only for very narrow, clearly defined problems. For instance, one chapter heading reads: “If computers are so smart, how come they cannot read?” Only 3 years later, Chat-GPT was launched to a wider public. In the same year, a Google engineer gained (unwanted) prominence for his claim that their machine was sentient (Wooldridge, 2022). Regardless of whether this may be true (and if or how this question can be answered), reading and writing text in natural language is now a common exercise of large language models (LLMs), with so far unpredictable consequences for a digital heritage future.
However, despite the undeniable success of LLMs, the eminent AI scholar Michael Wooldridge still claims that such machine learning systems are considerably restricted nonetheless (Wooldridge, 2022). What is missing, he asks and answers: the world. LLMs can write essays on words that are often used together. Typically based on co-occurrence analyses, LLMs combine words that are commonly found in close proximity in the training data, such as the words “rain” and “wet” (Wooldridge, 2022). However, for the machine, these words remain just symbols without any contextual experience. Clearly, even though Chat-GPT might write an essay on rain, it has never experienced rain. This, in essence, is the well-known and long-debated symbol grounding problem (Harnad, 1989; Belpaeme et al., 2009), a philosophical puzzle in disciplines such as linguistics, cognitive science, and AI, and questions how symbols are connected to objects in the real world.
The argument that we want to make here is that not only is AI transforming the landscape of humanities, but with regard to the symbol grounding problem, AI might also benefit from the humanities. More precisely, contemporary generative AI might benefit from taking into account methodological advancements in agent-based simulation (ABM), a domain that used to be but is no longer seen as a subfield of AI. By acknowledging the importance of small, i.e., rich, data in a big data world (Dirksen et al., 2021), the methodological advancement we propose concerns embedding ABM in qualitative interpretative methods in the social sciences. This enables us to identify how symbols relate to the situated meaning of social-cultural practices or how meaning is attached to the social world. In section 2 of this article, the symbol grounding problem will be described, after which we will provide an example in the field of cultural criminology in section 3. In section 4, we will explain how the notion of plausibility contributes to symbol grounding.
As the statement of Michael Wooldridge above exemplifies, progress in AI does not remain without skepticism. We contend that with respect to the symbol grounding problem, AI still has a lot to learn from how intelligence is enacted in human practices, that is, how symbols are connected to aspects of the real world through the work of interpretation. It is a specific feature of the human species that allows humans to orient themselves in the world by culturally embedded language games (Neumann and Cowley, 2013). Meaning is not a two-way relation between an object and a brain (or AI), but rather the negotiation of meaning happens in the triadic relation between objects, symbols, and human practices. Symbols arise from, and are thus tied to, particular social practices (situated meaning). For instance, whether an eye movement is an involuntary twitch or a conspiratorial signal depends on the context of actions and interactions in which it is situated (Geertz, 1973). Investigating how symbols relate to the human social world is exactly what interpretive social research is about. This is not to say that we contend that a computer might experience rain with the help of social scientific knowledge. However, for instance, ethnography, traditionally in pursuit of understanding foreign cultures, is faced with a problem similar to the symbol grounding problem: the ethnographer has to dissect how actors in a certain cultural system negotiate meaning and, hence, connect those cultural symbols to objects in the world. By discussing the methodology of interpretive agent-based modeling, which includes ethnography and hermeneutic analysis, we will show how the symbol grounding problem may possibly be tackled in generative AI. Before doing so, let us return to explaining the methodology of interpretive AMB, or iABM (Neumann, 2024).
In this qualitative interpretative approach to ABM, the development of a conceptual model is informed by ethnographic data analysis. The conceptual model consists of condition-action sequences based on ethnographic material providing the ‘micro level rules’ of the model. These micro rules are connected with the original codes of the ethnographic data analysis in the form of annotations. Due to this traceability, it is possible to generate narrative scenarios that bring contextualized meaning back into the output of the simulation. Hence, the simulation output remains attached to its intelligible context, revealing the societal “Eigendynamik” (Beuving, 2021).
Figure 1 shows how the simulation output (on the right) is traced to the transcripts of the ethnographic evidence on the input side of the model (on the left). Annotations feed the micro model rules consistent conceptual description (CCD) viewer. Following a declarative programming style, certain actions are executed during the simulation, depending on the preceding constellation of conditions (trace graph visualization).
The executed rules reflect the range of potentialities, so to speak, and change the pathway of further condition-action sequences. Traceability of the simulation output to the ethnographic material as instances of situated causality enables the generation of a diversity of counterfactual narrative scenarios. They depart from the potential transferability of the ‘chains of meaningful action’ observed in the ethnographic data referred to as social mechanisms. “A mechanism is a social process that is reliably traceable in multiple locations (or across case studies) (…). Meaningful mechanisms (…) explicitly involve signification and interpretation as part of the causal pushing and pulling on the world that they produce” (Lichterman and Reed, 2015, p. 613). Alternatively, the simulation output of iABM consists of meaningful social mechanisms transposed to different social situations and settings.
Symbol grounding in iABM does not occur only through ethnographic analysis but also on the level of interpretive validation of the ways in which the relationships of situated causality play out in different social situations. In this phase of the research procedure of iABM, the simulated counterfactual narratives are subjected to objective hermeneutic interpretation by using a sequence analysis. Sequence analysis is “a method of interpretation that attempts to reconstruct the meaning of any kind of human action sequence by sequence, that is, sense unit by sense unit” (Kurt and Herbrig, 2014, p. 281) with the aim of dissecting the ‘rules’ of the symbolic world. Counterfactually, one can then examine what course of events could be expected if one of these sense units is changed or deleted (Levy, 2008). Hence, hermeneutic interpretation of the counterfactual scenarios determines whether a particular scenario provides a plausible variation of the action found in the ethnographic material (Neumann et al., 2024). This step of the research procedure of iABM, i.e., output ‘validation’, enables us to make a move from the potential to the plausible. This is in sharp contrast to conventional tools of generative methods, where it is often simply claimed that the ‘behavior’ of LLM-ABM agents is similar to human behavior (Gao et al., 2024) without providing evidence to substantiate that claim.
The processes of (intersubjective) interpretation inherent in both the ethnographic data analysis on the input side of the methodology and the hermeneutic validation of the output side of the simulation provide an indirect connection of how human practices relate symbols to objects in the world.
In this section, an example will be provided that demonstrates how the machine is put in the loop of interpretive social research, that is: how the methodology enables human meaning-making with machines and how this contributes to symbol grounding.1 The example is taken from criminology as iABM was initially developed for the field of criminological research (Lotzmann and Neumann, 2017), although currently applied in other domains as well, such as the investigation of the conditions of organizational resilience to technological change (Secchi et al., 2024). For criminology, the cultural perspective and the method of ethnography are not unfamiliar, especially in the field of cultural criminology, having its origin in the tradition of subculture theorization (Ferrell, 1999; Hebdige, 1979; Rock, 2002). Cultural criminology entails “a methodological sensibility: a sensitivity to subtleties of meaning, an openness to the orientations of others–even if those others are textual in nature” (Ferrel et al., 2008, p. 189). Important to state here, however, is that this perspective article aims to make a methodological contribution to the problem of understanding; the criminological example is used for illustrative purposes only.
Deciphering meaning-making (i.e., understanding) is a key methodological problem of the humanities as a science of interpretation. First, we demonstrate how the machine is embedded in small data from ethnographic research through conceptual modeling in terms of micro-level condition-action sequences. This is what we call the “thick conditional,” a reformulation of Clifford Geertz’s famous concept of a “thick description” (Geertz, 1973). Like a traditional thick description, a thick conditional is composed of a combination of description, interpretation, and explanation of field data (Beuving, 2021). This conceptual modeling facilitates a micro-level description of the field data. However, condition-action sequences are not descriptions of a single observation but condense observations into social mechanisms. For instance, in a project analyzing police interrogations (see Lotzmann and Neumann, 2017), many descriptions of acts of aggression were found. These had been of different severity, ranging from an extortionist bringing the children of the blackmailed back home from kindergarten to holding machine guns in the stomach and victims looking like hunted cats. The reaction to experiencing aggression by the victims was very different, ranging from statements such as “I paid but I’m alive” to attempts to kill the offender. The different reactions to facing aggression have been condensed into an interpretation of this aggression by the victim as either “norm of trust demanded” or “norm of trust violated.” Like a thick description, the condition-action sequence is not just raw data but an interpretation that simultaneously explains the different kinds of reactions, i.e., it reflects the symbolic level. The world of human practices on the other hand is preserved in the annotations (Figure 2).
Next, we demonstrate how, in the output phase of the research procedure of iABM, narrative scenarios create novel, meaningful content. IABM enables contextualization, adding layers of meaning (Strathern, 2002) to a simulated scenario. The following example narrative is a counterfactual scenario in which one of the members of a criminal group reacted to intimidation by some of his co-offenders:2
“At the beginning of this story Toby began to mistrust Achim. Eventually, Achim embezzled his money as Toby had invested a significant amount of black money in Achim’s company structure. However, it remains ambiguous what exactly happened. Anyway, Toby wanted his money back. They had a meeting at the office of their lawyer to appraise the value and Achim obeyed the request.”
While being based on empirical data of police interrogations, the concrete “story” generated by the simulation had not been found in the data as such; it is artificially generated content. Sentences in italics are annotations from the data connected by the manually generated ‘hinge points’. The result is a text as a kind of ‘collage’, in which pieces of the original data are linked together in new sequences, depending on when the rules are executed during the simulation.
Sequence analysis revealed that this short story addresses the conceptual field of the meaning structure of trust and the dialectic of crisis and routine (Dickel and Neumann, 2024), substantiating it as a plausible counterfactual scenario. While at first sight rather unspectacular, the example reveals the role of counterfactual reasoning (Dirksen and Neumann, 2024). Namely, in reality (i.e., the police interrogations), three thugs with machine guns waited for Achim in the lawyer’s office, forcing him to his knees and pointing a machine gun at his stomach. Thus, the seemingly trivial example is something radically different from reality. Counterfactual reasoning of the machine allows for a “what if” analysis of what could have happened if certain conditions had been different (Tavori and Timmermans, 2013; Katz, 2001) to explain the facts (Reed, 2017). Hence, putting the machine in the loop of human reasoning enables us to make sense of human action. Symbol grounding results from a process of the negotiation of meaning in the triadic relation between objects, symbols, and human practices.
In iABM, a different composition of individual pieces of empirical evidence generated by the execution of the program code generates different narratives. These counterfactual narratives are novel and thus qualify iABM as a generative method. That is to say, it simulates the truly novel by transposing the real-world relationship of causality of situated action, i.e., the observed ‘chains of meaningful action’, to similar conditions, to subsequently understand the unfolding of this particular chain of action in that new situation where these chains were not observed as such (yet).
Plausible narratives “(…) commence with real-life situations, which are then extended with elements that are hypothetical or speculative, but that are rooted in real-world processes” (Clarke, 2016, p. 78).
This aspect of iABM is to be contrasted with the statistically probable in that interpretive social simulation modeling enables generating not only variations of statistically closely related words (by means of deep learning the co-occurrence of words) but also novel but plausible structural variations of observed social mechanisms. IABM, therefore, enables the imagination of “a wide range of ‘outside-of-the box possibilities’” (Bai et al., 2016, p. 352) rather than a projection of the past and the present into the future, i.e., predicting past variation (Svetlova, 2024, p. 11).
In light of the symbol grounding feature of iABM, we end this article with a reflection on its plausibility criteria. The notion of the ‘plausible’ in iABM refers to the extent to which a counterfactual scenario may be interpreted as a plausible structural and novel variation of an actual empirical case. This feature of iABM relates the interpretation to symbol grounding rather than being a mere hallucination. First, the notion of plausible futures in iABM refers to the credibility of a range of possible futures, which depends on an in-depth idiosyncratic understanding of social mechanisms making up a particular social phenomenon. Second, plausibility is here understood “as a relational or interactional criterion in the sense that it is not an intrinsic property of a scenario (…). Indeed, the plausible and the implausible need to be interrogated and better understood in the context they appear” (Uruena, 2019, pp. 19, 20). This feature of plausibility is safeguarded in iABM by the fact that interpretation, or meaning-making, in ethnography (in the input side of the simulation) and objective hermeneutics (in the output side of the simulation) is intersubjectively arrived at.
These criteria of plausibility correspond to the standards of evaluation developed for assessing trustworthy AI (HLEG AI, 2019, 2020), which are deemed relevant to the symbol grounding debate: human agency and oversight, transparency, and auditability. First, “transparency becomes crucial for investigating AI system behavior post hoc (Mittelstadt, 2021)” and includes traceability requirements. Second, traceability refers to “[t]he data sets and the processes that yield the AI system’s decision, including those of data gathering and data labelling as well as the algorithms used, should be documented to the best possible standard to allow for traceability and an increase in transparency. This also applies to the decisions made by the AI system” (HLEG AI, 2019, p. 18). Traceability, in turn, facilitates explainability and auditability. The traceability inherent in the methodology of iABM may rightly be said to increase the transparency and, hence, the explainability and auditability of how the counterfactual narratives are constructed based on the empirical material. Transparency in iABM, and with it the conformability of the social mechanisms and how they play out in the different counterfactual narratives, is thus safeguarded by way of the traceability of the micro rules of the model to the context of a particular social action and by the objective hermeneutic validation of the counterfactual narratives.
Generating multiple novel imaginations of the future in the form of counterfactual narratives is a key takeaway for contemporary AI technologies for prediction: “when considering the future, there is no single outcome that can serve as a focus for a narrative, and the best that can be done is to create multiple narratives (scenarios), each invoking different dimensions, none of which will entirely ‘predict’ what will happen” (Bai et al., 2016, p. 352). Similar efforts to generate multiple narratives for imagining the future using ML algorithms are sparse, yet initiatives can be found, such as for the imagination of novel climate futures (cf., Sanchez Querub and Niederer, 2022).
IABM, however, goes beyond future imaginations as fictional hallucination, as the integration of (any kind of) generative technologies with methods of interpretive social science enables a generation of symbolically grounded, and hence, plausible counterfactual narratives, enhancing our imagination empirically anchored and intersubjectively arrived at of what might happen in the near future. Adhering to the symbol grounding principles of iABM entails that the generated imaginations remain traceable to their intelligible context, i.e., that their context of meaning ‘travels’ with them, hence transparently providing the grounds on which it may be determined whether they are plausibly applicable to similar situations and settings. Rather than the positivist tradition of the hypothesis testing of the statistical branch of AI, in which “intelligence itself is a positivist experiment,” intelligence as an interpretivist experiment would for AI entail being open to divergent meaning-making in different (cultural) contexts and accommodating the prevailing situated interpretations. Such an AI might “allow other societies to define and construct ‘artificial intelligence’ in, and on, their own terms” (Blackwell, 2021, p. 209).
The original contributions presented in the study are included in the article/supplementary material, further inquiries can be directed to the corresponding author/s.
MN: Conceptualization, Funding acquisition, Writing – original draft, Writing – review & editing. VD: Conceptualization, Writing – original draft, Writing – review & editing.
The author(s) declare that financial support was received for the research and/or publication of this article. MN received funding from the VELUX Foundation for the project Determinants of Resilience in Organizational Networks (grant no 38917).
The authors declare that the research was conducted in the absence of any commercial or financial relationships that could be construed as a potential conflict of interest.
The authors declare that no Generative AI was used in the creation of this manuscript.
All claims expressed in this article are solely those of the authors and do not necessarily represent those of their affiliated organizations, or those of the publisher, the editors and the reviewers. Any product that may be evaluated in this article, or claim that may be made by its manufacturer, is not guaranteed or endorsed by the publisher.
1. ^For this example the police approached the research consortium because they could not understand why a group of drug dealers started killing each other instead of making money together. It turned out that answering their question required the development of a new research methodology which therefore can be denoted as an emergent methodology (Schreier, 2017).
2. ^Names are fictitious as for reasons of protection of privacy only anonymous data was provided to the researchers and ethical guidelines were respected, i.e., research was done with ethical clearance. Moreover, it has to be noted that even though the request came from the police, it came from an internal analysis unit, not criminal prosecutors. While the data was originally collected for the purpose of criminal investigation, this study was done with the aim of furthering scientific understanding of criminal acts and mechanisms (within the police). Thus, sensitivity is needed about the distinction between the purpose of the original data collection (i.e., criminal investigative analysis) and the purpose of the data analysis in the project (i.e. scientific understanding).
Bai, X., Van der Leeuw, S., O’Brian, K., Berkout, F., Biermann, F., Brondizio, E., et al. (2016). Plausible and desirable futures in the Anthropocene: a new research agenda, global Env. Change 39, 351–362. doi: 10.1016/j.gloenvcha.2015.09.017
Belpaeme, T., Cowley, S., and MacDorman, K. (2009). Symbol grounding. Amsterdam: John Benjamins Publishing Company.
Beuving, J. (2021). Problems of evidence in ethnography. A methodological reflection on the Goffman/Mead controversies (with a proposal for rules of thumb). Forum Qual. Soc. Res. 22:1. doi: 10.17169/fqs-22.1.3567
Blackwell, A. (2021). Ethnographic artificial intelligence. Int. Sci. Rev 46, 198–211. doi: 10.1080/03080188.2020.1840226
Dickel, S., and Neumann, M. (2024). Hermeneutics of social simulation. On the interpretation of digitally generated narratives, in an interpretive acccount to agent-based social simulation. Using criminology to explore cultural possibilities. London: Routledge, 130–152.
Dirksen, V., and Neumann, M. (2024). “On the construction of plausible futures in interpretive agent-based modelling” in An interpretive account to agent-based social simulation. Using criminology to explore cultural possibilities. ed. M. Neumann (London: Routledge), 170–188.
Dirksen, V., Neumann, M., and Lotzmann, U. (2021). From agent to action: the use of ethnographic social simulation for crime research. Futures 142:102994. doi: 10.1016/j.futures.2022.102994
Ferrell, J. (1999). Cultural Criminology. An. Rev. of Soc. 25, 395–418. doi: 10.1146/annurev.soc.25.1.395
Gao, C., Lang, X., Li, N., Yuan, Y., Ding, J., Zhou, Z., et al. (2024). Large language model empowered agent-based modeling and simulation: a survey and perspectives. Human. Soc. Sci. Commun. 11:1259. doi: 10.1057/s41599-024-03611-3
Harnad, S. (1989). Minds, machines and searle. J. Theo. Exp. Art. Int. 1, 5–25. doi: 10.1080/09528138908953691
HLEG AI (2019) Ethics Guidelines for Trustworthy AI, European Commission. Accessed online at: https://digital-strategy.ec.europa.eu/en/library/ethics-guidelines-trustworthy-ai (Accessed March 5, 2025).
HLEG AI. (2020). Assessment List for Trustworthy Artificial Intelligence (ALTAI) for self-assessment. Accessed online at: https://digital-strategy.ec.europa.eu/en/library/assessment-list-trustworthy-artificial-intelligence-altai-self-assessment (Accessed March 5, 2025).
Katz, J. (2001). From hw to why: on luminous description and causal inference in ethnography (part I). Ethnography 2, 443–473. doi: 10.1177/146613801002004001
Kurt, R., and Herbrig, R. (2014). “Sozialwissenschaftliche Hermeneutik und hermeneutische Wissenssoziologie” in Handbuch Methoden empirischer Sozialforschung. eds. N. Baur and J. Blasius (Wiesbanden: Springer), 473–491.
Levy, J. (2008). “Counterfactuals and case studies” in The Oxford handbook of political methodology. eds. J. Box-Steffensmeier, H. Brady, and J. Collier (Oxford: Oxford University Press), 627–643.
Lichterman, P., and Reed, I. A. (2015). Theory and contrastive explanation in ethnography. Soc. Meth. Res. 44, 585–635. doi: 10.1177/0049124114554458
Lotzmann, U., and Neumann, M. (2017). “A simulation model of intra-organizational conflict regulation in the crime world” in Social dimensions of orgaized crime: Modelling the dynamics of extortion rackets. eds. C. Elsenbroich, D. Anzola, and N. Gilbert (Cham: Springer), 177–213.
Marcus, G., and David, E. (2019). Rebooting AI: Building artificial intelligence we can trust. New York: Pantheon Books.
Mittelstadt, B. (2021). “Interpretability and transparency in artificial intelligence” in The Oxford handbook of digital ethics. ed. C. Véliz (Oxford: Oxford University Press), pp. 378–410.
Neumann, M. (2024). An interpretive acccount to agent-based social simulation. Using criminology to explore cultural possibilities. London: Routledge.
Neumann, M., and Cowley, S. (2013). “Human agency and the resources of reason” in Cognition beyond the brain. Computation, interactivity, and human artifice. eds. S. Cowley and F. Vallee-Tourangeau (London: Springer), 13–30.
Neumann, M., Dirksen, V., and Dickel, S. (2024). On the construction of plausible futures in interpretive agent-based modelling, in an interpretive acccount to agent-based social simulation. Using criminology to explore cultural possibilities. London: Routledge, 170–188.
Reed, I. A. (2017). Ethnography, theory, and sociology as human science: an interlocution. Ethnography 18, 107–129. doi: 10.1177/1466138115592417
Rock, P. (2002). “Sociological theories of crime” in The Oxford Handbook of Criminology. eds. M. Maguire, R. Morgan, and R. Reiner (Oxford: Oxford University Press), 2–42.
Sanchez Querub, N., and Niederer, S. (2022). Climate futures: machine learning from cli-fi, convergence. The Int., J. of Res. into New Media Technologies 30, 285–303. doi: 10.1177/13548565221135715
Schreier, M. (2017). Kontexte qualitativer Sozialforschung: arts-based research, mixed-methods und emergent methods. Forum qual. Sozialforschung 18, 1–27. doi: 10.17169/fqs-18.2.2815
Secchi, D., Gahrn-Andersen, R., and Neumann, M. (2024). Complexity in systemic cognition: theoretical explorations with agent-based modeling. Systems 12:287. doi: 10.3390/systems12080287
Strathern, M. (2002). “Abstraction and Decontextualization: an anthropological comment” in Virtual society? Technology, Cyberbole, reality. ed. S. Woolgar (Oxford: Oxford University Press), 303–313.
Svetlova, E. (2024). “Economic expectations and an AI agent” in Routledge handbook on economic expectations in historical perspective. eds. A. Nützenadel, I. Köhler, L. Lenel, and J. Streb (London: Routledge).
Tavori, I., and Timmermans, S. (2013). A pragmatist approach to causality in ethnography. Am. J. Sociol. 119, 682–714. doi: 10.1086/675891
Uruena, S. (2019). Understanding “plausibility”: a relational approach to the anticipatory heuristics of future scenarios, futures: the journal of policy, planning and futures studies, 08/2019. Volume 111, 15–25. doi: 10.1016/j.futures.2019.05.002
Keywords: symbol grounding, interpretive agent-based modelling (ABM), interpretive social research, generative methods, transparent AI
Citation: Neumann M and Dirksen V (2025) Symbol grounding for generative AI: lessons learned from interpretive ABM. Front. Comput. Sci. 7:1508004. doi: 10.3389/fcomp.2025.1508004
Received: 08 October 2024; Accepted: 24 February 2025;
Published: 21 March 2025.
Edited by:
Paola Di Giuseppantonio Di Franco, University of Essex, United KingdomReviewed by:
Terhi Nurmikko-Fuller, Australian National University, AustraliaCopyright © 2025 Neumann and Dirksen. This is an open-access article distributed under the terms of the Creative Commons Attribution License (CC BY). The use, distribution or reproduction in other forums is permitted, provided the original author(s) and the copyright owner(s) are credited and that the original publication in this journal is cited, in accordance with accepted academic practice. No use, distribution or reproduction is permitted which does not comply with these terms.
*Correspondence: Martin Neumann, bWFydG5ldW1Ac2R1LmRr
Disclaimer: All claims expressed in this article are solely those of the authors and do not necessarily represent those of their affiliated organizations, or those of the publisher, the editors and the reviewers. Any product that may be evaluated in this article or claim that may be made by its manufacturer is not guaranteed or endorsed by the publisher.
Research integrity at Frontiers
Learn more about the work of our research integrity team to safeguard the quality of each article we publish.