- 1Faculty of Applied Sciences, International School, Vietnam National University, Hanoi, Vietnam
- 2Department of Electrical Engineering, National Chung Cheng University, Chiayi, Taiwan
Early and accurate detection of plant diseases is crucial for making informed decisions to increase the yield and quality of crops through the decision of appropriate treatments. This study introduces an automated system for early disease detection in plants that enhanced a lightweight model based on the robust machine learning algorithm. In particular, we introduced a transformer module, a fusion of the SPP and C3TR modules, to synthesize features in various sizes and handle uneven input image sizes. The proposed model combined with transformer-based long-term dependency modeling and convolution-based visual feature extraction to improve object detection performance. To optimize a model to a lightweight version, we integrated the proposed transformer model with the Ghost module. Such an integration acted as regular convolutional layers that subsequently substituted for the original layers to cut computational costs. Furthermore, we adopted the SIoU loss function, a modified version of CIoU, applied to the YOLOv8s model, demonstrating a substantial improvement in accuracy. We implemented quantization to the YOLOv8 model using ONNX Runtime to enhance to facilitate real-time disease detection on strawberries. Through an experiment with our dataset, the proposed model demonstrated mAP@.5 characteristics of 80.30%, marking an 8% improvement compared to the original YOLOv8 model. In addition, the parameters and complexity were reduced to approximately one-third of the initial model. These findings demonstrate notable improvements in accuracy and complexity reduction, making it suitable for detecting strawberry diseases in diverse conditions.
1 Introduction
Detecting diseases in crops, especially in major crops, is a crucial issue in agriculture. Early disease detection and prevention are vital measures to minimize damage to crops and increase productivity. However, detecting diseases on strawberries poses a challenge due to variations in shape, color, and size among different diseases. Traditional methods for strawberry disease detection, reliant on the analyses of agricultural experts, are time-consuming and lack accuracy. With the significant progress in deep learning and computer hardware, advanced image recognition technologies are increasingly employed by scholars for agricultural disease recognition.
Recent applications of deep learning, particularly Convolutional Neural Networks (CNNs), in detecting crop diseases have shown promising results (Mahmud et al., 2019; Jayawardena et al., 2016). Scholars have proposed CNN techniques, such as GoogLeNet (Ferentinos, 2018), for plant leaf identification, achieving recognition rates exceeding 94%, even with partial leaf damage. CNNs have been utilized for discovering crop species and diseases (Ha and Chen, 2021), with reported accuracies of 99.35%. Deep Transfer Learning (DTL) (Chen et al., 2020) has been employed for banana disease detection, reaching an accuracy of 90%. Diverse deep CNN architectures (Cheng et al., 2017), including AlexNet, MobileNet, GoogLeNet, VGGNet, and Xception, have been proposed for inspecting strawberry quality, with reported accuracies of up to 95%. Supervised machine learning technologies (Selvaraj et al., 2019) have also been addressed to recognize strawberry powdery mildew disease with an accuracy of 94.34%. The classification model (Ha et al., 2024) for identifying plant diseases through the integration of local and global features utilizing a transformer-based approach demonstrated very high results with 99.18% and 94.05% accuracy. Object detection techniques, such as Fast RCNN (Mohanty et al., 2016) and Faster-RCNN (Chen et al., 2019; Girshick, 2015; Baweja et al., 2018) have found widespread applications in detecting insect diseases in plants. Additionally, mask R-CNN (Sa et al., 2016) has a demonstrated significant accuracy, especially in fruit discovery for strawberry harvesting.
Beyond strawberries, recent research has significantly expanded the application of object detection models to target plant diseases in various crops. Liu and Wang (2021), in their review “Plant Diseases and Pests Detection Based on Deep Learning,” emphasize the advancements in utilizing deep learning techniques to detect diseases such as powdery mildew (Erysiphe necator) and black spot (Alternaria alternata) in tomatoes and cucumbers, as well as apple scab (Venturia inaequalis) and downy mildew (Plasmopara viticola). Similarly, Shruthi and Nagaveni (2024) demonstrate a hybrid convolutional neural network (CNN) model using self-regulated layers and inception layer for accurate and efficient diagnosis of tomato diseases with severity levels. These studies highlight the promising capabilities of AI systems to revolutionize plant disease detection and management across diverse agricultural application.
In terms of attention mechanisms and transformer-based architectures, have significantly improved the performance of plant disease detection systems. Transformers, initially popularized in natural language processing, have demonstrated exceptional capability in modeling long-range dependencies and multiscale features, making them highly effective for visual tasks. For instance, Gu et al. (2024) proposed the Multi-Modal Fast Gated Transformer (MFGTN), which integrates spatial and temporal data for improved feature fusion, inspiring potential applications in plant disease detection under diverse environmental conditions. Similarly, Song et al. (2024) introduced CenterFormer, a transformer-based model that enhances segmentation accuracy through a cluster center-guided attention mechanism, offering a promising approach for localizing disease-affected regions in plants. These studies highlight the importance of attention mechanisms in achieving precise feature extraction and localization.
In the context of one-stage object detection, the YOLO (You Only Look Once) family, including YOLOv3, YOLOv4, YOLOv5 (Yu et al., 2019; Zhang et al., 2022; Sozzi et al., 2022), and YOLOv7 (Gallo et al., 2023), has shown promising results in detecting diseases in plants, including strawberries. These models efficiently combine feature extraction and prediction, with fair inference time. The evolution of disease detection methodologies, particularly through the integration of advanced deep learning techniques, provides a strong foundation for AI-mediated disease detection in agriculture.
In line with the prevailing trend and its application to the challenge of strawberry leaf disease detection, this work introduces models designed for accuracy enhancement model complexity minimization based on YOLOv8s released in 2023, a state-of-the-art model in single-stage object detection as well as within the YOLO family. Specifically, our contributions to this paper are as follows:
- A new dataset is proposed and collected from high-quality images on Google with farm settings, as well as from the Ministry of Agriculture, Vietnam. Through rigorous preprocessing and adherence to strict criteria regarding color, area, density of the diseased part, and species shape, we curated 1,000 high-quality images, categorizing them into five classes: Normal, Rubber, Gray Mold, Black Spot, and Powdery Mildew.
- We develop the SC3T module to ameliorate model accuracy. Inspired by the transformer module widely used in natural language processing, the SC3T module employs an attention mechanism designed for multiscale processing, effectively handling feature maps at different scales and ensuring accurate detection of objects of various sizes.
- The loss function of CIoU in YOLOv8s is replaced by the SIoU loss function, a variant of CIoU incorporating angle factors. This establishes the basis for inferring costs related to distance, ratio, and intrusion.
- The Ghost convolution module is specifically devised to address limitations in conventional deep neural network models like YOLOv8s, to successfully establish a lightweight model.
- Quantization through ONNX Runtime, leading to a streamlined ONNX file suitable for deployment, is conducted for aiming at enhancing model performance and efficiency.
- Subsequently, our object detection app was developed and demonstrated on Android devices, showcasing the tangible deployment and practical utility of the proposed deep learning model in real-world object detection scenarios.
The subsequent sections of this paper are organized as follows: Section 2 presents an extensive overview of existing methodologies in the literature employed for plant disease detection. Section 3 details the proposed methodology, offering more specific explanations on enhancement features. In Section 4, we illustrate the dataset, training environment, and both quantitative and qualitative results in three aspects: accuracy complexity, and loss functions. Additionally, comparative analyses of our models are addressed on different datasets. Finally, the conclusion is given in Section 5 with a brief summary of this work.
2 Related previous work
2.1 One-stage object detection
Among the prominent one-stage object detection frameworks (Yao et al., 2021), the YOLO series (Redmon et al., 2016) stands out for its real-time performance and unified, efficient architecture, consistently achieving high accuracy and versatility across various applications through iterative advancements. YOLOv1 and YOLOv2, while groundbreaking, relied on a rigid grid-based prediction mechanism that struggled with localization accuracy for small or occluded objects. To address these limitations, YOLOv3 introduced multi-scale feature detection, significantly enhancing object recognition across varying sizes. However, its deeper architecture increased training time and computational costs. Building on these improvements, YOLOv4 incorporated the CSPDarknet53 backbone, further boosting accuracy but at the expense of higher GPU resource requirements. YOLOv5 shifted focus toward lightweight design, achieving faster inference times but lacking advanced feature fusion capabilities, which limited its performance in cluttered environments. YOLOv7 enhanced training efficiency and detection speed, yet it remained dependent on intricate hyperparameter tuning and showed reduced robustness for objects in motion.
YOLOv8 addresses these challenges with significant architectural advancements by integrating the C2f module to enhance multiscale feature aggregation and reduce computational overhead compared to the C3 module used in YOLOv5 and YOLOv7. In addition, YOLOv8 supports ONNX Runtime and TensorFlow Lite, enabling seamless deployment across diverse platforms and enhancing performance in real-time applications. Its lightweight architecture and optimized inference reduce latency, making it particularly well-suited for time-critical tasks. Based on these strengths, we have utilized and implemented the experiments in YOLOV8s.
2.2 Object detection models with accuracy improvement
From foundational models, numerous studies have sought to improve the accuracy performance, notably in the context of YOLO-related research. For instance, in YOLOv3 (Zhao and Li, 2020), this model was introduced to accelerate the rate of convergence when initializing the width and height of the predicted bounding boxes. This method enables the selection of more representative initial dimensions, leading to a significant increase in mAP. Another study (Yao et al., 2020) employed double K-means to generate anchor boxes, aiming to lift localization accuracy. Several investigations have focused on refining the structures within the YOLO's backbone, such as an introduction of the bottleneck CSP-2 module in Yan et al. (2021) or the incorporation of special modules, as seen in Yao et al. (2021), which introduced SELayer (Xu et al., 2021) and integrated EfficientNet into the YOLO architecture.
One promising module inspired by a transformer, a natural language processing model developed by Google, is gaining attention. With its attention mechanism via matrix computations, a transformer can effectively link semantically relevant content. This mechanism performs well with image data, facilitating the correlation of related features. Leveraging this advantage, the adoption of the transformer has demonstrated significant effectiveness in models like YOLOv5s (Zhu et al., 2021; Yu et al., 2021). Referring to these enhancement ideas, we propose the SC3T transformer module to improve mAP accuracy in the state-of-the-art YOLO model, YOLOv8.
2.3 Object detection models with light weights
Enhancing the accuracy of YOLO often increases model complexity, leading to higher Floating-Point Operations per Second (FLOPs). Consequently, the challenge of improving the model in a lightweight direction for high-efficiency hardware computing has become a highly prospective research area. For example, tinier-YOLO (Fang et al., 2019) proposed a lightweight solution for tiny YOLOv3. This involved modifying the SqueezeNet module to reduce the number of model parameters and subsequently decrease the overall model size. Another noteworthy approach is found in Lu et al. (2020), named YOLO-compact, which separates the down-sampling layers from all network modules. This model is 3.7, 6.7, and 26 times smaller than tiny-YOLOv3, tiny-YOLOv2, and YOLOv3, respectively. The Ghost module, applied in YOLOv5 (Dong et al., 2022; Liu et al., 2021; Xu et al., 2022), has demonstrated notable efficiency in reducing computational operations and the number of parameters significantly. Such a module can be a good candidate for lightweight model designs. Recognizing its effectiveness, we explored incorporating the Ghost convolution module into the backbone architecture of YOLOv8s, yielding highly favorable results in experimental evaluations.
2.4 Loss functions
The loss functions serve as a crucial aspect for evaluating the overall model accuracy by calculating the deviation between the actual and predicted objects. This dimension associated with maximizing accuracies in object detection tasks is pivotal. In YOLOv5, the loss function comprises three components:
Classification loss: used to compute the deviation between the predicted probabilities of object classes and the actual ones of object classes in an image.
Localization loss: employed to calculate the accuracy of the predicted bounding box positions concerning the actual ones of objects in an image.
Objectness loss (equivalent to IoU loss): used to determine the accuracy of classifying image pixels as objects or non-objects.
This study focuses on object loss in order to heighten the model's accuracy. Specifically, in YOLOv5, the objectness loss utilized is CIoU loss (Zheng et al., 2021), which considers three factors: the distance between the centers of the predicted and actual boxes, the aspect ratio difference, and the diagonal distance ratio. These special factors are beneficial to improve detection accuracy, especially for small objects. The SIoU loss function, proposed by Gevorgyan (2022) in May 2022, is a variant of CIoU loss, incorporating an angle factor. This addition constructs the basis for inferring distance and ratio factors. Studies associated with replacing CIoU with SIoU have demonstrated promising results in YOLOv5. Inspired by this, we experimented with SIoU in YOLOv8, yielding favorable outcomes.
2.5 Pre-trained model
The early achievements of deep learning in the field of computer vision owe much to transfer learning. The pre-training based on ImageNet played a pivotal role in achieving advancements over state-of-the-art results in various recognition tasks, including object detection (Lin et al., 2017; Liu et al., 2016), semantic segmentation (Chen et al., 2017; He et al., 2017), and scene classification (Zhou et al., 2017; Herranz et al., 2016). The adaptability of pre-trained features has been thoroughly investigated (Azizpour et al., 2015; Cui et al., 2018; Kornblith et al., 2019). For instance, Azizpour et al. (2015) quantified the similarity between tasks using ImageNet classification; Cui et al. (2018) investigated the transfer of nsights gained from large classification datasets have been applied to smaller, detailed datasets; Kornblith et al. (2019) explored the relationship among ImageNet pre-training accuracies, transfer accuracies, and network architectures. In this work, the proposed model was trained on a large dataset, as detailed in Afzaal et al. (2021). Subsequently, we utilized these weights as the pre-trained parameters for further learning on our specific dataset.
3 Proposed model
This work proposes and optimizes models for practical use, specifically object detection models in a typical phase like those in the YOLO family. After training, evaluation, and testing, these models undergo quantization and are integrated into the application software. An overview of our system architecture is depicted in Figure 1. Initially, to assess the effectiveness of the proposed models, we trained them on a large dataset, followed by evaluation and selection of the outperformance candidates based on two criteria: accuracy and lightweight nature. These top models are then saved as pre-training models for the Vietnam strawberries dataset. With the proposed accuracy model, we aim to achieve high accuracy without considering its complexity, while with the lightweight model, we emphasize reducing model complexity as well as accuracy. Subsequently, the lightweight model is quantized to optimize its weights, suitably embedded into edge devices. Specifically, we built an Android app for disease detection on strawberries. To delve into the specifics, we will explore the original YOLOv8 architecture.
From an architectural point of view, YOLOv8s doesn't exhibit many differences from YOLOv5s. A key distinction lies in the integration of the C2f module, which supersedes the C3 module originally employed in YOLOv5. In YOLOv5, the C3 module consists of three standard convolutional layers and several bottleneck blocks. This structure incorporates two branches: one branch utilizes multiple stacked bottleneck blocks and three standard convolutional layers, while the other branch processes a single basic convolutional layer before merging with the first branch. The effective design of the bottleneck module minimizes the number of training parameters and computational load, thus mitigating issues of gradient explosion and vanishing in deep networks, and thereby enhancing the model's learning capabilities. YOLOv7 further refines gradient calculations by introducing multiple parallel gradient branches and implementing the ELAN module, resulting in improved accuracy and more reasonable latency. YOLOv8 designed the C2f module based on the C3 module and ELAN's concept to gather diverse gradient clues while maintaining a lightweight structure.
In Figure 2, YOLOv8s-Transformer is devised based on the basic architecture of YOLOv8s to enhance the accuracy. We have integrated SC3T into the final layer of the backbone to optimize the extracted data at different scales, thereby improving overall model efficiency. Subsequently, these features at various scales are extracted in the head section before moving to the prediction part. Here, we experimented with various loss functions to identify the most suitable one that achieves outstanding performance for our application.
Similar to YOLOv8s-Transformer, as shown in Figure 3, YOLOv8s-Trans-Ghost has been developed with the addition of the transformer module and a loss function similar to those in the YOLOv8s transformer. However, the positions of conventional convolutions are substituted with Ghost convolutions. This modification aims to balance enhanced accuracy with reduced computational load by mapping each input channel to a smaller number of output channels, rather than mapping each input channel to a corresponding output channel as done in typical convolutional layers.
In comparison to YOLOv8, we have implemented the following optimizations:
- Propose the S3CT module into the last layer of the backbone, aiding the model in more accurately extracting and localizing germination features, thereby improving the mAP accuracy of the model.
- Replace convolutional layers with Ghost convolution, enhancing not only the model's performance but also reducing its computational complexity, making it more lightweight and efficient.
- In YOLOv8s, the loss function utilizes CIoU, which has demonstrated significant effectiveness compared to GIoU and DIoU used in the earlier versions of YOLOv8. However, CIoU doesn't handle objects that change in scale whereas SIoU, as a variant of CIoU, addresses this issue by normalizing distance and diagonal distance via the width and height of the ground truth box. This helps the model ameliorate performance in detecting small objects as well as increase the mAP accuracy of the model.
3.1 Proposed SC3T
The proposed SC3T (Do et al., 2023) module which is integrated into the last layer of the backbone is a fusion of the SPP and the C3TR modules which is shown in Figure 4. The SPP module addresses the challenge posed by non-uniform input image sizes encountered in object detection tasks. In traditional CNN, the input image size is typically fixed, requiring resizing or cropping of input images to a predefined one before feeding inputs into the network. However, this approach is not ideal for handling images of different sizes efficiently. To overcome this limitation, the Spatial Pyramid Pooling (SPP) module allows the network to accept input images of various sizes without the need for resizing. We design SPP using kernels with a uniform stride of 1 but varying sizes (5 × 5, 9 × 9, and 13 × 13). This strategy ensures that important features at different scales are captured effectively. After pooling, each sub-region is processed independently to extract distinctive features. The extracted features from all sub-regions are then concatenated along the channel dimension. This feature fusion process through channel concatenation enables the model to combine features of different spatial resolutions efficiently, facilitating robust object detection across images of varying sizes. By incorporating the SPP module into the network architecture, we elevate the model's ability to handle non-uniform input sizes while preserving spatial information effectively, contributing to improved performance in object detection tasks. Subsequently, the output of the SPP module is combined with that from the C3TR module to create the SC3T module in Figure 4, replacing the C3 and the final SPPF layer in the YOLOv5s network to enhance the speed and accuracy of object detection with a smaller network size. The C3TR layer is shown in Figure 4, a combination of a transformer with the C3 layer of CSPDarknet53, which is utilized in the YOLOv5 model. This layer is pivotal for extracting and integrating features from various regions of the image, utilizing the transformer's ability to capture interdependencies among different data segments. Whereas the C3 layer in CSPDarknet53 is dedicated to feature extraction, the transformer is adept at identifying feature relationships. The fusion of these capabilities within the C3TR layer empowers the model to extract features and understand their relationships, which is essential for object detection tasks.
The C3TR layer capitalizes on the transformer's capability to learn hierarchical representations, ranging from low-level to high-level image features. This, in conjunction with CSPDarknet53's feature extraction prowess, enhances feature learning with a more comprehensive contextual insight. The C3TR layer promotes stable learning by utilizing the transformer's layer normalization and residual connections, thereby alleviating issues of gradient vanishing and explosion during the training process, which ensures stable learning in deep neural networks.
Designed for multiscale processing, the C3TR layer receives and integrates feature maps at different scales, ensuring efficient detection of varied-size objects. The head of the C3TR layer reduces the size of the feature map, integrates it with other feature maps, and channels it through the transformer, enabling the learning of characteristics from different image scales. Serving as the input feature map for channel numbers 512, 1,024, and 2,048, the C3TR layer is a pivotal element in YOLOv5's object detection model.
Transformers have demonstrated exceptional performance in sequence modeling and have proven to be more effective than traditional architectures in non-sequential tasks such as object detection. In particular, the use of transformer-based learning in C3TR capitalizes on the model's ability to capture long-range dependencies. These dependencies involve correlations between elements that are separated by significant distances in a sequence or within spatio-temporal data. Put simply, long-range dependencies reflect the meaningful relationships between a given element and those that come before or after it. For example, this includes the contextual links between words in a sentence, the spatial relationships between objects, and the movements observed across successive frames in a video (Zhao et al., 2019).
C3TR captures these long-term dependencies in input feature maps through a self-attention mechanism, allowing the model to consider a broader context and comprehend global object information, contributing to accurate object detection. The adept combination of lines (referring to the effective feature extraction pathways in the CNN backbone) and transformers (utilizing a self-attention mechanism) ensures high efficiency with a minimal number of parameters. In the context of our methodology, the term “lines” refers to the efficient feature extraction pathways established within the CNN backbone, such as the DarkNet architecture used in YOLO. These pathways are responsible for extracting hierarchical features from input images. On the other hand, “transformers” denote components of our model that leverage self-attention mechanisms to capture long-range dependencies within the input feature maps. By integrating these effective feature extraction pathways (lines) with the self-attention capabilities of transformers, our model achieves high efficiency. This adept combination grants in the model to process global contextual information while maintaining a compact parameter footprint, leading to improved performance in object detection tasks.
In conclusion, the amalgamation of SPP and C3TR demonstrates effectiveness in YOLOv8 by utilizing concatenation to synthesize features at various sizes. Given the non-uniform input image sizes, this combined with transformer-based long-term dependency modeling and convolution-based visual feature extraction, enhances precise object localization and increases classification performance. Our experiments have consistently shown results aligning with these claims, with an mAP index ~6.0% higher than that from the baseline model.
3.2 Ghost module
Ghost convolution which is depicted in Figure 5 emerges as a structure strategically designed to strike a balance between heightened accuracy and minimal computational overhead. It specifically tackles the limitations found in conventional deep neural network models, known for their excessive intricacy, making them challenging for deployment on resource-limited devices.
In this study, we leverage the potential of the Ghost convolution module to enhance the performance of the YOLOv8 framework, especially in scenarios constrained by resource limitations. The conventional convolution module is replaced with the Ghost convolution module, representing a streamlined alternative to traditional convolutional layers. This transition significantly reduces the count of model parameters, facilitating the efficient allocation of computational resources.
3.3 SIoU loss function
After amalgamating models to create a more robust framework, we opted for the YOLOv8s-Transformer model for hardware deployment. However, to lift the system's performance in accurately identifying smaller objects, especially for search and rescue operations which need extremely high accuracy, thus model optimization is essential. We observed that accuracy could still be improved by modifying or optimizing the loss function, specifically in this case, by utilizing the SIoU loss function that has demonstrated efficacy in the previous version of YOLOv5, and facilitated higher prediction accuracies for small objects due to incorporating a smoothing factor into the computation formula. This approach stabilizes the gradient of the SIoU loss function during model training. As a result, models using the SIoU loss function can better learn from small bounding boxes, thereby improving the accuracy of object localization predictions.
In the loss function officially used in YOLOv8, CIoU is adopted. It evaluates the distance between the centers of the predicted and ground-truth boxes, the aspect ratio difference, and the diagonal distance ratio. These factors aim to enhance the detection accuracy, especially for small objects. The loss function of SIoU (Gevorgyan, 2022) is a variant of CIoU that does not scale with the ratio. In SIoU, the “distance” refers to the distance between the centers of the predicted actual boxes, while the “diagonal distance” is the length of the diagonal line connecting the predicted actual boxes. Both the distance and diagonal distance are normalized by the width and height of the ground-truth box. This normalization allows SIoU to handle objects with different ratios, a common challenge in object detection. The SIoU loss function is defined by the sum of the following costs: angle cost, distance cost, shape cost, and IoU cost. As shown in Figure 6, the expression of angle cost is:
where σ represents the distance between the centroid of the ground truth bounding box and the prediction box as follows.
The distance cost is formulated in the following:
As α approaches 0, the impact of the distance cost diminishes significantly. Conversely, when α is closer to γ, the influence of Ldis increases. The difficulty of the problem grows with the angle. Therefore, as the angle increases, γ is given greater priority over the distance value. It is important to note that the distance cost decreases as α approaches 0.
The formula for shape cost is:
Where w and wgt refer to the widths of the prediction and ground-truth boxes, respectively, and h and hgt denote the heights of the prediction and ground-truth boxes, respectively. The total loss function is represented by:
In this study, the SIoU loss function is utilized to replace CIoU in the proposed YOLOv8s-Transformer model. We also compare it with the other loss functions such as DIoU, GIoU, and EIoU for an evaluation. Additionally, experimental results indicate that SIoU when integrated with adoption in YOLOv8s, achieves superior outcomes compared to other losses.
4 Experiment result and discussion
4.1 Dataset
Strawberries hold immense agricultural value globally. However, their susceptibility to a diverse range of diseases poses a significant threat, rapidly spreading within short periods. This not only diminishes strawberry yields but also inflicts financial losses on farmers. Consequently, we've developed an Android app for detecting strawberry diseases, utilizing a dataset comprising real-life images of both healthy and diseased strawberries. In this work, we leverage two datasets to validate the proposed model. The first dataset from Afzaal et al. (2021), encompasses images of strawberries afflicted by seven distinct diseases. Unlike datasets gathered from controlled laboratory settings, this dataset, collected from real fields and greenhouses, presents several challenges including variations in background, complex field conditions, and diverse lighting environments. These variations empower our model to be more robust and adaptable after learning. This dataset comprises 2,500 images of strawberry diseases captured in various greenhouses using mobile phones, under natural illumination conditions in South Korea. Expert verification ensured the accuracy of disease labels. The second dataset is built up by capturing the intricacies of real-world scenarios. We trained the models using images of strawberry diseases obtained from the Vietnam Ministry of Agriculture. This dataset consists of 1,000 images categorized into five classes: normal strawberries, gray mold disease, black spot disease, powdery mildew disease, and rubber disease as listed in Table 1. Classifications were based on crucial factors like color, area, density of the diseased part, and the species' shape. Rigorous verification, involving two individuals following guidelines, was conducted to minimize labeling errors. Incorrect images, such as non-strawberry entities, from the controlled lab settings, and out-of-scope images, were meticulously removed.
For the object detection task, precise bounding regions encompassing the strawberries in a full image are imperative. To accomplish this, we used Roboflow to annotate the leaves in each image with bounding boxes. Recognizing that real-world images may contain multiple strawberries or a combination of diseased and healthy strawberries, we carefully labeled each leaf with its corresponding class. During the labeling process, we ensured that the bounding box fully encompassed the strawberries and that its area was no less than approximately one-eighth of the image size. After completing the annotations, we divided the dataset into training, validation, and test sets with an 8:1:1 ratio. This division allowed us to train this proposed model on a substantial portion of the data while reserving separate subsets for model validation and final performance evaluation. Subsequently, the model training process commenced, encompassing the essential steps for achieving effective object detection on strawberry images.
4.2 Implementation details
4.2.1 Training details
The proposed models were trained on the strawberry dataset utilizing Google Colab with a high RAM runtime and Tesla V100 GPU configuration. After the training process was completed, we obtained the weight sets for each model. The effectiveness of each model was then evaluated using the test dataset. Lastly, the performance of the proposed models was compared with both the backbone version and alternative methods.
4.2.2 Metrics
In the domains of action recognition and detection, evaluation metrics include average accuracy and video-level mAP at specific IoU thresholds. For our study, we use an IoU threshold of 0.5 to assess detection performance. Furthermore, we report the computational complexity in terms of FLOPs associated with network inferences. To ensure accurate measurement and avoid unnecessary computations, the model is frozen prior to calculating FLOPs. The total number of operations across all convolution layers is then determined based on factors such as the number of output feature maps, kernel sizes, and both input and output channels.
4.3 Experimental results on the dataset
To verify the effectiveness of the proposed models, YOLOv8s-Transformer and YOLOv8s-Transformer-Ghost, we conducted experiments on the dataset sourced from the study (Afzaal et al., 2021). This dataset was collected in real fields and greenhouses and processed by Korean researchers, encompassing seven different diseases on strawberries, comprising 2,500 samples captured using camera-equipped mobile phones. We compared the proposed models, YOLOv8s-Transformer and YOLOv8s-Trans-Ghost, with the original YOLOv8s and YOLOv5s. The results of the comparative experiments are presented in Table 2.
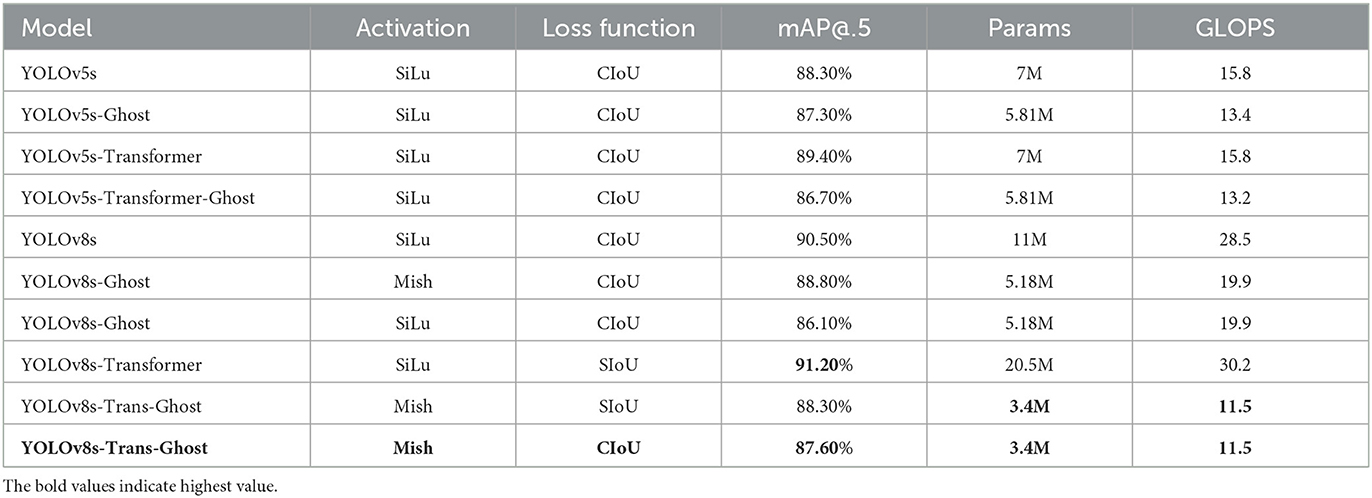
Table 2. Performance of proposed and conventional YOLO models in the Strawberry dataset (Afzaal et al., 2021).
Regarding accuracy, we observe a significant improvement in models incorporating the transformer in both YOLOv5s and YOLOv8s versions, with mAP values of 89.4 and 91.2%, respectively. These outcomes are higher than those from the original versions and alternatives. However, the number of parameters (params) increases considerably.
In the pursuit of a lightweight model that demands both compactness and accuracy, our YOLOv8s-Trans-Ghost reveals promising results. Combining the Ghost module with the YOLOv8s-Transformer shows favorable outcomes, with a substantial reduction in the number of parameters and GLOPS, at 3.4M and 11.5, respectively. The complexity of the YOLOv8s-Trans-Ghost is even lower than that of the YOLOv5s model, which is considered lightweight. This demonstrates efficiency and responsiveness to the problem of applying the model to the strawberry disease detection system in our context.
From the experiments conducted on the dataset, we observe that the transformer module proves effective in enhancing the model's accuracy. However, it comes with the overhead of an increased number of model parameters. In an effort to mitigate the parameter increase, the implementation of Ghost convolution has demonstrated efficiency by significantly reducing the number of parameters, down to three to four times less compared to that from the original YOLOv8s model. This reduction in parameters is achieved without compromising the overall performance, showcasing the effectiveness of Ghost convolution in optimizing the model's efficiency.
4.4 Experimental results on our dataset
Following the validation of the proposed models on the Afzaal et al. (2021) dataset, we proceeded to train the proposed models on our strawberry dataset.
4.4.1 Efforts for YOLOv8s-transformer accuracy model
The first approach involves augmenting accuracy. The proposed SC3T module is integrated into the final layer of the backbone, forming the YOLOv8s-Transformer. Subsequently, the Ghost module is incorporated to reduce the model's size and complexity. In this experiment, we compare the results of the improved YOLOv8s models with other integrated models in YOLOv5s with the embedded modules such as ShuffleNetv2, EfficientNet, ShuffleNetv2-Transformer, EfficientNet-Transformer, and the original ones.
As listed in Table 3 the original YOLOv8s model has 11,127,519 parameters, mAP@.5 of 72.3, and GPLOPs of 28.4. In contrast, the YOLOv8s-Transformer model has shown a significant increase improvement of nearly 6% compared to the original model in terms of mAP@.5. Similar results are observed for the YOLOv5s model, where the YOLOv5s-Transformer model with an accuracy of 75.7% outperforms the other integrated models based on YOLOv5s, yielding a 2.1% increase over the original version. However, a limitation arises as the increased accuracy comes with a substantial increase in the number of parameters, computational operations, and model complexity
The proposed models using the transformer and Ghost modules have demonstrated impressive results and outperformed all other models in all metrics. With precision at 80.4%, recall at 70.3%, mAP@.5 at 80.3%, and a reduced parameter count of 3.4M, half of the size of the YOLOv5s model, alongside a decreased GLOPS of 11.5, the YOLOv8s-Trans-Ghost model exhibits remarkable efficiency. Comparing the nine models in Table 3, it is evident that the YOLOv8s-Tran-Ghost model significantly improves accuracy while maintaining model simplicity. Moreover, the parameter amounts and GLOPS of this model are notably reduced, highlighting the superior performance of the YOLOv8s-Trans-Ghost model.
4.4.2 Efforts for YOLOv8s-trans-ghost lightweight model
With the goal of finding a sufficiently lightweight model applicable to real-time systems while ensuring high accuracy, we conducted experiments to explore and integrate the embedded modules that have shown promising results for a lightweight model, such as Ghost and CBam in YOLOv5, integrated into the YOLOv8s architecture to become the proposed YOLOv8s-Transformer. We compared the results of these models, considering various activations and loss functions in the experiments.
As illustrated in Table 4, YOLOv8s-Transformer models integrated with Ghost modules exhibited a substantial reduction in the number of parameters (Params), with 3.4M parameters, less than one-third of that from the original model and a half that from the YOLOv5s model. The GLOPS index also reveals a significant improvement, decreasing by over 2.5 times compared to that from the original model. Notably, the YOLOv8s-Trans-Ghost-Pretrain model with precision(P) and mAP@.5 both exceeding 80%, incorporating Silu activation and SIoU loss, not only maintains accuracy but also shows a remarkable increase compared to all other models. This underscores the effectiveness of applying proposed models to hardware systems, ensuring real-time performance while maintaining high accuracy in object detection.
4.5 Experiments for loss functions
To assess the effectiveness of different loss functions on the proposed YOLOv8s-Transformer model, we explored five loss functions—CIoU (2020), DIoU, GIoU, EIoU, and SIoU (2022). These functions are compared based on the mAP values, a critical indicator for evaluating the target detection model's performance, where a higher mAP signifies superior accuracy in detecting target objects.
As illustrated in Table 5, the YOLOv8s-Transformer model with SIoU loss outperforms the other models, with mAP@.5 values of 75.7 and 79.8% for YOLOv5s and YOLOv8s, representing increases of 2.1 and 7.5%, respectively, compared to the original versions. This indicates that employing the SIoU loss function effectively reduces sensitivity to position deviations of small objects, addressing the localization issue of small objects and enhancing training accuracy.
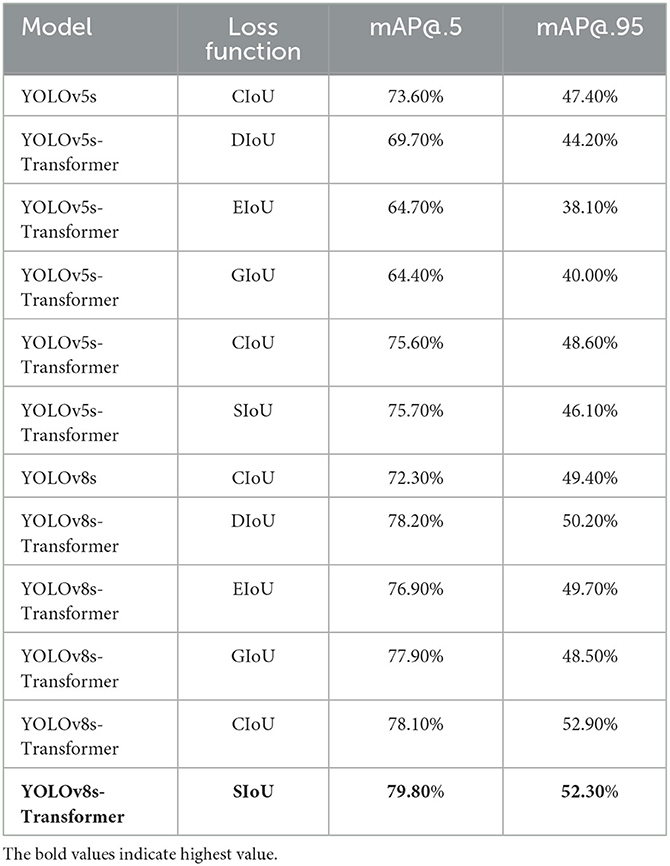
Table 5. Performance of YOLOv5s, YOLO5s-transformer, YOLOv8, and YOLOv8-transformer using different loss functions on our dataset.
4.6 Ablation experiments
We proposed four key improvements to the YOLOv8s model: (1) introduction of the SC3T module, (2) addition of the Ghost module to the backbone, (3) utilization of the SIoU loss function, and (4) application of pretraining from dataset (Afzaal et al., 2021) to our YOLOv8s-Trans-Ghost model.
In Table 6, aiming to create a model with higher accuracy, we introduced the transformer module, resulting in a 5.8% increase in mAP@.5. After replacing the loss function with SIoU, accuracy further lifted by 1.7%, reaching 79.8%. To create a lightweight model, the Ghost module is incorporated into the original model, reducing the parameter count by half to 5.2 million compared to the original one, 11 million, and significantly dropping the GLOPs index to 19.9. However, the accuracy decreases, prompting us to combine the two modules to form the YOLOv8s-Trans-Ghost model. This leads to an increase in mAP@.5 to 74.4%, a substantial reduction in parameters to 3.4 million, and a notable improvement in GLOPs to 11.5. This demonstrated an increased accuracy while significantly reducing the model's weight.
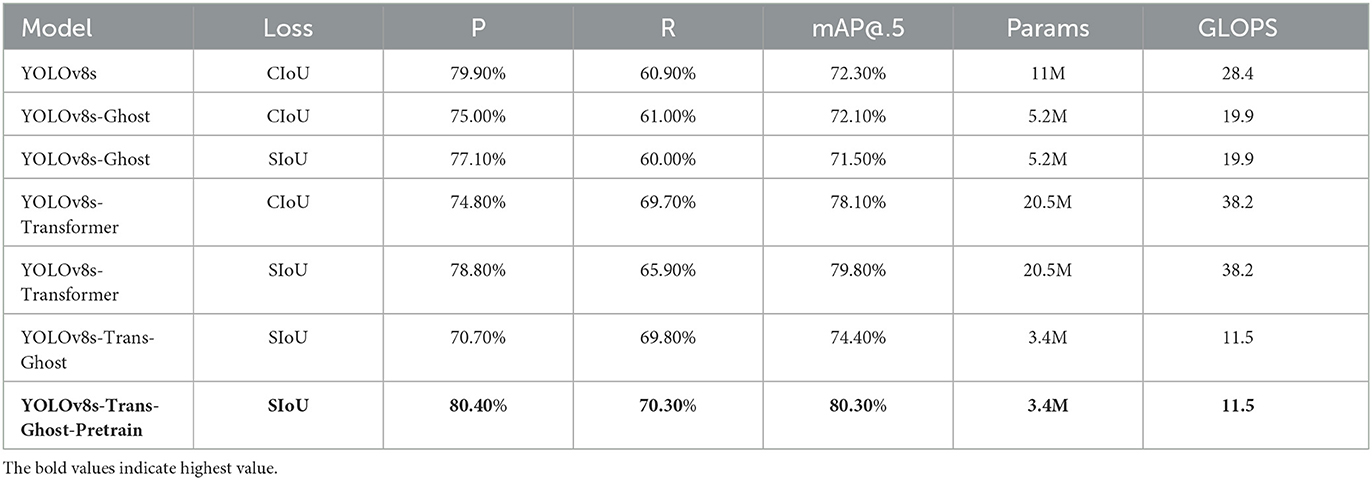
Table 6. Performance of the ablation analyses of the proposed model based on YOLOv8s on our dataset.
Continuing the pursuit of accuracy enhancement, we pre-trained the YOLOv8s-Trans-Ghost model by the dataset (Afzaal et al., 2021). The mAP@.5 in the proposed model surges to an astonishing 80.3%, surpassing those from the other models where the model maintains its lightweight structure.
In summary, our YOLOv8s-Trans-Ghost model outperforms the others due to the following key enhancements:
SC3T transformer module: the proposed SC3T module, combining the SPP and C3TR structures, is placed in the last layer of the backbone. The SPP kernel has a consistent stride but varying sizes (5 × 5, 9 × 9, and 13 × 13), with feature concatenation through channel concatenation. C3TR consists of a transformer block at the three outputs of the detection network, combined with concat weighted to fuse features obtained from the transformer block with those from other parts of the network, such as the SPP kernel outputs or intermediate features from the backbone. Furthermore, a standard transformer layer is employed to aggregate global information from the final block of the backbone network. The transformer encoder features a Multi-head Self-Attention (MSA) mechanism, which updates and combines query (Q), key (K), and value (V) tensors that encode global features from various spatial locations for linear projection. This self-attention mechanism excels at capturing contextual details and reducing the loss of global information.
Ghost module: this module is incorporated into the YOLOv8s backbone by replacing the original Conv module, compressing input feature layers through non-linear and linear convolution operations, resulting in a reduced parameter count and improved GLOPs index.
SIoU loss function: the SIoU loss function is a variation of CIoU loss that normalizes distance and diagonal distance by the width and height of the ground truth box. This normalization allows SIoU to handle objects with different scales, a common issue in object detection.
Pre-trained models: initially, we pre-trained the YOLOv8s-Trans-Ghost model on a test dataset. Subsequently, we trained the model on our dataset based on the pre-trained model's weights. The performance was improved significantly as the pre-trained model learned common features from a large dataset, enabling good generalization for different tasks.
These collective improvements make YOLOv8s more accurate and sensitive, especially in real-time detection scenarios, while maintaining a lightweight structure.
4.7 Comparision and discussion on the dataset of the proposed and conventional models
In Table 7, we present a comparative analysis of findings from other datasets with similar characteristics. Ouyang et al. (2013) conducted a study focusing on three types of strawberry diseases. Their approach began with initial segmentation, where diseased strawberries were isolated using digital image processing and pattern recognition techniques. They then compared the performance of a neural network with that of an SVM classifier. Although an exact accuracy figure was not provided, it was concluded that SVM achieved a higher recognition rate than the neural network as a classifier.
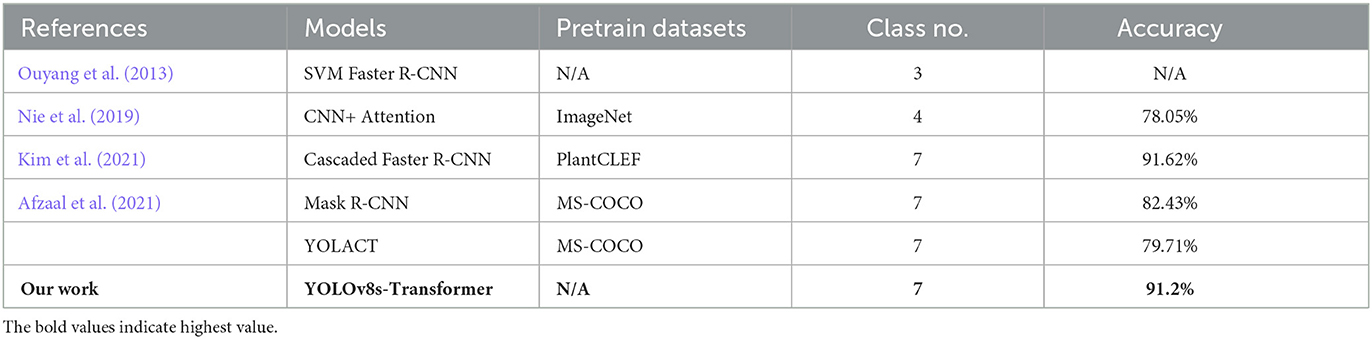
Table 7. Comparison of the proposed and relevant models in dataset (Afzaal et al., 2021).
Kim et al. (2021) dataset, on the other hand, indicated that the Cascaded Faster R-CNN model, pre-trained on ImageNet with four classes, yielded a result of 78.05%. Another study by Nie et al. (2019) reported a baseline mAP@.5 of 88.05% using Faster R-CNN with ImageNet pre-trained weights. This study further improved performance through a cascaded architecture and additional pre-trained weights from the PlantCLEF dataset. However, it is important to note that their model, designed for coarse-grained object detection, differs from our focus on fine-grained instance segmentation. In a related experiment (Afzaal et al., 2021), tests involving Mask R-CNN with pre-training on the MS COCO dataset achieved a mAP@.5 of 82.43%. In this same study, a comparison with YOLACT was conducted to validate their results. Regarding our accuracy model, the YOLOv8s-Transformer, used in experiments on this dataset, we opted not to use pretraining but rather trained the model from scratch. Nevertheless, the results were notably high, reaching 91.2%.
4.8 Visualization
Following the quantitative assessments presented above, we proceed to conduct a visual evaluation based on images identified by the models.
4.8.1 Qualitative evaluation of the proposed backbone and original models
To demonstrate the detection performance of the proposed model, we randomly selected images from the test dataset for evaluation. The results are shown in Figure 7, where the highlighted areas represent the network's detection outputs.
According to the experimental findings, it's apparent that the standard YOLOv8 model had difficulty detecting objects in the images, particularly when a strawberry was partially obscured by overlapping ones, which completely hid its visibility. The integration of SC3T significantly improved the network's sensitivity and adaptability for detecting small objects by expanding the receptive field. This enhancement also boosted feature recognition and utilization efficiency, reducing missed detections through effective feature synthesis across different scales. Additionally, the use of Ghost convolution in the model's lightweight optimization has simplified deployment. The enhanced YOLOv8s model shows improved detection performance and confidence compared to the standard YOLOv8 in certain cases. Nevertheless, some missed detections remain, highlighting the need for further optimization to meet practical detection requirements.
4.8.2 Qualitative assessment of model effectiveness with different loss functions
Next, in Figure 8 we visualize the performance improvement of the transformer model by comparing two versions, YOLOv5 and YOLOv8, with five different loss functions: DIoU, GIoU, CIoU, EIoU, and SIoU. Through the images of gray mold disease below, it is evident that most models detect accurately, and YOLOv8s-Trans with SIoU loss demonstrates the highest accuracy at 91.2%, surpassing most other models. This highlights the effectiveness of accurately detecting objects in the proposed models with the SIoU loss function.
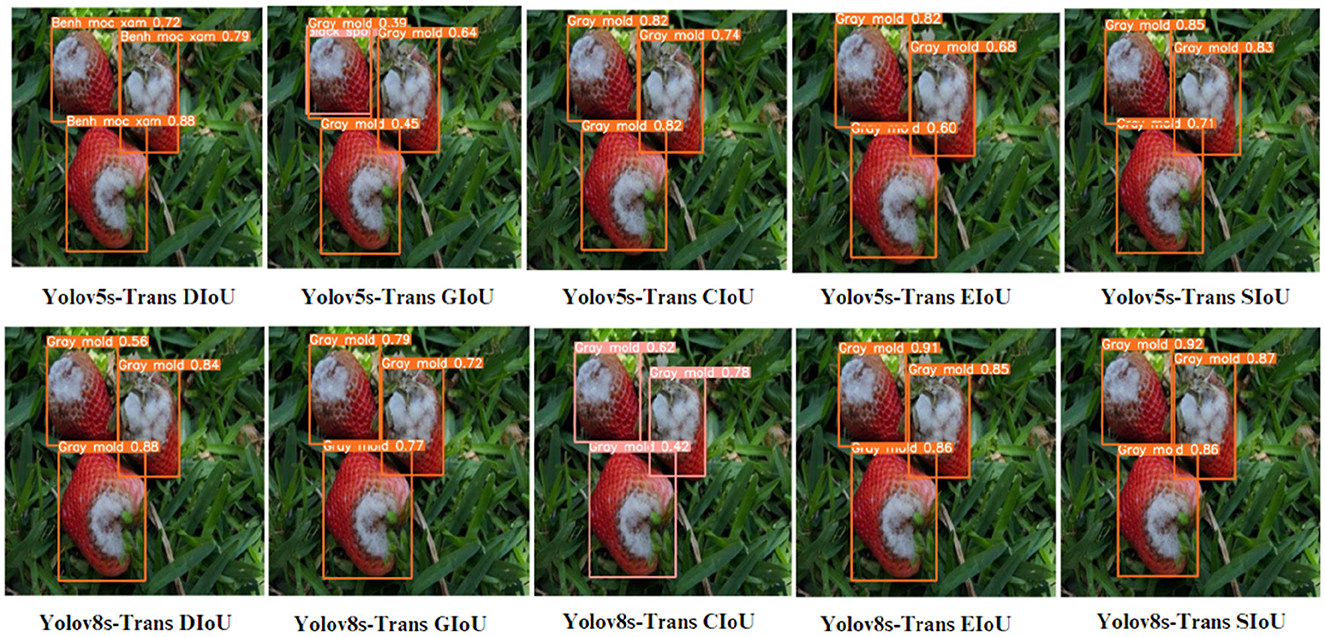
Figure 8. Diagram detection results of the YOLOv8s-transformer and the YOLOv8s-transformer models with five different loss functions.
4.8.3 Qualitative evaluation of the proposed and conventional models on the dataset
We conduct an inference evaluation on the test dataset (Afzaal et al., 2021). In Figure 9, we can observe that the YOLOv8s-Trans-Ghost model accurately detects various diseases on the leaves, even when the leaves are partially hidden in the image's upper corner. In contrast, the other models either fail to detect the precise frame of the leaves in the image or, if detected, result in a large bounding area with low accuracy. This exhibits that the proposed model is effective in object detection as compared to the other models.
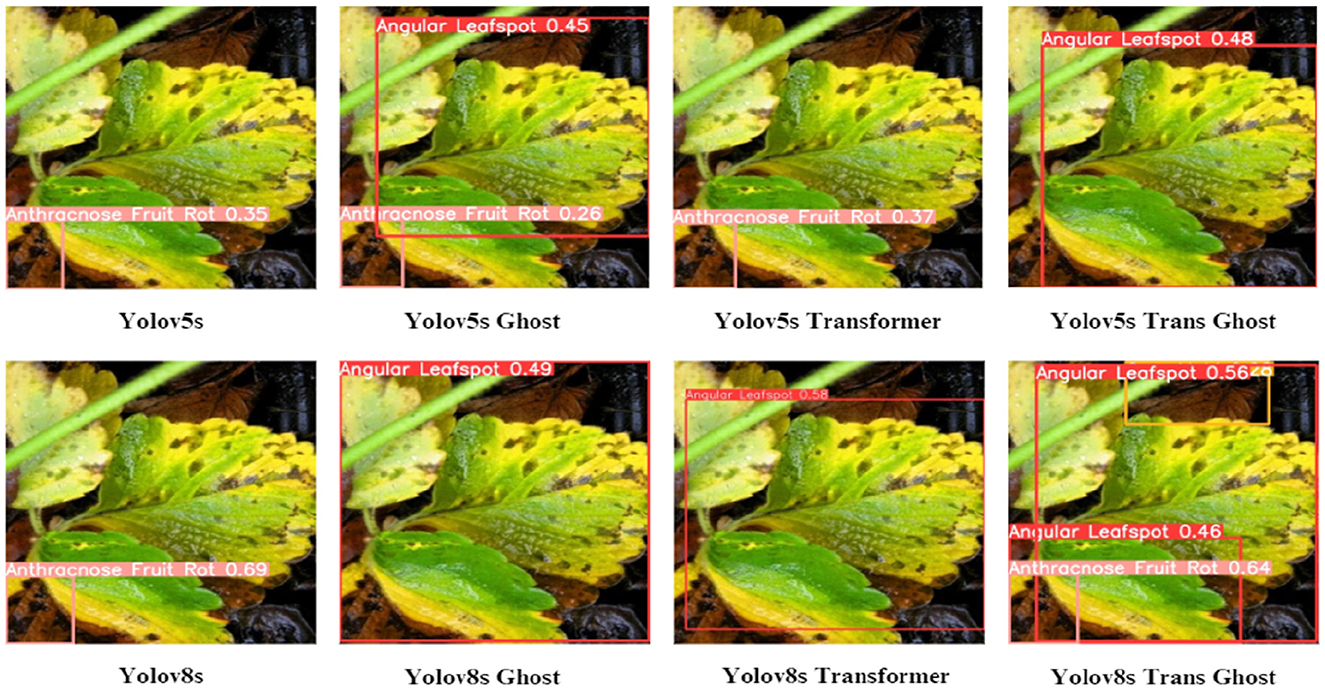
Figure 9. Diagram evaluation of the proposed and conventional models on the dataset (Afzaal et al., 2021).
4.8.4 Quantization model for edge devices
In this study, we converted the proposed YOLOv8s-Trans-Ghost-Pretrain model on our dataset to the standard format for Edge device applications. ONNX (Open Neural Network Exchange) serves as an open format designed for the representation of machine learning models, enabling their portability across diverse platforms. Through the process of exporting our model to ONNX, we gain the ability to deploy it across various devices, thereby harnessing hardware acceleration to enhance inference speed in real-time applications. Particularly, we loaded the checkpoint data during training and initialized a YOLOX model. To export the model to ONNX with different input sizes, we set the width and height input axes as dynamic. Finally, when performing inference with ONNX Runtime, we focused on the capabilities needed for inference. ONNX Runtime is a cross-platform inference engine which is a machine-learning accelerator suitable for mobile devices.
4.8.5 Building android apps
Upon completion of the training and quantization processes for the model, our next step involved the development of an Android application. Specifically, we followed seven steps outlined below:
1. Model training: we trained the model to generate a weight file in PyTorch (.pt format), leveraging the robust capabilities of this framework in deep learning research.
2. Conversion to ONNX format: the YOLO model (.pt format) was converted into the ONNX format with FP32 weights, ensuring compatibility and interoperability across different frameworks and platforms.
3. Quantization with ONNX runtime: to optimize model performance and efficiency, we performed quantization using ONNX Runtime, resulting in an optimized ONNX file ready for deployment.
4. Conversion to TensorFlow model: the ONNX Runtime model (.onnx file) was further converted into a TensorFlow model (.pb file), facilitating seamless integration with TensorFlow-based applications and frameworks.
5. Conversion to TensorFlow lite model: subsequently, the TensorFlow model (.pb model) underwent the conversion into a TensorFlow Lite model, suitable for deployment on mobile and edge devices with limited computational resources.
6. Android studio setup: to begin development for the Android platform, we downloaded and installed Android Studio, the official Integrated Development Environment (IDE) for Android application development.
7. App development and deployment: finally, leveraging the features and tools provided by Android Studio, we proceeded to build and run our object detection App on Android devices, demonstrating the practical implementation and real-world applicability of our deep learning model in object detection tasks.
Through these comprehensive steps, we seamlessly transitioned from model training and optimization to the development and deployment of a fully functional Android application, showcasing the practical utility of our deep learning research in a real-world application.
Figure 10 reveals our Android app interface, which is the product created by members of our research team at the International School at Vietnam National University. This app has performed excellently on mobile phones, detecting diseases on strawberries, and can flexibly switch between four different YOLOv5 and YOLOv8 models.
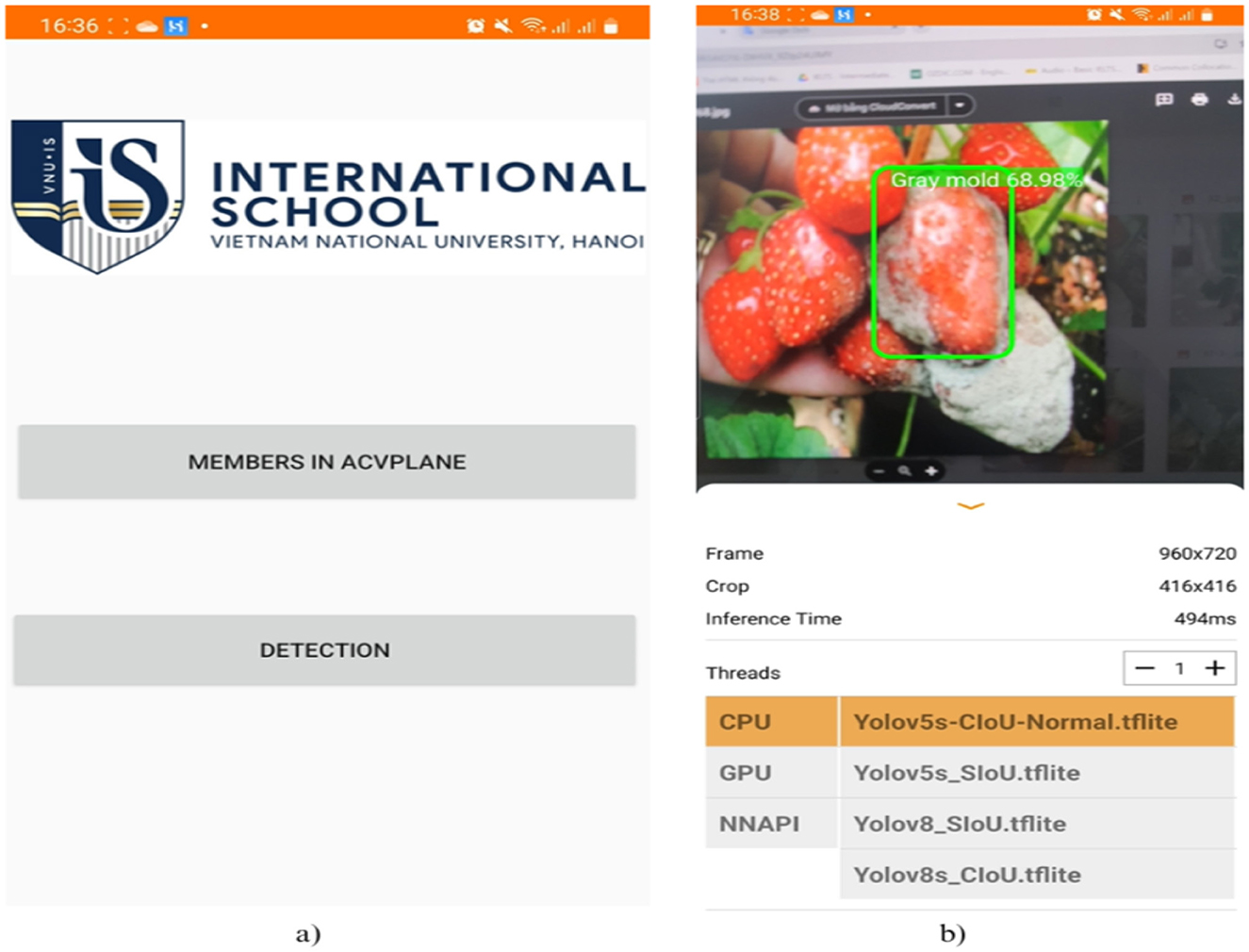
Figure 10. Diagram the Android app interface utilizes TFLite for disease detection on strawberries. (A) Startup interface (B) Object detection interface.
5 Conclusion
This work introduces a valuable dataset focusing on diseases in strawberries. Leveraging this dataset, we conducted experiments on the proposed YOLOv8s-Trans-Ghost, aiming for fast and accurate target detection. In this model, the SC3T module which is a combination of the SPP and C3TR modules, was proposed. This module excels in feature synthesis across different sizes, incorporating transformer-based long-term dependency modeling and convolution-based visual feature extraction to enhance object localization precision and classification performance. Experiments on the YOLOv8s-Transformer model demonstrated a promising mAP result of 78.1%, with an increase of 5.8% over the baseline model on our dataset. The adoption of the SIoU loss function, replacing CIoU, further increases the mAP up to 79.8%, showcasing the effectiveness of this loss function in precise object detection. However, the model's parameter count doubles to 20.5M, hindering real-time applicability when embedded in a practical hardware platform. To address this, we substituted Conv in the YOLOv8s-Transformer with Ghost Conv, which is proven effective in reducing parameters in YOLOv5 versions. Experimental results are also very promising, with a parameter a mount reduced to 3.4M, nearly one-third of the original one. Subsequently, we employed the weights of the proposed YOLOv8s-Trans-Ghost model which was trained on the large test dataset (Afzaal et al., 2021) as pretraining for the application on our dataset. The value of mAP significantly increases to 80.3%, accompanied by notable improvements in other metrics, such as precision at 80.4% and recall at 70.3%, surpassing those from the other models. Parameters and GLOPS are substantially lessened reduced to 3.4M and 11.5, respectively. These results reveal that the model proposed offers a lightweight and efficient solution for detecting diseases in strawberries while maintaining high precision, with the potential to significantly promote production efficiency. Finally, for deploying the proposed model, we adopted the compression technique for model optimization to meet the computation capabilities of mobile devices by ONNX Runtime. Additionally, the Android app was successfully built to effectively spread the proposed model for the applications of detecting strawberry diseases.
Data availability statement
The original contributions presented in the study are included in the article/supplementary material, further inquiries can be directed to the corresponding author.
Author contributions
M-TD: Conceptualization, Methodology, Writing – review & editing, Data curation, Software, Validation, Visualization, Writing – original draft. M-HH: Conceptualization, Methodology, Writing – review & editing, Funding acquisition, Investigation, Project administration, Supervision. D-CN: Conceptualization, Investigation, Methodology, Resources, Visualization, Writing – review & editing. OT-C: Conceptualization, Methodology, Resources, Supervision, Validation, Writing – review & editing.
Funding
The author(s) declare financial support was received for the research, authorship, and/or publication of this article. This research was supported by the International School, Vietnam National University, Hanoi (VNU-IS) under the Decree of No. 95/2014/NĐ-CP.
Conflict of interest
The authors declare that the research was conducted in the absence of any commercial or financial relationships that could be construed as a potential conflict of interest.
Publisher's note
All claims expressed in this article are solely those of the authors and do not necessarily represent those of their affiliated organizations, or those of the publisher, the editors and the reviewers. Any product that may be evaluated in this article, or claim that may be made by its manufacturer, is not guaranteed or endorsed by the publisher.
References
Afzaal, U., Bhattarai, B., Pandeya, Y. R., and Lee, J. (2021). An instance segmentation model for strawberry diseases based on mask R-CNN. Sensors 21:6565. doi: 10.3390/s21196565
Azizpour, H., Razavian, A. S., Sullivan, J., Maki, A., and Carlsson, S. (2015). Factors of transferability for a generic convnet representation. IEEE Trans. Pattern Anal. Mach. Intell. 38, 1790–1802. doi: 10.1109/TPAMI.2015.2500224
Baweja, H. S., Parhar, T., Mirbod, O., and Nuske, S. (2018). “Stalknet: a deep learning pipeline for high-throughput measurement of plant stalk count and stalk width,” in Field and Service Robotics, eds. M. Hutter, and R. Siegwart (Cham: Springer International Publishing), 271–284. doi: 10.1007/978-3-319-67361-5_18
Chen, L.-C., Papandreou, G., Kokkinos, I., Murphy, K., and Yuille, A. L. (2017). Deeplab: Semantic image segmentation with deep convolutional nets, atrous convolution, and fully connected CRFS. IEEE Trans. Pattern Anal. Mach. Intell. 40, 834–848. doi: 10.1109/TPAMI.2017.2699184
Chen, O. T. C., Ha, M. H., and Lee, Y. L. (2020). Computation-affordable recognition system for activity identification using a smart phone at home. IEEE Int. Symp. Circuits Syst. 2020, 1–5. doi: 10.1109/ISCAS45731.2020.9180826
Chen, Y., Lee, W. S., Gan, H., Peres, N., Fraisse, C., Zhang, Y., et al. (2019). Strawberry yield prediction based on a deep neural network using high-resolution aerial orthoimages. Remote Sens. 11:1584. doi: 10.3390/rs11131584
Cheng, X., Zhang, Y., Chen, Y., Wu, Y., and Yue, Y. (2017). Pest identification via deep residual learning in complex background. Comput. Electron. Agric. 141, 351–356. doi: 10.1016/j.compag.2017.08.005
Cui, Y., Song, Y., Sun, C., Howard, A., and Belongie, S. (2018). “Large scale fine-grained categorization and domain-specific transfer learning,” in Proceedings of the IEEE Conference on Computer Vision and Pattern Recognition (Salt Lake City, UT: IEEE), 4109–4118. doi: 10.1109/CVPR.2018.00432
Do, M.-T., Ha, M.-H., Nguyen, D.-C., Thai, K., and Do Ba, Q.-H. (2023). “Human detection based yolo backbones-transformer in UAVs,” in 2023 International Conference on System Science and Engineering (ICSSE) (Ho Chi Minh: IEEE), 576–580. doi: 10.1109/ICSSE58758.2023.10227141
Dong, X., Yan, S., and Duan, C. (2022). A lightweight vehicles detection network model based on yolov5. Eng. Appl. Artif. Intell. 113:104914. doi: 10.1016/j.engappai.2022.104914
Fang, W., Wang, L., and Ren, P. (2019). Tinier-yolo: a real-time object detection method for constrained environments. IEEE Access 8, 1935–1944. doi: 10.1109/ACCESS.2019.2961959
Ferentinos, K. P. (2018). Deep learning models for plant disease detection and diagnosis. Comput. Electron. Agric. 145, 311–318. doi: 10.1016/j.compag.2018.01.009
Gallo, I., Rehman, A. U., Dehkordi, R. H., Landro, N., Grassa, R. L., Boschetti, M., et al. (2023). Deep object detection of crop weeds: performance of yolov7 on a real case dataset from UAV images. Remote Sens. 15:539. doi: 10.3390/rs15020539
Gevorgyan, Z. (2022). Siou loss: more powerful learning for bounding box regression. arXiv [Preprint]. arXiv:2205.12740. doi: 10.48550/arXiv.2205.12740
Girshick, R. (2015). “Fast R-CNN,” in Proceedings of the IEEE International Conference on Computer Vision (Santiago: IEEE), 1440–1448. doi: 10.1109/ICCV.2015.169
Gu, Y., Hu, Z., Zhao, Y., Liao, J., and Zhang, W. (2024). MFGTN: a multi-modal fast gated transformer for identifying single trawl marine fishing vessel. Ocean Eng. 303:117711. doi: 10.1016/j.oceaneng.2024.117711
Ha, M. H., and Chen, O. T. C. (2021). Deep neural networks using residual fast-slow refined highway and global atomic spatial attention for action recognition and detection. IEEE Access 9, 164887–164902. doi: 10.1109/ACCESS.2021.3134694
Ha, M. H., Nguyen, D. C., Do, M. T., Kim, D. T., Le, X. H., Pham, N. T., et al. (2024). Plant pathology identification using local-global feature level based on transformer. Indones. J. Electr. Eng. Comput. Sci. 34, 1582–1592. doi: 10.11591/ijeecs.v34.i3.pp1582-1592
He, K., Gkioxari, G., Dollár, P., and Girshick, R. (2017). “Mask R-CNN,” in Proceedings of the IEEE International Conference on Computer Vision (Venice: IEEE), 2961–2969. doi: 10.1109/ICCV.2017.322
Herranz, L., Jiang, S., and Li, X. (2016). “Scene recognition with CNNs: objects, scales and dataset bias,” in Proceedings of the IEEE Conference on Computer Vision and Pattern Recognition (Las Vegas, NV: IEEE), 571–579. doi: 10.1109/CVPR.2016.68
Jayawardena, R. S., Huang, J. K., Jin, B. C., Yan, J. Y., Li, X. H., Hyde, K. D., et al. (2016). An account of colletotrichum species associated with strawberry anthracnose in china based on morphology and molecular data. Mycosphere 7, 1147–1191. doi: 10.5943/mycosphere/si/2c/6
Kim, B., Han, Y.-K., Park, J.-H., and Lee, J. (2021). Improved vision-based detection of strawberry diseases using a deep neural network. Front. Plant Sci. 11:559172. doi: 10.3389/fpls.2020.559172
Kornblith, S., Shlens, J., and Le, Q. V. (2019). “Do better imagenet models transfer better?” in Proceedings of the IEEE/CVF Conference on Computer Vision and Pattern Recognition (Long Beach: CA: IEEE), 2661–2671. doi: 10.1109/CVPR.2019.00277
Lin, T.-Y., Dollár, P., Girshick, R., He, K., Hariharan, B., Belongie, S., et al. (2017). “Feature pyramid networks for object detection,” in Proceedings of the IEEE Conference on Computer Vision and Pattern Recognition (Honolulu, HI: IEEE), 2117–2125. doi: 10.1109/CVPR.2017.106
Liu, H., Sun, F., Gu, J., and Deng, L. (2021). Sf-yolov5: a lightweight small object detection algorithm based on improved feature fusion mode. Sensors 21:7752. doi: 10.3390/s22155817
Liu, J., and Wang, G. (2021). Plant diseases and pests detection based on deep learning: a review. Plant Methods 17:22. doi: 10.1186/s13007-021-00722-9
Liu, W., Anguelov, D., Erhan, D., Szegedy, C., Reed, S., Fu, C.-Y., et al. (2016). “SSD: single shot multibox detector,” in Computer Vision-ECCV 2016: 14th European Conference, Amsterdam, The Netherlands, October 11–14, 2016, Proceedings, Part I 14 (Cham: Springer International Publishing), 21–37. doi: 10.1007/978-3-319-46448-0_2
Lu, Y., Zhang, L., and Xie, W. (2020). “Yolo-compact: an efficient yolo network for single category real-time object detection,” in 2020 Chinese Control and Decision Conference (CCDC) (Hefei: IEEE), 1931–1936. doi: 10.1109/CCDC49329.2020.9164580
Mahmud, M. S., Zaman, Q. U., Esau, T. J., Price, G. W., and Prithiviraj, B. (2019). Development of an artificial cloud lighting condition system using machine vision for strawberry powdery mildew disease detection. Comput. Electron. Agric. 158, 219–225. doi: 10.1016/j.compag.2019.02.007
Mohanty, S. P., Hughes, D. P., and Salathé, M. (2016). Using deep learning for image-based plant disease detection. Front. Plant Sci. 7:1419. doi: 10.3389/fpls.2016.01419
Nie, X., Wang, L., Ding, H., and Xu, M. (2019). Strawberry verticillium wilt detection network based on multi-task learning and attention. IEEE Access 7, 170003–170011. doi: 10.1109/ACCESS.2019.2954845
Ouyang, C., Li, D., Wang, J., Wang, S., and Han, Y. (2013). “The research of the strawberry disease identification based on image processing and pattern recognition,” Computer and Computing Technologies in Agriculture VI: 6th IFIP WG 5.14 International Conference, CCTA 2012, Zhangjiajie, China, October 19-21, 2012, Revised Selected Papers, Part I 6 (Cham: Springer Berlin Heidelberg), 69–77.
Redmon, J., Divvala, S., Girshick, R., and Farhadi, A. (2016). “You only look once: unified, real-time object detection,” in Proceedings of the IEEE Conference on Computer Vision and Pattern Recognition (Las Vegas, NV: IEEE), 779–788. doi: 10.1109/CVPR.2016.91
Sa, I., Ge, Z., Dayoub, F., Upcroft, B., Perez, T., McCool, C., et al. (2016). Deepfruits: A fruit detection system using deep neural networks. Sensors 16:1222. doi: 10.3390/s16081222
Selvaraj, M. G., Vergara, A., Ruiz, H., Safari, N., Elayabalan, S., Ocimati, W., et al. (2019). Ai-powered banana diseases and pest detection. Plant Methods 15, 1–11. doi: 10.1186/s13007-019-0475-z
Shruthi, U., and Nagaveni, V. (2024). Tomsevnet: a hybrid CNN model for accurate tomato disease identification with severity level assessment. Neural Comput. Appl. 36, 5165–5181. doi: 10.1007/s00521-023-09351-w
Song, W., Wang, X., Guo, Y., Li, S., Xia, B., Hao, A., et al. (2024). Centerformer: a novel cluster center enhanced transformer for unconstrained dental plaque segmentation. IEEE Trans. Multimedia 26, 10965–10978. doi: 10.1109/TMM.2024.3428349
Sozzi, M., Cantalamessa, S., Cogato, A., Kayad, A., and Marinello, F. (2022). Automatic bunch detection in white grape varieties using yolov3, yolov4, and yolov5 deep learning algorithms. Agronomy 12:319. doi: 10.3390/agronomy12020319
Xu, R., Lin, H., Lu, K., Cao, L., and Liu, Y. (2021). A forest fire detection system based on ensemble learning. Forests 12:217. doi: 10.3390/f12020217
Xu, X., Zhang, X., and Zhang, T. (2022). Lite-yolov5: a lightweight deep learning detector for on-board ship detection in large-scene sentinel-1 SAR images. Remote Sens. 14:1018. doi: 10.3390/rs14041018
Yan, B., Fan, P., Lei, X., Liu, Z., and Yang, F. (2021). A real-time apple targets detection method for picking robot based on improved yolov5. Remote Sens. 13:1619. doi: 10.3390/rs13091619
Yao, J., Qi, J., Zhang, J., Shao, H., Yang, J., Li, X., et al. (2021). A real-time detection algorithm for kiwifruit defects based on yolov5. Electronics 10:1711. doi: 10.3390/electronics10141711
Yao, S., Chen, Y., Tian, X., Jiang, R., and Ma, S. (2020). An improved algorithm for detecting pneumonia based on yolov3. Appl. Sci. 10:1818. doi: 10.3390/app10051818
Yu, Y., Zhang, K., Yang, L., and Zhang, D. (2019). Fruit detection for strawberry harvesting robot in non-structural environment based on mask-RCNN. Comput. Electron. Agric. 163:104846. doi: 10.1016/j.compag.2019.06.001
Yu, Y., Zhao, J., Gong, Q., Huang, C., Zheng, G., Ma, J., et al. (2021). Real-time underwater maritime object detection in side-scan sonar images based on transformer-yolov5. Remote Sens. 13:3555. doi: 10.3390/rs13183555
Zhang, B., Liu, X., Wang, H., and Li, Z. (2022). Enhancing plant disease recognition using deep learning and data augmentation. J. Plant Dis. Protect. 129, 45–56.
Zhao, L., and Li, S. (2020). Object detection algorithm based on improved yolov3. Electronics, 9, 537. doi: 10.3390/electronics9030537
Zhao, Q., Sheng, T., Wang, Y., Tang, Z., Chen, Y., Cai, L., et al. (2019). M2det: a single-shot object detector based on multi-level feature pyramid network. Proc. AAAI Conf. Artif. Intell. 33, 9259–9266. doi: 10.1609/aaai.v33i01.33019259
Zheng, Z., Wang, P., Ren, D., Liu, W., Ye, R., Hu, Q., et al. (2021). Enhancing geometric factors in model learning and inference for object detection and instance segmentation. IEEE Trans. Cybern. 52, 8574–8586. doi: 10.1109/TCYB.2021.3095305
Zhou, B., Lapedriza, A., Khosla, A., Oliva, A., and Torralba, A. (2017). Places: a 10 million image database for scene recognition. IEEE Trans. Pattern Anal. Mach. Intell. 40, 1452–1464. doi: 10.1109/TPAMI.2017.2723009
Zhu, X., Lyu, S., Wang, X., and Zhao, Q. (2021). “TPH-yolov5: improved yolov5 based on transformer prediction head for object detection on drone-captured scenarios,” in Proceedings of the IEEE/CVF International Conference on Computer Vision (Montreal, BC: IEEE), 2778–2788. doi: 10.1109/ICCVW54120.2021.00312
Keywords: DNN, transformer, Ghost Conv, SIoU loss function, pre-trained, quantization, android application
Citation: Do M-T, Ha M-H, Nguyen D-C and Tzyh-Chiang Chen O (2025) Toward improving precision and complexity of transformer-based cost-sensitive learning models for plant disease detection. Front. Comput. Sci. 6:1480481. doi: 10.3389/fcomp.2024.1480481
Received: 14 August 2024; Accepted: 31 December 2024;
Published: 22 January 2025.
Edited by:
Sokratis Makrogiannis, Delaware State University, United StatesReviewed by:
Nagaraju Yalavarthi, Central Silk Board, IndiaPreeta Sharan, The Oxford College of Engineering, India
Copyright © 2025 Do, Ha, Nguyen and Tzyh-Chiang Chen. This is an open-access article distributed under the terms of the Creative Commons Attribution License (CC BY). The use, distribution or reproduction in other forums is permitted, provided the original author(s) and the copyright owner(s) are credited and that the original publication in this journal is cited, in accordance with accepted academic practice. No use, distribution or reproduction is permitted which does not comply with these terms.
*Correspondence: Manh-Hung Ha, aHVuZ2htQHZudS5lZHUudm4=