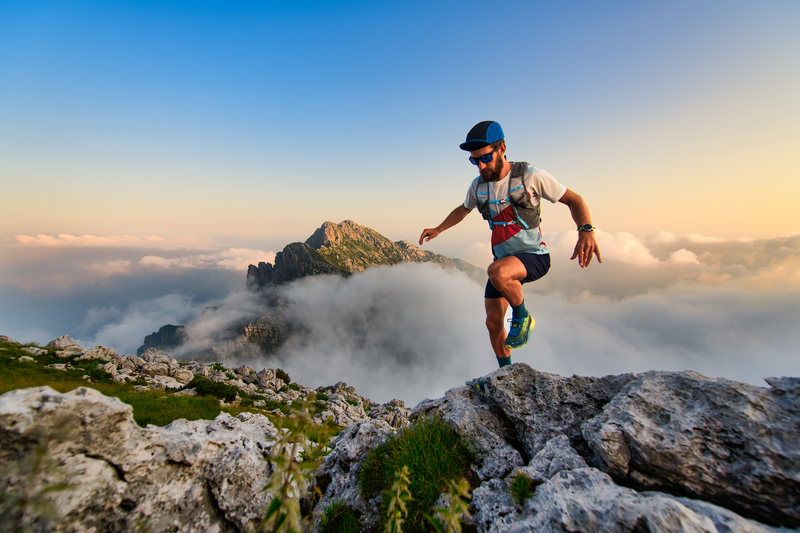
95% of researchers rate our articles as excellent or good
Learn more about the work of our research integrity team to safeguard the quality of each article we publish.
Find out more
ORIGINAL RESEARCH article
Front. Comput. Sci. , 15 November 2024
Sec. Computer Security
Volume 6 - 2024 | https://doi.org/10.3389/fcomp.2024.1473457
Introduction: The proliferation of social media platforms has facilitated the spread of fake news, posing significant risks to public perception and societal stability. Existing methods for multimodal fake news detection have made important progress in combining textual and visual information but still face challenges in effectively aligning and merging these different types of data. These challenges often result in incomplete or inaccurate feature representations, thereby limiting overall performance.
Methods: To address these limitations, we propose a novel framework named MCOT (Multimodal Fake News Detection with Contrastive Learning and Optimal Transport). MCOT integrates textual and visual information through three key components: cross-modal attention mechanism, contrastive learning, and optimal transport. Specifically, we first use cross-modal attention mechanism to enhance the interaction between text and image features. Then, we employ contrastive learning to align related embeddings while distinguishing unrelated pairs, and we apply optimal transport to refine the alignment of feature distributions across modalities.
Results: This integrated approach results in more precise and robust feature representations, thus enhancing detection accuracy. Experimental results on two public datasets demonstrate that the proposed MCOT outperforms state-of-the-art methods.
Discussion: Our future work will focus on improving its generalization and expanding its capabilities to additional modalities.
The widespread adoption of social media platforms has significantly transformed the way individuals share information and express their opinions. These platforms enable rapid and extensive dissemination of content, reaching vast audiences at minimal cost. However, their openness and ease of use have also facilitated the spread of fake news, posing significant risks to public perception and societal stability. Consequently, detecting and mitigating fake news has garnered considerable attention and research interest.
Analyzing textual content represents one of the earliest approaches to fake news detection efforts (Yu et al., 2017; Chen et al., 2019). However, as online social media content has rapidly evolved from purely text-based to multimodal, often containing text and images, the limitations of text-only approaches have become apparent. Studies have shown that analyzing cross-modal content can provide complementary advantages for fake news detection (Jin et al., 2016; Shu et al., 2017; Qi et al., 2019). As a result, numerous works have attempted to integrate multimodal features to enhance the performance of fake news detection models (Wang et al., 2018; Singhal et al., 2019; Khattar et al., 2019; Zhou et al., 2020; Wu et al., 2021; Xiao et al., 2023). These models often employ widely used and effective pre-trained models for feature extraction. In terms of feature fusion, the initial methods involved direct concatenation of feature vectors (Wang et al., 2018; Singhal et al., 2019), which have since evolved to include feature enhancement and fusion based on co-attention mechanisms (Wu et al., 2021; Xiao et al., 2023).
Despite the advancements in multimodal fake news detection, effectively aligning and integrating features from different modalities remains challenging. Text and image data possess distinct characteristics, making their combination inherently complex. Improper alignment and integration of these features can lead to suboptimal performance, underscoring the necessity for advanced techniques that can adeptly manage the intricacies of multimodal data fusion. To address these challenges, we introduce a novel framework for multimodal fake news detection, named MCOT (Multimodal Fake News Detection with Contrastive Learning and Optimal Transport). Among its components, contrastive learning, a technique widely used in representation learning (Guo Q. et al., 2023), helps improve the quality of learned embeddings by leveraging similarities and differences within the data. Optimal transport theory (Peyré et al., 2019), grounded in mathematics and economics, offers a robust method for aligning probability distributions.
More specifically, our approach begins with cross-modal attention mechanism to enhance the interaction between textual and visual features. Following this, contrastive learning is employed to align the embeddings of related pairs while distinguishing unrelated pairs. Additionally, optimal transport is used to refine the alignment of feature distributions from different modalities. By integrating these methods, MCOT effectively captures the complementary information from text and images, enhancing overall detection accuracy.
The key contributions of this paper are as follows:
• We propose the MCOT framework, which integrates contrastive learning and optimal transport to improve the alignment and integration of multimodal features.
• We utilize cross-modal attention mechanism to enhance the interaction between textual and visual features, effectively leveraging their complementary information.
• We conduct extensive experiments on the Weibo and Pheme datasets, demonstrating that our method achieves competitive performance and establishes a new benchmark in the field.
The remainder of this paper is structured as follows: Section 2 reviews related work in multimodal fake news detection, contrastive learning, and optimal transport. Section 3 details the proposed MCOT framework and its components. Section 4 presents the experimental setup and results, including comparisons with baseline methods and an ablation study. Finally, Section 5 concludes the paper.
Early approaches (Castillo et al., 2011; Pérez-Rosas et al., 2017) to fake news detection focus primarily on textual data and use single-modality techniques. With the increasing prevalence of images in news dissemination, recent works (Guo Y. et al., 2023; Lao et al., 2024; Zhu et al., 2024a,b) leverage deep learning models to extract features from both text and images and enhance the capability of fake news detection through various fusion strategies and additional tasks. For example, EANN (Wang et al., 2018) proposes an end-to-end framework that uses adversarial training to extract event-invariant features, enhancing the accuracy of detecting fake news related to new events. MVAE (Khattar et al., 2019) introduces a network combining a bimodal variational autoencoder and a binary classifier, identifying fake information by learning shared representations of multimedia content. CAFE (Chen et al., 2022) improves detection accuracy by performing cross-modal alignment, learning ambiguities between modalities, and capturing cross-modal correlations. LogicDM (Liu et al., 2023a) proposes a neural model that combines symbolic logic with neural representations to improve the interpretability of multimodal misinformation detection across various datasets.
Contrastive learning has emerged as a powerful technique in representation learning (Guo Q. et al., 2023; Liu and Chen, 2024), significantly advancing both natural language processing (NLP) (Yan et al., 2021; Gao et al., 2021; Hua et al., 2023; Gao and Das, 2024) and computer vision (CV) (He et al., 2020; Chen et al., 2020; Chen and He, 2021; Jiang et al., 2024). Contrastive learning effectively enhances the quality of learned representations by maximizing the agreement between related pairs and minimizing it between unrelated pairs. Recent works have demonstrated the effectiveness of contrastive learning in multimodal tasks. For instance, CLIP (Radford et al., 2021) uses contrastive learning to align visual and textual representations by predicting which caption matches a given image, enabling the model to perform zero-shot transfer to various downstream tasks without additional training. ALIGN (Jia et al., 2021) leverages a noisy dataset of over one billion image alt-text pairs to train a simple dual-encoder architecture that aligns visual and language representations using a contrastive loss. In contrast with previous works, our approach applies contrastive learning to align and distinguish multimodal features, focusing on cross-modal interactions to enhance fake news detection performance.
Optimal transport (OT) theory, grounded in mathematics and economics, has gained significant traction in machine learning for its ability to measure and align probability distributions (Peyré et al., 2019). By finding the most cost-effective way to transform one distribution into another, OT provides a powerful tool for various applications, including domain adaptation (Courty et al., 2017), generative modeling (Arjovsky et al., 2017), and representation learning (Xu and Chen, 2023). In multimodal learning, OT can align feature distributions from different modalities, ensuring that the learned representations capture the underlying relationships between modalities. MuLOT (Pramanick et al., 2022) leverages OT to align visual and textual representations for sarcasm and humor detection, improving performance on several benchmark datasets. TOT (Zhang et al., 2023) employs OT to address implicit harm in multimodal hate detection, achieving state-of-the-art performance on benchmark datasets by capturing complementary information from multiple modalities and eliminating the distributional modality gap. Unlike existing methods that use optimal transport for domain adaptation or distribution alignment, we integrate it with multimodal feature alignment, refining cross-modal representations to improve detection accuracy.
The task of multimodal fake news detection involves determining the veracity of a given news piece based on its textual content and accompanying image. In this section, we propose MCOT to address this problem. As shown in Figure 1, MCOT consists of five main modules: (a) Feature Extraction: extracts textual and visual features using specialized feature extractors. (b) Cross-Modal Attention: enhances the interaction between text and image features using multi-head attention. (c) Contrastive Learning: aligns textual and visual representations by computing similarity matrices. (d) Optimal Transport: models the alignment cost between text and image features using a cost matrix and transport plan. (e) Classification: produces final prediction scores using concatenated embeddings. The detailed introduction of each module is shown in the following subsections.
Figure 1. Model architecture overview of MCOT. (a) Feature extraction. (b) Cross-modal attention. (c) Contrastive learning. (d) Optimal transport. (e) Classification.
The input is represented as , where xt represents the textual component of the news item and xv denotes the corresponding image. The dataset comprises news snippets sourced from real-world social media platforms.
For the textual component xt, we employ a pre-trained BERT (Devlin et al., 2018) model to capture semantic and contextual representations accurately. The extracted features are denoted as , where m represents the number of tokens in the text and d represents the dimensionality of the embedding for each token.
Similarly, for the visual component xv, we utilize a pre-trained ViT (Dosovitskiy et al., 2020) model for preprocessing and encoding. The resulting features are represented as , where r indicates the number of image patches plus an additional [CLS] token, and d denotes the dimensionality of the embedding for each patch.
To effectively integrate textual and visual information, we employ the cross-modal attention mechanism. This mechanism allows the model to attend to the most relevant parts of both modalities, facilitating a more comprehensive understanding of the complementary relationships between text and image features. By integrating cross-modal attention, our model can better capture the subtle clues embedded in multimodal content and make more informed predictions.
The cross-modal attention mechanism consists of two parallel multi-head attention layers: one for focusing on text features using image features as queries, and the other for attending to image features using text features as queries. The multi-head attention mechanism is a fundamental component of the Transformer (Vaswani et al., 2017) architecture, enabling the model to focus on different parts of the input sequence simultaneously.
The basic principle of multi-head attention involves computing queries (Q), keys (K), and values (V) from the input features. The attention distribution is calculated by the dot product similarity between Q and K, followed by scaling and applying it to V. Formally, the attention output for a single head is computed as:
where dh is the dimensionality of each head's output feature. In the multi-head attention mechanism, multiple heads operate in parallel, enabling the model to capture various aspects of the input features. The outputs of these heads are then concatenated and linearly transformed.
In this module, we implement cross-modal attention by swapping the roles of keys (K) and values (V) between text and image features. Queries from each modality are fed into the multi-head attention block of the other modality, producing image-enhanced textual features ht→v and text-enhanced visual features hv→t.
The enhanced text and image features, and , are then obtained by applying residual connections followed by layer normalization:
The enhanced features and serve as the foundation for generating the final embeddings used in classification. The process involves several steps to aggregate and transform these features into compact, informative representations.
First, we extract the [CLS] token features from the enhanced features, which represent the overall information of the sequence.
Additionally, to capture global context, we perform average pooling on the enhanced features to get the pooled features .
For each modality, the [CLS] token feature and the average-pooled features are concatenated to form comprehensive feature vectors for both text and image with a dimension of 2d:
These concatenated features are then passed through fully connected layers with GELU activation functions to transform the dimension to d′, resulting in the final embeddings :
where Wt, Wv are learnable weight parameters, bt, bv are bias terms, and σ(·) represents the GELU activation function.
The goal of contrastive learning is to create a feature space where similar samples are close together while dissimilar samples are far apart. This is particularly effective for multimodal fake news detection, where aligning textual and visual representations is crucial. By leveraging contrastive learning, we can better align the representations of both modalities, reducing the modality gap and improving the model's ability to effectively integrate multimodal information for more accurate fake news detection.
Building on the design principles from existing work (Zhan et al., 2021; Wang et al., 2023) on contrastive learning in multimodal settings, we utilize the final embeddings et and ev to enhance feature alignment.
For a batch of N text-image samples, the text features and image features are defined as and , respectively. We consider the corresponding text-image pairs as N positive pairs, and the remaining N2−N unmatched pairs as negative pairs.
Following the approach of Wang et al. (2023), we compute similarity scores using the dot product of the feature vectors, scaled by a temperature parameter τ. This yields two similarity matrices: one for text-to-vision similarity pt→v and one for vision-to-text similarity pv→t:
The contrastive loss measures the difference between predicted similarity scores and actual labels. The loss for each direction, image-to-text and text-to-image, is computed using cross-entropy loss. Specifically, the cross-entropy loss for the vision-to-text direction and text-to-vision direction can be written as:
where yv→t and yt→v represent the ground-truth labels, which are 1 for positive pairs and 0 for negative pairs.
The final contrastive learning loss is calculated as the average of the individual losses from both directions:
This subsection models the optimal transport problem as a distance metric between two probability distributions. Specifically, we treat the text feature space as the target space and the image feature space as the source space. By measuring the distance between these two distributions, we align features from different modalities into a common space. The motivation for using optimal transport lies in its ability to accurately quantify the differences between heterogeneous modalities, providing a precise and effective way to reduce distributional discrepancies between text and image features.
Using the text features and image features defined in Section 3.3, we proceed with the following steps:
First, we define the cost matrix C ∈ ℝd′ × d′, where Cij represents the cost of transporting the text feature of the i-th news item to the image feature of the j-th news item. The cost matrix is calculated as , where denotes the squared Euclidean distance.
Assuming μ and ν are the probability distributions of the text feature space and image feature space, respectively, satisfying , , and ∀i, j, μi ≥ 0, νj ≥ 0. The solution space of the transport plan P is defined as:
where 1N is an N-dimensional column vector of ones.
Thus, the optimal transport problem from the image feature space to the text feature space can be expressed as:
where 〈·, ·〉F represents the Frobenius inner product.
Next, by introducing the entropy regularization term, the optimization objective becomes:
where denotes the entropy of P, and λ is the entropy regularization parameter.
We then use the Sinkhorn (Cuturi, 2013) iterative algorithm to solve for the optimal transport matrix and employ the Sinkhorn distance as the alignment loss, which measures the minimal transport cost of converting the image feature distribution to the text feature distribution:
The classifier's input is formed by concatenating the final embeddings et and ev. The concatenated features are then passed through a fully connected layer and a sigmoid activation function to produce the final prediction scores:
Since fake news detection is a binary classification task, we apply the binary cross-entropy loss over all labeled pairs between the ground-truth labels y and the predicted scores ŷ:
The final loss function combines the classification loss , the contrastive learning loss , and the optimal transport loss . This combination ensures that the model accurately classifies the news as real or fake and effectively aligns the text and image features. The overall loss function is defined as:
where β and γ are hyper-parameters that balance the three terms.
By jointly optimizing these three loss terms, the model improves its classification performance while simultaneously ensuring robust feature alignment and effective feature transport between modalities.
To verify the effectiveness of our approach, we conduct experiments on two benchmark real-world multimodal datasets: Weibo (Jin et al., 2017) and Pheme (Zubiaga et al., 2017). These datasets contain news represented by text and images. The statistics of the two datasets are shown in Table 1, and the details are as follows:
1. The Weibo dataset is sourced from the Chinese Sina Weibo platform, with fake posts collected from the official rumor debunking system of Weibo and real posts verified by Xinhua News Agency, an authoritative news agency in China.
2. The Pheme dataset is created by collecting tweets related to five breaking news events on the Twitter platform. Each data entry includes text and images, along with social context information that we do not use in this experiment.
Both datasets are split into a training set and a test set with an 8:2 ratio. For Weibo, we adopt the same division method as the existing work (Wang et al., 2018). For Pheme, the data is divided randomly. In both datasets, following previous works (Chen et al., 2022; Liu et al., 2023b), only samples containing both text and image are retained.
To validate the effectiveness of the proposed MCOT framework, we compare it with several representative methods:
• EANN (Wang et al., 2018): it uses an event adversarial neural network to learn event-invariant features based on extracting multimodal features.
• MVAE (Khattar et al., 2019): it employs a multimodal variational autoencoder coupled with a binary classifier to learn shared textual and visual representations.
• SAFE (Zhou et al., 2020): it uses a similarity-aware approach to investigate the relationship between textual and visual information in news articles.
• SpotFake+ (Singhal et al., 2020): it uses a multimodal approach leveraging transfer learning to capture semantic and contextual information from full-length news articles and associated images.
• MCNN (Xue et al., 2021): it proposes a neural network that captures the consistency of multimodal data, addressing issues like text-image mismatches and image tampering.
• CAFE (Chen et al., 2022): it introduces a cross-modal ambiguity-aware approach to adaptively aggregate unimodal features and cross-modal correlations.
• BMR (Ying et al., 2023): it uses improved multi-gate Mixture-of-Experts (MoE) networks to learn features through single-view prediction and cross-modal consistency learning.
• LogicDM (Liu et al., 2023a): it introduces a logic-based neural model combining neural networks with symbolic learning to enhance interpretability.
For the text feature extraction, we use the pre-trained bert-base-chinese1 model for the Weibo dataset and the pre-trained roberta-base2 model for the Pheme dataset. The maximum sequence length for text is set to 150 for Weibo and 60 for Pheme, respectively. For the image feature extraction, we employ the pre-trained vit-base-patch16-3843 model. The embedding dimension d for both modalities is 768.
For the cross-modal attention mechanism, we set the number of heads to 8 for each multi-head attention layer. For the final embeddings, both textual and visual features are processed through a fully connected layer with a hidden size of 64, resulting in d′ of 64.
During training, we set the batch size to 64 and use the Adam optimizer with an initial learning rate of 0.001. Training is conducted for 50 epochs with an early stopping strategy. We perform a grid search in the range of (0, 1) with a step size of 0.1 to find the optimal hyperparameters for β and γ. Ultimately, for the Weibo dataset, we set β and γ to 0.4; for the Pheme dataset, we set both β and γ to 0.1.
We evaluate the models using Accuracy (Acc), Precision (P), Recall (R), and F1-score (F1). Table 2 presents the performance comparison between our proposed MCOT framework and other baseline methods on the Weibo and Pheme datasets. MCOT consistently outperforms most compared methods in terms of accuracy and F1-score on both datasets, demonstrating its effectiveness.
On the Weibo dataset, recent methods (BMR, LogicDM, and MCOT) show significant performance improvements over earlier methods, partly due to the use of advanced pre-trained models. Earlier methods typically use text-CNN for text feature extraction, which has limitations in capturing dependencies between distantly related words. This limitation is less pronounced on the Pheme dataset, possibly due to the generally shorter text lengths.
Notably, MCOT achieves better performance in detecting real news on the Pheme dataset. However, it slightly lags in recall and F1-score for fake news compared to the best-performing method. This can be attributed to the imbalance between positive and negative samples within the dataset, reflecting a limitation of MCOT in addressing this issue. Nevertheless, MCOT still demonstrates outstanding overall performance, underscoring its capability in managing multimodal data.
To further investigate the effectiveness of each component in MCOT, we first introduce a base model, which simply concatenates the outputs of the pre-trained models (BERT and ViT) without utilizing the cross-modal attention, contrastive learning, or optimal transport modules. This base model serves as a foundation to understand the contribution of pre-trained models alone.
In addition, we design three simplified variants of MCOT. More specifically, the compared variants of MCOT are implemented as follows: MCOT w/o CA removes the cross-modal attention module, skipping the interaction between text and image features, and obtaining the final embeddings separately. MCOT w/o CL removes the contrastive learning module, meaning the contrastive loss is not considered during training. MCOT w/o OT removes the optimal transport module, meaning the optimal transport loss is not considered during training.
From the results shown in Table 3, we have the following observations:
The base model, while insufficient to fully address the challenges of multimodal fake news detection, demonstrates the strong foundational contribution of pre-trained models to the MCOT framework, especially on the Weibo dataset.
The subsequent ablation experiments further illustrate the contributions of each individual component of the MCOT framework.
On the Pheme dataset, removing the cross-modal attention module leads to a notable decrease in performance, highlighting the importance of capturing the interaction between textual and visual features. The variant without contrastive learning also shows reduced performance, indicating that contrastive learning enhances feature representation effectively. Similarly, the variant without the optimal transport module demonstrates lower accuracy and F1-score, underscoring the role of optimal transport in aligning feature distributions.
On the Weibo dataset, a similar trend is observed. This consistency across datasets emphasizes the robustness and generalizability of the MCOT framework. The cross-modal attention mechanism, contrastive learning, and optimal transport all contribute to the model's ability to accurately detect fake news by leveraging the complementary nature of multimodal data.
To illustrate the effectiveness of the MCOT framework, we conduct a visualization experiment comparing the complete MCOT model with the MCOT w/o COT variant, which removes both the contrastive learning and optimal transport modules. We visualize the features using t-SNE (Van der Maaten and Hinton, 2008) for 500 randomly sampled news items from the test set of Weibo, plotting at the last epoch of training for both models. The results are shown in Figure 2.
Figure 2. Visualization of text and image features generated by: (a) MCOT w/o COT; (b) MCOT; and visualization of real and fake news features generated by: (c) MCOT w/o COT; (d) MCOT.
In the first set of visualization (subplots a and b), each news item is represented by two points: one for text features (red) and one for image features (blue). In the second set of visualization (subplots c and d), we concatenate the text and image features for each news item and plot a single point, with colors indicating whether the news is real (green) or fake (yellow).
The t-SNE plots reveal distinct differences between the two models. For the text and image features, the MCOT model (subplot b) exhibits much tighter clustering compared to the MCOT w/o COT variant (subplot a). This indicates that contrastive learning and optimal transport effectively align and integrate multimodal features. While it is theoretically challenging to assert that this tighter clustering directly translates to better performance, our results suggest that this alignment is beneficial.
For the combined features, the distinction between real and fake news is more apparent in the MCOT model (subplot d) compared to the variant (subplot c). Even though the difference is not as pronounced as in the individual feature plots, the clearer separation of real and fake news points to the effectiveness of the combined learning strategy in the MCOT model.
In this article, we proposed a novel MCOT framework for fake news detection, which effectively integrates textual and visual information through cross-modal attention, contrastive learning, and optimal transport. Experimental results on the Weibo and Pheme datasets demonstrate the superior performance of MCOT compared to several advanced methods. The ablation study further confirms the importance of each component in the MCOT framework, showing that the combination of cross-modal attention, contrastive learning, and optimal transport enhances the model's ability to detect fake news. These findings highlight the potential of leveraging multimodal data and advanced learning techniques to improve the reliability and accuracy of fake news detection systems.
However, there are potential limitations to this work. First, the MCOT framework relies on pre-trained models for both text and image feature extraction. While these models improve performance, their generalization to domains that differ from their training data may be limited. Second, the Weibo and Pheme datasets, while widely used benchmarks, are relatively small in scale and may not fully capture the diversity of real-world multimodal fake news.
As this is one of the first works to leverage contrastive learning and optimal transport for multimodal fake news detection, further exploration is necessary to fully realize the potential of this approach. In the future, we plan to explore: (1) reducing the computational complexity of MCOT by employing model distillation or lightweight architectures, making it more suitable for resource-constrained environments; (2) extending the MCOT framework to more modalities, such as video and audio, to further enhance its capability in detecting multimodal fake news; (3) exploring the adaptability of MCOT across different languages and social media platforms to improve its generalizability, potentially achieved through multilingual pre-trained models or cross-domain transfer learning.
The original contributions presented in the study are included in the article/supplementary material, further inquiries can be directed to the corresponding author.
XS: Funding acquisition, Methodology, Project administration, Writing – original draft. MH: Data curation, Formal analysis, Methodology, Writing – original draft. ZH: Investigation, Validation, Writing – review & editing. SC: Funding acquisition, Project administration, Supervision, Writing – original draft, Writing – review & editing. TZ: Funding acquisition, Resources, Supervision, Writing – review & editing.
The author(s) declare financial support was received for the research, authorship, and/or publication of this article. This work was supported by the Ministry of Education of Humanities and Social Science Project, China (Grant No. 21JZD055).
We would like to express our deepest gratitude to the editors and all the reviewers for their invaluable help and support throughout the review process. We also wish to acknowledge the assistance of ChatGPT (version: GPT-4, source: OpenAI) in the translation, language refinement, and proofreading processes during the preparation of this manuscript.
The authors declare that the research was conducted in the absence of any commercial or financial relationships that could be construed as a potential conflict of interest.
All claims expressed in this article are solely those of the authors and do not necessarily represent those of their affiliated organizations, or those of the publisher, the editors and the reviewers. Any product that may be evaluated in this article, or claim that may be made by its manufacturer, is not guaranteed or endorsed by the publisher.
1. ^https://huggingface.co/google-bert/bert-base-chinese
Arjovsky, M., Chintala, S., and Bottou, L. (2017). “Wasserstein generative adversarial networks,” in International Conference on Machine Learning (New York: PMLR), 214–223.
Castillo, C., Mendoza, M., and Poblete, B. (2011). “Information credibility on twitter,” in Proceedings of the 20th International Conference on World Wide Web (New York, NY: Association for Computing Machinery), 675–684.
Chen, T., Kornblith, S., Norouzi, M., and Hinton, G. (2020). “A simple framework for contrastive learning of visual representations,” in International Conference on Machine Learning (New York: PMLR), 1597–1607.
Chen, X., and He, K. (2021). “Exploring simple siamese representation learning,” in Proceedings of the IEEE/CVF Conference on Computer Vision and Pattern Recognition (Piscataway, NJ: IEEE), 15750–15758.
Chen, Y., Li, D., Zhang, P., Sui, J., Lv, Q., Tun, L., et al. (2022). “Cross-modal ambiguity learning for multimodal fake news detection,” in Proceedings of the ACM Web Conference 2022 (New York, NY: Association for Computing Machinery), 2897–2905.
Chen, Y., Sui, J., Hu, L., and Gong, W. (2019). “Attention-residual network with cnn for rumor detection,” in Proceedings of the 28th ACM International Conference on Information and Knowledge Management (New York, NY: Association for Computing Machinery), 1121–1130.
Courty, N., Flamary, R., Habrard, A., and Rakotomamonjy, A. (2017). “Joint distribution optimal transportation for domain adaptation,” in Advances in Neural Information Processing Systems 30 (Red Hook, NY: Curran Associates, Inc.).
Cuturi, M. (2013). “Sinkhorn distances: lightspeed computation of optimal transport,” in Advances in Neural Information Processing Systems 26 (Red Hook, NY: Curran Associates, Inc.).
Devlin, J., Chang, M.-W., Lee, K., and Toutanova, K. (2018). Bert: Pre-training of deep bidirectional transformers for language understanding. arXiv [preprint] arXiv:1810.04805. doi: 10.48550/arXiv.1810.04805
Dosovitskiy, A., Beyer, L., Kolesnikov, A., Weissenborn, D., Zhai, X., Unterthiner, T., et al. (2020). An image is worth 16x16 words: transformers for image recognition at scale. arXiv [preprint] arXiv:2010.11929. doi: 10.48550/arXiv.2010.11929
Gao, T., Yao, X., and Chen, D. (2021). Simcse: simple contrastive learning of sentence embeddings. arXiv [preprint] arXiv:2104.08821. doi: 10.18653/v1/2021.emnlp-main.552
Gao, X., and Das, K. (2024). Customizing language model responses with contrastive in-context learning. Proc. AAAI Conf. Artif. Intellig. 38, 18039–18046. doi: 10.1609/aaai.v38i16.29760
Guo, Q., Liao, Y., Li, Z., and Liang, S. (2023). Multi-modal representation via contrastive learning with attention bottleneck fusion and attentive statistics features. Entropy 25:1421. doi: 10.3390/e25101421
Guo, Y., Ge, H., and Li, J. (2023). A two-branch multimodal fake news detection model based on multimodal bilinear pooling and attention mechanism. Front. Comp. Sci. 5:1159063. doi: 10.3389/fcomp.2023.1159063
He, K., Fan, H., Wu, Y., Xie, S., and Girshick, R. (2020). “Momentum contrast for unsupervised visual representation learning,” in Proceedings of the IEEE/CVF Conference on Computer Vision and Pattern Recognition (Seattle, WA: IEEE), 9729–9738.
Hua, J., Cui, X., Li, X., Tang, K., and Zhu, P. (2023). Multimodal fake news detection through data augmentation-based contrastive learning. Appl. Soft Comp. 136:110125. doi: 10.1016/j.asoc.2023.110125
Jia, C., Yang, Y., Xia, Y., Chen, Y.-T., Parekh, Z., Pham, H., et al. (2021). “Scaling up visual and vision-language representation learning with noisy text supervision,” in International Conference on Machine Learning (New York: PMLR), 4904–4916.
Jiang, M., Su, Y., Gao, L., Plaza, A., Zhao, X.-L., Sun, X., et al. (2024). Graphgst: Graph generative structure-aware transformer for hyperspectral image classification. IEEE Trans. Geosci. Remote Sens. 62, 1–16. doi: 10.1109/TGRS.2023.3349076
Jin, Z., Cao, J., Guo, H., Zhang, Y., and Luo, J. (2017). “Multimodal fusion with recurrent neural networks for rumor detection on microblogs,” in Proceedings of the 25th ACM international conference on Multimedia (New York, NY: Association for Computing Machinery), 795–816.
Jin, Z., Cao, J., Zhang, Y., Zhou, J., and Tian, Q. (2016). Novel visual and statistical image features for microblogs news verification. IEEE Trans. Multimed. 19, 598–608. doi: 10.1109/TMM.2016.2617078
Khattar, D., Goud, J. S., Gupta, M., and Varma, V. (2019). “MVAE: multimodal variational autoencoder for fake news detection,” in The World Wide Web Conference (New York, NY: Association for Computing Machinery), 2915–2921.
Lao, A., Zhang, Q., Shi, C., Cao, L., Yi, K., Hu, L., et al. (2024). Frequency spectrum is more effective for multimodal representation and fusion: a multimodal spectrum rumor detector. Proc. AAAI Conf. Artif. Intellig. 38, 18426–18434. doi: 10.1609/aaai.v38i16.29803
Liu, H., Wang, W., and Li, H. (2023a). “Interpretable multimodal misinformation detection with logic reasoning,” in Findings of the Association for Computational Linguistics: ACL 2023, eds. A. Rogers, J. Boyd-Graber, and N. Okazaki (Toronto: Association for Computational Linguistics), 9781–9796.
Liu, H., Wang, W., Sun, H., Rocha, A., and Li, H. (2023b). Robust domain misinformation detection via multi-modal feature alignment. IEEE Trans. Inform. Forens. Secur. 19, 793–806. doi: 10.1109/TIFS.2023.3326368
Liu, J., and Chen, S. (2024). Timesurl: Self-supervised contrastive learning for universal time series representation learning. Proc. AAAI Conf. Artif. Intellig. 38, 13918–13926. doi: 10.1609/aaai.v38i12.29299
Pérez-Rosas, V., Kleinberg, B., Lefevre, A., and Mihalcea, R. (2017). Automatic detection of fake news. arXiv [preprint] arXiv:1708.07104. doi: 10.48550/arXiv.1708.07104
Peyré, G., and Cuturi, M. (2019). Computational optimal transport: with applications to data science. Found. Trends Mach. Learn. 11, 355–607. doi: 10.1561/9781680835519
Pramanick, S., Roy, A., and Patel, V. M. (2022). “Multimodal learning using optimal transport for sarcasm and humor detection,” in Proceedings of the IEEE/CVF Winter Conference on Applications of Computer Vision (Waikoloa, HI: IEEE), 3930–3940. doi: 10.1109/WACV51458.2022.00062
Qi, P., Cao, J., Yang, T., Guo, J., and Li, J. (2019). “Exploiting multi-domain visual information for fake news detection,” in 2019 IEEE International Conference on Data Mining (ICDM) (Beijing: IEEE), 518–527.
Radford, A., Kim, J. W., Hallacy, C., Ramesh, A., Goh, G., Agarwal, S., et al. (2021). “Learning transferable visual models from natural language supervision,” in International Conference on Machine Learning (New York: PMLR), 8748–8763.
Shu, K., Sliva, A., Wang, S., Tang, J., and Liu, H. (2017). Fake news detection on social media: a data mining perspective. ACM SIGKDD Explorat. Newslett. 19, 22–36. doi: 10.1145/3137597.3137600
Singhal, S., Kabra, A., Sharma, M., Shah, R. R., Chakraborty, T., and Kumaraguru, P. (2020). Spotfake+: a multimodal framework for fake news detection via transfer learning (student abstract). Proc. AAAI Conf. Artif. Intellig. 34, 13915–13916. doi: 10.1609/aaai.v34i10.7230
Singhal, S., Shah, R. R., Chakraborty, T., Kumaraguru, P., and Satoh, S. (2019). “Spotfake: A multi-modal framework for fake news detection,” in 2019 IEEE Fifth International Conference on Multimedia Big Data (BigMM) (Singapore: IEEE), 39–47.
Vaswani, A., Shazeer, N., Parmar, N., Uszkoreit, J., Jones, L., Gomez, A. N., et al. (2017). “Attention is all you need,” in Advances in Neural Information Processing Systems 30.
Wang, L., Zhang, C., Xu, H., Xu, Y., Xu, X., and Wang, S. (2023). “Cross-modal contrastive learning for multimodal fake news detection,” in Proceedings of the 31st ACM International Conference on Multimedia (New York, NY: Association for Computing Machinery), 5696–5704.
Wang, Y., Ma, F., Jin, Z., Yuan, Y., Xun, G., Jha, K., et al. (2018). “EANN: Event adversarial neural networks for multi-modal fake news detection,” in Proceedings of the 24th ACM SIGKDD International Conference on Knowledge Discovery & Data Mining (New York, NY: Association for Computing Machinery), 849–857.
Wu, Y., Zhan, P., Zhang, Y., Wang, L., and Xu, Z. (2021). “Multimodal fusion with co-attention networks for fake news detection,” in Findings of the Association for Computational Linguistics: ACL-IJCNLP 2021 (Stroudsburg, PA: Association for Computational Linguistics), 2560–2569.
Xiao, T., Guo, S., Huang, J., Spolaor, R., and Cheng, X. (2023). “HiPo: Detecting fake news via historical and multi-modal analyses of social media posts,” in Proceedings of the 32nd ACM International Conference on Information and Knowledge Management (New York, NY: Association for Computing Machinery), 2805–2815.
Xu, Y., and Chen, H. (2023). “Multimodal optimal transport-based co-attention transformer with global structure consistency for survival prediction,” in Proceedings of the IEEE/CVF International Conference on Computer Vision, (Paris: IEEE), 21241–21251. doi: 10.1109/ICCV51070.2023.01942
Xue, J., Wang, Y., Tian, Y., Li, Y., Shi, L., and Wei, L. (2021). Detecting fake news by exploring the consistency of multimodal data. Inform. Proc. Manage. 58:102610. doi: 10.1016/j.ipm.2021.102610
Yan, Y., Li, R., Wang, S., Zhang, F., Wu, W., and Xu, W. (2021). Consert: a contrastive framework for self-supervised sentence representation transfer. arXiv [preprint] arXiv:2105.11741. doi: 10.18653/v1/2021.acl-long.393
Ying, Q., Hu, X., Zhou, Y., Qian, Z., Zeng, D., and Ge, S. (2023). Bootstrapping multi-view representations for fake news detection. Proc. AAAI conf. Artif. Intellig. 37, 5384–5392. doi: 10.1609/aaai.v37i4.25670
Yu, F., Liu, Q., Wu, S., Wang, L., Tan, T., et al. (2017). A convolutional approach for misinformation identification. IJCAI. 2017, 3901–3907. doi: 10.24963/ijcai.2017/545
Zhan, X., Wu, Y., Dong, X., Wei, Y., Lu, M., Zhang, Y., et al. (2021). “Product1m: Towards weakly supervised instance-level product retrieval via cross-modal pretraining,” in Proceedings of the IEEE/CVF International Conference on Computer Vision (Montreal, QC: IEEE), 11782–11791.
Zhang, L., Jin, L., Sun, X., Xu, G., Zhang, Z., Li, X., et al. (2023). TOT: topology-aware optimal transport for multimodal hate detection. Proc. AAAI Conf. Artif. Intellig. 37, 4884–4892. doi: 10.1609/aaai.v37i4.25614
Zhou, X., Wu, J., and Zafarani, R. (2020). “SAFE: similarity-aware multi-modal fake news detection,” in Advances in Knowledge Discovery and Data Mining - 24th Pacific-Asia Conference, PAKDD 2020, Singapore, May 11-14, 2020, Proceedings, Part II, volume 12085 of Lecture Notes in Computer Science (Singapore: Springer), 354–367.
Zhu, P., Hua, J., Tang, K., Tian, J., Xu, J., and Cui, X. (2024a). Multimodal fake news detection through intra-modality feature aggregation and inter-modality semantic fusion. Comp. Intellig. Syst. 2024, 1–13. doi: 10.1007/s40747-024-01473-5
Zhu, P., Pan, Z., Liu, Y., Tian, J., Tang, K., and Wang, Z. (2024b). “A general black-box adversarial attack on graph-based fake news detectors,” in Proceedings of the Thirty-Third International Joint Conference on Artificial Intelligence, IJCAI-24, ed. K. Larson (California: International Joint Conferences on Artificial Intelligence Organization), 568–576.
Keywords: fake news detection, multimodal data, cross-modal attention, contrastive learning, optimal transport
Citation: Shen X, Huang M, Hu Z, Cai S and Zhou T (2024) Multimodal Fake News Detection with Contrastive Learning and Optimal Transport. Front. Comput. Sci. 6:1473457. doi: 10.3389/fcomp.2024.1473457
Received: 31 July 2024; Accepted: 28 October 2024;
Published: 15 November 2024.
Edited by:
Stelvio Cimato, University of Milan, ItalyReviewed by:
Wenqian Shang, Communication University of China, ChinaCopyright © 2024 Shen, Huang, Hu, Cai and Zhou. This is an open-access article distributed under the terms of the Creative Commons Attribution License (CC BY). The use, distribution or reproduction in other forums is permitted, provided the original author(s) and the copyright owner(s) are credited and that the original publication in this journal is cited, in accordance with accepted academic practice. No use, distribution or reproduction is permitted which does not comply with these terms.
*Correspondence: Shimin Cai, c2hpbWluY2FpQHVlc3RjLmVkdS5jbg==
†These authors have contribute equally to this work
Disclaimer: All claims expressed in this article are solely those of the authors and do not necessarily represent those of their affiliated organizations, or those of the publisher, the editors and the reviewers. Any product that may be evaluated in this article or claim that may be made by its manufacturer is not guaranteed or endorsed by the publisher.
Research integrity at Frontiers
Learn more about the work of our research integrity team to safeguard the quality of each article we publish.