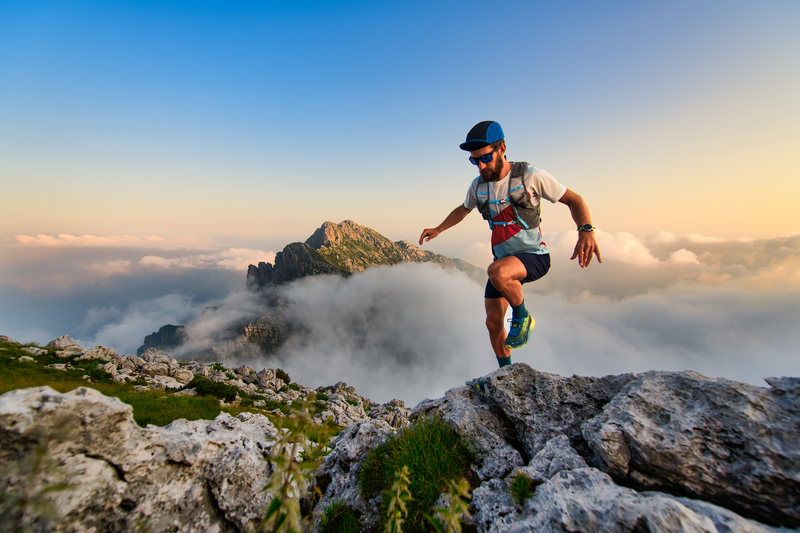
95% of researchers rate our articles as excellent or good
Learn more about the work of our research integrity team to safeguard the quality of each article we publish.
Find out more
SYSTEMATIC REVIEW article
Front. Comput. Sci. , 01 April 2025
Sec. Human-Media Interaction
Volume 7 - 2025 | https://doi.org/10.3389/fcomp.2025.1459787
Introduction: In an era where technology is revolutionizing the way business is done, specialists are continuously developing Interactive Voice Response (IVR) systems used in call centers in an attempt to meet the ever-changing needs of both customers and businesses. Before investing in an IVR system, call center managers must have a clear picture of the advantages and challenges associated with this technology, and for researchers, it is important to know what are the emerging topics that could be future research directions in the field. However, there is a lack of comprehensive reviews that present an overview of how IVR systems are used in call centers, and this paper aims to fill this gap in the literature by conducting a scientometric research on scientific production in the field.
Methods: A total of 284 documents indexed in the Web of Science database between 1991 and 2023 were analyzed using VOSviewer software. The scientometric analysis included a semantic examination of research trends and thematic clustering within the field.
Results: The semantic analysis of scientific production highlighted four main research directions: Automatic Speech Recognition, IVR flow optimization, Reliability of IVR systems as a methodology for studies, and Human-Computer Interaction for Development (HCI4D). These clusters highlight the intellectual structure of the field.
Discussion: The paper discusses the general intellectual structure of the field, with the four semantic groups being reviewed. Additionaly, emerging topics were identified and the advantages and challenges that accompany the use of this technology in call centers were discussed.
Call centers are now an established way to manage customer relationships (Colladon et al., 2013). In recent decades, a significant evolution in this area has been represented by the introduction of IVR technology, which has revolutionized the way incoming calls are handled. IVR (Interactive Voice Response) systems are „specialized technologies designed to enable self-service of callers without the assistance of human agents” (Khudyakov et al., 2010). They started being utilized commercially in the 1970s by the banking sector, with the aim of providing information to customers on bank account balances. Although at first, the applications were limited and involved significant costs, in the subsequent years, the technology advanced rapidly, introducing new functionalities such as speech recognition, text-to-voice conversion and Internet integration (Sánchez-Hevia et al., 2022).
It is important to understand that IVR technology has not only enabled the automation and optimization of call center processes but has also brought substantial benefits in terms of operational efficiency and customer satisfaction. Through IVR, customers can quickly and efficiently obtain the information they require, without the need to involve a human operator. This not only reduces customer waiting time but also costs for the company, as it requires fewer human resources to manage calls, with IVR systems being able to manage up to 60% of incoming calls (Karademir and Heves, 2013). This is the case of an inbound call center, which is called by users to request certain information, and services. On the other hand, in outbound call centers, it is the company that calls the customers or people who are part of the target groups either to sell them certain products/services, to monitor their health, to provide support, to promote a certain type of behavior or to question them about the quality of the company’s services (post-call questionnaire). In both cases, the same technology is used, but in outbound call centers human agents can be completely absent, using either only the classic conversation system or a virtual assistant.
In an era where efficiency and customer experience are a priority for any business, IVR technology is becoming increasingly vital. However, it is essential to ask to what extent the quality of service provided by IVR is comparable to that provided by human agents. Before investing in an IVR system, call center managers must have a clear picture of the advantages and challenges associated with this technology, as well as future research directions in the field. Literature review articles on IVR systems used in call centers are limited, with only articles on narrow topics. For example, Saberi et al. (2017) conducted a literature review on the evolution of call centers, addressing all aspects related to call center activity, but IVR systems are discussed very little. On the other hand, Inam et al. (2017) present a review of IVR technologies. However there is a lack of comprehensive reviews that present an overview of how IVR systems are used in call centers, and this paper aims to fill this gap in the literature by conducting a scientometric research on scientific production in the field.
The purpose of this paper is to identify current and future research directions in the field of IVR technology used in call centers, conducting a comprehensive analysis of the field, which is currently missing in specialized literature. In this regard, a scientometric research was carried out, which allows the identification of research directions that may be difficult to observe for a single researcher through “close reading.” The resulting data provides managers, developers, and researchers with a solid framework for understanding and guidance in this ever-evolving field. The questions underlying this research are:
1. What are the most important research directions in the field of IVR technology?
2. What emerging topics could be future research directions?
Given that the semantic analysis of scientific production will highlight the main research directions in the field, based on the identified thematic areas, an analysis of the relevant literature for each semantic group will be carried out to analyze the field in depth and emphasize the advantages and the challenges that accompany the use of this technology.
Call centers play a critical role in modern business operations, serving as a primary interface for customer communication. Traditionally, a call center is defined as a centralized and specialized facility designed to manage both inbound and outbound communications. Employees, equipped with computer systems, handle incoming and outgoing phone calls, which are efficiently managed through an automatic call distribution system or a predictive dialing system (Duggirala et al., 2011). Even if the fundamental concept of a call center remains the same, modern call centers have evolved significantly due to technological advancements and changing customer expectations (Saberi et al., 2017). Today, many call centers operate as part of multichannel contact centers, integrating voice calls, emails, live chat, and social media interactions. Despite the diversity of communication channels in contact centers, this paper will focus on call centers, as most customers still prefer direct human interaction (Lemon and Verhoef, 2016). This preference highlights a fundamental tension: while customers favor human interaction, companies increasingly invest in IVR and AI-driven systems to reduce operational costs (Suhm and Peterson, 2002). In 2022, the global number of call center agents was estimated at 17 million, but it is considered that AI-driven automation will reduce costs with agents by $80 billion in 2026 (Rimol, 2022). Under these conditions, technology has gained importance and has become a fundamental component of modern call centers.
Existing research has examined call centers and IVR systems separately, but a comprehensive review of how IVR is utilized within call centers is still missing. This gap in the literature has implications for both academia and industry, as a systematic understanding of IVR implementation can help call center managers select the most effective solutions for their business needs. Literature reviews on this topic are generally fragmented. Pinedo et al. offer foundational insights into call center management and present the IVR system as a simple decision tree used to streamline operations and reduce the time human agents spend on a call (Pinedo et al., 2000). Saberi et al. (2017) conducted a literature review on the evolution of contact centers, covering various aspects of their operations. However, IVR systems receive minimal attention. The authors emphasize the importance of making contact centers more interactive and integrating all customer information obtained through various channels. They also highlight that the topic of customer ID matching, from an operational process perspective, has not been thoroughly explored. On the other hand, Inam et al. (2017) present a review of IVR technologies and conclude that advancements in mobile technology have facilitated the development of IVR systems, highlighting opportunities for the growth of speech-enabled systems, which can help improve customer acceptance of the technology.
Shah et al. (2023) conducted a comprehensive literature review to explore the benefits of using natural language processing (NLP) in contact centers and argue that its implementation can help businesses eliminate the common frustrations customers face with IVR systems, however, there is limited research on this topic. NLP uses machine learning techniques to interpret the context and meaning of natural language input. It is used to develop AI virtual assistants that can instantly assess intent, understand complex queries, and engage in human-like interactions (Mocanu et al., 2022).
Several literature reviews have focused on speech signal processing but not on how it is applied in call centers. Bhardwaj et al. (2022) reviewed the literature on Automatic Speech Recognition (ASR) systems specifically designed for children’s speech recognition. Alharbi et al. (2021) analyzed ASR research from 2015 to 2020, identifying that researchers primarily focus on addressing issues that affect system performance, such as dialect variations, background noise, and speech interface challenges, aiming to improve the accuracy of speech recognition in different environments. Singh and Goel (2022) reviewed methods for identifying emotions in speech, the databases used, speech features, and various approaches.
Although these studies provide valuable insights into IVR technology, none specifically address its application in call centers. Furthermore, no scientometric or bibliometric studies were identified that analyzed research trends in IVR integration in call centers. This study aims to fill this gap by providing a structured, data-driven overview of existing research and emerging trends in IVR technology used in call centers. By mapping research on this topic, this study provides valuable information to call center managers, technology developers, and researchers for understanding current advances and future research opportunities.
The method used for this research was scientometric analysis, which allows the identification of the most current themes and research topics in a certain field, as well as the identification of the most cited papers and authors who have addressed a certain topic. Structural review involves examining the relationships between thematic areas and using some form of quantification to summarize a broad literature (Porter et al., 2002). Scientometrics is closely related to Bibliometrics and Informetrics, with the terms often used interchangeably as they share common principles and tools (Haghani, 2023). However, the three metrics belong to different disciplines, and each has a somewhat specialized focus. Bibliometrics places significant emphasis on the advancement of bibliometric methods in scientific research. Informetrics uses informetric methods and mathematical models to analyze the distribution and ranking within information systems. While scientometrics focuses on scientific development trends (Yang et al., 2020), providing deeper insight into thematic structures and research trends.
As a representative sample of the population of scientific papers in the field, the Web of Science database was chosen, as it indexes thousands of periodicals from most scientific fields, representing 90% of the most valuable journals for the progress of science and technology. At the same time, it records and links all references from scientific papers published in indexed journals, offering the possibility to follow the dissemination of scientific information and identify the impact that a particular article had in a particular field of research.
The search in the Web of Science database was conducted on 02.01.2024, to cover all indexed articles from 1991 to the end of 2023. The papers were searched by topic, namely “interactive voice response,” and “Call Center” or “Call Centre,” and the documents had to include the keyword: “interactive voice response.” The abbreviation “IVR” was not used because we found that it is also used in other fields, such as chemistry or ophthalmology, to designate other terms. Also, for the call center, both the British English name and the American version were used. 1,375 documents were identified. The resulting papers were refined according to the type of documents, being selected only scientific articles and papers published in conference volumes, resulting in 1,194 papers. Given that many of the documents resulting from the first filters mentioned the use of IVR Systems only as a data collection tool, without analyzing for example the efficiency of this tool, a filter was applied according to Web of Science categories, resulting in a sample of 288 articles. In order for Scientometric analysis to be possible, only papers published in English were selected, resulting in a final sample of 284 documents.
The raw data was downloaded as plain text files from the Web of Science (WoS) database. The results were analyzed using VOSviewer software version 1.6.18, which allows scientific mapping to analyze the content of titles and abstracts of scientific publications. Therefore, VOSviewer’s term identification function was employed to systematically pinpoint key terms in the database (co-word analysis) and structure large amounts of text in a semantic map. However, the software has some limitations in the way it processes text, which can affect the accuracy of the results. To mitigate this issue, elements associated with abstract structure and copyright statements that might be present were manually excluded. Subsequently, a threshold for the occurrence of the term was applied, so that a term must appear in at least 2 different articles to be considered for inclusion in the semantic map. This threshold helps ensure that relationships between terms are placed reliably on the map, eliminating nouns that include possible spelling or meaningless mistakes.
To get terms ready for mapping, VOSviewer measures the connection between terms using the association strength measure and indicates the number of terms that should be included in the map. In the case of this research, of the 1,151 terms identified, only 203 are used more than twice. Of these, only 195 are interconnected and have been included in the semantic map. To identify term groups (thematic areas), the default grouping resolution parameter 1 was used and the minimum group size was set at 25 terms (a number considered sufficient to review and be visible on a static map). The groups were analyzed, and research directions were identified. The realization of the scientometric study includes seven stages mentioned in Supplementary Table 1, starting with the formulation of the problem, the establishment of research protocols and criteria, and the extraction of data, which were later analyzed, synthesized, and discussed.
Although scientometric analysis is a powerful tool, it is based on quantitative and automated data and may not fully capture the complexity and nuances of scientific research. To enhance the depth of the analysis and address the potential limitations of scientometric methods, an in-depth review of the major thematic areas identified in the semantic map was conducted. The most relevant articles were selected for each cluster where the full version of the article was available, and a detailed content analysis of 55 papers across four research directions was performed. This qualitative approach added depth to the findings, ensuring that insights were not solely based on automated text analysis but were also supported by a thorough examination of key literature.
The resulting semantic map includes 195 terms, grouped into four thematic groups, with 990 links between terms. The program’s mapping algorithm determines the placement of terms on the map by minimizing the difference between the strength of association and the distance between two terms so that terms that tend to co-occurre in the analyzed content are placed closer to each other. The program offers four different map views, each providing different information. Figure 1 reveals interesting structural features that could not be identified by a traditional literature review in the field. It illustrates the network of terms and the four thematic groups identified by the color of the nodes. The groups were numbered by the program according to the number of terms it included.
Thus, the red cluster is the most numerous, comprising 68 terms. These refer mostly to automatic speech recognition (ASR), text transformation into speech, and vice versa, aspects necessary in establishing IVR functionalities in the call center. The green cluster has 47 terms. It seems to be centered on optimizing IVR flows, automation, and call center queues, algorithms, and artificial neural networks. The third group is the blue one and comprises 42 items. It addresses issues related to the reliability of using IVR in therapy or as a methodology in various studies. The last cluster is the yellow one and contains 38 terms. Within this group are treated aspects related to human-computer interaction (HCI4D), digital innovation, and emotion recognition.
Figure 2 illustrates an overlay view of the semantic map, which allows us to identify trends, but also topics that are no longer necessarily of interest. Topics marked in yellow represent trends in the field. It should be noted that the year of publication included in the legend is a calculated average value, considering all the articles in which the term is found. It can be observed that the emerging topics in the field of IVR technology used in Call Center are:
• Noise reduction/elimination for automatic speech recognition with greater accuracy;
• Using the Kaldi toolset (written in C++) for the software development for speech recognition and signal processing;
• Spoken query system (SQS);
• Word Error Rate (WER)—used to measure the performance of a speech recognition system;
• The use of artificial intelligence;
• Classifying IVR users according to the emotions they have during the call;
• Using IVR as a methodology to monitor patients’ health status;
• Digital innovation;
• Machine learning;
• Operations management.
Figure 3 allows the citation impact associated with each topic to be explored, which is calculated based on the average of the “normalized” citation scores of all documents in which that topic appears. Thus, terms that appear in papers with high citation scores, compared to other articles that were published in the same year, have a greater impact, and appear in yellowish-green and yellow. We note that higher impact terms refer to the reliability of using IVR in therapy or as a methodology in various studies (the use of IVR in outbound call centers).
For the in-depth analysis of the major thematic areas identified in the semantic map, the most relevant articles were chosen for each cluster where the full version of the article was available. The author analyzed the content of 55 papers in four directions: “Speech Recognition,” “Optimizing IVR Flows,” “The reliability of IVR Systems as a methodology for studies” “Human-Computer Interaction for Development” (HCI4D). Figure 4 illustrates the papers analyzed according to the research direction addressed.
Although this is the thematic group with the most terms included in the semantic map, the impact of many terms is weak, the works in which the terms appear being more than 10 years old. Therefore, in the review of the cluster emphasis was placed on new terms and those that have a great impact on scientific production in the field. In today’s age of technology, interaction between humans and information systems is becoming increasingly sophisticated, and speech recognition is a key area in this context. Although callers traditionally interact with the IVR system by pressing keys on the phone, specialists believe that the transition to natural language use needs to be made. The core voice technologies used to implement IVR systems that are capable of interacting with humans are: Voice Extensible Markup Language (VoiceXML), Text-to-Speech (TTS), and Automatic Speech Recognition (ASR) (Inam et al., 2017). However, the use of natural language raises certain problems, achieving increased accuracy in real-time speech recognition is still a difficult task, and many researchers are trying to improve the accuracy (Yadava et al., 2023). Real-time speech recognition is negatively influenced by the degradation of spoken speech signals due to both ambient noise (Hamidi et al., 2020) and the quality of the transmission network, as well as distortions introduced by encoding and decoding algorithms (Kumalija and Nakamoto, 2022).
Adjusting data to specific network requirements (eg transmission protocol and bandwidth limitations) involves compressing spoken signals, thus affecting the quality of message interpretation. Lovrenčič et al. (2015) analyze the influence of degradation factors such as transcoding and packet loss on ASR performance and IVR quality and propose a QoS classifier based on Gaussian mixture models (GMM) to determine the optimal input module for IVR depending on network conditions and speech quality. Thus there is the possibility to select the input method between voice input or keystroke depending on the network conditions. If they are degraded, the second option will be chosen, so as not to affect the caller’s experience.
Regarding ambient noise, Kumalija and Nakamoto (2022) performed a comparative analysis between a speech-to-text system trained on clean speech and one trained on integrated noise-network distorted speech. The authors find that the performance of an ASR model trained on clean speech is influenced by the type of noise affecting the speech, but performance drops considerably if network transmission problems also occur. The model trained on noise-network distorted speech demonstrated a 60% improvement in metrics such as word error rate (WER), word match rate (MER), and word information loss (WIL) compared to the model trained on clean speech. However, this improvement begins to diminish when jitter exceeds 0.3 and packet loss surpasses 15%. The authors recommend using ASR models trained on distorted speech and using more versatile speech codecs that include jitter buffering algorithms and data loss hiding support.
Hamidi et al. (2020) conducted experiments to evaluate the performance of the speech recognition system in noisy conditions at different decibel levels using the digits of the Amazigh language. The authors found a major degradation of the recognition accuracy in the case of the decoded speech signal, with the best results being obtained at 3db. Also, the recognition of digits containing the consonant “S” was most affected by the noise level. The authors recommend improving the interactive system in noisy environments and increasing the system’s accuracy by using hidden Markov models, popular methods for machine learning, and sequence modeling.
Another experimental study highlights that ASR models based on Time Delay Neural Networks (TDNN) achieved superior results compared to ASR models based on other modeling techniques, therefore they were used in the development of the proposed E2ECKASR system to be applied in various fields where real-time continuous speech recognition is crucial (Yadava et al., 2023).
Another problem is related to the ability of systems to recognize speech in lesser-used languages. While engineering is the primary challenge in designing language technologies for resource-rich languages, the main problem in resource-poor languages lies in developing effective data collection methods upon which language technology can be constructed. For example, creating a speech-to-text interface in a specific language requires a minimum of 500 h of audio content (Mehta et al., 2021). Mehta et al. (2021) proposed four technological methods of data collection for the Gondi language, spoken by only about 2.3 million tribal people in south and central India. These methods are: creation of a Hindi-Gondi dictionary on the basis of workshops organized with representatives of the Gondi language from 6 states, which allowed the purification of the language from dialects; translation of children’s books into the respective language through workshops; Crowdsourcing of translations (community involvement through a dedicated platform to contribute to data collection); information dissemination using Learn2Earn technology.
Yadava and Jayanna (2017) developed a spoken query system that can be utilized to access up-to-date agricultural commodity prices and weather information in the Kannada language (mainly spoken in the Indian state of Karnataka). For the development of the SR models, the Kaldi speech recognition set was used and it was necessary to collect in an uncontrolled environment data about the language of farmers (in all four dialects of the language), transcribe them, validate the data, create the dictionary, create a set of Kannada phonemes, training the system, decoding/testing, developing ASR models at different phoneme levels and finally developing a query system using the developed ASR models. Praveen Kumar et al. (2020) subsequently developed various acoustic models, including monophone, triphone1, triphone2, triphone3, subspace Gaussian mixture models (SGMM), combinations of deep neural networks (DNN) and hidden Markov models (HMM), DNN and SGMM, and SGMM and maximum mutual information. They conducted a series of experiments to determine the word error rate depending on the modeling techniques used, and the results demonstrated that the combination of DNN and HMM provided superior recognition rates compared to conventional ASR modeling techniques.
Shahnawazuddin et al. (2017) have developed a voice query system in Assamese (Assam state, India) which they continue to improve by incorporating a background noise suppression module based on zero-pass filtering. At the same time, for acoustic modeling, they used techniques based on the Gaussian mixture of subspaces (SGMM) and deep neural networks (DNN), which proved to be more powerful than the previously used GMM-HMM approach. These improvements increased the system’s performance by 39% in terms of word error rate. The development of ASR models for less-used languages is a rather difficult process, requiring a high-quality and large data set.
Another study introduces a system for speech recognition and synthesis tailored for the Kazakh and Russian languages. Khomitsevich et al. (2015) designed a Kazakh-Russian bilingual system because most Kazakh speakers are bilingual. A TTS voice was created for the Kazakh language and an additional Russian voice using the same bilingual voice artist’s recordings. A Kazakh language speech database was gathered and utilized to train deep neural network acoustic models for the speech recognition system. These models have shown adequate performance for use in practical applications, such as interactive voice response and keyword identification scenarios. The issue of IVR functioning in multiple languages is also raised by other researchers. Bhat et al. (2013) believe that customers generally prefer to talk directly to a human agent, especially in a multilingual country like India, where the agent must necessarily speak the customer’s language, making the system difficult to scale.
Nicmanis and Salimbajevs (2022) developed a prototype of a verbal dialogue system that integrates automatic speech recognition (ASR), natural language understanding (NLU), robot management system, and expressive text-to-speech (TTS) functionalities. The authors claim that replacing legacy IVR systems with this prototype can significantly improve the customer experience.
Interactive Voice Response must address the challenges of spoken language conversations, pronunciation variations, recognition problems in noisy environments, limitations of human cognition, working memory, and user differences. Khan et al. (2013) outline the methods used to address these challenges, applied in an IVR system for farmers with limited literacy, but the majority of the evaluation methods and metrics are not specific to any particular domain and can be used for similar systems. The error recovery models were „Signal Analysis and Decision, Confidence Measure and Polling, Complementary Information, Runtime model generation.” Error recovery mechanisms are very important in voice inquiry systems. Voice Activity Detection (VAD) can be used to detect not only no response but also weak responses from users. In these cases, the system will ask him to repeat the query louder. The user will be asked to say “Yes” or “No” at each decision-making stage, following the recognized output. To enhance the level of confidence in the recognized output, multiple parallel decoders can be employed to recognize the user’s response and their outputs will be analyzed to create the final response (Yadava and Jayanna, 2017).
The studies included in this thematic group demonstrate that technological advancement in the field of speech recognition is vital for improving the interaction between humans and computer systems, with an emphasis on the shift towards the use of natural language instead of traditional keystroke interaction. The main underlying technologies for implementing human-interacting IVR systems include Voice Extensible Markup Language (VoiceXML), Text-to-Speech (TTS), and Automatic Speech Recognition (ASR). However, real-time speech recognition is still a difficult task, with challenges related to the degradation of oral speech signals, ambient noise, and transmission network quality. Studies have shown that ASR models trained on distorted speech and using more versatile speech codecs can improve speech recognition performance in environments with high levels of noise.
The development of ASR models for lesser-used languages requires a high-quality and large-scale data set, as well as innovative data collection techniques, so in some countries IVR technology is not very developed. There is an increased concern for the development of voice query systems in multiple languages, with technologies and methods adapted to the specifics of each language and environment of use.
Studies show that using time-delay neural network (TDNN) models has led to superior results in real-time continuous speech recognition in comparison to other modeling techniques. Also, the use of error correction mechanisms is very important for correctly understanding the caller’s intent. The continuous improvement of speech recognition technologies will significantly contribute to the efficiency and accessibility of human-machine interaction systems in different fields and environments of use.
The way the IVR flow is designed influences the duration of calls, the quality of service perceived by customers, and, to a certain extent, the performance of the company. First of all, the technology used is very important. An analysis of 38 IVR systems, used in various fields, highlights that the most commonly utilized tools for developing voice and touch tone applications are: VoiceXML voice interface, mostly used in Customer Care (67%), but also in other areas, probably because it is free and user-friendly; Voxeo voice engine—a commonly utilized voice server for hosting IVR systems, valued for its flexibility and security features; MySQL database is the primary database at the backend of the IVR system. Users access information by pressing keys based on a predefined menu structure. Typically, the information and menu structure remains static throughout the system, necessitating manual intervention for any changes (Inam et al., 2017).
Given that the services offered by companies and the needs of different customers are constantly changing, a real problem is the static nature of IVR trees. Redesigning the IVR tree, adding new modules, or changing certain flows requires the intervention of experienced programmers to modify the underlying source code used for IVR programming. Keeping up with this dynamic takes time and money. To prevent this problem Karademir and Heves (2013) developed a dynamic interactive voice response platform that allows non-programmers to design, modify, and manage all IVR scenarios. The platform uses Voice XML technology as the phone interface through which the user interacts with the ASP.NET website, C# Windows Service as the middleware, Microsoft SQL Server as the database for information storage, and C# for the user interface. The Graphical User Interface (GUI) allows performing various transactions based on access rights, such as designing parameters, scenario flows, imposing system-related work rules, testing operations, etc. The interface also allows real-time situation monitoring and system reports. Creating a flow is very simple requiring only the placement of action elements one after the other to create a flow, without the need to rewrite the source code. All scenarios, flows, and actions are stored in the database of the dynamic IVR platform, allowing them to be utilized for creating new scenarios or applications by various users. Considering that the standard scenarios comprise 156 flows and 1,200 actions, the process of creating or modifying such scenarios becomes straightforward and feasible using the developed dynamic IVR platform. Furthermore, the platform offers testing capabilities, enabling users to test scenarios and flows before deploying them into production, and being able to identify and correct errors, if any.
Salcedo-Sanz et al. (2010) propose an evolutionary algorithm employing Dandelion coding for obtaining near-optimal IVR trees, using it for the design of a call center of an Italian telecommunications company. In the design of the IVR tree, the number of services is determined in advance, the challenge being to group these services as efficiently as possible to minimize the resulting average service time. The authors’ proposed approach considerably improves the results of a Huffman approach.
The duration of the service provided by the IVR constitutes a significant part of the Quality of Service (QoS) provided by a call center as a whole. An analysis of IVR structure and service time in five call centers within the telecommunications sector reveals that the current design of IVR trees results in service times that often exceed the time needed to wait for a human agent due to overly lengthy announcements which negatively impact the service quality. The maximum service time within an IVR can reach 450 s. Therefore, to improve the service, it is necessary to reduce the total service time in the IVR and reduce the length of the chain of announcements and the options presented at each step (Colladon et al., 2013).
Thirumaran et al. (2016) believe that the current IVR systems do not offer comfort of use for customers, being monotonous. Callers must press a number on the phone keypad to select the option associated with the audio prompt. In certain instances, the structure of the service may require customers to make up to eight choices by following the ad chain (Colladon et al., 2013). The authors developed a self-tuning IVR system that generates context-based menus using the Backpatching technique, which includes ontology-based knowledge representation and abstraction of the necessary information from the ontology using semantic web services to improve the menu generated by the IVR system. The Java Expert System Shell (JESS) rule engine is used to generate the context-based menu and thereby optimize the final menu according to the user’s expectations when interacting with the IVR system. Subsequently, this type of IVR system dynamically uses the top-down and bottom-up approach, thereby reducing response time and call duration, and improving user satisfaction and automation levels (Thirumaran et al., 2016).
Another important aspect of the operation of the IVR is the algorithm for routing calls to human agents. Andrade and Moazeni (2023) performed an analysis of real data from the call centers of a large USA insurance company, identifying the characteristics that significantly influence the transfer rate to a human operator, these being billing and payment attempts, various channels, caller types, specific caller intentions, and location. The authors argue that to improve the customer experience calls with high transfer probabilities should be proactively transferred before the customer explicitly requests it.
On the other hand, Tezcan and Behzad (2012) propose dynamic routing policies to direct customers to different IVR service modes according to each customer’s characteristics and answers to a series of questions. The authors state that IVR systems should automatically transfer the customer to a second-stage agent if its estimation of the probability that the customer cannot be assisted by the IVR system surpasses a certain threshold value. However it is necessary to dynamically change the value of the threshold used according to the congestion level of the agents. Raising this threshold could decrease agent workload, yet it might also escalate customer frustration. The authors argue that the design of call centers must provide the flexibility to choose between two service modes of the IVR system with different performance indicators. When a call comes in, the manager can assign a certain IVR service mode to this customer based on system congestion. This solution allows minimizing the total cost associated with the staff (reduction by approximately 8%) but also reducing the dropout rate.
Although the literature on call centers addresses homogeneous service, i.e., all agents have the same function and process the same type of calls (Avdagić-Golub et al., 2020), Sánchez-Hevia et al. (2022) believe that the effectiveness of call routing to the agent can be improved if machine learning systems are implemented to obtain biometric data from the customer’s voice, such as the caller’s age, gender and emotional state. The authors analyzed deep neural networks of different sizes to gain insight into how the performance in estimating caller age and gender depends on the network architecture and the number of free parameters. It was found that the most efficient neural networks for the proposed application are the feed-forward ones, and the larger size of the networks determines a higher performance. In another study researchers combined a fundamental frequency detection (PD) extractor based on Yet Another Algorithm for Pitch Tracking (YAAPT) and a voice classifier to identify gender and divide callers into adults and children. The experimental results demonstrated that the proposed classifier is promising in terms of coverage, precision, and accuracy (Lin et al., 2023).
Biometric data, however, can be used not only for call routing but also for authentication, so that callers no longer must answer certain questions to authenticate. Kao and Chueh (2023) applied biometric authentication technology to the IVR system to achieve a smart IVR. To achieve high accuracy, the researchers followed a method based on dynamic time warping (DTW) to achieve speaker identification. Also, to avoid potential errors, it is necessary to filter the noises from the speech signals and delete any expressions that could negatively affect the recognition results. Based on the experimental findings, the method suggested in this study demonstrates a high recognition rate and has the potential to be used to train a virtual assistant in customer relationships. Das et al. (2016) developed a multi-level voice authentication system using VP (voice-to-password), TD (text-dependent speaker verification), and TI (text-independent speaker verification) to enable remote authentication through an IVR system.
If the studies presented above talk about data-based routing, other studies emphasize skill-based routing (SBR). The latter routing method can adversely affect the quality of the customer experience because of the complexity and duration of the process. If this is not implemented properly, it will often result in calls being routed to the wrong agent and the caller will have to describe the issue multiple times in order to reach the appropriate agent. Avdagić-Golub et al. (2020) propose applying machine learning methods based on past experience to match customers and agents, aiming to reduce time and connect customers with agents possessing suitable problem-solving skills. The authors used four machine learning algorithms for call classification: Naive Bayes, Decision Tree, Random Forest, and Support Vector Machine (SVM) and found that the best results were obtained by using the Random Forest method which, out of the 66 tested records correctly assigned 59 users to the right agent, with an accuracy of 89.39%. The percentage of correct predictions would be higher if a larger data set were used that would allow for stronger links between the caller’s words and the assigned agent type.
Routing frequently employs touch-tone interfaces (TUIs) in an IVR system, but the interaction between the user and the system is typically quite restricted. Therefore, having a call routing system that accepts natural language input from users and prompts for additional information to fulfill their requests, similar to a human agent, is preferable. The development of core technologies for Natural Language Call Routing (NLCR) applications enables call centers to automatically route callers to the desired destination based on natural voice responses to an open request (Kuo et al., 2003). An NLCR system integrates several essential technologies, primarily automatic speech recognition (ASR) and subject identification. Lee et al. (2000) propose a dialog-based call routing system that aims to eliminate the need for long IVR menus, encourage user intuition through friendlier natural language interactions, initiate dialog sessions to resolve ambiguities, and use machine learning of language model parameters. The authors cite the unique nature of the system’s ability to derive an automatic dialogue from the user’s initial utterance, setting it apart from other existing systems, with laboratory testing indicating a routing accuracy of approximately 89%. However, further research is needed to address issues such as dynamically updating the system when destinations are added or removed, and managing the ambiguity resolution dialog effectively.
An essential aspect of the development of IVR systems is managing waiting queues. Azadeh et al. (2018) investigated the failures occurring in the IVR systems of two companies and observed that in the case of both firms, the failure of the systems had an exponential distribution. These failures affect servers, and customers are removed from queues, and call transfers to agents fail. The frequency of system failures can be reduced by improving equipment but will increase costs and may not be feasible. Therefore, the authors developed a G/G/K queuing system, which simultaneously optimizes operator cost, lost sales cost, consolidation cost, customer time in the system, and the number of customers served without interruption. The optimal scenario is selected through data packing analysis.
The articles included in this thematic group emphasize the need for dynamism in IVR design, in such a way that it is possible to modify and manage IVR flows by non-programmers, thus providing an efficient and practical solution. It is also very important to optimize service time, call routing, and waiting queues using evolutionary algorithms, skill-based routing, and biometric recognition methods. Natural Language Call Routing (NLCR) systems are the future, eliminating long IVR menus and encouraging friendly and intuitive interactions. However, continued research is needed to improve the accuracy and efficiency of these systems.
Optimizing IVR flows is critical to improving service quality, operational efficiency, and customer satisfaction within a call center. The integration of advanced technologies and the dynamic approach to IVR design and management are key to creating an efficient and user-friendly environment.
In this thematic group, we have included papers that analyze how effective the IVR is as a data collection tool, as a monitoring and information system used in health services, or for informing the population about various issues. Basically, it is analyzed more from the perspective of outbound calls from the call center.
When conducting empirical studies, an important decision is the choice of method of administering questionnaires: paper, online, by telephone with a human operator, or by telephone using IVR. There is a limited number of studies that have empirically examined the differences between these response methods. French et al. (2018) investigated differences in compliance, data quality, and participant burden when utilizing interactive and online voice-response surveys. For this purpose, a survey was conducted among 107 students on the topic: conflicts between work and school. The first 47 answered the survey via Precision Polling (the service required participants to call in and answer pre-recorded questions), and the remaining 60 answered via the Qualtrics online survey platform. The results show that there are no disparities in compliance rates and the quantity of responses between the two methods. Even though the qualitative responses collected by IVR were longer, no notable difference in clarity was identified compared to those obtained by the other method. On the other hand, online surveys could reduce the time burden, especially as the number of questions increases. Another study (Ndashimye et al., 2022) compared response rates between WhatsApp and IVR systems, using 8,446 contacts in Senegal and Guinea. Response rates for WhatsApp surveys were almost 8% lower than response rates for IVR surveys. Nevertheless, WhatsApp provides higher survey completion rates and significantly lower costs, without introducing additional sample selection compared to IVR.
Automated dialog systems are a promising approach for promoting healthcare, as they can simulate the experience of face-to-face interactions between healthcare staff and patients. IVR systems are used for health counseling to patients, generally using recorded voice output and automatic voice recognition for user input. They have been used to promote certain diets (Sajjadi et al., 2022), promote physical activity (Ainsworth et al., 2020), encourage smoking or alcohol cessation (Reid et al., 2007; Helzer et al., 2008), and relapse prevention (McDaniel and Roesener, 2002), promote medication adherence (Kamal et al., 2018; Simate, 2014), self-treatment management in chronic diseases (Pinna et al., 2007). Zeb et al. (2019) designed an IVR system for self-management of the disease for less literate people with diabetes in Pakistan called “Sugar ka Saathi” (Diabetes Companion).
A recent study (Adjei et al., 2021) attempts to identify the factors that determine and moderate mIVR system use among caregivers of sick children in a rural setting (a district in Ghana) using the Unified Theory of Acceptance and Use of Technology (UTAUT) model. The mIVR system was developed to provide real-time data on common symptoms of childhood illnesses based on caregivers’ responses to a series of questions. A structured closed-ended questionnaire was used to collect data from 354 caregivers of children under 5 years of age in rural communities for a period of 4 months following the system’s introduction. The results show that only 28.5% of caregivers used the system. Level of education, mobile phone experience, and household wealth were correlated with system use. Behavioral intention to use the mIVR system (92.7% expressed this intention) was positively influenced by the perceived utility of the system, ease of operation, social influences, and enabling conditions. Another study (Acquah-Gyan et al., 2022), also based on the same IVR system in Ghana, focused on exploring user experiences. 35 users who used the IVR system for at least half a year participated in the study, they were recruited through convenience sampling. Their experience was analyzed through in-depth interviews and focus groups. It was found that the caregivers’ attitude towards the system is positive, as it is useful for improving access to healthcare. However, the poor quality of the telephone network, unstable electricity supply, and dropped calls were perceived as notable barriers to system usage.
Regarding automated calls received by patients to remind them to take their treatment or to analyze their progress, it was found that patients prefer easy-to-apply content, receive these calls in the evening, and last less than 5 min per call. People also prefer robocalls over SMS to deliver information (Mathur et al., 2019). Dialogue systems used for health services offer many advantages, but their design involves certain difficulties. Unlike other dialog systems, in healthcare, the validity and accuracy of data are critical, as well as the confidentiality of information. At the same time, continuity in several interactions is very important, especially if weeks or even months of counseling are needed (Bickmore et al., 2018). To minimize errors that can occur in dialog systems, Bickmore et al. (2018) present a series of IVR system design recommendations as follows:
• Structuring user input. Throughout the dialogue, the system should effectively communicate the available options to users. It can provide examples of expected responses to guide user input. In situations where accuracy is crucial, user input should be completely restricted to certain options.
• Reduction of ASR errors. Its accuracy is highly dependent on the acoustic and linguistic models used.
• Detection and recovery of errors in natural language understanding either through explicit confirmation, which requires the system to ask a direct verification question, or through implicit confirmation, which requires the system to display what it has understood in an indirect way.
• Facilitating the interpretation of system responses by the user. Current conversational assistants, such as Siri, provide information based on web searches, but interpreting this information can be difficult for the user, especially if they have limited medical literacy. While doctors can apply various strategies to ensure that the patient has correctly understood the received advice, this is more difficult when interacting with virtual assistants.
The IVR is also used to provide agricultural advice. A field experiment conducted among approximately 4,000 smallholder maize farmers in Urgada sought to highlight the effectiveness of an ICT-mediated approach to providing agricultural information to farmers. The results show that watching videos on how to become better farmers led to a 10.5% increase in yield, but supplementing these videos with an interactive voice response service had no incremental effect. A positive effect of IVR was found only on the use of a single input: hybrid maize seed. However, the results were also affected by the small number of farmers in the sample who were invited to call the IVR (less than 10%) (Van Campenhout et al., 2021).
An interesting use of the IVR is to give rewards in the form of mobile network credit or an amount that allows it to communicate if the user listens to an advertisement to the end. Thus, telecommunications operators become a distribution channel for advertisements, while giving everyone the opportunity to make calls even if they cannot pay for the services. Ndiaye and his collaborators present a proposal for the development and implementation of an IVR solution using Asterisk and AGI (Asterisk Gateway Interface) to handle calls and route users to an interactive voice interface. Processing and validating mobile credit requests requires the integration of a Flask RESTFul application. The authors argue that it is important to create a simplified user experience for customers through IVR that allows them to apply for credit and receive real-time feedback. This solution can later be adapted and extended for other scenarios and applications within telephone networks (Ndiaye et al., 2020). Another study shows the use of IVR to raise awareness about the significance of voting in India, providing the same benefits as in the previously presented study. People can call a phone number and answer some questions about voting. If they answer correctly, they get extra mobile credit (mobile airtime top-up), otherwise, they get an explanation of the correct answer. In 24 days, more than 1900 people used the IVR system, of which 1,245 answered the questions correctly on the first try, and 234 respondents answered correctly after learning from their initial incorrect answers (Kommiya Mothilal et al., 2019).
IVR is also used as an educational resource to reduce low levels of childhood literacy. A study in a village in Côte d’Ivoire demonstrated that even if the parents have low literacy, a complex support network is created in the community to help children use IVR systems (Madaio et al., 2019).
Studies show that IVR systems are an effective method of administering questionnaires and collecting medical data. However, it is important that the interaction with the user is clearly structured, emphasizing error reduction methods and facilitating the interpretation of system responses by users. It is observed that IVR systems are used in many contexts both to facilitate communication and to provide information with quite good efficiency.
In this topic group, we have included articles that refer to recent developments in human-computer / human-robot interaction. Voice-based artificial intelligence (AI) systems have recently been implemented to supersede traditional IVR systems used in call centers. However there is little data on how this change affects customer behavior and service performance.
Using data from a natural experiment at a big Chinese telecommunications company serving more than 3 million customers, Wang et al. (2023) performed an econometric analysis to identify the impact that the introduction of a voice-based AI system has on call duration, customer demand for human services and the number of customer complaints. Interacting with an AI system involves customers verbalizing requests succinctly, and the system provides instant responses to them. If the requests are not clearly described, the AI system asks specific questions to obtain more information. If the AI system cannot adequately respond to their requests, customers have the option of being transferred to a human operator. The researchers found that implementing the AI system increases the duration of the automated service and customer demand for a transfer to a human agent, but this increase is temporary. On the other hand, customer complaints are considerably reduced, especially for elderly and female customers, as well as for frequent users of IVR systems. Over time, customers seem to learn from previous interactions with the AI system, and complaints are further reduced. Over time, customers seem to learn from their previous experience interacting with the AI system and complaints are further reduced. But what actually leads to the increased demand for human services are voice recognition errors. At the same time, the study points out that, compared to the IVR system, the AI system improves the flexibility of service flows, with customers able to get the service they need much more easily and transfer to a human agent whenever they want.
Hoang et al. (2023) present one of the first solutions applying AI technologies to a VoIP (Voice Protocol over Internet) Private Branch Exchange (PBX) in Vietnam. The study uses an open-source VoIP PBX (specifically Asterisk) built on the Google Cloud platform, with the benefits of cost savings, increased flexibility, security, and stability compared to complex infrastructure design, system operation, and maintenance. Central processing unit (CPU) parameters were found to have the greatest impact on the system and adding call logging reduced capacity per call by 25% if there were 60 to 80 concurrent calls. If added to the system with the G.729 codec, then the required capacity is reduced by 50%. The proposed solution is intended for “inbound” calls to the PBX according to customer needs, being much more complex than solutions for “outbound” calls. At the same time, the proposed solution is much more efficient than the existing traditional PBXs that are popular in the market, improving both the customer and employee experience.
As for speech emotion estimation, this is a relatively new topic, with little research in this area, but this feature can improve the functionality of call centers. Emotion changes articulation, phonation, and breathing in human speech, and these changes can be measured empirically. Unfortunately, most studies focus on the analysis of speech performed by actors rather than natural conversations in everyday life, where emotions may not be so obvious. Chakraborty et al. propose a method for emotion recognition with memory using not only real-time conversation but also knowledge of previous events. The model was tested on IVR calls in different languages (Hindi, English, Marathi) and the findings demonstrate that is possible to identify emotions in natural speech if the knowledge that led to that speech is used (Chakraborty et al., 2015).
At this point, if all human agents are busy and the caller requests to be picked up by an operator, the caller will be placed in the waiting queue regardless of their urgency. Bojanić et al. (2020) believe that emotion detection by IVR is even more important in emergency call centers because calls can be prioritized or not based on emotional states. Once emotions are identified, higher priority can be given to calls displaying emotions such as fear, anger, and sadness, and lower priority to calls displaying neutral and happy speech, as these callers are better able to tolerate a longer hold time. The results of an experimental study carried out in a simulated call center show that the waiting time for calls considered to be urgent can be significantly reduced, especially in the case of callers who show emotions of fear and anger. Indeed, the authors used an actor-recorded corpus of verbal expressions of emotions and attitudes in Serbian (GEES), and probably if expressions from real conversations were used, emotions would be more difficult to detect.
The quality of service provided in a call center can be improved if problematic calls are detected and analyzed, and the IVR system is adapted to manage such calls. Schmitt et al. (2008) provide a supervised machine-learning approach for identifying problematic dialogues between a caller and an automated agent. The proposed model is based on a corpus of 69,000 dialogues and is able to distinguish difficult calls after only 5 caller inputs with 90% accuracy.
Another concern relates to the flexibility of interacting with an automated agent. Buck et al. (2018) argue that currently dialog-based systems that support natural language communication are limited in terms of flexibility, with human-system interaction being reduced to tasks of requesting actions and searching for information. They developed a toolkit for automatically building mixed-initiative dialogue systems using a bag-of-words model and a k-nearest-neighbor classifier, allowing the two participants in the action to be equal. As a case study, the authors use natural language ordering at Subway. The proposed method allows mixed initiative, with the user having the possibility to provide several answers to a single question, as well as to request information from the system instead of answering a question addressed to him. The system is trained to extract the additional information provided by the user and process it, thus reducing redundancies.
However, developed the technology used in the call center may be, the most important aspect that managers must consider is the users’ perception of the interaction with the IVR system. An exploratory study of how the US public perceives interaction with the IVR when calling a call center highlights that people perceive the interactive voice response technology used in the call center to be configured in a way that discourages them from continuing the call, but most of the time people are not willing to give up the objectives that originally motivated them to call the call center, wanting at all costs to be taken over by a human operator, who has the role of final arbiter. The authors found that people are frustrated that interacting with the IVR prolongs the call, there are some difficulties in correctly routing to the right agents, and “the bot has no sense of urgency.” Therefore, the IVR bot needs to be personified in a humanistic way, because customers feel that it is less concerned or empathetic than a human being, who can be influenced to help them even if they bend the rules (Walsh et al., 2018).
Different types of questionnaires are used to assess the experience users have had with the IVR. Lewis and Hardzinski (2015) investigated the psychometric properties of these questionnaires. In the 2000s, the Subjective Assessment of Speech System Interfaces (SASSI) was frequently used, a questionnaire containing 34 questions, which evaluates the accuracy of the system’s response (9 items), aggregability (9 items), cognitive demand (5 items), annoyance (5 items), ease of use (5 items) and speed (2 items). But because it focused on the quality of the speech input and less on the quality of the service provided by the company, Polkosky developed the SUISQ (Speech User Interface Service Quality) questionnaire to assess the key usability attributes of IVR applications. The questionnaire contains 25 questions that assess the user’s goal orientation (8 items), customer service behaviors (8 items), speech characteristics (5 items), and verbosity (4 items). Lewis and Hardzinski (2015) developed a shorter version of the SUISQ questionnaire with only 14 items, preserving the psychometric properties of the original questionnaire.
The introduction of voice-based AI systems is gradually replacing traditional interactive voice response (IVR) systems. This change brings significant changes in customer behavior and service performance. If at first people are reluctant and call duration and customer demand for human services increase, over time customers seem to adapt to interacting with AI systems, reducing the number of complaints, but speech recognition errors can generate additional requests for human services. Introducing AI technology into IVR systems brings benefits in cost savings, increased flexibility, and improved customer and employee experience.
The scientometric analysis carried out in this paper highlighted four research directions that may be difficult to observe through “close reading”: 1. Automatic Speech Recognition (ASR); 2. IVR Flow Optimization papers; 3. Reliability of IVR systems as a methodology for studies; 4. Human-Computer Interaction for Development (HCI4D). Reviewing the major topic areas reveals the complexity and diversity of research in speech recognition and human interaction with computer systems. Specific issues related to ambient noise and speech recognition in lesser-used languages are highlighted, which are currently challenging researchers and developers. In line with other literature review research (Alharbi et al., 2021), the need to improve speech recognition accuracy in different environments is emphasized. Advanced models such as Time-Delay Neural Networks (TDNN) have shown promise in improving speech recognition accuracy.
The literature acknowledges the importance of optimizing IVR flows for efficiency (Shaikh and Giannakopoulos, 2024), but existing review studies lack a detailed analysis of dynamic routing strategies. This study highlights emerging solutions like evolutionary algorithms, skill-based routing, and biometric recognition, which can significantly enhance service quality and user satisfaction. IVR optimization strategies and error correction mechanisms reflect ongoing efforts to improve the user experience and performance of voice inquiry systems. It is critical to improve call routing efficiency, reduce waiting times, and improve user interaction through dynamic and easily modifiable IVR flows. The scientometric findings indicate an increasing trend in using IVR for research and data collection, such as monitoring patients’ health. This marks a shift from traditional applications to more diverse and interdisciplinary uses.
Developments in the field of human-computer interaction bring several benefits and challenges, and effective implementation of AI technologies requires a deep understanding of user needs and perceptions, as well as constant adaptability to changes in business and technology. Similar to the review conducted by Shah et al. (2023), the scientometric analysis also highlighted the importance of improving human-computer interaction through NLP and AI-driven enhancements. It must be highlighted that the introduction of voice-based AI systems is gradually replacing traditional interactive voice response (IVR) systems and brings benefits in cost savings, increased flexibility, and improved customer and employee experience. Improving the human-computer relationship could be achieved by detecting emotions in customer speech, identifying calls deemed more urgent, such as those expressing fear or anger, and identifying problematic calls to transfer directly to human agents. It is also important to build dialogue systems with mixed initiatives to allow the user and the system to be equal participants in an action. It is essential to consider the users’ perception of the interaction with the IVR in the development and implementation of these systems. Questionnaires such as the SUISQ are used to assess IVR service quality, focusing on user goal orientation, customer service behaviors, and other key usability attributes.
This paper provides a comprehensive overview of the intellectual structure of literature and emerging trends in the field of Interactive Voice Response (IVR) technology used in call centers. This analysis can guide future research and have practical applications for call center managers. On the one hand, analysis helps researchers identify research opportunities, avoid redundant efforts, and focus on areas with the greatest potential. Moreover, the paper provides managers with essential information needed to make informed decisions regarding investments in IVR technology. The paper highlights the benefits of the IVR system such as reduced costs and improved service efficiency, as well as challenges such as user adaptation and the need for continuous system refinement.
Emerging topics that could constitute future research directions are: the development of noise reduction/elimination methods for automatic speech language recognition with greater accuracy; reducing the word error rate; use of artificial intelligence; classifying IVR users according to emotions; using IVR as a methodology to monitor patients’ health status; machine learning and operations management. Therefore, future research should focus on identifying noise reduction techniques and error correction mechanisms, AI integration in IVR systems, development of user-friendly, adaptable IVR flows that can be managed by non-programmers such as call center managers and developing IVR technology to monitor population health. There is an increased concern for the development of voice query systems in multiple languages, with technologies and methods adapted to the specifics of each language and environment of use.
While this study offers a robust scientometric perspective, it has certain limitations. The analysis was conducted by a single researcher and the set of documents selected for scientometric research might be different if other sampling strategies were used. Overall, this study successfully identifies current and future research directions in the application of IVR technology within call centers, providing a solid foundation for both scholars and industry practitioners, and future studies should continue to build on these findings, addressing the identified challenges in the field of using IVR technology in call centers.
The raw data supporting the conclusions of this article will be made available by the authors, without undue reservation.
EC: Conceptualization, Data curation, Formal analysis, Funding acquisition, Investigation, Methodology, Project administration, Resources, Software, Supervision, Validation, Visualization, Writing – original draft, Writing – review & editing.
The author(s) declare that no financial support was received for the research and/or publication of this article.
The author declares that the research was conducted in the absence of any commercial or financial relationships that could be construed as a potential conflict of interest.
All claims expressed in this article are solely those of the authors and do not necessarily represent those of their affiliated organizations, or those of the publisher, the editors and the reviewers. Any product that may be evaluated in this article, or claim that may be made by its manufacturer, is not guaranteed or endorsed by the publisher.
The Supplementary material for this article can be found online at: https://www.frontiersin.org/articles/10.3389/fcomp.2025.1459787/full#supplementary-material
Acquah-Gyan, E., Acheampong, P. R., Mohammed, A., Adjei, T. K., Agyapong, E., Twumasi-Ankrah, S., et al. (2022). User experiences of a mobile phone-based health information and surveillance system (mHISS): a case of caregivers of children under-five in rural communities in Ghana. PLoS One 17:e0261806. doi: 10.1371/journal.pone.0261806
Adjei, T. K., Mohammed, A., Acheampong, P. R., Acquah-Gyan, E., Sylverken, A., Twumasi-Ankrah, S., et al. (2021). Determinants of a mobile phone-based interactive voice response (mIVR) system for monitoring childhood illnesses in a rural district of Ghana: empirical evidence from the UTAUT model. PLoS One 16:e0248363. doi: 10.1371/journal.pone.0248363
Ainsworth, M. C., Rogers, L. Q., Perumean-Chaney, S. E., Thirumalai, M., Brown, N., Jackson, E. A., et al. (2020). Effects of interactive voice response (IVR) counseling on physical activity benefits and barriers. Health Behav. Policy Rev. 7, 407–415. doi: 10.14485/HBPR.7.5.3
Alharbi, S., Alrazgan, M., Alrashed, A., Alnomasi, T., Almojel, R., Alharbi, R., et al. (2021). Automatic speech recognition: systematic literature review. IEEE Access 9, 131858–131876. doi: 10.1109/ACCESS.2021.3112535
Andrade, R., and Moazeni, S. (2023). Transfer rate prediction at self-service customer support platforms in insurance contact centers. Expert Syst. Appl. 212:118701. doi: 10.1016/j.eswa.2022.118701
Avdagić-Golub, E., Begović, M., and Kosovac, A. (2020). Optimization of agent-user matching process using a machine learning algorithms. TEM J. 9, 158–163. doi: 10.18421/TEM91‐22
Azadeh, A., Lhoseiny, M. S. N., and Salehi, V. (2018). Optimum alternatives of tandem G/G/K queues with disaster customers and retrial phenomenon: interactive voice response systems. Telecommun. Syst. 68, 535–562. doi: 10.1007/s11235-017-0397-x
Bhardwaj, V., Ben Othman, M. T., Kukreja, V., Belkhier, Y., Bajaj, M., Goud, B. S., et al. (2022). Automatic speech recognition (asr) systems for children: a systematic literature review. Appl. Sci. 12:4419. doi: 10.3390/app12094419
Bhat, C., Mithun, B.M., Saxena, V., Kulkarni, V., and Kopparapu, S.K. (2013). “Deploying usable speech enabled IVR systems for mass use.” in 2013 International Conference on Human Computer Interactions (ICHCI). pp. 1–5.
Bickmore, T., Trinh, H., Asadi, R., and Olafsson, S. (2018). “Safety first: Conversational agents for health care,” in Studies in Conversational UX Design. Human–Computer Interaction Series. eds. R. Moore, M. Szymanski, R. Arar, and G. J. Ren (Cham: Springer), 2018, 33–57.
Bojanić, M., Delić, V., and Karpov, A. (2020). Call redistribution for a call center based on speech emotion recognition. Appl. Sci. 10:4653. doi: 10.3390/app10134653
Buck, J.W., Perugini, S., and Nguyen, T.V. (2018). “Natural language, mixed-initiative personal assistant agents.” in Proceedings of the 12th International Conference on Ubiquitous Information Management and Communication. pp. 1–8.
Chakraborty, R., Pandharipande, M., and Kopparapu, S. (2015). “Event based emotion recognition for realistic non-acted speech.” in TENCON 2015–2015 IEEE Region 10 Conference. pp. 1–5.
Colladon, A. F., Naldi, M., and Schiraldi, M. M. (2013). Quality Management in the Design of TLC call Centres. Int. J. Eng. Bus. Manag. 5:48. doi: 10.5772/56921
Das, R. K., Jelil, S., and Mahadeva Prasanna, S. R. (2016). Development of multi-level speech based person authentication system. J. Sig. Proces. Syst. 88, 259–271. doi: 10.1007/s11265-016-1148-z
Duggirala, M., Kambhatla, N., Polavarapu, R., and Garg, D., (2011). “An integrated framework of service quality for global delivery of contact center services.” in 2011 Annual SRII Global Conference. pp. 557–564.
French, K. A., Falcon, C. N., and Allen, T. D. (2018). Experience sampling response modes: comparing voice and online surveys. J. Bus. Psychol. 34, 575–586. doi: 10.1007/s10869-018-9560-y
Haghani, M. (2023). What makes an informative and publication-worthy scientometric analysis of literature: a guide for authors, reviewers and editors. Trans. Res. Interdiscip. Persp. 22:100956. doi: 10.1016/j.trip.2023.100956
Hamidi, M., Satori, H., Zealouk, O., and Satori, K. (2020). Amazigh digits through interactive speech recognition system in noisy environment. Int. J. Speech Technol. 23, 101–109. doi: 10.1007/s10772-019-09661-2
Helzer, J. E., Rose, G. L., Badger, G. J., Searles, J. S., Thomas, C. S., Lindberg, S. A., et al. (2008). Using interactive voice response to enhance brief alcohol intervention in primary care settings. J. Stud. Alcohol Drugs 69, 251–258. doi: 10.15288/jsad.2008.69.251
Hoang, H. S., Tran, A. K., Doan, T. P., Tran, H. K., Dang, N. M. D., and Nguyen, H. N. (2023). Design and implementation of a VoIP PBX integrated Vietnamese virtual assistant: a case study. J. Inform. Telecommun. 7, 201–226. doi: 10.1080/24751839.2023.2183631
Inam, I.A., Azeta, A.A., and Daramola, O. (2017). “Comparative analysis and review of interactive voice response systems.” in 2017 Conference on Information Communication Technology and Society (ICTAS). pp. 1–6.
Kamal, A. K., Khalid, W., Muqeet, A., Jamil, A., Farhat, K., Gillani, S. R. A., et al. (2018). Making prescriptions ‘talk’ to stroke and heart attack survivors to improve adherence: results of a randomized clinical trial (the talking Rx study). PLoS One 13:e0197671. doi: 10.1371/journal.pone.0197671
Kao, C.-Y., and Chueh, H.-E. (2023). Voice response questionnaire system for speaker recognition using biometric authentication Interface. Intellig. Autom. Soft Comp. 35, 913–924. doi: 10.32604/iasc.2023.024734
Karademir, R., and Heves, E. (2013). “Dynamic interactive voice response (IVR) platform.” in Eurocon 2013. IEEE. pp. 98–104.
Khan, S., Basu, J., Bepari, M.S., and Roy, R. (2013). “Evaluation and error recovery methods of an IVR based real time speech recognition application.” in 2013 International Conference Oriental COCOSDA Held Jointly with 2013 Conference on Asian Spoken Language Research and Evaluation (O-COCOSDA/CASLRE). pp. 1–6.
Khomitsevich, O., Mendelev, V., Tomashenko, N.A., Rybin, S., Medennikov, I., and Kudubayeva, Saule (2015). “A bilingual Kazakh-Russian system for automatic speech recognition and synthesis.” in Speech and Computer: 17th International Conference, SPECOM 2015, Athens, Greece, September 20–24, 2015, Proceedings 17. Springer International Publishing. pp. 25–33.
Khudyakov, P., Feigin, P. D., and Mandelbaum, A. (2010). Designing a call center with an IVR (interactive voice response). Queueing Syst. 66, 215–237. doi: 10.1007/s11134-010-9193-y
Kommiya Mothilal, R., Mehta, D., Sharma, A., Thies, W., and Sharma, A. (2019). “Learnings from an ongoing deployment of an IVR-based platform for voter awareness.” in Conference Companion Publication of the 2019 on computer Supported Cooperative Work and Social Computing. pp. 257–261.
Kumalija, E., and Nakamoto, Y. (2022). Performance evaluation of automatic speech recognition systems on integrated noise-network distorted speech. Front. Sig. Proces. 2:999457. doi: 10.3389/frsip.2022.999457
Kuo, H.-K. J., Siohan, O., and Olive, J. P. (2003). Advances in natural language call routing. Bell Labs Tech. J. 7, 155–170. doi: 10.1002/bltj.10040
Lee, C.-H., Carpenter, B., Chou, W., Chu-Carroll, J., Reichl, W., Saad, A., et al. (2000). On natural language call routing. Speech Comm. 31, 309–320. doi: 10.1016/S0167-6393(99)00064-3
Lemon, K. N., and Verhoef, P. C. (2016). Understanding customer experience throughout the customer journey. J. Mark. 80, 69–96. doi: 10.1509/jm.15.0420
Lewis, J. R., and Hardzinski, M. L. (2015). Investigating the psychometric properties of the speech user Interface Service quality questionnaire. Int. J. Speech Technol. 18, 479–487. doi: 10.1007/s10772-015-9289-1
Lin, C.-H., Lai, H.-Y., Huang, P.-T., Chen, P., and Li, C. (2023). Vowel classification with combining pitch detection and one-dimensional convolutional neural network based classifier for gender identification. IET Sig. Proces. 17:12216. doi: 10.1049/sil2.12216
Lovrenčič, T., Štular, M., Kačič, Z., and Žgank, A. (2015). QoS estimation and prediction of input modality in degraded ip networks. Wirel. Pers. Commun. 80, 837–857. doi: 10.1007/s11277-014-2044-0
Madaio, M., Kamath, V., Yarzebinski, E., Zasacky, S., Tanoh, Fabrice, Hannon-Cropp, J., et al. (2019). “You give a little of yourself: family support for children’s use of an IVR literacy system.” in Proceedings of the 2nd ACM SIGCAS Conference on Computing and Sustainable Societies. pp. 86–98.
Mathur, A., Bhowmick, Shimmila, and Sorathia, K. (2019). “A study of outbound automated call preferences for DOTS adherence in rural India.” In Human-Computer Interaction–INTERACT 2019: 17th IFIP TC 13 International Conference, Paphos, Cyprus, September 2–6, 2019, Proceedings, Part III 17. Springer International Publishing. pp. 24–33.
McDaniel, A.M., and Roesener, G.H. (2002). Interactive voice response Technology for Smoking Cessation Relapse Prevention. Proceedings of the AMIA Symposium. p. 1101. Available online at: https://www.ncbi.nlm.nih.gov/pmc/articles/PMC2244163/ (Accessed January 19, 2023).
Mehta, D., Santy, S., Mothilal, R.K., Srivastava, B.M.L., Sharma, A., Shukla, A., et al. (2021). Learnings from technological interventions in a low resource language: a case-study on Gondi. Available online at: arXiv.org.
Mocanu, B.-C., Filip, I.-D., Ungureanu, R.-D., Negru, C., Dascalu, M., Toma, S.-A., et al. (2022). Odin ivr-interactive solution for emergency calls handling. Appl. Sci. 12:10844. doi: 10.3390/app122110844
Ndashimye, F., Hebie, O., and Tjaden, J. (2022). Effectiveness of WhatsApp for measuring migration in follow-up phone surveys. Lessons from a mode experiment in two low-income countries during COVID contact restrictions. Soc. Sci. Comput. Rev. 42, 460–479. doi: 10.1177/08944393221111340
Ndiaye, L., Gueye, K., Degboe, Bessan Melckior, and Ouya, S. (2020). “Proposal of an IVR solution and granting of credit with Asterisk’s AGI and a flask RESTFul framework in an IMS network: case of an advertisement.” in 2020 22nd International Conference on Advanced Communication Technology (ICACT). pp. 254–360. IEEE.
Nicmanis, D., and Salimbajevs, A. (2022). Spoken dialogue system for call centers with expressive speech synthesis. In Proc. Interspeech 2022, pp. 5215–5216. Available online at: https://www.isca-archive.org/interspeech_2022/nicmanis22_interspeech.pdf (Accessed March 19, 2024).
Pinedo, M., Seshadri, S., and Shanthikumar, J. G. (2000). “Call centers in financial services: strategies, technologies, and operations” in Creating value in financial services. eds. E. L. Melnick, P. R. Nayyar, M. L. Pinedo, and S. Seshadri (Boston, MA: Springer US), 357–388.
Pinna, G. D., Maestri, R., Andrews, D., Witkowski, T., Capomolla, S., Scanferlato, J. L., et al. (2007). Home telemonitoring of vital signs and cardiorespiratory signals in heart failure patients: system architecture and feasibility of the HHH model. Int. J. Cardiol. 120, 371–379. doi: 10.1016/j.ijcard.2006.10.029
Porter, A. L., Kongthon, A., and Lu, J.-C. (2002). Research profiling: improving the literature review. Scientometrics 53, 351–370. doi: 10.1023/a:1014873029258
Praveen Kumar, P. S., Thimmaraja Yadava, G., and Jayanna, H. S. (2020). Continuous Kannada speech recognition system under degraded condition. Circ. Syst. Sig. Proces. 39, 391–419. doi: 10.1007/s00034-019-01189-9
Reid, R. D., Pipe, A. L., Quinlan, B., and Oda, J. (2007). Interactive voice response telephony to promote smoking cessation in patients with heart disease: a pilot study. Patient Educ. Couns. 66, 319–326. doi: 10.1016/j.pec.2007.01.005
Rimol, M. (2022). Gartner predicts conversational AI will reduce contact center agent labor costs by $80 billion in 2026. Gartner. Available online at: https://www.gartner.com/en/newsroom/press-releases/2022-08-31-gartner-predicts-conversational-ai-will-reduce-contac (Accessed February 4, 2025).
Saberi, M., Khadeer Hussain, O., and Chang, E. (2017). Past, present and future of contact centers: a literature review. Bus. Process Manag. J. 23, 574–597. doi: 10.1108/BPMJ-02-2015-0018
Sajjadi, P., Edwards, C. G., Zhao, J., Fatemi, A., Long, J. W., Klippel, A., et al. (2022). Remote iVR for nutrition education: from design to evaluation. Front. Comp. Sci. 4:927161. doi: 10.3389/fcomp.2022.927161
Salcedo-Sanz, S., Naldi, M., Pérez-Bellido, Á. M., Portilla-Figueras, A., and Ortíz-García, E. G. (2010). Evolutionary optimization of service times in interactive voice response systems. IEEE Trans. Evol. Comput. 14, 602–617. doi: 10.1109/TEVC.2009.2039142
Sánchez-Hevia, H. A., Gil-Pita, R., Utrilla-Manso, M., and Rosa-Zurera, M. (2022). Age group classification and gender recognition from speech with temporal convolutional neural networks. Multimed. Tools Appl. 81, 3535–3552. doi: 10.1007/s11042-021-11614-4
Schmitt, A., Hank, C., and Liscombe, J. (2008). Detecting problematic dialogs with automated agents. Lect. Notes Comput. Sci. 5078, 72–80. doi: 10.1007/978-3-540-69369-7_9
Shahnawazuddin, S., Thotappa, D., Dey, A., Imani, S. M., Prasanna, S. R., and Sinha, R. (2017). Improvements in IITG Assamese spoken query system: background noise suppression and alternate acoustic modeling. J. Sig. Proces. Syst. 88, 91–102. doi: 10.1007/s11265-016-1133-6
Shah, S., Ghomeshi, H., Vakaj, E., Cooper, E., and Fouad, S. (2023). A review of natural language processing in contact Centre automation. Pattern. Anal. Applic. 26, 823–846. doi: 10.1007/s10044-023-01182-8
Shaikh, K.M., and Giannakopoulos, G. (2024). Evolution of IVR building techniques: from code writing to AI-powered automation.
Simate, Z. (2014). “Investigating the use of interactive voice response (IVR) in medical adherence monitoring.” in 2014 8th international symposium on medical information and communication technology (ISMICT). pp. 1–4.
Singh, Y. B., and Goel, S. (2022). A systematic literature review of speech emotion recognition approaches. Neurocomputing 492, 245–263. doi: 10.1016/j.neucom.2022.04.028
Suhm, B., and Peterson, P. (2002). A data-driven methodology for evaluating and optimizing call center IVRs. Int. J. Speech Technol. 5, 23–37. doi: 10.1023/A:1013674413897
Tezcan, T., and Behzad, B. (2012). Robust design and control of call centers with flexible interactive voice response systems. Manuf. Serv. Oper. Manag. 14, 386–401. doi: 10.1287/msom.1120.0378
Thirumaran, M., Sivakumar, N., Banupriya, P., and Bhargavi, T. (2016). “Self-regulating interactive voice response system using backpatching technique.” in Proceedings of the International Conference on Informatics and Analytics. pp. 1–8.
Van Campenhout, B., Spielman, D. J., and Lecoutere, E. (2021). Information and communication technologies to provide agricultural advice to smallholder farmers: experimental evidence from Uganda. Am. J. Agric. Econ. 103, 317–337. doi: 10.1002/ajae.12089
Walsh, J., Andersen, B., Katz, J., and Groshek, J. (2018). Personal power and agency when dealing with interactive voice response systems and alternative modalities. Media Commun. 6, 60–68. doi: 10.17645/mac.v6i3.1205
Wang, L., Huang, N., Hong, Y., Liu, L., Guo, X., and Chen, G. (2023). Voice-based AI in call center customer service: a natural field experiment. Prod. Oper. Manag. 32, 1002–1018. doi: 10.1111/poms.13953
Yadava, G. T., Nagaraja, B. G., and Jayanna, H. S. (2023). An end-to-end continuous Kannada ASR system under uncontrolled environment. Multimed. Tools Appl. 83, 7981–7994. doi: 10.1007/s11042-023-15854-4
Yadava, T. G., and Jayanna, H. S. (2017). A spoken query system for the agricultural commodity prices and weather information access in Kannada language. Int. J. Speech Technol. 20, 635–644. doi: 10.1007/s10772-017-9428-y
Yang, S., Yuan, Q., and Dong, J. (2020). Are Scientometrics, Informetrics, and Bibliometrics different? Data Sci. Inform. 1:50. doi: 10.4236/dsi.2020.11003
Zeb, K., Lindsay, S., Shahid, S., Riaz, W., and Jones, M. (2019). “Sugar Ka Saathi–a case study designing digital self-management tools for people living with diabetes in Pakistan.” in Human-computer interaction–INTERACT 2019: 17th IFIP TC 13 international conference, Paphos, Cyprus, September 2–6, 2019, proceedings, part III 17. pp. 161–181. Springer International Publishing.
Keywords: IVR systems, call center, call center management, automatic speech recognition, IVR flow optimization, HCI4D, data collection
Citation: Coman E (2025) IVR systems used in call center management: a scientometric analysis of the literature. Front. Comput. Sci. 7:1459787. doi: 10.3389/fcomp.2025.1459787
Received: 04 July 2024; Accepted: 14 March 2025;
Published: 01 April 2025.
Edited by:
Subarna Shakya, Tribhuvan University, NepalReviewed by:
Makuochi Nkwo, University of Greenwich, United KingdomCopyright © 2025 Coman. This is an open-access article distributed under the terms of the Creative Commons Attribution License (CC BY). The use, distribution or reproduction in other forums is permitted, provided the original author(s) and the copyright owner(s) are credited and that the original publication in this journal is cited, in accordance with accepted academic practice. No use, distribution or reproduction is permitted which does not comply with these terms.
*Correspondence: Ecaterina Coman, ZWNhdGVyaW5hLmNvbWFuQHVuaXRidi5ybw==
Disclaimer: All claims expressed in this article are solely those of the authors and do not necessarily represent those of their affiliated organizations, or those of the publisher, the editors and the reviewers. Any product that may be evaluated in this article or claim that may be made by its manufacturer is not guaranteed or endorsed by the publisher.
Research integrity at Frontiers
Learn more about the work of our research integrity team to safeguard the quality of each article we publish.