- 1Department of Computer Science and Engineering, United International University, Dhaka, Bangladesh
- 2Faculty of Science and Technology, Charles Darwin University, Darwin, NT, Australia
- 3Department of Information Technology, Australian Catholic University, North Sydney, NSW, Australia
Introduction: An automated computerized approach can aid radiologists in the early diagnosis of lung disease from video modalities. This study focuses on the difficulties associated with identifying and categorizing respiratory diseases, including COVID-19, influenza, and pneumonia.
Methods: We propose a novel method that combines three dimensional (3D) models, model explainability (XAI), and a Decision Support System (DSS) that utilizes lung ultrasound (LUS) videos. The objective of the study is to improve the quality of video frames, boost the diversity of the dataset, maintain the sequence of frames, and create a hybrid 3D model [Three-Dimensional Time Distributed Convolutional Neural Network-Long short-term memory (TD-CNNLSTM-LungNet)] for precise classification. The proposed methodology involves applying morphological opening and contour detection to improve frame quality, utilizing geometrical augmentation for dataset balance, introducing a graph-based approach for frame sequencing, and implementing a hybrid 3D model combining time-distributed CNN and LSTM networks utilizing vast ablation study. Model explainability is ensured through heatmap generation, region of interest segmentation, and Probability Density Function (PDF) graphs illustrating feature distribution.
Results: Our model TD-CNN-LSTM-LungNet attained a remarkable accuracy of 96.57% in classifying LUS videos into pneumonia, COVID-19, normal, and other lung disease classes, which is above compared to ten traditional transfer learning models experimented with in this study. The eleven-ablation case study reduced training costs and redundancy. K-fold cross-validation and accuracy-loss curves demonstrated model generalization. The DSS, incorporating Layer Class Activation Mapping (LayerCAM) and heatmaps, improved interpretability and reliability, and PDF graphs facilitated precise decision-making by identifying feature boundaries. The DSS facilitates clinical marker analysis, and the validation by using the proposed algorithms highlights its impact on a reliable diagnosis outcome.
Discussion: Our proposed methodology could assist radiologists in accurately detecting and comprehending the patterns of respiratory disorders.
1 Introduction
Respiratory diseases such as COVID-19 and pneumonia vary in severity, impacting the monitoring of their spread. Every year, pneumonia leads to over one million hospitalizations and more than 50,000 fatalities (American Lung Association, 2022). Pneumonia is a pathological condition characterized by infection and inflammation in either one or both lungs, resulting in fluid accumulation (Kruckow et al., 2023). It can potentially hinder the process of oxygen exchange. Pneumonia severity depends on the cause, patient’s age, and overall health, with symptoms ranging from mild to life-threatening (Kassaw et al., 2023). The severity of COVID-19 can vary, ranging from modest symptoms to severe sickness and even death (Kapusta et al., 2023). Older adults and individuals with existing medical issues are more susceptible to experiencing severe results (Kapusta et al., 2023). The World Health Organization (WHO) reported a total of 396,558,014 confirmed cases of COVID-19 globally, resulting in 5,745,032 fatalities. COVID-19 is a highly contagious disease that is closely connected to severe acute respiratory syndrome (Rao et al., 2024). The complex characteristics and mutations of the COVID-19 virus provide significant difficulties in promptly identifying patients (Kapusta et al., 2023). Influenza and other respiratory diseases can cause illnesses ranging from mild to severe, with the severity differing among various age groups and communities (Ambrosch et al., 2023).
Various modalities are available for the detection of lung disease, including computed tomography (CT), LUS (LUS) images and video, and chest X-rays (Philip et al., 2023). LUS video is valuable for monitoring the progression of respiratory illnesses because it provides continuous motion. This technology enables quick and non-invasive evaluation of lung abnormalities, including pneumonia and lung damage caused by COVID-19. It assists in the early detection and monitoring of disease progression (Dugar et al., 2023; Philip et al., 2023). Moreover, it can be utilized for immediate direction during medical operations such as thoracentesis and endotracheal intubation, improving patient care quality in managing respiratory diseases.
The utilization of computer-aided approaches in LUS videos helps accelerate the classification process of respiratory disorders. Computer-aided ultrasound analysis can utilize artificial intelligence (AI) algorithms to detect and classify lung abnormalities automatically. This integration could decrease the time required for interpretation and enhance the accuracy of diagnosis. Moreover, 3D deep learning models have demonstrated the potential to improve respiratory disease diagnosis significantly compared to alternative approaches (Wu et al., 2023). These models can analyze volumetric ultrasound data, allowing for a thorough evaluation of lung pathology and enhancing the accuracy of disease classification and monitoring. After getting an optimal result, ensuring the transparency and comprehensibility of models is essential for establishing user confidence and facilitating the efficacy of decision support systems, especially in the context of medical artificial intelligence (Panigutti et al., 2023). The deep learning model must be explained in complex AI-based solutions, such as respiratory disease prediction, to foster trust among clinicians, developers, and researchers.
In this regard, several studies have incorporated LUS videos (Barros et al., 2021; Diaz-Escobar et al., 2021; Ebadi et al., 2021; Li et al., 2023; Magrelli et al., 2021; Muhammad and Hossain, 2021; Roy et al., 2020; Shea et al., 2023; Tsai et al., 2021), however, a notable gap in the literature was identified in incorporating 3D models for enhanced visualization and understanding. Moreover, the absence of integrating explainability into prior investigations was a significant oversight in the decision-making process. To address the significant shortcomings found in previous studies, our research aims to fill these gaps by including three-dimensional models in the analysis of LUS videos, introducing model explainability, and proposing a Decision Support System (DSS).
Following are the contributions made about the proposed approach:
• To enhance the quality of the frames of a video, we applied morphological opening and largest contour detection techniques to eliminate unwanted artifacts and noise, enabling the model to extract meaningful features effectively.
• We utilize geometrical augmentation techniques to increase the frame number of the videos, balance the dataset, and increase the diversity of the samples as well.
• To preserve the sequence of the frames, we introduced a graph-based approach. In this regard, Mean Squared Error (MSE), Structural Similarity Index (SSIM), and Minimum Spanning Tree (MST) based approaches establish the frame sequence and continuity.
• After a vast ablation study, we proposed a hybrid model Three-Dimensional Time Distributed Convolutional Neural Network-Long short-term memory (TD-CNN-LSTM-LungNet) by combining a time-distributed convolutional neural network (TD-CNN) and a long short-term memory (LSTM) network, which can capture spatial–temporal dependency and improve contextual learning from the LUS videos.
• To ensure the explainability of the model, a heatmap within the LUS frames and highlights the ROI of the frames that contribute to the model’s prediction has been generated.
• The region of interest (ROI) from the video frame is segmented to extract the crucial features of that region to create PDF graphs.
• The PDF graph illustrates the feature distribution to the corresponding classes and leads to creating a precise DSS for the decision-making process.
2 Related works
The increasing severity of lung diseases has prompted a rise in research efforts, with numerous researchers actively exploring the integration of computer-aided diagnostics (CAD) to enhance the accuracy and efficiency of lung disease prediction. These efforts aim to transform early identification and improve the treatment of respiratory conditions by recognizing the crucial requirement for enhanced technologies.
In this regard, Tsai et al. (2021) proposed an automatic deep learning-based method for classifying pleural effusions in LUS images and videos to diagnose respiratory diseases on a custom dataset. Preprocessing techniques include digital imaging and communications in medicine processing, color space conversion, overlay removal, and image cropping have been performed. The mean accuracy of the video-based labeling approach was 91.12%, while the frame-based labeling approach achieved 92.38, 1.26% higher than the video-based labeling approach. In another study, Muhammad and Hossain (2021) recommended using multi-layer fusion from LUS videos to classify between COVID-19 and non-COVID-19. Mean normalization and standardization were performed throughout the dataset using calculated standard deviation and mean for preprocessing. It employed several augmentation techniques (Shamrat et al., 2022), such as scaling, rotations, and random reflections. The proposed efficient CNN model with five blocks of convolution connectors and multi-layer feature fusion attained an accuracy of 91.8%. Ebadi et al. (2021) developed an automated method for detecting pneumonia in LUS utilizing deep video classification for COVID-19. They proposed a Two-Stream Inflated 3D ConvNet (I3D) for classifying video sequences with a 90% accuracy and a 95% average precision score. To classify LUS features related to pneumonia in videos using deep learning (Shea et al., 2023) worked. Every image was converted to grayscale and resized to a particular input size for preprocessing, and standard data augmentation techniques such as blurring, random pixel intensity adjustment, zero padding, frame averaging, and contrast adjustment have been utilized. By efficiently using frame-level and video-level annotations, the architectures provided an accuracy of 90%. Another study investigated by Barros et al. (2021) developed a model that effectively classified LUS videos to identify pulmonary appearances of COVID-19 by combining CNN and LSTM networks. Rulers and other artifacts were eliminated from the videos during pre-processing. The hybrid model (CNN-LSTM) outperformed models that relied only on spatial approaches. They evaluated several hybrid models, overall Xception-LSTM performed the best, with an average accuracy of 93% and sensitivity of 97% for COVID-19. Li et al. (2023) detected weakly semi-supervised video classification for LUS with temporal context. Spatial augmentation, such as rotation, scaling, shearing, translation, center cropping, random horizontal flipping, and temporal augmentation, were used during training. CNN and LSTM were used to detect and classify video sequences simultaneously with minimal frame-level annotation burden, achieving an accuracy of 93.6%. Another study by Diaz-Escobar et al. (2021) employed LUS imagery to develop a deep-learning-based method for detecting COVID-19. Splitting images at a frame rate of 3 Hz, cropping to a quadratic window, and resizing to 224 × 224 pixels were used to preprocess LUS videos. They implemented and verified deep learning models. InceptionV3 classified COVID-19, pneumonia, and healthy classes with 89.1% accuracy and COVID-19 vs. non-COVID-19 with 91.5% accuracy. Their model POCOVID-net achieved 94.1% accuracy in COVID-19 and pneumonia classification.
An investigation by Magrelli et al. (2021) classified lung disease in children by using deep CNN and LUS images. Preprocessing includes RGB conversion, template-matching for artifact removal, cropping, resizing, and normalization. Data augmentation involves random Gaussian noise, pixel shifts, flips, rotations, regional zoom, and blurring. With 97.75% accuracy for healthy vs. bronchiolitis and 91.5% accuracy for healthy vs. bronchiolitis vs. bacterial pneumonia, the Inception-ResNet-v2 model performed the best. Roy et al. (2020) proposed deep learning for classifying and localizing COVID-19 markers in point-of-care LUS. Affine transformations, constant multiplication, Gaussian blurring, contrast distortion, horizontal flipping, and additive white Gaussian noise were all part of the augmentation method. A deep network based on Spatial Transformer Networks achieved a frame-based F1 score of 71.4% and a video-based F1 score of 61%. Furthermore, the segmentation model distinguishes areas in B-mode LUS images with a pixel-wise accuracy of 96% (Dastider et al., 2021) combined an autoencoder with the hybrid CNN-LSTM model to identify the COVID-19 severity score employing LUS. To extract the intended region, they remove the undesired parts during preprocessing. For the hospital-independent scenario, a frame-based 4-score disease severity prediction architecture achieved an accuracy of 79.2%, whereas, for the hospital-independent scenario, it reached 67.7% (Shandiz and Tóth, 2022) employed ConvLSTM and 3d-CNN to improve ultrasound tongue video processing. Down-sampling and normalization were part of preprocessing methods. This hybrid architecture obtained mean and MSE of 0.73 and 0.276, respectively. Studies from Dastider et al., and Shandiz et al. lacked a time-distributed CNN, which limited their ability to capture spatial–temporal processing. They also failed to compare their models with transformers or explore transfer learning. To address these gaps, we developed a time-distributed CNN and LSTM model that effectively captures spatial and temporal motions (Bhandari et al., 2022) employed deep learning and XAI to classify chest X-ray images into COVID-19, Pneumonia, and Tuberculosis. As part of the preprocessing, the images were resized, and data augmentation techniques, such as horizontal flipping, were applied. They proposed a lightweight CNN for lung disease detection, achieving an accuracy of 94.31 ± 1.01%. Though their work is novel, our approach differs in that we have focused on lung ultrasound videos and proposed a framework like DSS.
After conducting a comprehensive analysis of the available research, it was determined that most researchers relied on publicly available datasets. Only a small number of researchers obtained datasets directly from clinics. Some researchers employed preprocessing and augmentation techniques to enhance and expand their datasets. However, several crucial aspects were found to be lacking in most cases. These include frame augmentation, sequencing, denoising, hybrid model construction, spatial–temporal processing, comparison with transfer learning and transformers, and evaluations of diverse datasets. Additionally, only a limited number of studies made efforts to ensure the interpretability of their models.
Our study addresses these significant gaps in the current state-of-the-art research by introducing innovative graph-based frame sequencing methods. We also propose a time-distributed deep hybrid model and present a comprehensive approach for ensuring the interpretability of the model using a decision support system. In addition to explaining the model’s decision-making using heat maps, we performed an ablation study on the baseline model to identify the optimal configurations for our proposed model. It is worth noting that, out of the eleven reviewed literature sources, only one has conducted a similar analysis. To demonstrate the substantial differences in shapes and areas between classes, we segmented the ROI from the entire frame. We further extracted and analyzed numerous clinically essential features from this ROI. We used these features to develop a DSS approach based on ultrasound videos. Our approach includes an algorithm that generates a PDF graph depicting the range of a specific feature for a particular class to facilitate accurate disease classification prediction. Notably, no other studies have featured this particular DSS and this system. In addition to Explainable Artificial Intelligence (XAI), our research novelty lies in conducting an ablation study, analyzing and extracting clinically significant features, developing a DSS, and proposing an algorithm incorporating precise feature ranges for individual classes. For a thorough comparison between the state-of-the-art studies and our proposed study, please refer to Table 1.
3 Medical analogy
LUS has become increasingly popular for detecting lung diseases due to its inherent convenience, absence of ionizing radiation, and notable accuracy. LUS differs from other ultrasound examinations in that it is mainly artifact-based. Several artifacts or abnormal results in LUS can distinguish between normal and infected lungs. Below is an explanation of a few of the artifacts.
3.1 A line
A-lines are bright, slowly diminishing horizontal lines placed at equal intervals beneath the pleural line that indicates the repeated artifact of the parietal pleura (Bard, 2021). The gap of A-lines is nearly identical to the gap between the skin surface and the pleural line. Alines are most visible in the normal lung because they are caused by air gas beneath the pleura (Bard, 2021). Figure 1A demonstrates the A-line of the lungs.
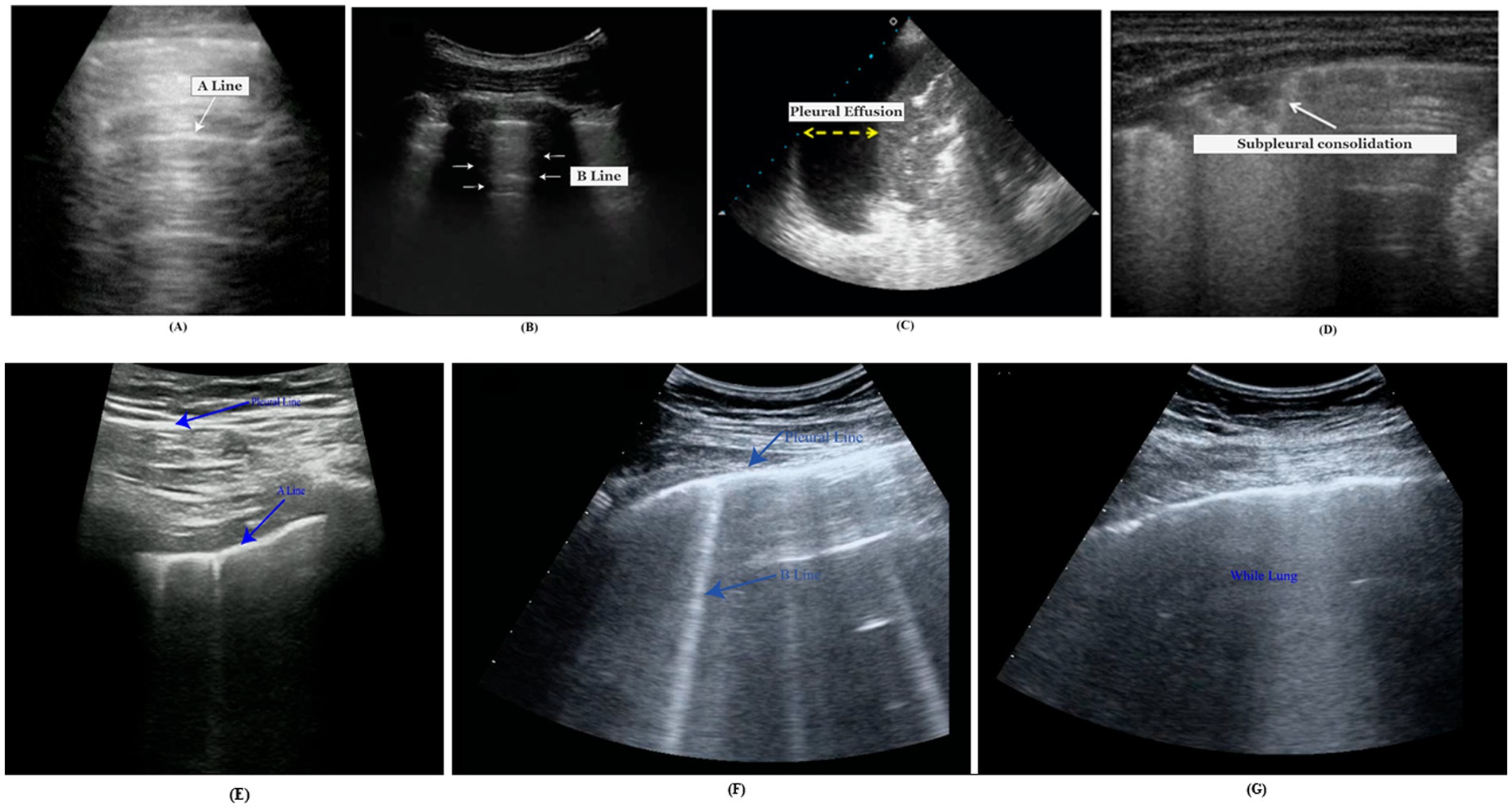
Figure 1. Medical markers, (A) A-lines, (B) B-lines, (C) pleural effusion, (D) subpleural consolidation, (E) normal LUS, (F) COVID-19 LUNG, and (G) white LUS for severe COVID-19 pneumonia.
3.2 B line
B-lines, called LUS comets, are caused by separate vertical reverberation artifacts starting at the pleural line, extending throughout the image without intensity reduction, and moving synchronously with lung sliding (Ostras et al., 2021). Their distinguishing feature is that they conceal A-lines. Normal lungs may have diffused B-lines (less than two in each intercostal space). They are considered severe if three or more B-lines are seen in a single image within two ribs. The quantity of B-lines is strongly related to disease severity.
In Figure 1B, the B-lines conceal the horizontal Alines visible in the neighboring intercostal space (represented by an asterisk).
3.3 Pleural effusion
A pleural effusion is a fluid in the pleural cavity that appears as a dark, hypoechoic, or anechoic area (de Groot et al., 2023). An ultrasound video clip captures the lateral motion between the lung and the chest cavity during respiration. An infrequent finding in COVID-19 is pleural effusion, which usually arises from a coexisting disease. Ultrasound is a highly dependable pleural effusion detection, measurement, and follow-up technique (de Groot et al., 2023). A pleural effusion is represented by the yellow arrow in Figure 1C.
3.4 Consolidations
When the air content in lung tissue falls below 10% of regular lung aeration, the pleural line is disrupted, and consolidative lesions occur (Soldati et al., 2020). Consolidations are more common in the lower posterior regions of patients with COVID-19 pneumonia, are often multiple, and may appear with or without air bronchograms. In Figure 1D, a B-line (dot) is illustrated with subpleural consolidation (arrow).
A normal LUS shows the pleural line as a continuous and consistent structure. Horizontal A-lines or fewer than two vertical B-lines are frequently observed. In ultrasound imaging, this pattern reflects the lung’s healthy state. Scattered B-lines are the most common US findings for diagnosing COVID-19. Pleural line irregularities and subpleural consolidations are the most likely findings, while pleural effusion is less frequent. In COVID-19 pneumonia, interstitial patterns, pleural abnormalities, and consolidations are the most typical LUS findings. These abnormalities typically have a bilateral, inconsistent distribution and clearly defined spared regions. The most common sign of severe COVID-19 pneumonia is a “white lung” that is entirely diffused with B-lines. Identifying COVID-19 pneumonia from other illnesses may be made easier using B-lines.
In clinical practice, LUS is being used more frequently to treat other diseases such as infection, asthma, pulmonary edema, pulmonary fibrosis, and pneumothorax. As in the case of interstitial lung disease/pulmonary fibrosis and acute respiratory distress syndrome (ARDS), the pleural line frequently appears thickened, irregular, or broken in affected areas. There will be an irregular hyperechoic line at the interface between the consolidated pathological lung and the aerated healthy lung. A focal subpleural hypoechoic region could be an indication of a minor infection. B-lines and pleural effusions define pulmonary edema. Irregular thickening of the hyperechoic pleural line is seen in asthma LUS. The lung point is where the pneumothorax and normal lung meet. Identifying a lung point on LUS allows for attaining 100% specificity in diagnosing pneumothorax. In the Figure 1 comparison, (E) represents normal LUS, (F) COVID-19 indicative lung, and (G) white lung for severe COVID-19 pneumonia. LUS findings in COVID-19 pneumonia are similar to those seen before the COVID-19 era. Common ultrasound symptoms, such as multiple B-lines, consolidations, and pleural line irregularities, emphasize the remarkable resemblance between the two diseases.
4 Methodology
This research has been completed in seven stages: (1) dataset preparation, (2) preprocessing and augmenting data, (3) sequencing frames, and (4) developing a 3D model that includes an ablation study, (5) analysis of performance, (6) explainability of the model which involves generating a heat map, segmenting the ROI, and constructing a PDF graph, and (7) propose decision support system. The workflow for this study is shown in Figure 2.
4.1 Dataset description
In this research, a publicly accessible dataset of LUS videos called COVIDx-US (Ebadi et al., 2022) is used. The dataset is an Open-Access Benchmark of COVID-19 Analytics Powered by AI for Ultrasound Imaging. With a standardized and consistent lung ultrasonography score for each video file, COVIDx-US is the first and biggest fully curated open-access benchmark dataset for lung ultrasonography imaging. This dataset contains four classes: pneumonia, COVID-19, normal, and others. The dataset is the merge format of nine different sub datasets. Therefore, our study works with a single merge dataset, COVIDx-US. The nine sub-datasets (Born et al., 2020; Etter et al., 2024; Nehary et al., 2023) were collected in different hospitals and organizations. Firstly, the Butterfly Network dataset, where the images were produced by Butterfly Network Inc. and are restricted for use only during the MIT GrandHack 2018. This large dataset contains ultrasound images from 31 individuals, all taken using the Butterfly IQ device. Next is the PocusAtlas dataset, part of a collaborative ultrasound education platform. Additionally, we have the GrepMed dataset, a publicly accessible resource for medical images and videos. Another dataset is Life in the Fast Lane (LITFL), an emergency and critical care educational resource repository. Following that is the Radiopaedia dataset, an open-access educational platform that includes a radiology encyclopedia and imaging archives. We also have the Papers dataset, a video collection that provides over 23,000 high-resolution frames from four ultrasound video sub-datasets. The core ultrasound dataset is a valuable medical imaging and research resource, especially for point-of-care ultrasound (POCUS). Additionally, there is the University of Florida (UF) dataset, where data has been collected from UF’s Department of Anesthesiology webpage. Lastly, Clarius produces portable ultrasound machines and scanners to collect raw data. Table 2 shows each of the datasets with their corresponding video numbers.
4.2 Image preprocessing
It is essential to provide models with appropriate input to classify video frames correctly. Image preprocessing is a necessary step to achieve the required accuracy before providing any input to the neural network (Raiaan et al., 2024). It involves multiple steps, such as removing artifacts and noise, finding the largest contour, and demonstrating important objects. In this study, we performed several sequential preprocessing techniques to improve the quality of the images. The videos contain irrelevant numerical numbers and logos, which distort the feature pattern. Hence, it becomes challenging to obtain satisfactory performance in classifying LUS videos without preprocessing techniques, as the neural network model used for classification tends to require clean and improved data.
Figure 3 shows the preprocessing technique steps. Initially, Otsu thresholding is used to create a binarized image of the original image. The application of binarized images helps to separate the foreground and background of the images, which contributes to simplifying the images and extracting the object region. To remove artifacts, we use morphological openings. We employ the largest contour detection technique on the image to obtain the largest contour mask. Finally, we used the Bitwise AND operation to get the output image, which we can then feed into the model to get the correct accuracy. A brief description of the mentioned preprocessing techniques is discussed in the subsequent section.
4.2.1 Morphological opening
After binarizing the images, morphological opening is applied to eliminate small objects from the images (Raiaan et al., 2023). Additionally, this technique helps to locate specific shapes in an image. The morphological opening operation consists of two individual operations such as erosion and dilation.
Here, Equations 1, 2 represent the erosion and dilation operation denoted by ee and dd , respectively. In these equations, “∗” and “∘” refer to the point-by-point minimum and maximum value, whereas I and I′ denote the actual and marker images. The combining operation of these techniques helps to remove the redundant artifacts from the lung frame.
4.2.2 Largest contour detection
The morphological opening removes most of the small objects from the frames successfully. However, there are still some thin, tiny artifacts at the border of the frames (Khan et al., 2023). After removing the artifacts from the mask image, several contours are explored, as seen in step 4. The find_contour function is utilized to find these individual contours. This function iterates over the image and returns the list of contours where each contour is the coordinates of the boundary points of each distinct object. We employ the largest contour detection technique, where the contour area is calculated, and a max function compares the area of all contours. This function returns the largest contour based on the maximum contour area.
Equation 3 defines the area of a contour, denoted by CiCi , where A(Ci)A(Ci) represents the area of contour CiCi . The area is calculated as the sum of all pixels pp that belong to the contour. In the Equation 4, CmaxCmax is the contour that has the largest area among the n contours. The argarg maxmax function returns the index i corresponding to the contour with the maximum area A(Ci)A(Ci) . In other words, CmaxCmax is the contour for which A(Ci)A(Ci) is maximized, making it the largest contour based on its area. Largest contour detection is the appropriate step that detects the most prominent objects and eliminates the irrelevant artifacts. Additionally, it obtains the precise ROI from the frames and focuses only on the significant features. Figure 3 shows the complete process.
4.3 Frame augmentation
Frame augmentation is an effective technique to artificially expand the size, diversity, and quality of a video dataset. A frame is a single image from a video that is considered a unit, and these frames are organized sequentially to create the video. Our study extracts the frames from ultrasound videos at a specific frame rate. These frames’ spatial and geometric transformations are then performed by flipping and rotating techniques.
4.3.1 Flipping
Flipping techniques reverse the image regarding the vertical or horizontal axis of the frame, whereas rotational augmentation transforms the frame pixel at a specific angle value. In this study, we performed horizontal and vertical flips.
Equations 5, 6 are employed for horizontal and vertical flipping. The output frame is denoted by ‘I,’ x, and y is the pixel coordinates, and n,d, the width and height of the corresponding frame, are indicated by w and h, respectively.
4.3.2 Rotation
Rotation is a common frame augmentation technique that involves rotating an image by a certain angle, enhancing the diversity of the training dataset for improved model generalization. Equation 5 is employed to rotate a 2D frame in θ angle and create the rotational frame of the original frame.
In Equation 7, the variables, fx and fy denote the updated positions of individual pixels following the rotation operation, with x and y representing pixels from the original image. The roles of cosine ( cosθ ) and sine ( sinθ)in the equation are to ascertain the angular adjustments involved in the rotation.
These techniques significantly increase the frame number for a particular video, create more diversity, and improve the generalizability of the model for extracting key features. However, frame augmentation can distort the temporal consistency of a video sequence. In some cases, it leads to the potential frame dropping or shuffling, which can affect the flow of the video and cause information loss. Consequently, it reduces the performance of the model. To address these challenges, we applied a novel frame sequencing strategy.
4.4 Frame sequencing
Frame sequencing is an effective solution to mitigate the discontinuity of frames by capturing temporal dependencies and identifying patterns within frames (Chen et al., 2023). This research performs frame sequencing steps before reconstructing the video using augmented frames. Various techniques, such as clustering, wrapping, and deep learning, have been employed to find the optimal strategy. In this study, we propose a novel and efficient graph-based technique for finding the optimal frame sequence. After applying augmentation techniques, all the frames from a video are stored in a list. To evaluate the quality of each frame, two metrics are calculated consecutively, MSE and SSIM. The MSE between two consecutive frames Fi and Fj is calculated using Equation 8.
where k represents each pixel in the frames, and N is the total number of pixels. A lower MSE value denotes a higher-quality frame. The SSIM between two consecutive frames Fi and Fj is given in the Equation 9.
Here, μi and μj are the mean intensities of frames Fi and Fj , and σ2i , σ2j , and σij represent the variance and covariance. A higher SSIM value indicates greater consistency between frames. Each frame Fi is represented as a node in a graph, and the edge between two consecutive frames Fi and Fj is assigned a weight wij , which depends on both the MSE and SSIM values in the Equation 10.
Here, α and β are scaling factors used to balance the MSE and SSIM contributions to the weight.
The frame sequencing graph is constructed using the frames as nodes and the edge weights based on wij . To determine the optimal sequence, we utilize the MST approach, which ensures that the frames are sequenced with minimal disruption. The MST is calculated using Equation 11.
Here, EMST is the set of edges in the MST. The MST ensures that the frame sequence is coherent by minimizing the edge weights, which represent frame quality based on MSE and SSIM.
To further refine the sequence, a filtering technique is applied. Frames that meet the following threshold conditions are selected, MSE(Fi,Fj)〈TMSEandSSIM(Fi,Fj)〉TSSIM . In this threshold, TMSE and TSSIM are predefined thresholds for MSE and SSIM, respectively. This filtering step eliminates low-quality frames and selects those that ensure a smooth transition between consecutive frames. The final optimal sequence Sopt is composed of the frames that satisfy these conditions and are part of the MST, Sopt={F1,F2,…,Fm}where(Fi,Fi+1)∈EMSTand MSE(Fi,Fi+1) 〈TMSE,SSIM(Fi,Fi+1)〉TSSIM. This final sequence Sopt is then used for video restoration, as well as for subsequent classification and analysis tasks. Through this graph-based approach, we ensure that the frame sequence is optimized, reducing disruptions and enhancing the overall video quality. Figure 4 shows several graphs for different class videos.
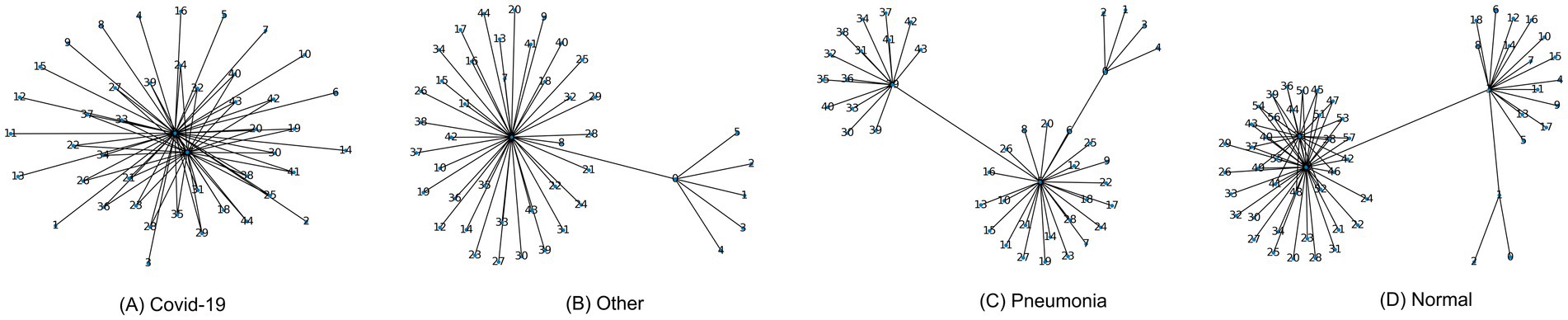
Figure 4. Abstract frame sequence graph for (A) covid-19 class, (B) other class, (C) pneumonia class, (D) normal class.
4.5 3D model reconstruction
For the classification of videos of lung disease, we construct a 3D model. Reconstruction has several steps, which are detailed in this section.
4.5.1 Baseline model
In deep learning, the baseline model denotes an initial model used as a reference point for evaluating performance over time. It is crucial as it facilitates the benchmark comparison and highlights the improvement that validates further optimization techniques for the model. In this study, we performed extensive experiments and trials on the baseline model to obtain the best-optimized 3D model. A hybrid model combining CNN and LSTM for the classification of LUS video frames has been constructed as a baseline model. The model receives input as sequential data and processes it to predict the lung label. Initially, we utilized three convolutional layers with subsequent pooling layers to extract the local features. Average pooling was preferable at the beginning to down sample the features. Besides, the avg-pooling technique prevents the overfitting from the model initially. The 3×3 kernel size is traversed in the input frames for extracting and filtering the features and creating the corresponding feature map. The kernel numbers for the three convolutional layers are 16, 32, and 64, respectively. After performing the filtering operation, the ReLU activation function activates the complex patterns. The Categorical Cross entropy loss function is used for the baseline model as it is a multi-class classification task. The proposed baseline model utilized a 0.0001 learning rate throughout the experiments. The reason behind the low learning rate is to ensure slow convergence in the model and not be stuck in the local solutions. The LSTM architecture handled the temporal frequency of the frame sequence. We have utilized a total of 128 LSTM units to capture the longer sequence pattern from the LUS frames.
4.5.2 Ablation study
An ablation is conducted on the baseline model to determine the optimal configurations of the proposed 3D model. We have run 11 experiments to fine-tune its many hyperparameters and attain the best accuracy and computational cost performance. The ablation study is completed in 11 steps, with the conv and pooling Layer, filter size, number of filters, pooling layer, activation function, batch size, loss function, optimizer, learning rate, dilation rate, and LSTM units all being altered. The best test accuracy is 92.02% % in the first step of altering the conv and pooling layers, where the number of conv and pooling layers is 5 and 3, respectively. The second step is to modify the filer sizer, with the best test accuracy of 93.41 and a filter size 2×2. Then, in the following step, change the number of filters. In that step, we achieved 95.28% accuracy. The fourth step is to change the type of pooling layer to average pooling, which has a near-perfect accuracy of 94.83%. The test accuracy we obtained when altering the activation function is 96.09% when the activation function is tanh. When adjusting for batch size, the accuracy is 96.09%, and the batch size is 16. The same accuracy was obtained by changing the optimizer. With a learning rate of 0.001, our accuracy is at its highest, 96.23%. The step known as the dilation rate, where the best accuracy is 96.39%, comes before the final one. Finally, the best test accuracy is achieved at 96.57% when the LSTM unit is 256 after altering the LSTM units. The ablation studies of our proposed hybrid model are shown in Table 3.
4.5.3 Proposed model (TD-CNNLSTM-LungNet)
This section elaborately discusses our proposed 3D model (TD-CNNLSTM-LungNet) after a comprehensive ablation study.
4.5.3.1 Backbone of time distributed CNN and LSTM
In our study, we combine LSTM and Time-Distributed CNN in our proposed model. CNN and LSTM are combined to build the architecture and covered with a Time Distributed layer (Montaha et al., 2022). Within CNN, the most important layers are the convolutional layer and the activation layer (). The derivation of the convolution operation is given in Equation 12.
Where X denotes the input data, Wk denotes the kth convolution kernel, b represents the offset and ‘*’ symbolizes the convolution operator. In a convolutional operation, the stride and padding methods work together to determine the size of the kth feature matrix Zk . The nonlinear activation is denoted by f. The 3D ConvNets use 3D convolution and 3D pooling operations (Arif et al., 2019). Three-dimensional convolution is an extension of two-dimensional convolution. The 2D convolution produces two-dimensional feature maps, whereas the 3D convolution produces a volume with multiple dimensions. When compared to standard RNNs or other variants, LSTM has been demonstrated to be the most reliable and effective model for learning lengthy temporal relationships in practical applications (Qiao et al., 2018). Time Distributed function is used to configure the input shape before moving on to convolution and pooling layers. A Time Distributed layer generally adds dimension to the corresponding argument layer’s input shape. As a result, CNN can receive multiple frames as a single input. When applied to an input tensor, the distributed layer acts as a layer wrapper that holds the CNN model itself. This wrapper allows to addition of a layer to each sequential slice of input data, where the inputs can be in 3D. The input dimensions in our experiment are (height, width, frame, and channel). LUS videos have provided the frame of input.
Three gate structures are used by the LSTM to regulate the memory cell ct . The cell state can have information added or removed by the three gates (Arif et al., 2019). The three gates, input gate it , forget gate ft , and output gate ot , can be considered as a means to allow information to pass through on an optional basis. From Equation 13–18 illustrate the information passing and updating process in LSTM.
Here the Hadamard product is indicated by ∘ . Every time step t, the hidden state ht−1 , memory cell state ct−1 , and current input xt can be used to update the hidden state ht and memory cell state ct respectively. Upon receiving a new input, ft can determine the number of data in ct−1 that should be overlooked. After that it , and ∼ct will determine what fresh data can be kept in the cell state. Updating the old cell state ct−1 into the new cell state ct is the next step. Ultimately, the output ht is determined by xt , ht−1 , and ct . The LSTM’s input, cell state, and output are all one-dimensional vectors.
Time-distributed CNN is extremely useful in a variety of fields, including magnetic resonance imaging (MRI), action recognition, health monitoring, speech-emotion recognition, and so on. In our case, we classified LUS videos to determine the disease.
4.5.3.2 Model description
Our proposed model combines LSTM and time-distributed CNN. Eleven layers comprise this hybrid model: five convolutional layers, three max pool layers, one LSTM layer, one flattened layer, and one dense layer. A frame taken from LUS videos is the input. The input image has dimensions of (224,224,3,1) for height, width, channel, and frame, respectively. The input image is fed into a convolution layer with a dilation rate of (1,1) and corresponding height, width, channel, and frame values of 224,224,3,16. There are 16 filters of size 5×5 while padding remains “same.” A max pool layer with the height, width, channel, and frame values of (224,112,2,16) follows this convolution layer. The pool size and strides are 2×2. Here, padding remains the “same” as well. Two convolution layers in a row are different in frame size but have the same height, width, and channels 224, 112, and 2. The frame sizes of Conv2 and Conv3 are 32 and 64, subsequently. The dilation rates of these two layers are (2,2) and (3,3), respectively. There are 32, and 64 filters of size 5×5 for Conv2, and Conv3, respectively. A second max pool layer, with height, width, channel, and frame size of 224, 56, 1, and 64, consequently, comes after those convolution layers. The pool size, stride, and padding is as same as the previous pooling layer. Two convolution layers are present again with various frame sizes. The frame sizes of Conv4 and Conv5 are 32 and 64, respectively. These two convolution layers have height, width, and channel measurements of 224,56,1 and dilation rates of (4,4) and (5,5), respectively. The filter number and size remain same like previous two convolutional layers.
Then, it is passed to the third max pool layer, where 224,28,1,64 represents the height, weight, channel, and frame. The pool size, stride, and padding is same as the previous two pooling layers.
In every convolution and pooling layer, the activation function is ReLU. The input is sent to a flattened layer with 1792 neurons and 224 heights. All of the layers have the same height up until the flattened layer. The width of the input image is reduced by half after each max pool layer. The flattened layer is followed by the LSTM layer, and the dropout is 0.5, which produces 256 outputs. It is then passed to the dense layer, where the activation function is softmax. The categorical cross-entropy loss function is used to construct the model. Training is done with the Adam optimizer.
The output is a disease, which can be COVID-19, pneumonia, normal, or other.
Figure 5 represents the framework of our proposed model. Using frames taken from LUS videos, we categorize the videos according to the type of disease that has occurred including pneumonia, COVID-19, other, or normal. Our proposed model is a hybrid model that combines LSTM and TD CNN. This minimizes the computational cost and produces a satisfactory result that has correctly classified the frames while preserving their temporal and spatial dependencies.
5 Experimental results
5.1 Performance analysis
Performance metrics, such as train accuracy, validation accuracy, test accuracy, precision, recall, specificity, and F1 score (%), are used in this section to assess the models’ performance. Table 4 shows that, with the optimal configuration, the model we proposed, TD-CNNLSTM-LungNet, was evaluated using the COVIDx-US dataset, a comprehensive merged format of nine individual video datasets. The model demonstrated exceptional performance, achieving a training accuracy of 96.16%, validation accuracy of 96.26%, and test accuracy of 96.57%. Additionally, the model exhibited strong precision (95.56%), recall (96.51%), specificity (96.24%), and F1 score (96.02%).
The accuracy curve, loss curve, and confusion matrix are shown in Figure 6. The accuracy curve between training and validation begins low initially, as seen in Figure 6A, but increases with epochs. There is significantly less distance between the lines, representing training and validation accuracy. Since there is a close margin between these two lines, indicating that neither overfitting nor underfitting occurs. For each epoch, two curves fall into the same range. Similarly, Figure 6B demonstrates the loss curve where the loss is large in the first epoch but eventually diminishes. The optimized model’s confusion matrix is shown in Figure 6C. The diagonal value indicates the true positive value in the data set, while the column and row represent the actual and predicted data, respectively. It is neutral toward any classes and can accurately classify all four diseases.
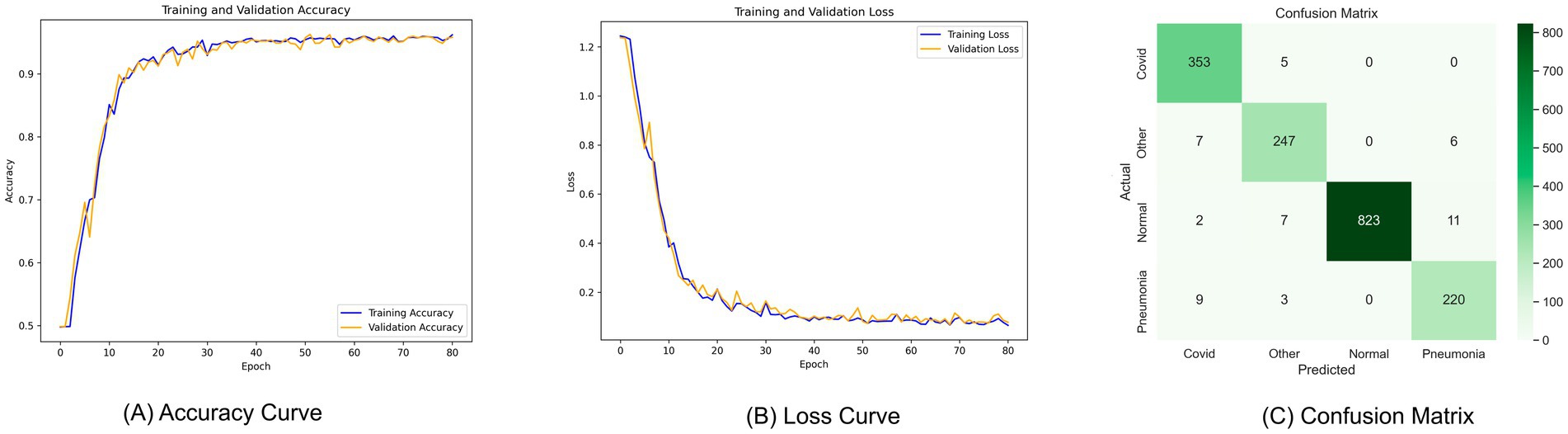
Figure 6. Curve analysis of the proposed model (A) accuracy curve, (B) loss curve, (C) confusion matrix.
To ensure the robustness of our results, we conducted tests on each of the nine individual datasets. Although the accuracy was slightly lower on these sub-datasets, likely due to the smaller number of videos, the model still performed admirably. For example, the ButterflyNetwork dataset achieved a training accuracy of 87.32%, validation accuracy of 88.42%, and test accuracy of 87.97%, with an F1 score of 86.02%. Similarly, on the PocusAtlas dataset, the model recorded a training accuracy of 85.17%, validation accuracy of 86.81%, and test accuracy of 85.82%, with an F1 score of 83.94%. The lowest performance was observed on the GrepMed dataset, where the model still managed a training accuracy of 81.7%, validation accuracy of 83.72%, and test accuracy of 81.91%, with an F1 score of 79.9%. These results highlight the effectiveness and robustness of the TD-CNNLSTM-LungNet model across diverse datasets, with slightly lower but still competitive performance in scenarios with fewer training samples.
5.2 K-fold cross validation
After curve analysis, we applied K-fold cross-validation in our proposed model to ensure robustness. The K-fold cross-validation method divides the sample into K groups, each treated as a testing data set to assess the model. Then, model weights are selected by minimizing the total squared prediction errors acquired from every group. K-Fold cross-validation is performed with 3-fold, 5-fold, 7-fold, 9-fold, and 11-fold values, achieving testing accuracy of 96.41, 96.33, 96.55, 96.59, and 96.47%, respectively. The highest accuracy is found at 9-fold, which was 96.59%. The accuracies at each fold are all close to one another. There are no statistically significant differences between the accuracies of each fold. As a result, our model will achieve the same level of test accuracy in other training scenarios using the same dataset.
5.3 Comparison with transfer learning models
TD-CNNLSTM-LungNet has been compared with ten transfer learning models (Khan et al., 2023; Raiaan et al., 2024) to validate the performance with respect to other models. The proposed model, TD-CNNLSTM-LungNet, achieves an impressive accuracy of 96.57%, significantly outperforming several well-established transfer learning models in computer vision. Among the compared models, the closest competitor is ResNet101V2, which achieves an accuracy of 92.03%. While this is a strong performance, it falls short by a notable margin of 4.54% compared to our model. DenseNet121 and InceptionResNetV2 also demonstrate robust results with 91.54 and 91.23% accuracy, respectively. However, they lag by approximately 5%. Other models such as VGG19, InceptionV3, and ResNet50V2 offer competitive accuracies of 90.74, 89.65, and 88.45%, respectively. Nonetheless, they are outperformed by a considerable 5–8% margin. The relatively lower performances of ResNet50 and ResNet101, at 81.32 and 83.71%, respectively, emphasize our proposed model’s substantial leap in accuracy. The exceptional performance of TD-CNNLSTM-LungNet highlights its robustness and superior capability in handling complex video classification tasks, demonstrating its potential as a highly reliable model in this domain.
5.4 Comparison with transformers
Transformer architectures have significant advantages in processing sequencing data like time sequences, video frame sequences (Khan et al., 2023; Raiaan et al., 2024). It has its own attention mechanism and parallelism, facilitating feature extraction and achieving an optimal outcome. In addition to comparing TL models, this study also compares the performance with widely applied transformer variants, including vision transformer (ViT), swine transformer (ST), and compact convolutional transformer (CCT). It is stated previously that utilizing distributed convolutional layers substantially reduces the parameter numbers. This comparison aims to highlight the proposed TD-CNN-LSTM-LungNet model, which can attain the desired performance by requiring less time. The Vit, ST, and CCT achieved comparatively higher accuracy than the TL models. They obtain 93.78, 92.44, and 94.27%, respectively. However, the proposed model marginally outperforms by obtaining 96.57% accuracy. Moreover, it requires 205s–2024s times on average per epoch, whereas the ViT and ST execute per epoch for a longer time, taking an average of 927 s and 805 s, respectively. CCT requires a comparatively lower operation time of 439 s. This assessment upholds the benefits of the proposed model for efficient LUS video classification.
6 Explainability of proposed model (TD-CNN-LSTM-LungNet)
In this section, the explainability of the (TD-CNN-LSTM-LungNet) is included to provide insight into the model’s decision-making. Explainable AI is an emerging and advanced field integrated into several domains to explain the model predictions and visualize the area that needs to be focused. Especially in medical domains, it ensures clinical transparency and acceptance of the diagnosis tool. To increase the reliability of the deep learning model, this content is integrated. This study incorporates LayerCAM, provides a class activation map for both shallow and final layers hierarchically, and offers a better visual interpretation of the proposed model’s prediction (Jiang et al., 2021). Gradient-weighted Class Activation Mapping (GradCAM) or its updated version often struggles to capture the shallow convolution layer’s heatmap output, heavily relying on the final layer. LayerCAM addresses this limitation, providing a detailed extraction of the feature map for the shallower layer also. Therefore, this explainability technique is more effective for the multi-layered model. To enhance the output of the heatmap, we fuse the class activation map of layers 2, 3, and 4. The dimension of the class activation map is resized for each of these layers, and the fusion operation aggregates the positive weighted gradients. This fusion substantially increases the measurement of the actual ROI area, indicating the crucial area that contributes to the determination of the influential class label. Figure 7 shows the heatmaps of multiple frames of the corresponding classes. The dynamic changes in ROI across the frames are clearly displayed in the figure.
These regions can be utilized for further analysis, and the features of these shapes can be leveraged into a DSS that can profoundly validate the proposed approach for diagnosing lung diseases from the ultrasound videos. To introduce a DSS, a systematic approach needs to be followed. All of the processes are comprehensively defined in the subsequent section.
6.1 Segment the ROI
The primary step is to segment the ROI from the whole frame. Figure 8 demonstrates the distinct variations in shapes and areas among different classes, and the intensity values of the pixel can also vary. As a result, it will create significant variance among the classes and effectively distinguish them from one another. Considering the entire frame will include unwanted ambiguity to the feature value. Several image processing techniques are employed to segment the ROI efficiently.
Figure 8 (I) illustrates the sequential process of ROI segmentation, including all five steps. Initially, the original image and its corresponding heatmap are acquired. Then, the binarization is performed on a heatmap where the pixel value is converted to black and white. Otsu thresholding is utilized for this step. A mask is subsequently generated using this binarized image. Finally, the bitwise AND operation is performed on the mask and original image to segment the ROI. Additionally, Figure 8 (II) displays the associated geometric and pixel-based features calculation of the segmented ROI area. In general, these features indicate the underlying characteristics of the ROI area and utilizing these features distinguish the class label effectively. The following section facilitates feature extraction using mathematical expressions.
6.2 Feature extraction
Our heat map generation is based on how interpretable the model is. Considering that each class’s hand-crafted features are created utilizing that ROI. These features are significantly essential to represent the fundamental characteristics of the ROI area, and using them to distinguish the class label is beneficial. These features are described as follows:
6.2.1 Sphericity
Sphericity measures how much an object’s shape resembles a perfect sphere. It is calculated using the following equation where Ψ is the sphericity, Vp is the object’s volume, and Ap is the surface’s area.
It clarifies from the Equation 19 that, sphericity is the ratio of an object’s volume and the surface’s area of a sphere. Figure 8 (B) shows the sphericity from the lung’s ultrasound frame.
6.2.2 Eccentricity
Eccentricity is a measure of how far a conic section deviates from a circular shape. To be specific, a circle has 0 eccentricity, and an ellipse that is not a circle has an eccentricity that ranges from 0 to 1. Figure 8 (D) depicts the eccentricity and it can be measured using Equation 20.
Here, e refers to the eccentricity, c refers to the distance from the center to the focus and a refers to the distance from the center to the vertex.
6.2.3 Perimeter
A closed path that covers, encompasses, or shapes a one-dimensional length or a shape with 2 dimensions is called a perimeter. In a circle, the perimeter is calculated using Equation 21.
Here p is the perimeter and r is the radius of the circle. Figure 8 (C) illustrates the perimeter of the lung’s ultrasound frame.
6.2.4 Standard deviation
The standard deviation indicates how measurements within a group depart from the mean expected value or average. Most of the data are close to the mean when the standard deviation is low, and the data varies more widely when the standard deviation is high.
6.2.5 Equivalent diameter
The diameter of a sphere with the same projected area as the projection of the particle is known as the area-equivalent diameter, also known as the circular-equivalent diameter. This translates into a pixel-by-pixel measurement of the projection area, made possible by the development of digital image analysis, and the equivalent diameter depicted in the Figure 8 (G).
6.2.6 Region entropy
In image processing, entropy is a measurement of an image’s content of information. An image with a large range of pixel values and a high entropy number is said to be complex, while an image with a low entropy value is simpler and more consistent. Entropy can be used to determine the most informative areas of an image for further processing or analysis, as well as to evaluate the quality or complexity of the image.
6.2.7 Extent
The area of an image object divided by the area of its bounding rectangle is the definition of its extent. The extent of lung ultrasound is illustrated in Figure 8 (A).
6.2.8 Elliptical ratio
The elliptical ratio is the ratio of the major axis to the minor axis. Figure 8 (E) shows the elliptical ratio and Equation 22 describes it.
Here, an ellipse’s major axis is its longest diameter, and its minor axis is its shortest diameter.
6.2.9 Width-height ratio
An image’s width-to-height ratio, sometimes referred to as its aspect ratio, is the ratio of its width to its height, depicted in Equation 23 and Figure 8 (F) shows it.
7 Decision support system
A DSS method mitigates data scarcity, computational resource constraints, and time complexity and ensures explainability; the main goal of this study was to create a DS system with features based on frames extracted from ultrasound videos. The disease class can be predicted using the proposed DS system. Identifying the frames that led to accurate categorization was critical in the classification tasks. We used LayerCAM to identify the important region and extract the geometrical features of that ROI. These features, detailed in Section 6.2, were generated from each frame of every video. We developed our DSS using these extracted feature values. Each feature produces a value for every video frame, which helps reveal distinct geometrical patterns. Each disease has a specific threshold value associated with a single feature that is clear from the PDF graphs. The probability function that shows the density of a continuous random variable falling inside a given range of values is the PDF graph. The PDF graphs provide the continuous random variable’s probable values (Azam et al., 2024). The distribution of data points along a variable’s range is graphically shown in PDF plots. In total, we extracted nine features. If at least seven out of the nine features for a frame fall within the defined range for a particular class, we classify that frame as belonging to that class. This also allows us to understand the underlying connections and patterns in the data, allowing for more accurate decision-making and predictive modeling. Figure 9 depicts the clear range visible from the PDF graphs. The range has been verified by three experienced medical professionals, along with the whole DSS process and explainability.
The relationships between the nine distinct features for the classification task were evaluated in this study using PDF graphs. Every feature was a distinct quality that influenced the classification outcome. One can quickly overview the feature distributions by generating PDFs for each feature. We can spot patterns in the graph in this way. Figure 9 displays the PDF graph for each feature.
In Figure 9, the orange graph represents the feature values for the normal class, whereas the green graph represents the feature values for the other class, the blue graph represents the COVID-19 class, and the red graph represents the pneumonia class. To determine whether a disease is normal, other, COVID-19, or pneumonia, a DSS was developed using the PDF graphs shown in Figure 9. The DSS analyzed the extracted features. After rapidly analyzing the plots, a class was identified by determining the threshold values for each feature.
Algorithm 1 and Figure 9 displays the threshold value for each feature in each class. The sphericity graph displays four different types of ranges of values for each of the four distinct classes. While the pneumonia class ranges from 0.136 to 1.507, the COVID-19 class has the second-highest density value, ranging from 0.04 to 0.13. The other class has the widest range, ranging from 0.15 to 0.23. The normal class has the highest density and the shortest range, ranging from 0.235 to 0.258. With a range of 0.022 to 0.213, the normal class has the highest density in the case of the eccentricity graph. The COVID-19 class is characterized by the lowest density and the highest range, which falls between 0.2 and 0.4. The density of the pneumonia class and the other class is the same, falling between 0.815 and 1.130 and 0.397 and 0.968, respectively. There are two groups of density if the perimeter graph is analyzed. The normal and the other classes, which have the highest and same density, are both contained in one group. The COVID-19 and pneumonia classes with the lowest and same density are found in the other group. The ranges for pneumonia, COVID-19, normal, and other illnesses are 9,680 to 13,540, 5,070 to 9,830, 81 to 2073, and 2000 to 5,312. According to the PDF graph, the normal class has the second-highest density and a range of 80 to 94.78. Although the other class’s range is the largest, spanning from 90 to 122.38, its density level is extremely low. Conversely, the COVID-19 class has the highest density, although it only varies from 119.85 to 130.05 over a very narrow range. With ranges of 128.44 to 141.55, the pneumonia class density is nearly identical to the normal class density. In that order, the three classes, normal, pneumonia, and COVID-19, have nearly identical densities in the equivalent diameter graph, with ranges of 17 to 118.57, 493.63 to 600.01, and 388.24 to 498.06. When it comes to density, the other class has fallen behind, with the largest range occurring between 117.57 and 391.433. Two classes with the same density in the region entropy graph are pneumonia and the other class, which have ranges of 0.862 to 1.014 and 0.495 to 0.728, respectively. The traits of the COVID-19 class have the widest range, ranging from 0.682 to 0.875. The normal class has the highest density and ranges from 0.395 to 0.499. With a narrow range between 5,000 and 6,435, COVID-19has the highest density in the extent graph. The range from 6,388 to 8,415 is also very restricted for the other class. Compared to the prior two classes, the pneumonia class has a slightly wider range, ranging from 170 to 5,040. The normal class, which encompasses from 8,165 to 17,048, is the largest ranged class. The density of the other COVID-19 and pneumonia classes has nearly equal ranges of 0.207 to 0.568, 0.6 to 0.862, and 0.833 to 1.892, respectively, according to the elliptical ratio graph. The density of the normal class is highest, but its range is narrowest, ranging from 0.568 to 0.618. The other and the COVID-19 class have the same and highest density, with ranges between 0.977 to 1.492 and 1.306 to 1.814, respectively, if we look at the width-height ratio graph. The normal class extends from 0.5 to 1.115. Pneumonia falls most widely within the range of 1.762 to 2.735.
Algorithm 1 depicts the pseudocode to understand the DSS deeply and clearly. The pseudocode has three stages, which are shown in Algorithm 1.
ALGORITHM 1 : Decision Support System
Stage 1: CSV = Read the CSV for normal, other, COVID-19, or pneumonia class; Stage 2: Call Check_NormalValues function or Call Check_OtherValues function; or Call Check_ COVID-19Values function; or Call Check_PneumoniaValues function; Stage 3: Call the Caluculate_Accuracy function and pass the count value; #Functions Check_NormalValues(){ Initialize count = 0 if 0.235 <= 'sphericity' <= 0.258: increase count value; if 0.022 <= 'eccentricity'<= 0.213: increase count value; if 81 <= 'perimeter' <= 2073: increase count value; if 80 <= 'standard_deviation' <= 94.78: increase count value; if 17 <= 'equivalent_diameter<= 118.57: increase count value; if 0.395 <= 'region_entropy' <= 0.499: increase count value; if 8165 <= 'extent' <= 17048: increase count value; if 0.568 <= 'elliptical_ratio' <= 0.618: increase count value; if 0.5 <= 'width_height_ratio' <= 1.115: increase count value; return count } Check_OtherValues(){ Initialize count = 0 if 0.15 <= 'sphericity' <= 0.23: increase count value; if 0.2 <= 'eccentricity'<= 0.4: increase count value; if 2000 <= 'perimeter' <= 5312: increase count value; if 90 <= 'standard_deviation' <= 122.38: increase count value; if 117.57 <= 'equivalent_diameter<= 391.433: increase count value; if 0.495 <= 'region_entropy' <= 0.728: increase count value; if 6388 <= 'extent' <= 8415: increase count value; if 0.207 <= 'elliptical_ratio' <= 0.586: increase count value; if 0.977<= 'width_height_ratio' <= 1.492: increase count value; return count } Check_ COVID-19Values(){ Initialize count = 0 if 0.04 <= 'sphericity' <= 0.13: increase count value; if 0.397 <= 'eccentricity'<= 0.968: increase count value; if 5070 <= 'perimeter' <= 9830: increase count value; if 119.85 <= 'standard_deviation' <= 130.05: increase count value; if 388.24 <= 'equivalent_diameter<= 498.06: increase count value; if 0.682 <= 'region_entropy' <= 0.875: increase count value; if 5000 <= 'extent' <= 6435: increase count value; if 0.6 <= 'elliptical_ratio' <= 0.862: increase count value; if 1.306 <= 'width_height_ratio' <= 1.814: increase count value; return count } Check_PneumoniaValues(){ Initialize count = 0 if 0.136 <= 'sphericity' <= 1.507: increase count value; if 0.815 <= 'eccentricity'<= 1.130: increase count value; if 9680 <= 'perimeter' <= 13540: increase count value; if 128.44 <= 'standard_deviation' <= 141.55: increase count value; if 493.63 <= 'equivalent_diameter<= 600.01: increase count value; if 0.862 <= 'region_entropy' <= 1.014: increase count value; if 170 <= 'extent' <= 5040: increase count value; if 0.833 <= 'elliptical_ratio' <= 1.892: increase count value; if 1.762 <= 'width_height_ratio' <= 2.735: increase count value; return count } Calculate_Accuracy(){ #Check the value for each row for row in CSV: if count < 7: Not Passed -> Save the row as non passed value; else: Passed = Passed + 1; TotalRows = Passed + len(not_passed) PassPercentage(Accuracy) = (passed / TotalRows) * 100 }
The DSS first reads the normal, other, COVID-19, or pneumonia class data. Since the DSS calls the functions Check_NormalValues, Check_OtherValues, Check_COVID-19Values, or Check_PneumoniaValues in this second stage, the second stage is critical. This function counts the number of normal values in a row by taking the row of the CSV file as input. As shown in Algorithm 1, the functions compare each value in the row to a set of predetermined thresholds. The function increases the count if a value falls inside the threshold. The function does nothing with the count variable and moves on to the next value if a value is outside of the threshold. When we get the value from stage 2 of the Algorithm 1, we call the Calculate_Accuracy function. This function determines the model’s accuracy by counting the number of rows greater than or equal to 7. The accuracy is then determined by dividing the total number of rows in the CSV file by the number of rows with counts greater than or equal to 7. If an image meets the seven-feature threshold, it is considered predicted true. Our DSS performed so elegantly, and only two videos of COVID-19 and three from pneumonia could not meet the ranges in the DSS; other than that, all other videos meet the threshold. It implies the robustness of the DSS.
7.1 Validation of DSS
Table 5 includes the validation result for the proposed DSS. We randomly selected 20 videos and applied the algorithm to evaluate the final class label.
The class distribution of the tables is 5 samples from the COVID-19 and Normal classes and 4 samples for both pneumonia and other lung diseases. According to Algorithm 1, if at least 7 features obtain the same class labels, then that class label will be assigned for the corresponding sample. The overall assessment highlights that the DSS successfully predicts the actual class label. In every case, the samples meet the threshold range and confirm the true class label. This comprehensive analysis indicates the robustness of the proposed DSS, providing a reliable diagnostic method using the clinical marker and assessing the efficacy of this study in lung abnormality diagnosis.
7.2 Medical validation
We have generated a heatmap for each video in every class and extracted features from ultrasound video frames feature maps to develop a DSS approach. If seven or more of the nine feature values fall into the range of that specific class, we classify the frame as that particular class. We have validated our model’s heatmaps to ensure explainability with medical professionals. Information on four classes of diseases was collected from multiple medical experts and accumulated in Table 6. It perfectly aligns with our model’s findings, which shows our model’s competence in effectively classifying the disease into four classes: COVID-19, pneumonia, normal, and others.
8 Discussion
This study presented a complete framework for performing multiclass classification on LUS videos. The aim of this study is to develop a robust model (TD-CNN-LSTM-LungNet) that can accurately learn the temporal consistency from the videos and predict the actual class label. All the experiments were successfully executed and emphasize the effectiveness of this study contributing to the lung disease diagnosis.
This work adheres to a systematic approach to conducting all the experiments. An ultrasound video dataset consisting of four distinct classes is utilized for the experiments. Each class possesses videos of different durations containing crucial temporal features. Developing a deep learning model for video classification is quite challenging since it contains an additional temporal feature. In this study, we propose a hybrid framework comprised of a time-distributed CNN model and LSTM. The time-distributed CNN layer wraps up the input with a temporal sequence. Consequently, it helps to extract the spatial features from each frame. The LSTM layer captures the temporal dependency and predicts the video label. The primary novelty that adheres to this proposed TD-CNN-LSTM model is the ability to capture the temporal features, handling the videos frame by frame, which cannot be processed in baseline CNN models. Additionally, the (2 + 1) D convolutional layer of this model reduces the total parameters number compared to the 3D convolutional layer, resulting in a more efficient execution process. Several image processing techniques are implemented to reduce unwanted artifacts from frames. Moreover, this technique denoises the frames and substantially aids in obtaining remarkable accuracy. Another novelty of this study is the augmentation and frame sequencing technique. This frame sequencing technique includes another key advantage to this study as it maintains the temporal order of the frames, enhances the context utilization as well as improves the overall feature extraction process. In this study, a graph-based approach is applied to sequence the frame after performing the frame augmentation. Each frame is associated with its respective MSE and SSIM values, and the MST concept efficiently determines the best frame sequence and restores the video using that order. The main benefits of this technique compared to the traditional frame sequencing algorithm are the scalability and flexible, efficient representation. It assigns each frame as a node, and the relationship between nodes symbolizes an edge, which improves the structural representation of the algorithm. Moreover, this structural approach increased the scalability and efficiency of this proposed frame sequencing algorithm. The proposed model, combined with the preprocessing and frame sequencing, achieves a satisfactory outcome, obtaining a test accuracy of 96.57%. Additionally, a higher value of precision of 96.56%, recall of 96.51%, and F1 score of 96.02%, along with a lower value of FPR of 0.037%, FDR 0.044%, indicates the consistency and effectiveness of the implemented technique. TD-CNN-LSTM-LungNet has been compared with ten transfer learning models to validate the performance with respect to other models, suppressing the result for all the models. It is also compared with several transformer architectures to highlight the efficiency of the proposed model. Besides, this model is tested on a separate LUS video dataset to establish the applicability of this study in a wide range. The explainability of the proposed model is investigated to increase the reliability of the approach. It generates the heatmap in the frame and highlights the influential area for the classification. This interpretation technique will aid the radiologist in localizing the focus area and improve clinical transparency substantially. In addition to utilizing heat maps to evaluate the model’s decision-making process through explainability, we also conducted an ablation on the baseline model. We segmented ROI from the entire frame to emphasize the significant differences in shape and area between classes. We extracted different shapes, textures, and intensity-based features containing distinctive patterns for different lung diseases using that ROI. We developed a DSS method using features extracted from ultrasound videos. To help with accurate disease classification prediction, we proposed an algorithm and designed a PDF graph with a defined range of a single feature for a specific class. Apart from XAI, our contributions include creating DSS, analyzing features, conducting an ablation study, and coming up with an algorithm that specifies the exact ranges of a certain feature for each class. The application of PDF graphs and DSS performs the critical feature analysis and validates the model’s prediction. PDF graph interprets the feature interaction according to the classes, and the proposed DSS enhances the overall reliability of the classification process by obtaining a remarkable result. The amalgam of these different approaches presents an effective automated solution for efficient lung disease classification.
9 Conclusion
This study introduced a hybrid framework to perform multiclass classification from the LUS video dataset and highlighted the advancements of the computer-aided system in healthcare. The proposed model (TD-CNN-LSTM-LungNet) is developed by integrating the time-distributed CNN layer and LSTM to capture the spatial and temporal dependency of the videos. The frame augmentation technique is applied to prevent the overfitting tendency. Subsequently, a novel frame sequencing technique is employed to establish the flow and continuity of the video. The developed model attained a remarkable accuracy of 96.57% in classifying the videos into pneumonia, COVID-19, normal, and other lung disease classes. Moreover, eleven ablation studies are adopted to determine the model’s optimal parameters, which successfully reduce the training cost and redundancy of the parameters in the model. K-fold cross-validation and the accuracy and loss curve demonstrate the generalization of the model. Ten transfer learning models are also utilized for experimentation, and our model performs best across all the models. Besides, incorporating LayerCAM improves the interpretability and reliability of deep learning, and the heatmap generation helps localize the ROI from the frames for each class. A precise segmentation technique helps to separate the ROI, and some intensity and shape-based features are extracted to create PDF graphs. The graphs facilitate identifying the boundaries of the feature and develop a DSS to increase the practical applicability of this study. Additionally, the validation of this DSS highlights the precise outcome and offers crucial insights and aids the medical expert in making a precise diagnosis. In conclusion, this study advances lung disease classification by integrating the optimal denoising, augmentation, and hybrid models. The incorporation of explainability of DSS underscores the transparency of the model. The intersection of these approaches contributed to a more efficient and accurate diagnosing process and improved the outcomes of AI models in real-world scenarios.
10 Limitations and future works
While this study has significantly contributed to the advancement of multiclass classification from LUS videos, it is also associated with several limitations. It is necessary to acknowledge these limitations to highlight the potential constraints of the proposed methodology. This study conducted the experiment on ultrasound videos; however, including other imaging modalities datasets, such as CT scans and X-rays, would increase the data sets’ diversification and validate the model’s generalizations. Besides, multi-modal and demographic data may increase the stability of the model over time. The deployment of the model in the web-based interface will substantially facilitate real-world clinical practice. Nevertheless, addressing these potential limitations will significantly increase the quality of the paper. This study will investigate other datasets and establish the model’s effectiveness on a broad scale. Longitudinal data will be utilized to perform the lung disease progression simultaneously. Real-time implementation techniques will be integrated to offer an efficient and immediate diagnosis. The geometric deep learning concept can be explored to find the temporal–spatial relationship.
Data availability statement
Publicly available datasets were analyzed in this study. This data can be found here: https://github.com/nrc-cnrc/COVID-US.
Ethics statement
Ethical review and approval was not required for the study on human participants in accordance with the local legislation and institutional requirements. Written informed consent from the patients/participants or patients/participants’ legal guardian/next of kin was not required to participate in this study in accordance with the national legislation and the institutional requirements.
Author contributions
AA: Conceptualization, Investigation, Methodology, Writing – original draft. MK: Data curation, Visualization, Writing – original draft. AK: Formal analysis, Validation, Writing – review & editing. SA: Conceptualization, Investigation, Validation, Writing – review & editing. NF: Data curation, Formal analysis, Visualization, Writing – original draft. NS: Methodology, Supervision, Validation, Writing – review & editing. KY: Formal analysis, Validation, Writing – review & editing. FB: Supervision, Validation, Writing – review & editing.
Funding
The author(s) declare that no financial support was received for the research, authorship, and/or publication of this article.
Acknowledgments
We express our heartfelt gratitude to Dr. Mohammad Ariful Islam (MBBS/MD; Assistant Professor, Kuwait Bangladesh Moitri Govt. Hospital, twenty years of experience), Dr. Sajib Saha (MBBS, BCS, DO- National Institute of Ophthalmology, nine years of experience) and Dr. Synthia Kor (MBBS, BCS, CMU, MO – Sylhet MAG Osmani Medical College, eight years of experience) for their valuable contribution as a medical professional to validate and verify our XAI, generated from our model. All three are licensed under the Bangladesh Medical & Dental Council (BMDC). Dr. Mohammad Ariful Islam with License Number A-26612, Dr. Sajib Saha with License Number A80377, and Dr. Synthia Kor with License Number A82399.
Conflict of interest
The authors declare that the research was conducted in the absence of any commercial or financial relationships that could be construed as a potential conflict of interest.
Publisher’s note
All claims expressed in this article are solely those of the authors and do not necessarily represent those of their affiliated organizations, or those of the publisher, the editors and the reviewers. Any product that may be evaluated in this article, or claim that may be made by its manufacturer, is not guaranteed or endorsed by the publisher.
References
Ambrosch, A., Luber, D., Klawonn, F., and Kabesch, M. (2023). Focusing on severe infections with the respiratory syncytial virus (RSV) in adults: risk factors, symptomatology and clinical course compared to influenza A/B and the original SARS-CoV-2 strain. J. Clin. Virol. 161:105399. doi: 10.1016/j.jcv.2023.105399
American Lung Association (2022). Five facts you should know about pneumonia. Available at: https://www.lung.org/lung-health-diseases/lung-disease-lookup/pneumonia/five-facts-you-should-know
Arif, S., Wang, J., Ul Hassan, T., and Fei, Z. (2019). 3D-CNN-based fused feature maps with LSTM applied to action recognition. Fut. Internet 11:42. doi: 10.3390/fi11020042
Azam, S., Montaha, S., Raiaan, M. A. K., Rafid, A., Mukta, S. H., and Jonkman, M. (2024). An automated decision support system to analyze malignancy patterns of breast masses employing medically relevant features of ultrasound images. J. Imaging Inform. Med. 37, 45–59. doi: 10.1007/s10278-023-00925-7
Bard, R. L. (2021). Multimodality 3D lung imaging. In R. L. Bard (Ed.), Image-guided management of COVID-19 lung disease (pp. 95–130). Springer International Publishing. doi: 10.1007/978-3-030-66614-9_8
Barros, B., Lacerda, P., Albuquerque, C., and Conci, A. (2021). Pulmonary COVID-19: learning spatiotemporal features combining CNN and LSTM networks for LUS video classification. Sensors 21:5486. doi: 10.3390/s21165486
Bhandari, M., Shahi, T. B., Siku, B., and Neupane, A. (2022). Explanatory classification of CXR images into COVID-19, pneumonia and Tuberculosis using deep learning and XAI. Comput. Biol. Med. 150:106156. doi: 10.1016/j.compbiomed.2022.106156
Born, J., Brändle, G., Cossio, M., Disdier, M., Goulet, J., Roulin, J., et al. (2020). POCOVID-net: automatic detection of COVID-19 from a new lung ultrasound imaging dataset (POCUS). arXiv [Preprint] 2004:12084. doi: 10.48550/arXiv.2004.12084
Chen, X., Peng, H., Wang, D., Lu, H., and Hu, H. (2023). Seqtrack: Sequence to sequence learning for visual object tracking. Proceedings of the IEEE/CVF conference on computer vision and pattern recognition (CVPR), 14572–14581. IEEE.
Dastider, A. G., Sadik, F., and Fattah, S. A. (2021). An integrated autoencoder-based hybrid CNN-LSTM model for COVID-19 severity prediction from LUS. Comput. Biol. Med. 132:104296. doi: 10.1016/j.compbiomed.2021.104296
De Groot, P. M., Jimenez, C. A., Godoy, M. C., and Wu, C. C. (2023). Pleural effusions: clues for diagnosis and characterization. Semin. Roentgenol. 58, 431–439. doi: 10.1053/j.ro.2023.06.002
Diaz-Escobar, J., Ordonez-Guillen, N. E., Villarreal-Reyes, S., Galaviz-Mosqueda, A., Kober, V., Rivera-Rodriguez, R., et al. (2021). Deep-learning based detection of COVID-19 using lung ultrasound imagery. PLoS One 16:e0255886. doi: 10.1371/journal.pone.0255886
Dugar, S., Fox, S., Koratala, A., Moghekar, A., and Mehta, A. C. (2023). Lung ultrasonographic signs in pulmonary disease–a video review. J. Intensive Care Med. 38, 220–231. doi: 10.1177/08850666221120221
Ebadi, S. E., Krishnaswamy, D., Bolouri, S. E. S., Zonoobi, D., Greiner, R., Meuser-Herr, N., et al. (2021). Automated detection of pneumonia in lung ultrasound using deep video classification for COVID-19. Inform. Med. Unlocked 25:100687. doi: 10.1016/j.imu.2021.100687
Ebadi, A., Xi, P., MacLean, A., Florea, A., Tremblay, S., Kohli, S., et al. (2022). COVIDx-US: An open-access benchmark dataset of ultrasound imaging data for AI-driven COVID-19 analytics. Frontiers in Bioscience (Landmark Edition), 27:198. doi: 10.31083/j.fbl2707198
Etter, L., Betke, M., Camelo, I. Y., Gill, C. J., Pieciak, R., Thompson, R., et al. (2024). Curated and annotated dataset of lung US images in Zambian children with clinical pneumonia. Radiol. Artif. Intell. 6:e230147. doi: 10.1148/ryai.230147
Jiang, P. T., Zhang, C. B., Hou, Q., Cheng, M. M., and Wei, Y. (2021). Layercam: Exploring hierarchical class activation maps for localization. IEEE Transactions on Image Processing, 30, 5875–5888.
Kapusta, J., Chudzik, M., Kałuzińska-Kołat, Ż., Kołat, D., Burzyńska, M., Jankowski, P., et al. (2023). Do selected lifestyle parameters affect the severity and symptoms of COVID-19 among elderly patients? The retrospective evaluation of individuals from the STOP-COVID registry of the PoLoCOV study. J. Infect. Public Health 16, 143–153. doi: 10.1016/j.jiph.2022.12.008
Kassaw, G., Mohammed, R., Tessema, G. M., Yesuf, T., Lakew, A. M., and Tarekegn, G. E. (2023). Outcomes and predictors of severe community-acquired pneumonia among adults admitted to the University of Gondar Comprehensive Specialized Hospital: a prospective follow-up study. Infection Drug Resistance 16, 619–635. doi: 10.2147/IDR.S392844
Khan, I. U., Raiaan, M. A. K., Fatema, K., Azam, S., Rashid, R., Mukta, S. H., et al. (2023). A computer-aided diagnostic system to identify diabetic retinopathy, utilizing a modified compact convolutional transformer and low-resolution images to reduce computation time. Biomedicines 11:1566. doi: 10.3390/biomedicines11061566
Kruckow, K. L., Zhao, K., Bowdish, D. M., and Orihuela, C. J. (2023). Acute organ injury and long-term sequelae of severe pneumococcal infections. Pneumonia 15, 1–20. doi: 10.1186/s41479-023-00110-y
Li, G. Y., Chen, L., Zahiri, M., Balaraju, N., Patil, S., Mehanian, C., et al. (2023). Weakly semi-supervised detector-based video classification with temporal context for LUS. Proceedings of the IEEE/CVF International Conference on Computer Vision, 2483–2492. IEEE.
Magrelli, S., Valentini, P., De Rose, C., Morello, R., and Buonsenso, D. (2021). Classification of lung disease in children by using LUS images and deep convolutional neural network. Front. Physiol. 12:693448. doi: 10.3389/fphys.2021.693448
Montaha, S., Azam, S., Rafid, A. R. H., Hasan, M. Z., Karim, A., and Islam, A. (2022). Timedistributed-cnn-lstm: a hybrid approach combining CNN and LSTM to classify brain tumor on 3d mri scans performing ablation study. IEEE Access 10, 60039–60059. doi: 10.1109/ACCESS.2022.3179577
Muhammad, G., and Hossain, M. S. (2021). COVID-19 and non-COVID-19 classification using multi-layers fusion from LUS images. Inform. Fusion 72, 80–88. doi: 10.1016/j.inffus.2021.02.013
Nehary, E. A., Rajan, S., and Rossa, C. (2023). Lung ultrasound image classification using deep learning and histogram of oriented gradients features for COVID-19 detection. 2023 IEEE sensors applications symposium (SAS), 1–6. IEEE. doi: 10.1109/SAS58821.2023.10254002
Ostras, O., Soulioti, D. E., and Pinton, G. (2021). Diagnostic ultrasound imaging of the lung: a simulation approach based on propagation and reverberation in the human body. J. Acoust. Soc. Am. 150, 3904–3913. doi: 10.1121/10.0007273
Panigutti, C., Beretta, A., Fadda, D., Giannotti, F., Pedreschi, D., Perotti, A., et al. (2023). Co-design of human-centered, explainable AI for clinical decision support. ACM Trans. Interact. Intell. Syst. 13, 1–35. doi: 10.1145/3587271
Philip, B., Jain, A., Wojtowicz, M., Khan, I., Voller, C., Patel, R. S., et al. (2023). Current investigative modalities for detecting and staging lung cancers: a comprehensive summary. Indian J. Thoracic Cardiovasc. Surgery 39, 42–52. doi: 10.1007/s12055-022-01430-2
Qiao, H., Wang, T., Wang, P., Qiao, S., and Zhang, L. (2018). A time-distributed spatiotemporal feature learning method for machine health monitoring with multi-sensor time series. Sensors 18:2932. doi: 10.3390/s18092932
Raiaan, M. A. K., Fahad, N. M., Chowdhury, S., Sutradhar, D., Mihad, S. S., and Islam, M. M. (2023). IoT-based object-detection system to safeguard endangered animals and bolster agricultural farm security. Future Internet 15:372. doi: 10.3390/fi15120372
Raiaan, M. A. K., Fahad, N. M., Mukta, M. S. H., and Shatabda, S. (2024). Mammo-light: a lightweight convolutional neural network for diagnosing breast cancer from mammography images. Biomed. Signal Proc. Control 94:106279. doi: 10.1016/j.bspc.2024.106279
Rao, G. E., Rajitha, B., Srinivasu, P. N., Ijaz, M. F., and Woźniak, M. (2024). Hybrid framework for respiratory lung diseases detection based on classical CNN and quantum classifiers from chest X-rays. Biomed. Signal Process. Control 88:105567. doi: 10.1016/j.bspc.2023.105567
Roy, S., Menapace, W., Oei, S., Luijten, B., Fini, E., Saltori, C., et al. (2020). Deep learning for classification and localization of COVID-19 markers in point-of-care LUS. IEEE Trans. Med. Imaging 39, 2676–2687. doi: 10.1109/TMI.2020.2994459
Shamrat, F. M. J. M., Azam, S., Karim, A., Islam, R., Tasnim, Z., Ghosh, P., et al. (2022). LungNet22: a fine-tuned model for multiclass classification and prediction of lung disease using X-ray images. J. Pers. Med. 12:680. doi: 10.3390/jpm12050680
Shandiz, A. H., and Tóth, L. (2022). Improved processing of ultrasound tongue videos by combining convlstm and 3d convolutional networks. International conference on industrial, engineering and other applications of applied intelligent systems. (pp. 265–274). Springer International Publishing (Cham).
Shea, D. E., Kulhare, S., Millin, R., Laverriere, Z., Mehanian, C., Delahunt, C. B., et al. (2023). Deep learning video classification of LUS features associated with pneumonia. Proceedings of the IEEE/CVF conference on computer vision and pattern recognition, 3103–3112. IEEE.
Soldati, G., Smargiassi, A., Inchingolo, R., Buonsenso, D., Perrone, T., Briganti, D. F., et al. (2020). Is there a role for lung ultrasound during theCOVID‐19 pandemic? J. Ultrasound Med. 39, 1459–1462. doi: 10.1002/jum.15284
Tsai, C.-H., van der Burgt, J., Vukovic, D., Kaur, N., Demi, L., Canty, D., et al. (2021). Automatic deep learning-based pleural effusion classification in LUS images for respiratory pathology diagnosis. Phys. Med. 83, 38–45. doi: 10.1016/j.ejmp.2021.02.023
Keywords: lung ultrasound, COVID-19, LayerCAM, decision support system, LSTM, CNN
Citation: Abian AI, Khan Raiaan MA, Karim A, Azam S, Fahad NM, Shafiabady N, Yeo KC and De Boer F (2024) Automated diagnosis of respiratory diseases from lung ultrasound videos ensuring XAI: an innovative hybrid model approach. Front. Comput. Sci. 6:1438126. doi: 10.3389/fcomp.2024.1438126
Edited by:
Md. Mohaimenul Islam, The Ohio State University, United StatesCopyright © 2024 Abian, Khan Raiaan, Karim, Azam, Fahad, Shafiabady, Yeo and De Boer. This is an open-access article distributed under the terms of the Creative Commons Attribution License (CC BY). The use, distribution or reproduction in other forums is permitted, provided the original author(s) and the copyright owner(s) are credited and that the original publication in this journal is cited, in accordance with accepted academic practice. No use, distribution or reproduction is permitted which does not comply with these terms.
*Correspondence: Asif Karim, asif.karim@cdu.edu.au