- 1School of Mathematics, Statistics and Computer Science, University of KwaZulu-Natal, Durban, South Africa
- 2Department of Industrial Engineering, Stellenbosch University, Stellenbosch, South Africa
Lung cancer is the leading cause of cancer deaths worldwide. It is a type of cancer that commonly remains undetected due to unpresented symptoms until it has progressed to later stages which motivates the requirement for accurate methods of early detection of lung nodules. Computer-aided diagnosis systems have adapted to aid in detecting and segmenting lung cancer, which can increase a patient's chance of survival. Automatic lung cancer detection and segmentation is a challenging task in aspects of segmentation accuracy. This study provides a comprehensive review of current methods and popular techniques which will aid in further research in lung tumor detection and segmentation. This study presents methods and techniques implemented to solve the challenges associated with lung cancer detection and segmentation and compares the approaches with each other. The methods used to evaluate these techniques and the accuracy rates are also discussed and compared to give insight for future research. Although several combination methods have been proposed over the past decade, an effective and efficient model still needs to be improvised for routine use.
1 Introduction
The leading cause of cancer death is lung cancer (Medical News Today, 2022). Cancer is a disease caused by the uncontrolled growth and multiplication of cells in the body. Lung cancer is the type of cancer that originates in the lungs and can be categorized into two groups which are non-small cell lung cancer (NSCLC) and small cell lung cancer (SCLC). These two types of cancer present and grow differently as seen under a microscope, thus are treated differently (Centers for Disease Control and Prevention, 2022).
Approximately 80% to 85% of lung cancer is categorized as NSCLC which can be further subgrouped as adenocarcinoma, squamous cell carcinoma, or large cell carcinoma depending on the type of lung cells they originate in. Adenocarcinoma starts in cells that normally secrete substances such as mucus and is most often seen in people who currently smoke or formerly smoked. This type of lung cancer is also the most common type of cancer seen in people who do not smoke. Younger people are more prone to adenocarcinoma and is more seen in women than in men. This type of cancer is also detected in outer parts of the lung and commonly found before it has spread giving patients a better outlook than those with other types of lung cancer. Squamous cell carcinoma originates in the squamous cells which are flat cells lining the inside of the airways in the lungs and are often found near the main airway in the central part of the lungs. This lung cancer type is often linked to a history of smoking. Large cell or undifferentiated carcinoma may appear in any part of the lung and tends to progress quickly which can make it difficult to treat. Large cell neuroendocrine carcinoma is a type of large cell carcinoma which is a fast-growing cancer that is similar to small cell lung cancer. Other less common types of NSCLC are adenosquamous carcinoma and sarcomatoid carcinoma. Figure 1 shows an example of lung cancer.
SCLC makes up approximately 10% to 15% of all lung cancer diagnosis. This type of cancer grows and spreads faster than NSCLC and has often spread by the time it is diagnosed in patients. Since it is fast growing, it responds well to radiation and chemotherapy, however; for most patients, the cancer eventually recurs. Other types of lung cancers are lung carcinoid tumors which grow slowly, adenoid cystic carcinomas, lymphomas, and sarcomas, as well as benign lung tumors (American Cancer Society, 2022). Symptoms of lung cancer may only occur at later stages of the cancer and usually resemble symptoms of a respiratory infection. Possible symptoms include changes to a person's voice, frequent chest infections such as bronchitis or pneumonia, swelling of lymph nodes in the middle of the chest, persistent coughing or coughing blood, chest pain, shortness of breath, and wheezing (Medical News Today, 2022).
The prognosis and treatment of lung cancer is dependent on cancer detection and diagnosis. The quicker and more accurate the diagnosis, the faster the prognosis which can increase the likelihood of survival. Once symptoms of lung cancer start to present, it is usually visible on an X-ray and appears as an abnormal mass or nodule (WebMD, 2022). A computed tomography (CT) scan is also used to detect lung cancer and can reveal small lesions in the lungs that may not be detected in an X-ray. Other ways to detect lung cancer include sputum cytology and a biopsy which is extracting a tissue sample to confirm the diagnosis. Imaging techniques such as CT and PET scans can determine areas where the cancer occurs and how far it has spread (Mayo Clinic, 2022). CT is extensively utilized by clinical radiologists for the rationale of identifying and treating thoracic diseases.
This comprehensive survey aims to achieve the following:
• Show that deep learning techniques have allowed for significant contributions within the field of lung cancer detection and segmentation.
• Identify the challenges associated with the detection and segmentation of lung cancer within lung CT/MRI scans.
• Highlight the methods and techniques existing in recent studies that have successfully overcome identified challenges.
This study serves to review the current lung nodule segmentation techniques for lung cancer detection. The different strategies used to approach the problem are discussed and analyzed. The study is organized as follows. Section 2 discusses the challenges presented by lung nodule segmentation. In Section 3, a diverse number of techniques are reviewed including pre-processing methods, region of interest extraction techniques, and lung nodule segmentation techniques. In Section 4, commonly used datasets are highlighted, and in Section 5, evaluation methods are presented. Section 6 includes an overall discussion, and Section 7 concludes the review study.
2 Lung nodule segmentation challenges
Accurate lung nodule segmentation is crucial for various lung cancer diagnosis and treatment procedures such as screening for early detection, diagnosis of tumor malignancy, and monitoring tumor response to therapy. Currently, it is standard practice to manually segment the lung nodules with the assistance of CAD systems. However; manual segmentation and detection relies heavily on user interaction and is subjective with high intra- and inter-observer variability in assessing and reporting (Kadir and Gleeson, 2018). Different radiologists may interpret the medical images differently which also depends on the performance of the radiologists. Manual lung nodule segmentation and detection is also poorly reproducible and can be very time-consuming. With manual segmentation and detection of lung cancer, there is always room for human error. CAD systems have proved to have the ability to detect cancer that is undetectable. Approximately 30% of lung nodules go undetected at the initial screening stage for lung cancer using routine manual screening where imaging modalities are used, such as X-ray, CT, and MRI, but are interpreted by human medical professionals (Svoboda, 2020). With the number of experienced radiologists in comparison with the amount of CT scans needing to be analyzed, the demand for consistent performance of these professionals is relatively high and the work load can cause over-stretched radiologists to make mistakes which may pose a risk to accurate segmentation and detection of the cancer. The limitations of the human eye make it easy for radiologists to overlook tiny lesions or lung spots invisible to the naked eye.
Differences in imaging protocols such as scanner models, settings, and patient positioning can lead to inconsistencies in image quality which can influence the performance of the segmentation model. Images can contain noise and artifacts which can obscure nodules or create false positives, especially in low-dose scans (Song et al., 2021). Inter- and intra-patient variability also pose significant challenges in lung nodule segmentation. The variability in nodule characteristics such as size, shape, and texture between patients makes it difficult for segmentation algorithms to consistently identify and delineate nodules. Even within the same patient, scans taken at different times can present nodules differently due to changes in size, shape, or density which can add complication to the segmentation process (Gao et al., 2024). The heterogeneity of lung tissue can also vary in appearance due to factors such as age, disease, and smoking history which contributes to making it difficult for the segmentation model to distinguish nodules from other anatomical structures within the lung (Osadebey et al., 2021).
Different types of nodules appear differently on CT scans because each type has unique characteristics. Segmentation of large solid nodules is not complex whereas the segmentation of small nodules attached to the vessels or parenchymal wall and diaphragm can be challenging. Small nodule detection plays a vital role in early lung cancer detection and is needed to assess malignancy of lesions. One of the challenges associated with small nodules is the partial volume effect (PVE) where only part of the small nodule volume is visible in the CT scan. Since these nodules are small and attached to another tissue's surface, they are also difficult to detect for segmentation. Some nodules are sub-solid and are referred to have ground-glass opacity (GGO) where the CT values are lower than typical solid nodules making them difficult to detect as well (Pati et al., 2022). According to growth pattern and morphology, lung cancer presents differently depending on the sub-type of the cancer but this can be challenging as sometimes multiple morphology sub-types exist across a single scan of a patient. It is common practice to use the dominant sub-type to diagnose the patient which may result in loss of information for other sub-types and this analysis can be time-consuming and challenging for pathologists (Wang et al., 2019). Table 1 summarizes the challenges of lung cancer detection and segmentation.
Research has shown that the curability of lung cancer is 75% if it is diagnosed early as it is easier to treat and there are fewer risks (Mahersia et al., 2015). Therefore, the early diagnosis of lung nodules is crucial for reducing morbidity and mortality. CAD systems are developed for the detection and characterization of lesions to diagnose lung cancer where the main objective is to provide assistance to radiologists in the different steps of analysis and offer a second opinion or support less experienced or non-specialized clinicians in the field. The use of CAD systems also aims to reduce variability in assessing and reporting lung nodule segmentation and detection (Kadir and Gleeson, 2018). Recently, many studies have been conducted where research is focused on making such systems more automatic. However, many pulmonary nodule detection and segmentation systems lack adequate accuracy, and for diagnostic systems involving terminal illnesses, it is vital that these systems are developed to reach the highest accuracy rate of 100%.
3 Review of existing lung nodule detection and segmentation methods
3.1 Introduction
To overcome the aforementioned challenges, authors have proposed various methods for automatic and semi-automatic detection and segmentation of lung nodules. Thus far, the proposed methods have shown great success; however, it is important to note that machine learning methods have far surpassed the results achieved by non-deep learning methods. The typical phases incorporated into a lung cancer detection and segmentation framework include pre-processing, region of interest extraction, lung cancer segmentation, and lastly, post-processing. To summarize the current progress of the application of various methods to lung cancer detection and segmentation, a survey of recent publications was performed.
3.2 Pre-processing
Image pre-processing techniques are applied to images to standardize the dataset that will be processed further. In medical image processing, the datasets used are often the likes of CT or MRI scans where the image sizes, spacing between voxels, contrast, and quality may vary from patient to patient. Pre-processing techniques are also explored and applied to improve the quality of the images which can improve the segmentation accuracy. Many pre-processing techniques exist that can be applied to these images to prepare the dataset.
Low image quality can be an obstruction for effective feature extraction, analysis, identification, and quantitative measurements (Vijayaraj et al., 2021). To improve image quality, Madan et al. (2019) used smoothing of the images. A middle outlet technique was used by Bhaskar and Ganashree (2020) which is a non-linear programmed channel used to remove noise from the images. The images were then converted to grayscale. Bushra et al. (2019) and Kamal et al. (2020) also used a simple grayscale conversion as a pre-processing method. Halder et al. (2020), Sasikala et al. (2019), and Akter et al. (2021) convolved the lung CT images used median filtering to minimize the effects of the degradations that occur during acquisition of the CT scans. Median filtering is a technique that removes noise while keeping the edges intact. Joon et al. (2019) converted chest X-ray images to grayscale and applied median filtering to remove noise in a study to segment and detect lung cancer as well. Meraj et al. (2021) applied a low pass filter to remove noise in 2D images, called the weiner2 filter, which uses the variance and local mean around every pixel in the image. Kalaivani et al. (2020) employed pre-processing where resizing and blur removal of images were done using histogram equalization. Manoharan et al. (2020) utilized morphological operations such as erosion and dilation to obtain a top hat result. The original images are superimposed with the top hat, and the bottom hat is subtracted from the image. Bushra et al. (2019) converted images to grayscale and used the sobel operator to find the edges by finding the gradient magnitude.
Another technique for pre-processing is cropping, rescaling, or dividing the images to create smaller patches in the event that the next stage in the process required the images to be a smaller size or resolution. Liu et al. (2018) also used this technique to extract 50 × 50 blocks to be fed to the stage following pre-processing. Serj et al. (2018) rescaled the images to a smaller size. The data pre-processing implemented by Ozdemir et al. (2019) included windowing the image range between [–1,000, 400] HU and resampling the images to set the voxel size to 1mm in all dimensions. Thereafter, the images were normalized to have a mean standard voxel value of 0 and variance of 1. Normalization, a technique applied to window pixel values within a specific range, is also a commonly used technique for pre-processing. Bhatia et al. (2019) standardized the dataset with normalization and zero centering, and Gunasekaran (2023) used rescaling and normalization. Liu et al. (2018) applied normalization where the images were windowed between [–1,024, 800] Hounsfield Units (HU) as it was found that those pixel values were relevant to nodule detection. Bansal et al. (2020) also transformed the pixel values to Hounsfield Units which were then windowed between [–1,000, –320] HU to reduce the search space. Baek et al. (2019) resampled pairs of co-registered PET-CT images with an isotropic spacing in all dimensions, cropped the images, and clipped the voxel intensity values to appropriate ranges to remove outliers. Dong et al. (2020) applied resampling to the CT images to standardize the images and then performed multi-view patch extraction where 30 × 30 patches are extracted from the axial, coronal, and sagittal views of the CT image. Borrelli et al. (2022) also truncated CT images to a HU range of [–800, 800] and the PET image to [0, 25], and then, both images were rescaled to [–1, 1]. Thereafter, lung tumors and thoracic lymph nodes with tumor lesion glycolysis were removed from the dataset. Kamal et al. (2020) converted 3D volumes into 2D grayscale, 256 × 256 images. Thereafter, 8 consecutive images are concatenated to create 256 × 256 × 8 patches. As part of the pre-processing step, Riaz et al. (2023) converted 3D images to 2D, resized, and then normalized to improve contrast issues.
Data augmentation is a commonly known technique used for pre-processing especially when it comes to training machine learning models (Perez and Wang, 2017). The more data fed into these models, the more effective the training can be therefore making the model perform efficiently and accurately. With smaller datasets, models suffer from the problem of over-fitting and data augmentation allows for the alleviation of this. In the studies done by Madan et al. (2019), Zhang et al. (2018), Ozdemir et al. (2019), Gunasekaran (2023), and Zhang et al. (2020) all implemented some type of data augmentation to increasing the amount of data propagated through their respective proposed models with the intention of improving their accuracy rates. Zhang et al. (2018) utilized data augmentation which was used to increase the size of the dataset using random cropping, rotation, and flipping of the images to increase their training sample size. Ozdemir et al. (2019) utilized data augmentation extensively. Affine transform augmentation consisted of uniformly sampled 3D rotations and reflections were used and smaller random scaling from 0%–0.06% and translations from 0-1 independently in all dimensions was used. Image transformations also included random gamma transformations, Gaussian blur, unsharp masking, and additive Gaussian noise. Zhang et al. (2020) also implemented data augmentation techniques to enrich their dataset where applying flipping, translations, scaling, and cropping operations to make minor adjustments to the position, shape, and size. Liu et al. (2018) also implemented data augmentation to increase their negative samples as it was discovered that there was an imbalance in the number of positive samples where nodules existed and negative samples where the blocks did not include nodules so data augmentation was used to increase the number of positive samples by more than 20 times. Borrelli et al. (2022) employed data augmented using rotations from –0.15 radians to 0.15 radians, scaling from –10% to 10% and intensity shifts from –100 to 100 HU for CT images and –0.5 to 0.5 for PET images. Kamal et al. (2020) and Riaz et al. (2023) utilized data augmentation techniques at runtime such as random rotation, random cropping, random global shifting, random global scaling, random noise addition, and multiplication, horizontal flipping and blurring have been applied to expand the dataset. Figure 2 shows an example of data augmentation.
Overall, there were a few different techniques that were used such as noise reduction techniques contrast enhancement, rescaling, and data augmentation. A summary of the pre-processing methods and their advantages and disadvantages are presented in Table 2. The most prevalent technique used is data augmentation or it is used in conjunction with one of the other pre-processing techniques as it is the most useful for training deep learning models. Several researchers have made it evident that the use of pre-processing techniques are key contributors to obtaining a higher accuracy rate for lung nodule detection and segmentation (Mahersia et al., 2015).
3.3 Lung region of interest extraction
Segmentation of the lungs from the images is usually the second step in the process of lung cancer segmentation. This lung extraction step aims to separate the pixels or voxels corresponding to lung tissue and eliminate the surrounding regions which should not be considered for further processing (Mahersia et al., 2015). This process reduces the search space for lung nodule detection. There are many methods proposed to achieve this as mentioned below which aims to extract the lungs for further processing where lung tumors can be extracted. An example of lung region of interest extraction can be seen in Figure 3, where the first column shows the original CT slices including lung tissue, the second column highlights the ground truth data for the segmented lung, the third column shows the segmented lung binary mask using a lung segmentation technique, and the fourth column presents the segmented lung.
Bhatia et al. (2019) implemented a series of region growing and morphological operations to identify and extract the lungs and nodules to aid the feature extraction. Bhaskar and Ganashree (2020) proposed a lung cancer detection method from CT images where for their lung region extraction phase bit-plane slicing, erosion, median filter, and dilation are applied to identify the lung area within the CT scan. During the lung region segmentation phase, a Fuzzy Possibility C-Mean (FPCM) which is a combination of hybridization of Possibilistic C-Means (PCM) and Fuzzy C-Means (FCM) is utilized with watershed transformation.
Halder et al. (2020) used a method where the lungs are extracted using iterative thresholding and the two largest regions, the left and right lungs, are obtained. Morphological closing is used to create a final lung mask which is then used to extract the lung region of interest from the original pre-processed images. The extracted lung regions of interest are further processed in the next step: internal structure segmentation. Meraj et al. (2021) used the Otsu thresholding method for segmentation of the lung region of interest. The resulting image is binary and exposes the lungs, but there are still unnecessary blobs present which are removed by only extracting the largest regions in the image.
Christe et al. (2019) carried out a study aimed at evaluating the performance of the INTACT system which is a CAD designed for automatic classification of IPF (idiopathic pulmonary fibrosis). The INTACT system consists of multiple stages where the first stage segments the airways and lung parenchyma. The algorithm used for this consists of extraction of lung airways, segmentation of lung regions, separation of the left and right lungs, and morphological 3D smoothing. In a study for automated classification of lung cancer sub-types using deep learning and CT scan-based radiomic analysis by Dunn et al. (2023), the CT scans were first analyzed and then a bounding box was used to manually select the tumor ROI. A few slices were added above and below the bounding box as a buffer, and these volumes were fed into the segmentation model.
In many of the recent studies carried out, this step of lung extraction is not considered entirely. This is especially in deep learning-based methods where the models are trained to search and recognize the lung nodules or tumors directly from whole CT scan images without reducing the search space for detection.
3.4 Lung cancer detection and segmentation
Lung cancer radiotherapy requires accurate delineation of the tumor to design precise patient-specific radiotherapy plans which are based on CT images to deliver high irradiated doses to the target volume while sparing surrounding organs as much as possible. Therefore, accurate segmentation of the target volume is very important for successful delivery of radiotherapy (Zhang et al., 2020). An example of a segmented tumor in a lung CT is shown in Figure 4. In this section, a variety of lung nodule segmentation methods are discussed. A summary of lung cancer detection and segmentation techniques is presented in Table 3.
3.4.1 2D methods
Kalinovsky et al. (2017) conducted a study to examine the capabilities of deep convolution networks to automatically detect different types of tuberculosis lesions. Two different 2D techniques were explored in this study. The first is the sliding window technique. For this, 2D regions of size 128 × 128 pixels were automatically extracted and were manually divided into two classes regions without any lesions and regions with lesions. These ROIs were resized to 256 × 256 to fit the input size of the deep convolutional network being used. For this technique, GoogLetNet was trained on the training set of 2D images and demonstrated a classification accuracy rate of 93.2%. The second technique is a 2D slice-wise segmentation technique where for each slice its two neighboring slices were used to construct a single RGB image to utilize spatial information. Then, each 512 × 512 was split into four quadrants and resized to 256 × 256 to fit the network. For each quadrant, a corresponding label image was developed manually dividing the image into three different regions which are non-lesion regions, lesion regions, and “don't care” regions which refer to regions outside the lung regions. AlexNet was employed for this technique and achieved an accuracy rate of 88.7% for segmentation. The results of these techniques were also evaluated using the receiver operating characteristic curve as the metric. The 2D-sliding window and the 2D slice-wise technique achieved a ROC-curve equal to 0.784 and 0.785, respectively.
Liu et al. (2018) presented a method to approximate pulmonary nodules on 2D slices using a Mask R-CNN to predict the nodule position and the nodule contour size. The Mask R-CNN model was trained on the COCO dataset and then validated using the LUNA16 dataset. The ResNet101 was used as the Mask R-CNN classification network and FPN as the Mask R-CNN as the detection network. Two methods to train the Mask R-CNN were used. First, the training data were used to fine-tune all layers directly on the pre-trained model of COCO data set. The accuracy obtained from this model was a mean average precision of 0.733. The second method was to train the network heads first and then fine-tune ResNet stage 3 and above and lastly fine-tune all the layers. This obtained a mean average precision of 0.796.
Serj et al. (2018) proposed a new deep CNN (dCNN) architecture to diagnose lung cancer. The network consists of four convolution layers, two max-pooling layers, a full body convolution layer, and one fully connected layer with two softmax units. Each convolution layer in the network uses a ReLu layer. There are two convolution layers at the beginning of the network in which the first convolution layer consists of 50 feature maps with an 11 × 11 kernel and takes as input a 120 × 120 image. The second convolution layer consists of 120 feature maps with 5 × 5 kernel, and the last convolution layer consists of 120 feature maps with a 3 × 3 kernel. The max-pooling kernel size is 2 × 2 with a stride of 2 pixels. The fully connected layer generates 10 outputs which are then passed to another fully connected layer containing 2 softmax units which represent the probability of lung cancer or not. A softmax loss function is used in this model. The model performs forward propagation on each mini-batch and computes the output and loss and then back-propagation is used to compute gradients on the batch and network weights are then updated using stochastic gradient descent. The proposed CNN is evaluated using three metrics: sensitivity, specificity, and F1 Score. The proposed lung cancer diagnosis method achieved a sensitivity of 0.87, specificity of 0.991, and an F1 score of 0.95 which proves that this method performed well and produced desirable results.
An investigation carried out by Zhang et al. (2018) included experimenting with different deep neural networks to determine the most accurate for the purpose of lung cancer tumor region segmentation. After experimenting with a few models, the U-Net structure proved to achieve the highest accuracy which was the chosen model for this study. Some minor modifications were made to the U-Net structure so that it could be adapted for the purpose of lung tumor segmentation. Each block in the architecture consists of two convolution layers with 3 × 3 filters followed separately by a rectified linear unit layer. Downsampling is used on the encoding half of the structure with the use of 2 × 2 max-pooling layers with a stride of 2, and 2 × 2 upsampling is used in the decoding half of the structure. Feature maps are copied from each layer on the downsampling half to the corresponding upsampling half of the U-Net architecture. Finally, a 1 × 1 convolution is used which followed by a sigmoid function, and dice loss is used as the loss function. A few experiments were done to determine the efficiency of the proposed approach which were evaluated using six metrics: dice Coefficient, Hausdorff distance, slice-wise missing rate, false-alarm rate, and CT scan-based accuracy. The slice-wise missing rate is defined as the percentage of missed detection of slices with a tumor. CT scan-based accuracy is based on the intersection-over-union of the prediction of three consecutive slices. The experiments were done using two threshold variations with the U-Net model which are 0.5 and 0.0001 as threshold values. The results obtained using the U-Net with threshold 0.5 were dice coefficient of 0.547, mean surface distance of 12.505, 95% Hausdorff distance of 29.336, slice-wise missing rate of 25.4%, false-alarm rate of 33.9%, and CT scan-based accuracy of 90%, and the results obtained using the U-Net with threshold 0.0001 were dice coefficient of 0.475, mean surface distance of 27.014, 95% Hausdorff distance of 75.978, slice-wise missing rate of 22.2%, false-alarm rate of 75.2%, and CT scan-based accuracy of 95%.
Bhatia et al. (2019) proposed the use of a modified ResNet architecture where the feature extraction is done by the ResNet-50 imagenet11k + Places365 which is an architecture consisting of ResNets or stacked residual units. The feature set is then fed into classifiers like XGSBoost and Random Forest. A few experiments were done to determine the most accurate ensemble of techniques. First, the U-Net architecture was used for feature extraction paired with the Random Forest classifier which achieved an accuracy of 74%. Then, the ResNet architecture was paired with the XGBoost which achieved an accuracy rate of 76%. Finally, the ResNet was used for feature extraction which was paired with an ensemble of the Random Forest and XGBoost classifiers which achieved a higher accuracy rate of 84%.
For the study conducted by Christe et al. (2019), multiple databases were used for the training and evaluation of the different components of the INTACT system such as the Lung Tissue Research Consortium Database (LTRC-DB), Multimedia Database of Interstitial Lung Diseases (MD-ILD), and the Inselspital Interstitial Lung Disease Database (INSEL-DB). The ground truth data were determined by four radiology specialists, and the data were classified into four categories: Typical UIP CT pattern, Probably UIP CT pattern, CT pattern indeterminate for UIP, and CT features most consistent with non-IFP diagnosis. Once the lungs are segmented, a CNN is used for tissue characterization in the second stage. The proposed system for pathological tissue segmentation uses texture to detect classify and calculate the extent of disease in tissue based on pathologies such as reticulation, honeycombing, ground glass opacity, consolidation, micronodules, and normal lung. The CNN takes as input a section of a 2D CT slice and outputs a corresponding tissue pathology label map for each pixel. The next stage is the diagnosis based on the results of the lung tissue characterization. In this stage, the lungs are segmented into 12 lung segments to calculate the distribution of the different pathological tissue types in the different segments of the lung. The techniques used for this were a volume-based split for the upper, middle, and lower segmentation, and k-means clustering was used for the central and peripheral segments and the fast-marching method. The distribution of the different tissue types estimated for each segment was used to train multiple one-versus-all random forest classifiers to classify the lung fibrosis for each of the 4 cases. The proposed system was evaluated using sensitivity, accuracy, and positive predictive values. The positive predictive values and sensitivity were used to calculate the F-Score. The results achieved by the INTACT system were also compared to that of 2 radiologists who were blind to the ground truth data. The accuracy achieved by the system for classifying the pulmonary fibrosis was 0.6 and an average of 0.55 by the radiologists. The system achieved an F-Score of 0.56, and the radiologists achieved an average F-Score of 0.57.
The lung cancer detection method proposed by Madan et al. (2019) was done using a convolutional neural network (CNN) made up of convolution and pooling layers which produced the segmented image. This model was validated using 1623 images and achieved a validation accuracy of 93%, a preciseness of 89.2%, recall of 72%, and sensitivity of 98.2%.
Park and Monahan (2019) carried out an investigation using a genetic algorithm to conduct a neural architectural search to generate a novel CNN to detect lung cancer in chest X-rays. The NEAT algorithm is modified to evolve a CNN's architecture which is named DeepNEAT-Dx. Convolution and pooling layers with pseudo-random hyper parameters are injected into a minimal convolutional architecture and weights are optimized through back-propagation on the training set. Schiffman encoding which is a direct graph encoding scheme was used which allows for easily programmable rules for mutations. This is to ensure that mutations do not result in illegal architectures such as convolving to negative dimensions. Each vertex in the genome graph encoding represents a CNN layer which stores hyper parameter information such as filter size, stride, padding, and weight initialization method. After the genetic algorithm produced a graph encoding of a network which was then exported to be tested. The DeepNEAT-Dx produced an accuracy rate of 97.15% for lung cancer detection.
In a study done by Kalaivani et al. (2020), images were fed into a CNN where the images were classified and an output was obtained. The algorithm used for classification is ADABOOST where accuracy calculation is done based on sample weights of images. To measure the accuracy of the detection and classification of lung cancer in images using these approaches, 11 images were used to evaluate the proposed method. The accuracy was determined by the number true positives divided by the sum of true positives and false positives. The results achieved were 90.85%; however, only a small number of images were considered for the evaluation out of a relatively large dataset.
Zhang et al. (2020) used a modified version of ResNet to segment the tumor volumes in patients with inoperable NSCLC where an encoder-decoder structure similar to U-Net was adopted. The encoding path uses a ResNet34 backbone to extract deep features, and a lightweight dense-prediction branch was applied in the decoding path. Deep semantic features at multiple spatial resolutions were concatenated in the channel dimensions and then merged with shallow features to generate dense pixel outputs. The ResNet34-based encoder is divided into five stages where each feature map is generated at different scales. The ResNet34 architecture employed cross-layer connection via identity mapping. The structure of the residual learning block contained an identity residual block and a convolutional residual block. The convolutional residual block adds the convolutional values to the appropriate branch of the identity map to change the dimension of the feature map. The feature maps of stages 3, 4, and 5 were upsampled by bilinear upsampling and convolution until they reached of the input size. These deep semantic features including different levels of global information were concatenated in the channel dimension and passed through a stack of convolutional layers to fuse the features until they reached the number of feature maps in stage 2. Thereafter, the values are added and upsampled until they reach the size of the input image. Finally, pixel-wise classification is carried out using the sigmoid function and weighted cross-entropy was used to force the loss function to more attention to the foreground class. The size of the feature maps is reduced until they were of size 16 × 16 and then gradually restored by the decoding network until they reached the input image size of 512 × 512. The modified ResNet architecture is depicted in Figure 5.
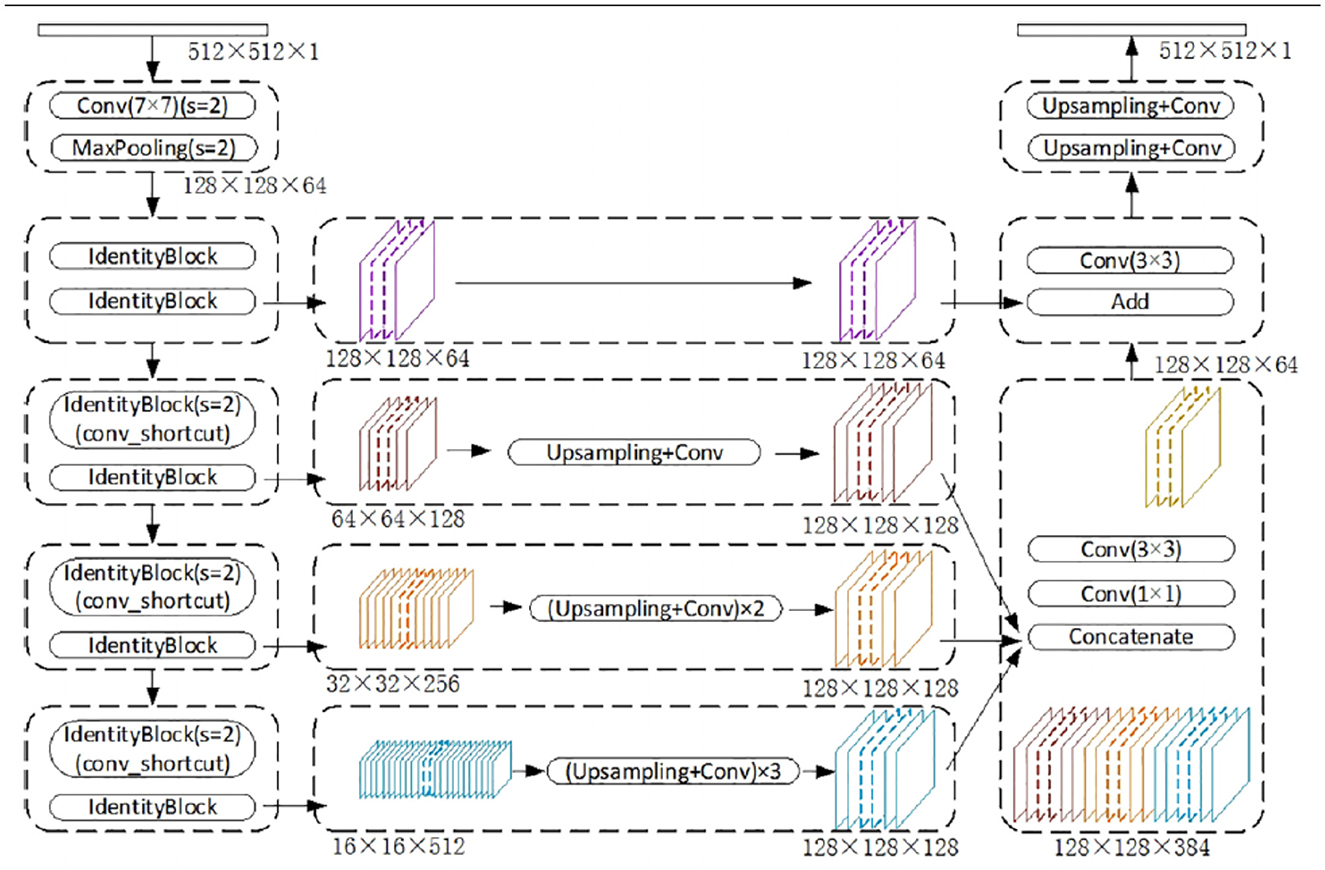
Figure 5. Modified ResNet architecture proposed by Zhang et al. (2020).
The proposed method achieved an average DSC of 0.73, JSC of 0.68, TPR of 0.74, and FPR of 0.0012 which was comparable to manual segmentation especially for larger tumors. The proposed method also performed better than a U-net architecture where the U-Net model achieved a DSC of 0.64, JSC of 0.52, TPR of 0.61, and FPR of 0.0008. However, this study has its limitations where the training set did not have many cases because of limited availability of datasets and the segmentation results were affected by tumor position, size, shape, and respiratory and cardiac motion. Second, distance metrics such as Hausdorff distance were not applied to measure the contour's degree of spatial conformity. Third, inter-observer and intra-observer variability was not considered in this study, and finally, tumors that were small and attached to the mediastinum were challenging to segment accurately as 2D networks ignore the inter-slice relationship between slices of the same patient.
In the study done by Ismail (2021), three different datasets were used to evaluate the performance of deep learning methods for lung cancer detection, namely, the cancer imaging archive (TCIA), the lung image database consortium image collection (LIDC-IDRI), and the Kaggle data science bowl 2017. First a U-Net CNN model for nodule segmentation was used which gave a dice coefficient of 67.8%. A second CNN was used for reducing the false positives of detected nodules which converged to a validation accuracy of 84.4% at detecting a nodule as true positive or false negative. Together, the models achieved a sensitivity of 0.75 and the average false positives per scan was 0.06.
Meraj et al. (2021) carried out a study where the segmentation of the lung nodules is done by a CNN model for the semantic segmentation of candidate nodules. The lung ROI produced in the previous stage is used as the input to the CNN and is of size 512 × 512 × 1 with zero center normalization. The next layer is a convolution layer that performs 64 filters with a size of 3 × 3 × 1 followed by the ReLu activation function and max-pooling of size 2 × 2. This is repeated with a filter size of 3 × 3 × 64 convolutions. Thereafter, a transpose layer which uses 4 × 4 × 64 convolutions with a 64 filter size is applied and is followed by 3 convolution layers, two fully connected layers, the softmax layer calculates the probability of the pixels being nodules, vessels, or background.
Angeline et al. (2022) conducted a study focusing on the identification of malignancy of lung cancer using deep learning methods. This study focused on the detection of tumor types such as benign, unsure, or malignant, using the VGG-16 neural network on the LIDC-IDRI dataset. The VGG-16 network has 16 neural network layers and filters which locate the nodules in the CT scans allowing for the detection of malignant and non-cancerous cells which is achieved by multiple parameters and hyper-parameters to detect benign and unsure tumors. The network consists of five group convolutions that are shared by the subsequent sub-networks. For the region proposal network, an image is set as the input to a network composed of an FCN which outputs a set of rectangular object proposals. To generate region proposals, a small network is slid over the feature map output by the feature extraction network. This small network uses 3 × 3 spatial windows as input into the model. The accuracy rate achieved by the proposed framework was 78.87% and a precision on 83.22%.
To evaluate the performance of a deep learning-based lung nodule detection system, Cui et al. (2022) carried out an experiment where the performance of a deep learning system was compared to that of manual CT scan readings carried out by radiologists. The system uses maximum intensity projection (MIP) technique and was trained on the LIDC/IDRI dataset comprising of nodules larger than 3mm. The system consists of four CNNs with a U-Net architecture which are applied to predict the possible nodule candidates. The nodule candidates produced by these four networks are merged and passed as input to two CNNs with a VGG-Net architecture which are used to differentiate true nodules from false positives. The FROC curve measuring the sensitivity at various false positive rates was used to present the performance of the DL-CAD system. The nodule detection performance of the system showed a sensitivity of 90.1% whereas the double reading by the radiologists achieved a sensitivity of 76.0%. The number of false positives found by the DL-CAD system was more than that found by the radiologists in the double reading which included pulmonary vessels, fibrosis, gastric mucosa, and irrelevant small nodules. It was also found that the DL-CAD system found a large number of nodules that were missed by the radiologists which proves that the system improves nodule detection performance.
The study by Salama et al. (2022) introduced a framework that employs a generative model to synthesize chest X-ray (CXR) images featuring tumors of various sizes and positions. This approach helps balance class distribution in datasets, which is crucial for training robust classification models. The generated images were used to train a ResNet50 model, achieving high accuracy in distinguishing between benign and malignant tumors. The authors present a generalized framework that utilizes two types of deep models: a generative model and a classification model. The generative model captures the distribution of important features in a set of small, class-unbalanced CXR images. This model can synthesize any number of CXR images for each class, effectively balancing the dataset. By creating synthetic images that mimic real lung cancer images, including tumors of various sizes and positions, the generative model helps overcome the challenge of limited annotated datasets in medical imaging. The results demonstrated high performance, with an overall detection accuracy of 98.91%, an AUC of 98.85%, a sensitivity of 98.46%, a precision of 97.72%, and an F1 score of 97.89%.
Shimazaki et al. (2022) proposed a CNN based on the encoder-decoder architecture to produce a segmentation of the tumors present and has a bottleneck structure which reduces the resolution of the feature map. The model was trained on both chest radiographs as well as a black and white inversion of the radiograph which was considered to be augmentation of the data. The deep learning model had an average sensitivity of 0.73. For the lung tumors that overlapped with blind spots such as apices, pulmonary hila, chest wall, heart, or sub-diaphragmatic space, the average sensitivity achieved was 0.52, 0.64, 0.52, 0.56, and 0.50, respectively. The average DSC achieved was 0.52. For the lesions detected by the model, the average DSC was 0.71 and the DSC achieved for all the lesions that were overlapping the blind spots was 0.34. The total number of false positives was 13% where 95% of the FPs overlapped with vascular shadows and ribs and some were nodules were overlapped with normal anatomical structures. The total number of false negatives were 27% which were also made up of lesions overlapping with normal anatomical structures. It was difficult for the model to identify lung cancers that overlapped with blind spots even when the tumor size was large.
Gunasekaran (2023) conducted a study that leverages object detection for the identification of lung cancer. The objective was to explore the application of YOLOv5, an object detection framework, in lung cancer detection. The cancer detection technique used is the YOLOv5 which is a model that combines model assembly and hyper-parameter. The model is made up of three sections: the Backbone module consisting of Cross Stage Partial Darknet (CSPNet) which is responsible for extracting features form the input images, the Neck module which creates the pyramid features for generalization using the PANet, and the Head module is used for detection and add a bounding box with a score around the detected cancer. The proposed method exhibited proficient results where it was able to detect malignant areas in the chest X-rays. This was evident in the evaluation of the method using the Kaggle chest X-ray dataset that the method achieved a sensitivity was 94%, a specificity was 90.5%, a precision was 100%, and a recall was 95%.
Riaz et al. (2023) developed a hybrid model that infuses the MobileNetV2 and the U-Net models for lung tumor segmentation from CT images. The pre-trained MobileNetV2 was used, retaining the convolution layers as the encoder of the U-Net architecture and the decoder consists of upsampling and convolutional layers. Skip connections with the ReLU activation function are established between the encoder layers of the MobileNetV2 to the decoder layers of the U-Net. A final convolution layer is added to the end of the decoder part to obtain the correct number of classes to generate probability maps which determine the tumor and the background. The proposed model was further trained and fine-tuned with optimized hyper-parameters to improve the segmentation accuracy. The dataset used to train and evaluate the model was from the TCIA dataset. The proposed model achieved a dice score 0.8793, a recall of 0.8602, and precision of 0.93. The result achieved by this proposed hybrid architecture proved to have significant accuracy; however, using of a more diverse dataset for evaluation of the model as well as the exploration of post-processing methods could aid in possible improvements to the outcome.
Various GAN architectures were employed in a study by Cai et al. (2024) to perform image translation, converting original lung images into segmented images. The GAN consists of two main components: a generator and a discriminator. This network is responsible for generating segmented lung images from the input CT scans. It learns to produce realistic and accurate segmentation by translating the original lung images into segmented versions. This network distinguishes between real segmented images (ground truth) and the generated segmented images. It helps the generator improve by providing feedback on the realism of the generated images. The GAN was trained using a dataset of lung CT images with corresponding ground truth segmentation. The training process involves the generator creating segmented images, which are then evaluated by the discriminator. The discriminator's feedback helps the generator refine its outputs to produce more accurate segmentation. The loss functions for both the generator and discriminator are optimized iteratively to improve the quality of the generated segmentation. The GAN leverages its image translation capabilities to convert the original lung images into segmented images. This process involves learning the mapping from the input CT scans to the desired segmented outputs. This approach leverages the powerful image generation capabilities of GANs to enhance segmentation accuracy.
The research presented by Wang et al. (2024) focuses on improving early lung cancer detection using a growth predictive model based on the Wasserstein Generative Adversariel Network framework. The model predicts the growth patterns of lung nodules in follow CT scans using baseline scans. The model was trained on a dataset containing pairs of nodule images taken approximately 1 year apart. The model was tested on an independent set of 450 nodules. It predicted the appearance of nodules in follow-up scans and classified them as malignant or benign using a lung cancer risk prediction (LCRP) model. The model achieved a test AUC (area under the curve) of 0.827, which was comparable to the AUC of 0.862 achieved by the LCRP model using real follow-up nodule images. This indicates that the model's predictions were nearly as accurate as actual follow-up scans.
3.4.2 3D methods
In the investigation to automatically detect different types of tuberculosis lesions, Kalinovsky et al. (2017) proposed a third technique which explored 3D segmentation where the lung boundary segmentation and the lesion detection are conducted in a single step using a deep convolutional network for semantic segmentation. Since it was difficult to detect smaller lesions, for this experiment, only the larger lesion types were considered such as the infiltrative and fibro-cavernous lesions. Here, a convolutional encoder-decoder model was used which trained on the 3D images to segment the lungs to obtain a region of interest. As input to the network, three layers of the 3D image were applied where the output of the network matched the 2D mask of the central layer. The result achieved using this technique for the lung segmentation was 0.95 of intersection of union (IoU) score. For the lesion detection task, a lower resolution of the 3D image was used. This technique was evaluated using the receiver operating characteristic (ROC) curve as the metric and achieved 0.775.
Baek et al. (2019) carried out an investigation to show the effect of deep segmentation networks have on the prediction of survival of non-small cell lung cancer. Two independent 3D U-Net models were trained to segment lung cancer in CT and PET scans, respectively where both models followed the standard encoder-decoder architecture. After evaluating each of the model's segmentation performance, the average DSC achieved by the U-Net for CT was 0.861 and for PET was 0.828. The architecture of a standard U-Net model is depicted in Figure 6.
Ozdemir et al. (2019) presented a system based entirely on 3D CNNs for both lung nodule detection and malignancy classification tasks on the LUNA16 dataset. The system consists of two components where the first is a computer-aided detection (CADe) module that detects and segments suspicious lung nodules and the second is a computer-aided diagnosis (CADx) module that performs both nodule detection and patient-level malignancy classification. The CADe system takes the patient's image as input and produces as output the detected lung nodules. The dataset used in this research was the LIDC-IDRI dataset with annotated ground truth data as well as the dataset provided by the National Cancer Institute for the 2017 Data Science Bowl on Kaggle. The CADe system is made up of a 3D segmentation network which produces a probability map of whether a voxel is nodule or not and a 3D scoring network which computes refined nodule probability estimates for full nodule candidates which are generated from the segmentation. The nodule segmentation network is a 3D fully CNN based on the V-Net architecture which uses three encoder-decoder block pairs with corresponding skip connections in addition to the input and output blocks. The encoder blocks are made up of two downsampling convolution layers, two layers of kernel size 3 convolutions, and residual connection to the output. The decoder blocks are the same but with two upsampling deconvolution layers. The innermost encoder-decoder block pair includes channel-wise dropout between the sampling convolution and the two main convolution layers. All the blocks in the network use instance normalization instead of batch normalization as well as RELU non-linearities. For training the network, the LUNA16 dataset was used. Since the CT scans are too large to train the network, 64 × 64 × 64 blocks near known nodules are extracted and used to train the network. The network is trained with a cross-entropy loss function that weights voxels within a nodule twice as much as the background voxels. When the test images are passed through the network, the images are split into 8 256 × 256 × 256 overlapping blocks and stitch the output segmentation together appropriately which then undergo post-processing to produce the candidate nodules.
Sasikala et al. (2019) proposed a CNN based technique to classify the lung tumors as malignant or benign from chest CT images. A back-propagation algorithm is used to train the Deep CNN to detect lung tumors in CT images of size 2 × 50 × 50 which consists of two phases. In the first phase, a CNN is used to extract valuable volumetric features from input data and the second phase is the classifier. The CNN consists of multiple volumetric convolution to the first phase and multiple fully connected layers and threshold layers followed by a Softmax layer to perform the high-level reasoning of the classification step. Once the CNN classifies whether the volume has a cancerous tumor or not, watershed segmentation is used to detect the cancer. The proposed method was evaluated and achieved a specificity of 1, sensitivity of 0.875, and overall accuracy of 0.96. The proposed system was able to detect the presence and absence of cancerous cells with a 96% accuracy rate.
The research done by Bansal et al. (2020) proposed a novel approach for detecting lesions using the inner structures of the nodule voxels. For the segmentation of the lung nodules, a fully convolutional network is used without pooling layers and is subdivided into two halves. The left half uses “down convolution” and performs compression, while the right half decompresses the signal back to its original dimensions. The network includes skip connections which go from each stage on the left half of the network to the corresponding stage on the right half of the network. Each stage in the architecture consists of three convolutional layers. The skip connections do not only go from left to right but from the first layer to the third layer within each stage on the left half of the network. The resulting feature map is converted into two probabilistic segments to produce a foreground and background using a softmax filter. To determine whether the voxel is foreground or background, a threshold of 0.4 is used. The segmentation network is based on the dice loss function. The input image is broken up into multiple batches of 128 x 128 x 64 voxel blocks, and the network input is a tensor of the form 16 × 128 × 128 × 64 × 1. The output including the probabilities of foreground and background has the same spatial dimensions as the input. After the segmentation step, the 2D slices are extracted from the 3D segmented results and are passed to a ResNet model for cancer classification. This study made use of the LUNA16 dataset where the test set consisted of 60 patients where 30 patients had cancerous nodules and 30 did not. To highlight the accuracy of the segmentation network, the dice coefficient metric is used where the model achieved a dice coefficient of 0.958.
Kamal et al. (2020) presented a Recurrent 3D-DenseUNet model for lung tumor segmentation. This model was inspired by the DenseNet, U-Net and convolutional Recurrent Network architectures and consists of three parts which are the encoder, recurrent block, and the decoder. The encoder block consists of 3D convolutional layers, a batch-normalization layer, ReLu activation layers, and 2D max pooling where each convolution block is designed to perform as a dense block to create a connection between the inner and middle layers of the block. The recurrent block is used as a transition section from the encoder to the decoder and consists of many convolutional long short-term memory (ConvLSTM) layers which is made up of 2D convolution layers. The final block is the decoder takes the features from the recurrent block, upsamples, and generates the predicted volumetric segmentation. The dataset used consists of 300 patients from the NSCLC-Radiomics dataset. An average of multiple prediction results are used as the final prediction. The proposed method was evaluated and achieved a mean dice coefficient of 0.7228 and a median dice coefficient of 0.7556. Figure 7 depicts the Recurrent 3D-DenseUNet architecture.
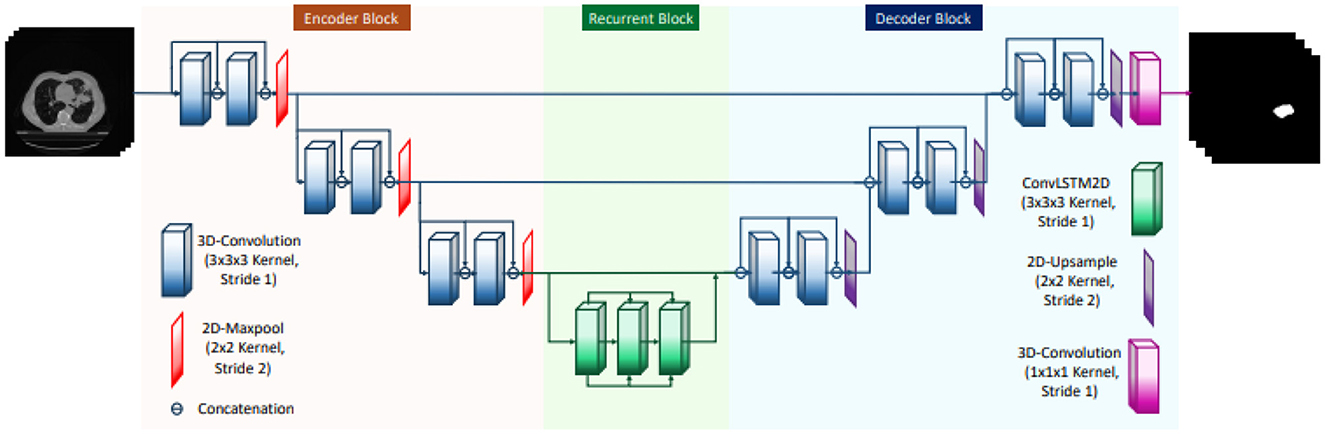
Figure 7. Recurrent 3D-DenseUNet proposed by Kamal et al. (2020).
Nishio et al. (2021) developed a pre-trained model for lung cancer segmentation using an artificial dataset generated by a GAN. This approach addresses the small dataset problem by creating synthetic images that resemble real lung cancer images. When fine-tuned on actual lung cancer datasets, the pre-trained model demonstrated improved segmentation accuracy. Three datasets were utilized: LUNA16, Decathlon lung dataset, and NSCLC radiogenomics. The LUNA16 dataset was used to generate the artificial dataset for segmentation with the help of the GAN and 3D graph cut techniques. The Decathlon lung dataset was employed to construct the main segmentation model from the pre-trained models. The NSCLC radiogenomics dataset was used to evaluate the performance of the main segmentation model. The artificial dataset generated by the GAN helped overcome the small dataset problem often encountered in medical imaging. Pre-trained models were constructed from this artificial dataset, and transfer learning was applied to fine-tune these models using the Decathlon lung dataset. The main segmentation model was then evaluated using the NSCLC radiogenomics dataset. The results showed that the mean DSC for the NSCLC radiogenomics dataset improved overall when using the pre-trained models, with a maximum increase of 0.09 compared to models without pre-training.
Borrelli et al. (2022) used a CNN to segment lung tumors and thoracic lymph nodes. The CNN model uses a U-Net 3D architecture where the final convolutional layer contains 3 channels with softmax activation, one for the background, one for the lung tumor, and one for the thoracic lymph node. The network accepts as separate inputs, the CT image, the PET image, and a one-hot encoded organ mask. The organ mask aids the network with a rough anatomical localization. It uses one channel each for bone, liver, lung, heart, aorta, and adrenal gland. The model was trained using patches of minimal size. The pixels were categorized into four groups namely, background, lung tumor, thoracic lymph node, and other abnormal uptake so the patches were chosen so that there was good balance between the different groups. The center point of each patch was randomly chosen. The model was trained 10,000 patches per epoch and then retrained with 20% of the patches focusing on incorrectly classified pixels and this step was repeated four times. The model's performance was evaluated using the Hazard Ratios. The CNN achieved an HR of 1.64 which was compared to a manual segmentation HR of 1.54.
Kasinathan et al. (2022) presented a strategy for classifying and validating different stages of lung tumor progression where a deep neural model and data collection using cloud system for categorizing phases of pulmonary illness. Included as part of their strategy, lung nodule segmentation is implemented prior to nodule classification was done using the active contour model (ACM). In the proposed ACM, the number of points to fit the curve is reduced in the tumor portion which is segmented. The modified ACM is evaluated using a gradient value which helps for edge detection. Using the Mumford-Shah model, an expression is derived for intensity outside and inside the curves with level set method and energy approximation. The resulting segmented tumors are then passed through a CNN model for classification. The effect of the ACM segmentation on the final classification results was evaluated. Without the use of the ACM tumor segmentation, the proposed method achieved an accuracy of 80%, specificity of 81%, and a sensitivity of 97% whereas with the application of the ACM tumor segmentation the method achieved an accuracy of 97.1%, a specificity of 93.9%, and a sensitivity of 95.9%.
A study for automated classification of lung cancer sub-types using deep learning and CT scan-based radiomic analysis was conducted by Dunn et al. (2023). In the study, the incremental multiple resolution residual network (iMRRN) was used for lung tumor segmentation. The dataset included 436 lung cancer CT images from the TCIA dataset. The CT scans were first analyzed, and then, a bounding box was used to manually select the tumor ROI. These 3D volumes were passed through the iMRRN for tumor segmentation; however, the results showed that the iMRRN was able to accurately segment only 44.7% of the images. The tumors in the other images were incorrectly placed outside the manually selected bounding box. The incorrectly segmented images were reprocessed limiting the processing to the ROI within the bounding box. This resulted in 92.1% of the remaining images being segmented correctly leaving a failure rate of 4.35%. The images that the model could not segment were removed from the dataset for further analysis in the remainder of the study. It was evident here that limiting the search space in an ROI improves the models performance. The model used in this study performs better with smaller tumors which could be a result of a limitation to the dataset and it relies on the manual delineation of the ROI.
In a study conducted by Said et al. (2023) for lung cancer diagnosis, a deep learning system was proposed where part of the system utilizes the UNETR architecture to segment tumors in the lung. The UNETR is a model that consists of a combination of U-Net and transformers to collect features from 3D images. It uses transformers, which operate using a 1D sequence, as the encoder to determine global multi-scale information and learn sequence representations in the data. The encoder and decoder are connected via skip connections in a contracting-expanding pattern using a stack of transformers. A dataset of 96 3D image volumes was used which were spilt into training and testing sets. The network optimizer was experimented with to determine the most accurate results. Using the AdamW optimizer yielded an accuracy rate of 96.79%, whereas the Nadam optimizer achieved an accuracy rate of 97.83%. The achieved results from this experiment were a dice of 96.42%, a sensitivity of 96.85%, and a specificity of 97.12% which are promising results; however, this architecture is computationally intensive and requires a high-performance specifications to run smoothly.
3.4.3 4D and hybrid methods
Chen et al. (2019) proposed a hybrid segmentation network (HSN) based on CNN where the model combines a lightweight 3D CNN and a 2D CNN to accurately segment small cell lung cancer from CT scans. A hybrid feature fusion model (HFFM) was also proposed to fuse the 2D and 3D features and jointly train the 2 CNNs. Figure 8 shows the structure of the hybrid model.
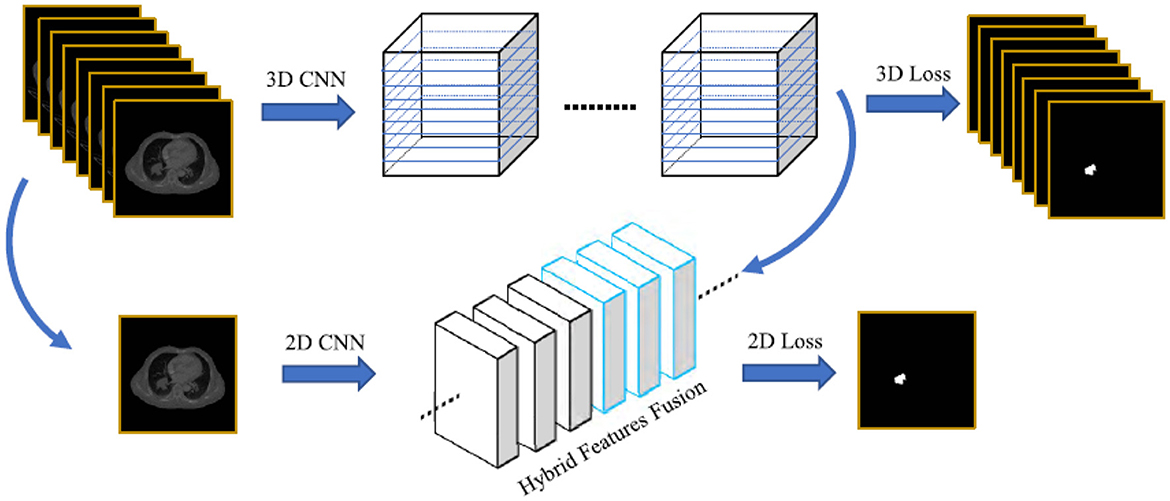
Figure 8. Structure of hybrid segmentation network with hybrid feature fusion model proposed by Chen et al. (2019).
The 3D CNN follows the standard structure of a U-Net architecture with encoder and decoder halves. The encoder begins with an Spatiotemporal-separable 3D (S3D) block, which consists of two consecutive convolutional layers where one is a 2D convolutional layer and the other is a 1D convolution to learn temporal features, and an multi-scale separable convolution (MSC) block followed by a few layers of MSC blocks where regular convolutional layers would be in a U-Net model. An MSC block is an Inception-ResNet-like architecture consisting of S3D convolutions to capture multi-scale 3D contextual information. Downsampling is done via the S3D convolution. The decoder half consists of layers of 1D convolution paired with MSC blocks and 3D bilinear upsampling. Each layer on the encoder half is concatenated to the corresponding layer on the decoder half. The CNN ends with a convolution and softmax layer. The 2D CNN incorporates the use of Dilated Unit Blocks (DUBs) which consists of two sequentially dilated convolutions with the residual connection. The 2D CNN starts with convolutions to produce 16 feature maps, and this is followed by alternating DUBs and striding S3D convolutions. Thereafter, upsampling, concatenation, and Squeeze-and-Excitation blocks are used to combine features. The HFFM model that is proposed to combine the features produced by the 3D and 2D CNNs, batches of adjacent slices from each CNN result are stacked, cropped, permuted so that they are of equal dimensions and concatenated. Thereafter, this is passed through a convolution layer, upsampling, further convolution and a softmax layer to produce the final segmentation. General dice loss is used to optimize the networks proposed. Data augmentation techniques were not utilized as focus was placed on the network structure. The network was quantitatively evaluated using DSC, sensitivity, and precision. The 2D CNN and 3D CNN were evaluated separately and compared to the results of the HSN. The 2D CNN achieved a mean DSC of 0.692, a mean sensitivity of 0.690, and mean precision of 0.766, the 3D CNN achieved a mean DSC of 0.840, a mean sensitivity of 0.830, and a mean precision of 0.856, and the HSN produced improved result with a mean DSC of 0.888, a mean sensitivity of 0.872, and a mean precision of 0.909. This proves that the HSN outperforms the 2D and 3D networks. This method does not include any pre-processing, ROI extraction, or post-processing techniques which could have improved the results achieved in this study.
In the investigation done by Barrett et al. (2021), the feasibility of a commercially available autocontouring system's performance in delineation of lung GTV was compared to that of manual GTV delineation. For this study, a unique 4DCT dataset of NSCLC patients was used. The manual contour was delineated by a single experienced radiation oncologist and was used as the ground truth data. For each 4DCT, a lung window was utilized to locate the approximate mid volume slice, and the auto contour was generated using the Smart Segmentation function on Varian Eclipse V15.5. Then, a user-adjusted auto contour was also generated. This was done by duplicating the auto contour and manually adjusting the contour which was done by an experienced radiation therapist. Three metrics were used to quantify the accuracy of the delineation methods, namely, DSC, shift in structure center of mass (COM), and volume difference in cm3. The results achieved by the autocontour method in comparison with the manual contour were a median DSC of 0.69, a volume difference of 3.35, and a COM offset of 0.39 and a mean DSC of 0.69, volume difference of 11.15, and COM offset of 0.49. The results achieved by the user-adjusted auto contour method were a median DSC of 0.8, volume difference of 1.4, and a COM offset of 0.2 and a mean DSC of 0.77, volume difference of 8.05, and a COM offset of 0.39. These results show that user intervention to correct the incorrect delineation of the auto contour system produced better results that the auto contouring system alone. One limitation of this study was that it did not include any node-positive cases where the target delineation is known to be more challenging.
Yan et al. (2022) proposed a deep learning model for lung CT image segmentation with the intention to improve the diagnosis rate of clinical lung cancer and improve the quality of life of patients after surgery. A hybrid segmentation model was proposed which includes a 2D CNN and a 3D CNN was constructed where the 3D model was used to obtain 3D information and the 2D model was used to obtain detailed semantic information. The hybrid feature fusion model (HFFM) was used to fuse the features effectively. The 3D model was structured similarly to the U-Net model with some improvements where the convolutions were replaced by a multi-scale separable convolution (MSC) module, a separable spatial 3D (S3D) was used in place of the pooling operation to reduce the size of the feature map, and lastly, the upsampling feature map was cascaded with the feature map produced by the encoder and the MSC was used to adjust the number of feature maps. The 2D model design included the adoption of the dilated convolution structure. The input image size was 512 × 512. The image was first decomposed into 16 feature maps using a convolution layer of 3 × 3 × 3 structure, and features were extracted using DUB with a stride convolution iteratively until the feature map was size 32 × 32. Thereafter, the feature maps were upsampled, followed by a cascading operation, and the Squeeze-and-Excitation and extrusion operations were adopted to build the dependency between the feature maps. This was followed by 2D convolutions layers, global pooling, Leaky ReLu layer, and a sigmoid function. The HFFM was constructed to fuse the feature maps produced by the two models. The cascaded feature map integrates the advantages of the two models while simultaneous training occurs. A convolution layer was added after fusion for optimization, and then, upsampling was applied. Finally, a softmax layer was used to segment the image. The accuracy of the lung cancer segmentation performed by the proposed model was measured using dice, sensitivity, and positive predicate value. The accuracy rate of the 2D CNN, 3D CNN and U-Net was also evaluated to compare with the proposed model. The dice value of the 2D CNN was 70%, the 3D CNN was 82%, the U-Net was 80%, and the proposed model was 87%. The sensitivity value of the 2D CNN was 70%, the 3D CNN was 82%, the U-Net was 81%, and the proposed model was 85% and the PPV of the 2D CNN was 78%, the 3D CNN was 83%, the U-Net was 79%, and the proposed model was 88%.
A novel method using GANs for 3D lung tumor reconstruction from CT images was proposed by Rezaei and Ahmadi (2023). The method involves three stages: lung segmentation, tumor segmentation, and 3D reconstruction. The first stage involves segmenting the lungs from CT images using snake optimization and Gustafson-Kessel (GK) clustering. The second stage focuses on segmenting the tumors within the lung regions. The final stage involves reconstructing the 3D model of the lung tumor. A Generative Adversarial Network (GAN) is used to create 3D shapes that closely match the ground truth. The generator produces 3D shapes from sequences of 2D images, while the discriminator distinguishes between real and generated images to help the generator improve. Features from the last unit of an LSTM network are fed into the generator, which then predicts a 3D image. These generated 3D images are compared with real 3D images by the discriminator to ensure accuracy and realism. Based on the HD and ED metrics, the proposed method achieves the lowest values, specifically 3.02 and 1.06, respectively. This approach enhances the visualization and analysis of lung tumors, aiding in better diagnosis and treatment planning.
To overcome the previously mentioned challenges, various image processing techniques and approaches have been explored; however, it is vital to note the advantages of deep learning techniques over non-deep learning techniques. Of late, deep learning techniques have showed great progress in medical imaging analysis and lung cancer detection (Wang et al., 2019). The success and improvement found from machine learning is not from improved hardware and more encompassing datasets but from innovations into model structure. From convolutions into fully connected layers, to the addition of dropout layers, to optimization techniques, the approach to deep learning is constantly changing and improving (Park and Monahan, 2019).
3.5 Post-processing
Post-processing is used to refine the segmentation results produced by the primary segmentation techniques. Often, there is a high number of inaccuracies which presents in the results of the initial segmentation which is alleviated with the application of appropriate post-processing techniques which allow for more clear and sharp results thereby increasing the accuracy rate of the methodology.
To reduce the number of false positives from the results obtained from the U-Net model proposed by Zhang et al. (2018), radiomic analysis was used where deep features of the tumor regions were used to aid a classifier to determine whether a segmented region is a tumor or not. Two different classification models were used to determine which model achieves more accurate tumor classification. Between AlexNet and ResNet-18, ResNet-18 achieved better results in reducing the number of false positives while maintaining the true positives. A 170 × 170 window is centered at the segmented region. The window size is based on the size of the largest tumor in the dataset allowing it to cover all the tumors in the dataset. Pairing their proposed U-Net using a threshold value of 0.5 with AlexNet achieved a dice coefficient of 0.611, mean surface distance of 8.137, 95% Hausdorff distance of 18.143, slice-wise missing rate of 30.3%, false-alarm rate of 11.1%, and CT scan-based accuracy of 85%. Pairing this U-Net with Res-Net-18 achieved a dice coefficient of 0.592, mean surface distance of 8.835, 95% Hausdorff distance of 21.176, slice-wise missing rate of 26.4%, false-alarm rate of 15.6%, and CT scan-based accuracy of 85%. Pairing their proposed U-Net using a threshold value of 0.0001 with AlexNet achieved a dice coefficient of 0.588, mean surface distance of 9.336, 95% Hausdorff distance of 21.243, slice-wise missing rate of 28.7%, false-alarm rate of 17.6%, and CT scan-based accuracy of 82.5%. Pairing this U-Net with Res-Net-18 achieved a dice coefficient of 0.563, mean surface distance of 10.896, 95% Hausdorff distance of 26.052, slice-wise missing rate of 23.3%, false-alarm rate of 24.6%, and CT scan-based accuracy of 90%. The results show that using only the U-Net model to distinguish tumors results in many false-alarms but when combined with the Res-Net or AlexNet the results produced are better. It is also evident that the combination of the U-Net and Res-Net-18 produced the most desirable results.
Following the nodule segmentation step proposed by Ozdemir et al. (2019), the nodule candidates are generated after undergoing some post-processing which includes thresholding the output voxel scores, applying the nearest neighbors binary opening filter, and then labeling all separate regions based on a voxel connectivity of one. For the false positive reduction, a scoring network was developed that operates on 32 × 32 × 32 blocks around the candidate center. The network architecture consists of three convolution blocks followed by a fully connected layer with PReLu, a dropout function, and lastly a fully connected layer. Each convolution block consists of a convolutional layer with PReLu, batch normalization, and a max pool layer. The network was trained with an SGD optimizer with batches of 16 candidates for 2,500 epochs with true nodule candidates weighted twice as much as false positive candidates. The CADe system was evaluated on the LUNA16 dataset and achieved a sensitivity of 0.921.
Meraj et al. (2021) utilized different morphological operations on the output results such as erosion and dilation to refine the segmentation results of the lung nodules and vessels. The results of the segmentation were then used for further processing where the candidate nodules were classified as benign or malignant. Kamal et al. (2020) also incorporated post-processing in the segmentation framework which includes thresholding of 0.7 and dilation using a 7 × 7 circular kernel. An example of the effect of morphological dilation as a post-processing technique is shown in Figure 9.
Post-processing methods are chosen based on what aspect of the initial results is to be improved to improve the accuracy rate. Simpler techniques such as morphological operations to more complex techniques such as further deep learning models have been experimented with to improve results. However, it is evident that not many authors have employed post-processing techniques to improve the results achieved.
4 Datasets
To train, validate, and test lung cancer detection and segmentation methods, lung cancer datasets need to be used. The datasets also need to have some reliable ground truth data included for evaluation of the methods. Some of the most common datasets used for lung cancer detection and segmentation are:
• The Cancer Imaging Archive (TCIA) is a free access database of medical images for cancer research. The site is funded by the National Cancer Institute Cancer Imaging Program and is administered by University of Arkansas for Medical Sciences (National Cancer Institute, 2024). The archive consists of collections of cancer-related datasets across different modalities including lung cancer CT databases.
• The Lung Image Database Consortium (LIDC) and Image Database Resource Initiative (IDRI) collected a database of lung cancer screening data for public availability. This dataset is part of the TCIA database. The database includes 1018 low-dose lung CT scans for a total of 1,010 patients (Armato et al., 2015) as well as the annotations from four experienced thoracic radiologists. Within the dataset, 7,371 lung cancer nodules were recorded where the main focus were nodules the size of 3 mm or greater.
• The National Lung Screening Trial (NLST) was a controlled trial conducted by the Lung Screening Study group (LSS) and the American College of Radiology Imaging Network (ACRIN). A database consists of low-dose computed tomography (CT) with chest radiography images from over 75,000 CT screening exams. Over 1,200 pathology images from a subset of 500 NLST lung cancer patients are available upon request (National Cancer Institute, 2017). This dataset is part of the TCIA image database.
• NSCLC-Radiogenomics is a collection of publicly available data with CT images for a group of 211 patients with non-small cell lung cancer (NSCLC). Also, NSCLC-Radiogenomics is the only general dataset consisting of paired information about the status of gene mutations associated with lung cancer (Bakr et al., 2018).
• LUNA16 (Lung Node Analysis) is a dataset for lung segmentation consisting of 1,186 lung nodes detailed in 888 CT scans (Setio et al., 2017). This dataset is a subset of the LIDC-IDRI dataset.
• The Lung Tissue Resource Consortium (LTRC) collated a database of volumetric high-resolution CT scans from 1,200 patients for lung cancer research.
• Data Science Bowl 2017 (DSB17) presented a dataset consisting of 2,102 low-dose CT scans for a lung cancer detection competition (Kaggle, 2017).
• Japanese Society of Radiological Technology (JSRT) Dataset, which includes 154 conventional chest radiographs with a lung nodule (100 malignant and 54 benign nodules) and 93 radiographs without a nodule (Japanese Society of Radiological Technology, 2024).
• ChestX-ray14 Dataset which contains over 100,000 frontal-view X-ray images annotated for various lung diseases (NIH Clinical Center, 2024).
• NIH Chest X-rays Dataset which is an extensive dataset includes over 100,000 images for detecting multiple thoracic diseases (NIH Clinical Center, 2024).
The LIDC-IDRI, LUNA16, and NLST datasets are the most commonly used for the detection and segmentation of lung tumors. These datasets are also the most suitable for detection and segmentation methods where deep learning techniques are utilized as they are large datasets with a high variability of tumors within the datasets. Tables 4, 5 show a summary of the most commonly used datasets.
5 Evaluation methods
The mechanism used to evaluate image segmentation techniques is an important aspect in estimating the fitness of a segmentation approach for its application. The evaluation of a technique is necessary to validate the performance on the data and allows for it to be compared to other approaches. Here are some of the most common evaluation measures used to determine the accuracy of a method developed for lung tumor segmentation.
Accuracy is described as the amount of true positive (TP) classified examples and true negative (TN) classified examples relative to the total number of cases.
Recall is the number of TPs relative to all true positive cases, including missed examples, which are called false negatives (FN).
Precision is defined as the number of true positives divided by the number of true positives plus the number of false positives.
Sensitivity is also called the true positive rate (TPR) and is the proportion of samples that are genuinely positive that give a positive result.
Specificity is also referred to as the true negative rate (TNR) and is the proportion of samples that are genuinely negative that give a negative result.
The dice similarity coefficient measures the similarity and overlap between the ground truth B segmentation and the segmentation results A achieved. The performance index ranges between zero and one with an index zero signifying no overlap between B and A, while index one signifies a perfect overlap between them.
6 Discussion
All categories of lung cancer detection and segmentation approaches and methods have their own advantages and drawbacks and may be particularly effective for a particular case. In this section, the advantages and limitations of the various methods presented are discussed as well as the approaches with significant results.
A common structure of lung segmentation frameworks is pre-processing, region of interest extraction, lung cancer detection or segmentation, and lastly post-processing. Each of these phases in the process plays a role which contributes to the results achieved by the framework. However, it is observed that many of the works presented that implement machine learning techniques have omitted the utilization of some of these phases such as pre-processing, region of interest extraction, or post-processing (Angeline et al., 2022; Serj et al., 2018; Cui et al., 2022; Shimazaki et al., 2022; Yan et al., 2022; Ismail, 2021; Chen et al., 2019). The drawback here is that in most cases, the addition of these phases contributes to the improvement of the overall accuracy of the methodology but they have not been used.
An interesting aspect that is to be considered when using machine learning techniques is the dataset that is used for training and testing. Each dataset comes with its own specifications such as the modality used to produce the image, the type of image produced, dimensions, as well as the quality. Different datasets also come with inter-patient and inter-image variability which affect the training as well as the capabilities of the model that is trained. The size of that dataset also impacts the training of a model as the larger the dataset the more data the model has to train on allowing it to become more accurate in its capability. The information within the images fed to the model also plays a vital role in the model's ability to detect and segment the lung tumors. Another interesting factor is the size bias within different datasets which may be due to a selection bias during data collection (Kadir and Gleeson, 2018). If a dataset only contains large tumors, the model will find it challenging to detect smaller tumors or nodules and may miss them completely. The use if limited datasets for training and testing increases the possibility for model over-fitting. Hence, efficient generalization of proposed algorithms cannot be demonstrated. The application of data augmentation may alleviate this. Currently, the amount of data available with high-quality ground truth is not adequate. With time, data will accumulate and deep learning models can be retrained and fine-tuned to avoid “forgetting” during fine-tuning processes with newer data (Liu et al., 2021).
Network design is yet another consideration. Approaches where the algorithms include a fusion of features or models aided in overcoming the drawbacks of one feature by the advantage of another which increased the accuracy. With increasing numbers of layers in a deep learning network, the deep learning algorithm becomes smarter making predictions easier and more accurate. However, as the network complexity increases, the time taken to train the network as well as memory consumed during the training also increases (Liu et al., 2021).
Although certain systems lack adequate detection accuracy, many systems have been developed with the objective to reach a 100% accuracy rate for lung cancer detection and segmentation (Abdullah et al., 2021). An advantage of such systems and future innovations in the field is that they can do the heavy lifting of screening a large number of possible lung cancer patients which aids in early diagnosis and treatment due to the increased automation and without imposing a burden on radiologists. Apart from the improvement of accuracy rate, the application of these AI systems is yet another issue. Although the aforementioned studies show promising results in the application of AI to lung cancer detection and segmentation, real implementation of the workflow is rare. Aspects such as user interfaces, speed of data analysis, expanse of the programs, the infrastructure they require, and the resources they consume are all barriers of application in the real world (Chiu et al., 2022).
Table 6 presents the approaches that produced some of the most significant results. It is also evident that these approaches include some element of deep learning in their methods. The lung cancer detection method proposed by Madan et al. (2019) incorporated a CNN which produced the segmented image after a smoothing filter and data augmentation was applied as pre-processing methods. This model achieved a validation accuracy of 93%. Sasikala et al. (2019) proposed a CNN-based technique to classify the lung tumors as malignant or benign from chest CT images. A back-propagation algorithm is used to train the deep CNN to detect lung tumors in two phases where in the first phase a CNN is used to extract valuable volumetric features from input data and the second phase is the classifier. A smoothing filter was applied as a pre-processing method prior to segmentation, and thereafter, the CNN classifies whether the volume has a cancerous tumor or not. Finally, watershed segmentation is used to detect the cancer. The proposed method achieved a 96% accuracy rate. Park and Monahan (2019) carried out an investigation using a genetic algorithm to conduct a neural architectural search to generate a modified evolved novel CNN named DeepNEAT-Dx to detect lung cancer in chest X-rays. The NEAT algorithm is modified to evolve a CNN's architecture which is named DeepNEAT-Dx. The state-of-the art lung cancer segmentation model, DeepNEAT-Dx, produced an accuracy rate of 97.15%. Bansal et al. (2020) proposed a novel approach for detecting lesions using the inner structures of the nodule voxels. A full convolutional network is used for the segmentation of the nodules where skip connections are included in the network. After the segmentation step, 2D slices are extracted from the 3D segmented results and are passed to a ResNet model for cancer classification. The model achieved a dice coefficient of 0.958. Kasinathan et al. (2022) presented a strategy for classifying and validating different stages of lung tumor progression where lung nodule segmentation is implemented using the active contour model (ACM). The effect of the ACM segmentation with a deep learning method was evaluated which resulted in an accuracy rate of 97.1%. Said et al. (2023) proposed an architecture that utilized the UNETR architecture; however, during the ablation study that was conducted, it was discovered that using the Nadam optimizer to train their model improved their accuracy rate from 96.79% to 97.83% proving that optimizers also influence the performance of the model used for segmentation.
Furthermore, there are some major observations that are evident in current research:
• The incorporation of pre-processing, region of interest extract, and post-processing within the methodology supports the improvement of the results produced by the segmentation phase.
• The dataset used in the research plays a vital role in choosing the methods used to achieve the most accurate results.
• Data augmentation is the most commonly used pre-processing technique which allows for increasing the variability and diversity of the data in the dataset, therefore enabling the model trained on the dataset to be more robust, improve generalization, and reduce over-fitting.
• The use of techniques that localize the search space to detect or segment the lung tumor has a high impact on the results achieved by the detection or segmentation result.
• Deep learning techniques are not only used in the feature extraction (detection and segmentation) phase in the presented methodologies but also in the region of interest localization and post-processing phases.
• The use of hybrid methods or a combination of different deep learning techniques has been used in majority of the presented methodologies. Many of the methodologies consisting of combinations of deep learning models achieved high accuracy rates.
Generative models can enhance training data by creating synthetic data to augment training datasets, addressing issues of data scarcity and imbalance. Pre-trained models on synthetic data can be fine-tuned on real data, leading to better segmentation performance and improved segmentation accuracy. Using generative models for 3D reconstruction of lung tumors provides detailed insights, aiding in precise diagnosis and treatment planning. Generative deep learning models offer several advantages in lung nodule segmentation, making them a valuable tool in medical imaging. Generative models can produce high-quality, realistic images that help in accurately identifying and segmenting lung nodules. This leads to better detection rates and fewer false positives. By producing synthetic data, which is especially beneficial in medical imaging due to the scarcity of annotated datasets, generative models help train more robust models through data augmentation. These models can be fine-tuned for specific tasks or adapted to new datasets with minimal additional training, increasing their versatility across various clinical settings (Gao et al., 2024). Generative models can minimize variability in segmentation outcomes, ensuring consistent performance across various datasets and imaging conditions. Once trained, these models can be effortlessly scaled to handle large volumes of medical images, making them ideal for widespread clinical applications. In addition, automated segmentation with generative models reduces the risk of human error, which is often encountered in manual segmentation. A significant benefit of generative models is their ability to enhance feature learning. By generating images, these models can identify complex patterns and features that traditional models might overlook. This deep comprehension of the data results in more precise segmentation (Yang et al., 2016). There are some limitations to the use of generative models such as data dependency and computational cost. While GANs can generate synthetic data to augment training datasets, the quality and diversity of synthetic data may not fully capture the complexity of real-world medical images (Nishio et al., 2021). Training GANs, especially 3D models with attention mechanisms, requires significant computational resources, including powerful GPUs and large memory capacities. This can be a barrier for institutions with limited resources. Another disadvantage of generative models is the complexity of model architectures. In the study presented by Dabass et al. (2023), the inclusion of attention learning modules and the 3D nature of the model add to its complexity. This can make the model harder to interpret and debug, posing challenges for clinical adoption. Incorporating these advanced models into current clinical workflows can be difficult. It necessitates smooth integration with hospital information systems and radiology processes. In addition, securing regulatory approval for the clinical application of AI models is a stringent process that can postpone their implementation (XenonStack, 2023).
Different deep learning methods can perform variably depending on the type of imaging data used for lung nodule segmentation. CNNs are widely used for lung nodule segmentation in CT scans due to their ability to capture spatial hierarchies in the data. Studies have shown that models such as U-Net and its variants perform exceptionally well, achieving high accuracy and Dice coefficients. 3D CNN models are particularly effective for volumetric data such as CT scans. They can capture the 3D context of nodules, which is crucial for accurate segmentation (Gao et al., 2024). A study by Astley et al. (2022) evaluated several 3D CNNs for segmenting ventilated lung regions on hyperpolarized gas MRI scans. The study found that the 3D nn-U-Net outperformed other deep learning methods and conventional segmentation techniques, achieving high accuracy and robust segmentation. Hybrid models where CNNs are combined with other techniques, such as recurrent neural networks (RNNs) or attention mechanisms, can improve performance on MRI data by capturing both spatial and temporal features (Thanoon et al., 2023). CNNs are also effective for X-ray images, particularly for detecting and classifying lung nodules. Using transfer learning where pre-trained models on large datasets and fine-tuning them on specific X-ray datasets can enhance performance. This approach leverages the broad knowledge captured in the pre-trained model. A study by Chavan et al. (2022) compared various deep learning models, including U-Net, ResUNet, FCN, SegNet, and ResUNet++, for lung image segmentation on chest X-rays. The study found that CNN-based models, particularly those using transfer learning, achieved high accuracy and outperformed traditional methods. These findings underscore the importance of choosing the right deep learning method based on the specific imaging modality and the characteristics of the data.
Generalizing lung cancer detection using deep learning models is a complex challenge, especially when dealing with instances that differ from the training data. A study by Javed et al. (2024) highlighted that deep learning models, particularly CNNs, can achieve high accuracy in lung cancer detection. However, it emphasizes the importance of diverse training data to improve generalization. The more diverse the training dataset, the better the model can generalize to new, unseen data. This includes variations in patient demographics, nodule types, imaging protocols, and disease stages.Data augmentation techniques can also help create a more robust model by simulating a wider range of possible scenarios which can improve the model's ability to handle variations not present in the original training data. Transfer learning also enhances generalization as presented by Kumar et al. (2024) in a study where it was shown that combining models such as ResNet-50, EfficientNet-B3, and ResNet-101 with transfer learning, there was an improvement of generalization. Regularization techniques such as dropout, weight decay, and batch normalization are widely recognized for their ability to prevent over-fitting and improve generalization to new data (Analytics Vidhya, 2024). Cross-validation techniques such as k-fold and leave-one-out are also crucial techniques for assessing the generalization capability of machine learning models. It involves creating multiple subsets of datasets and iteratively training and evaluating models on different training and testing datasets. This ensures consistent performance across different data subsets (Stack Abuse, 2023). In a study done by Ganaie et al. (2022), it was emphasized that ensemble methods, such as bagging, boosting, and stacking, which involves combining predictions from multiple models can reduce the risk of errors from any single model, leading to more robust and generalized predictions (Ganaie et al., 2022). Despite these strategies, there are inherent limitations. Deep learning models can struggle with out-of-distribution samples-instances that are significantly different from the training data. Continuous updates and retraining with new data are essential to maintain and improve the model's performance.
Deep learning techniques have indeed made significant contributions to lung cancer detection and segmentation, particularly in terms of their efficacy in identifying true positives (TP), false positives (FP), true negatives (TN), and false negatives (FN). DCNNs have shown high sensitivity in detecting lung nodules, leading to a high number of true positives. Studies using U-Net and its variants have demonstrated excellent performance in accurately identifying cancerous nodules (Javed et al., 2024). Particularly effective for volumetric data such as CT scans, 3D CNNs can capture the 3D context of nodules, improving TP rates (Gayap and Akhloufi, 2024). Methods such as false positive reduction, which involve additional filtering steps or secondary models, help in reducing the number of false positives. Incorporating clinical knowledge and rule-based systems can further enhance accuracy. Ensemble methods can also reduce the risk of false positives by averaging out errors from individual models (UrRehman et al., 2024). Regularization techniques can help in preventing over-fitting, ensuring that the model correctly identifies non-cancerous cases, thus increasing the number of true negatives (Thanoon et al., 2023). Employing cross-validation techniques ensures that the model's performance is consistent across different subsets of the data, indicating better generalization and accurate TN identification (Wang, 2022). By artificially increasing the diversity of the training data using data augmentation, models can become more robust and less likely to miss cancerous nodules, thereby reducing false negatives (Gayap and Akhloufi, 2024). Using pre-trained models on large, diverse datasets and fine-tuning them on specific lung cancer datasets can enhance the model's ability to detect subtle features and therefore reduce FN rates.
Many of these deep learning methods are available for users through open-source platforms and research collaborations. For instance, pre-trained models and code repositories are often shared on platforms such as GitHub, enabling researchers and clinicians to utilize and build upon existing study. This allows for further research into lung nodule detection and segmentation using techniques such as transfer learning, model adaptability, result reproducibility, and practical monitoring. Transfer learning leverages the knowledge obtained by pre-trained models. This can also significantly reduce training time and improve performance by utilizing knowledge from large, diverse datasets. Studies have shown that transfer learning can improve the accuracy of lung cancer segmentation models by providing a strong initial model that can be fine-tuned for specific tasks (Saha et al., 2024). However, models trained on general datasets may not perform well on specialized medical data due to domain shift (Nishio et al., 2021). Fine-tuning and adapting models to new data is crucial for maintaining high performance across different datasets. Models that can adapt to new data are more robust and can be used in a variety of clinical settings. These models are more flexible and scalable in that they can handle different types of medical imaging data, improving their utilization across various applications. The challenge here is that often new data have different characteristics compared to the training data making generalization difficult. This also makes the integration of such models into existing clinical workflows significantly challenging (Kumaran et al., 2024). Fine-tuning techniques allow models to learn specific features of the target dataset, improving their performance on specific tasks which enables the customization of models for specific clinical needs, making them more relevant and effective. However, over-fitting can occur on small datasets (Davila et al., 2024). Ensuring reproducibility of results is essential for the reliable deployment of deep learning models in clinical settings and allows for better validation and comparison of different models, leading to continuous improvement. Nonetheless, difficulties regarding variability and transparency may arise where there are differences in data collection, pre-processing, and model training which can lead to variability in results and lack of transparency in model development and training processes, making reproducibility difficult (Javed et al., 2024).
While deep learning models have shown great promise in research settings, their deployment in clinical practice is still in the early stages. To ensure that deep learning technologies enhance rather than disrupt existing practices, it is crucial to address challenges related to generalization across datasets, interoperability, standardization, and technology adaptation. In addition, developing models that easily integrate useful features and apply segmentation in clinical practice highlights the evolving landscape of lung cancer diagnosis. The convergence of deep learning with traditional medical practices holds significant promise but requires coordinated efforts in workflow design, infrastructure planning, training, and policy development (Gayap and Akhloufi, 2024). There are ongoing studies monitoring the efficacy of these methods in practical environments. There is need for rigorous validation of deep learning models in clinical settings to ensure their reliability and effectiveness. Some of the current literature has limited applicability in clinical practice because non-medical investigators often lack experience in selecting relevant clinical outcomes (Wang, 2022). Many deep learning techniques have been developed by non-medical professionals with minimal input from radiologists, who will ultimately be the end users of these resources once they become more widely available. Clinicians have also noted that adopting certain clinical models is challenging because they require complex information from multiple sources. The absence of this information hinders the practical application of these models (Park and Lee, 2022).
Different types of techniques have been proposed over the last decade where many included the application of machine learning techniques such as supervised learning, unsupervised learning, and reinforcement learning (Abdullah et al., 2021). Other techniques such as structure-based or texture-based techniques were used, but it was evident in the results achieved that machine learning techniques generally outperformed other techniques with regard to segmentation accuracy. To overcome the previously mentioned challenges, various image processing techniques and approaches have been explored; however, it is vital to note the advantages of machine learning techniques over non-machine learning techniques. Of late, machine learning techniques have showed great progress in medical imaging analysis and lung cancer detection (Wang et al., 2019). The success and improvement found from machine learning is not from improved hardware and more encompassing datasets, but from innovations into model structure. From convolutions into fully connected layers, to the addition of dropout layers, to optimization techniques, the approach to deep learning is constantly changing and improving (Park and Monahan, 2019).
7 Conclusion
This literary study provides a discussion and evaluation of current lung nodule segmentation approaches. This analysis provides a comprehensive overview of lung nodule segmentation strategies to identify and extract nodules for further analysis. Lung nodule detection and segmentation techniques have definitely improved over the past decade. However, there is still room for improvement. There are still issues to be resolved with respect to developing better techniques, improving contrast enhancement as well as selecting better criteria for evaluating the performance of proposed frameworks.
There are a number of interesting future research areas of focus in lung tumor segmentation:
• Although there have been various efforts toward achieving a high accuracy in lung tumor segmentation, the results are not quite good enough for it to be applicable in the medical field with the existing challenges.
• Deep learning models are most often trained on a single dataset which limits the ability of the model performance. Training models on many datasets can be explored to produce more accurate results.
• Studying pathological patterns in the differences of lung tumors should be explored to be utilized to aid in the refinement of lung tumor segmentation and classification.
• Furthermore, there have been a variety of research done on the detection and segmentation of non-small cell lung cancer tumors (NSCLC) but not enough done on small cell lung cancer (SCLC) which is a far more aggressive type of cancer which will be a research focus area with great value for the future.
Machine learning techniques including supervised, unsupervised, and reinforcement learning as well as combinations of these are among the most effective techniques proposed for lung nodule detection and segmentation and a comparative analysis of these techniques has been presented. An understanding of the current approaches serves to provide a guide for choosing methods and techniques for future research studies.
Author contributions
AH: Conceptualization, Formal analysis, Writing – review & editing, Writing – original draft. SV: Conceptualization, Formal analysis, Writing – review & editing, Supervision. MG: Conceptualization, Supervision, Writing – review & editing.
Funding
The author(s) declare that no financial support was received for the research, authorship, and/or publication of this article.
Conflict of interest
The authors declare that the research was conducted in the absence of any commercial or financial relationships that could be construed as a potential conflict of interest.
Publisher's note
All claims expressed in this article are solely those of the authors and do not necessarily represent those of their affiliated organizations, or those of the publisher, the editors and the reviewers. Any product that may be evaluated in this article, or claim that may be made by its manufacturer, is not guaranteed or endorsed by the publisher.
References
Abdullah, D. M., and Ahmed, N. S. (2021). A review of most recent lung cancer detection techniques using machine learning. Int. J. Sci. Bus. 5, 159–173.
Akter, O., Moni, M. A., Islam, M. M., Quinn, J. M., and Kamal, A. (2021). Lung cancer detection using enhanced segmentation accuracy. Appl. Intell. 51, 3391–3404. doi: 10.1007/s10489-020-02046-y
American Cancer Society (2022). What is lung cancer. Available at: https://www.cancer.org/cancer/lung-cancer/about/what-is.html (accessed April 26, 2022).
Analytics Vidhya (2024). Regularization in deep learning with python code. Available at: https://www.analyticsvidhya.com/blog/2018/04/fundamentals-deep-learning-regularization-techniques/ (accessed August 28, 2024).
Angeline, R., Kanna, S. N., Menon, N. G., and Ashwath, B. (2022). “Identifying malignancy of lung cancer using deep learning concepts,” in Artificial intelligence in healthcare, 35–46. doi: 10.1007/978-981-16-6265-2_3
Armato, S. G., McLennan, G., Bidaut, L., McNitt-Gray, M. F., Meyer, C. R., Reeves, A. P., et al. (2015). Data from the lung image database consortium (LIDC) and image database resource initiative (IDRI): a completed reference database of lung nodules on ct scans (LIDC-IDRI). Available at: https://wiki.cancerimagingarchive.net/pages/viewpage.action?pageId=1966254 (accessed June 30, 2024).
Astley, J. R., Biancardi, A. M., Hughes, P. J., Marshall, H., Smith, L. J., Collier, G. J., et al. (2022). Large-scale investigation of deep learning approaches for ventilated lung segmentation using multi-nuclear hyperpolarized gas MRI. Sci. Rep. 12:10566. doi: 10.1038/s41598-022-14672-2
Baek, S., He, Y., Allen, B. G., Buatti, J. M., Smith, B. J., Tong, L., et al. (2019). Deep segmentation networks predict survival of non-small cell lung cancer. Sci. Rep. 9:17286. doi: 10.1038/s41598-019-53461-2
Bakr, S., Gevaert, O., Echegaray, S., Ayers, K., Zhou, M., Shafiq, M., et al. (2018). A radiogenomic dataset of non-small cell lung cancer. Sci. Data 5, 1–9. doi: 10.1038/sdata.2018.202
Bansal, G., Chamola, V., Narang, P., Kumar, S., and Raman, S. (2020). Deep3Dscan: deep residual network and morphological descriptor based framework for lung cancer classification and 3D segmentation. IET Image Process. 14, 1240–1247. doi: 10.1049/iet-ipr.2019.1164
Barrett, S., Simpkin, A., Walls, G., Leech, M., and Marignol, L. (2021). Geometric and dosimetric evaluation of a commercially available auto-segmentation tool for gross tumour volume delineation in locally advanced non-small cell lung cancer: a feasibility study. Clin. Oncol. 33, 155–162. doi: 10.1016/j.clon.2020.07.019
Bhaskar, N., and Ganashree, T. (2020). “Lung cancer detection with fpcm and watershed segmentation algorithms,” in Advances in Decision Sciences, Image Processing, Security and Computer Vision: International Conference on Emerging Trends in Engineering (ICETE) (Springer), 687–695. doi: 10.1007/978-3-030-24322-7_81
Bhatia, S., Sinha, Y., and Goel, L. (2019). “Lung cancer detection: a deep learning approach,” in Soft Computing for Problem Solving: SocProS 2017 (Springer), 699–705. doi: 10.1007/978-981-13-1595-4_55
Borrelli, P., Góngora, J. L. L., Kaboteh, R., Ulén, J., Enqvist, O., Trägårdh, E., et al. (2022). Freely available convolutional neural network-based quantification of pet/CT lesions is associated with survival in patients with lung cancer. EJNMMI Phys. 9:6. doi: 10.1186/s40658-022-00437-3
Bushra, K. A., Lasrado, S., and Prasad, K. (2019). Detection of lung cancer by modified irregular tree structure Bayesian network model based image segmentation. Mater. Today 11, 1130–1138. doi: 10.1016/j.matpr.2018.12.047
Cai, J., Zhu, H., Liu, S., Qi, Y., and Chen, R. (2024). Lung image segmentation via generative adversarial networks. Front. Physiol. 15:1408832. doi: 10.3389/fphys.2024.1408832
Centers for Disease Control and Prevention (2022). What is lung cancer? Available at: https://www.cdc.gov/cancer/lung/basic_info/what-is-lung-cancer.htm (accessed April 27, 2022).
Chavan, M., Varadarajan, V., Gite, S., and Kotecha, K. (2022). Deep neural network for lung image segmentation on chest X-ray. Technologies 10:105. doi: 10.3390/technologies10050105
Chen, W., Wei, H., Peng, S., Sun, J., Qiao, X., and Liu, B. (2019). HSN: hybrid segmentation network for small cell lung cancer segmentation. IEEE Access 7, 75591–75603. doi: 10.1109/ACCESS.2019.2921434
Chiu, H.-Y., Chao, H.-S., and Chen, Y.-M. (2022). Application of artificial intelligence in lung cancer. Cancers 14:1370. doi: 10.3390/cancers14061370
Christe, A., Peters, A. A., Drakopoulos, D., Heverhagen, J. T., Geiser, T., Stathopoulou, T., et al. (2019). Computer-aided diagnosis of pulmonary fibrosis using deep learning and CT images. Invest. Radiol. 54:627. doi: 10.1097/RLI.0000000000000574
Cui, X., Zheng, S., Heuvelmans, M. A., Du, Y., Sidorenkov, G., Fan, S., et al. (2022). Performance of a deep learning-based lung nodule detection system as an alternative reader in a Chinese lung cancer screening program. Eur. J. Radiol. 146:110068. doi: 10.1016/j.ejrad.2021.110068
Dabass, M., Chandalia, A., Datta, S., and Mahapatra, D. (2023). “Ale-GAN: a 3D conditional generative adversarial network with attention learning modules for lung nodule segmentation,” in International Conference on Advances in Data-driven Computing and Intelligent Systems (Springer), 321–332. doi: 10.1007/978-981-99-9531-8_26
Davila, A., Colan, J., and Hasegawa, Y. (2024). Comparison of fine-tuning strategies for transfer learning in medical image classification. Image Vis. Comput. 146:105012. doi: 10.1016/j.imavis.2024.105012
Dong, X., Xu, S., Liu, Y., Wang, A., Saripan, M. I., Li, L., et al. (2020). Multi-view secondary input collaborative deep learning for lung nodule 3D segmentation. Cancer Imag. 20, 1–13. doi: 10.1186/s40644-020-00331-0
Dunn, B., Pierobon, M., and Wei, Q. (2023). Automated classification of lung cancer subtypes using deep learning and CT-scan based radiomic analysis. Bioengineering 10:690. doi: 10.3390/bioengineering10060690
Ganaie, M. A., Hu, M., Malik, A. K., Tanveer, M., and Suganthan, P. N. (2022). Ensemble deep learning: a review. Eng. Appl. Artif. Intell. 115:105151. doi: 10.1016/j.engappai.2022.105151
Gao, C., Wu, L., Wu, W., Huang, Y., Wang, X., Sun, Z., et al. (2024). Deep learning in pulmonary nodule detection and segmentation: a systematic review. Eur. Radiol. 2024, 1–12. doi: 10.1007/s00330-024-10907-0
Gayap, H. T., and Akhloufi, M. A. (2024). Deep machine learning for medical diagnosis, application to lung cancer detection: a review. BioMedInformatics 4, 236–284. doi: 10.3390/biomedinformatics4010015
Gunasekaran, K. P. (2023). Leveraging object detection for the identification of lung cancer. arXiv preprint arXiv:2305.15813.
Halder, A., Chatterjee, S., and Dey, D. (2020). “Superpixel and density based region segmentation algorithm for lung nodule detection,” in 2020 IEEE Calcutta Conference (CALCON) (IEEE), 511–515. doi: 10.1109/CALCON49167.2020.9106569
Ismail, M. B. S. (2021). Lung cancer detection and classification using machine learning algorithm. Turkish J. Comput. Mathem. Educ. 12, 7048–7054.
Japanese Society of Radiological Technology (2024). Digital image database. Available at: http://db.jsrt.or.jp/eng.php (accessed September 6, 2024).
Javed, R., Abbas, T., Khan, A. H., Daud, A., Bukhari, A., and Alharbey, R. (2024). Deep learning for lungs cancer detection: a review. Artif. Intell. Rev. 57:197. doi: 10.1007/s10462-024-10807-1
Joon, P., Bajaj, S. B., and Jatain, A. (2019). “Segmentation and detection of lung cancer using image processing and clustering techniques,” in Progress in Advanced Computing and Intelligent Engineering: Proceedings of ICACIE 2017 (Springer), 13–23. doi: 10.1007/978-981-13-1708-8_2
Kadir, T., and Gleeson, F. (2018). Lung cancer prediction using machine learning and advanced imaging techniques. Transl. Lung Cancer Res. 7:304. doi: 10.21037/tlcr.2018.05.15
Kaggle (2017). Data science bowl 2017. Available at: https://www.kaggle.com/datasets (accessed June 30, 2024).
Kalaivani, N., Manimaran, N., Sophia, S., and Devi, D. (2020). “Deep learning based lung cancer detection and classification,” in IOP Conference Series: Materials Science and Engineering (IOP Publishing), 012026. doi: 10.1088/1757-899X/994/1/012026
Kalinovsky, A., Liauchuk, V., and Tarasau, A. (2017). Lesion detection in ct images using deep learning semantic segmentation technique. Int. Arch. Photogram. Rem. Sens. Spatial Inf. Sci. 42:13. doi: 10.5194/isprs-archives-XLII-2-W4-13-2017
Kamal, U., Rafi, A. M., Hoque, R., Wu, J., and Hasan, M. K. (2020). “Lung cancer tumor region segmentation using recurrent 3d-denseunet,” in Thoracic Image Analysis: Second International Workshop, TIA 2020, Held in Conjunction with MICCAI 2020, Lima, Peru, October 8, 2020, Proceedings 2 (Springer), 36–47. doi: 10.1007/978-3-030-62469-9_4
Kasinathan, G., and Jayakumar, S. (2022). Cloud-based lung tumor detection and stage classification using deep learning techniques. Biomed Res. Int. 2022:4185835. doi: 10.1155/2022/4185835
Kumar, V., Prabha, C., Sharma, P., Mittal, N., Askar, S. S., and Abouhawwash, M. (2024). Unified deep learning models for enhanced lung cancer prediction with resnet-50-101 and efficientnet-b3 using DICOM images. BMC Med. Imaging 24:63. doi: 10.1186/s12880-024-01241-4
Kumaran, S. Y, Jeya, J. J., Khan, S. B., Alzahrani, S., and Alojail, M. (2024). Explainable lung cancer classification with ensemble transfer learning of vgg16, resnet50 and inceptionv3 using grad-cam. BMC Med. Imaging 24:176. doi: 10.1186/s12880-024-01345-x
Liu, M., Dong, J., Dong, X., Yu, H., and Qi, L. (2018). “Segmentation of lung nodule in CT images based on mask R-CNN,” in 2018 9th International Conference on Awareness Science and Technology (iCAST) (IEEE), 1–6. doi: 10.1109/ICAwST.2018.8517248
Liu, X., Li, K.-W., Yang, R., and Geng, L.-S. (2021). Review of deep learning based automatic segmentation for lung cancer radiotherapy. Front. Oncol. 11:717039. doi: 10.3389/fonc.2021.717039
Madan, B., Panchal, A., and Chavan, D. (2019). “Lung cancer detection using deep learning,” in 2nd international Conference on Advances in Science &Technology (ICAST). doi: 10.2139/ssrn.3370783
Mahersia, H., Zaroug, M., and Gabralla, L. (2015). Lung cancer detection on ct scan images: a review on the analysis techniques. Lung Cancer 4:10-14569. doi: 10.14569/IJARAI.2015.040406
Manoharan, S. (2020). Early diagnosis of lung cancer with probability of malignancy calculation and automatic segmentation of lung ct scan images. J. Innov. Image Proc. 2, 175–186. doi: 10.36548/jiip.2020.4.002
Mayo Clinic (2022). Lung cancer - diagnosis and treatment. Available at: https://www.mayoclinic.org/diseases-conditions/lung-cancer/diagnosis-treatment/drc-20374627#::text=An%20X%2Dray%20image%20of,presence%20of%20lung%20cancer%20cells (accessed April 27, 2022).
Medical News Today (2022). Lung cancer: everything you need to know. Available at: https://www.medicalnewstoday.com/articles/323701 (accessed April 26, 2022).
Meraj, T., Rauf, H. T., Zahoor, S., Hassan, A., Lali, M. I., Ali, L., et al. (2021). Lung nodules detection using semantic segmentation and classification with optimal features. Neural Comput. Applic. 33, 10737–10750. doi: 10.1007/s00521-020-04870-2
National Cancer Institute (2017). Cancer data access system: National lung screening trial. Available at: https://cdas.cancer.gov/nlst/ (accessed June 30, 2024).
National Cancer Institute (2024). The cancer imaging archive. Available at: https://www.cancerimagingarchive.net/ (accessed August 29, 2024).
NIH Clinical Center (2024). Cxr8. Available at: https://nihcc.app.box.com/v/ChestXray-NIHCC (accessed September 6, 2024).
Nishio, M., Fujimoto, K., Matsuo, H., Muramatsu, C., Sakamoto, R., and Fujita, H. (2021). Lung cancer segmentation with transfer learning: usefulness of a pretrained model constructed from an artificial dataset generated using a generative adversarial network. Front. Artif. Intell. 4:694815. doi: 10.3389/frai.2021.694815
Osadebey, M., Andersen, H. K., Waaler, D., Fossaa, K., Martinsen, A. C., and Pedersen, M. (2021). Three-stage segmentation of lung region from CT images using deep neural networks. BMC Med. Imaging 21, 1–19. doi: 10.1186/s12880-021-00640-1
Ozdemir, O., Russell, R. L., and Berlin, A. A. (2019). A 3D probabilistic deep learning system for detection and diagnosis of lung cancer using low-dose CT scans. IEEE Trans. Med. Imaging 39, 1419–1429. doi: 10.1109/TMI.2019.2947595
Park, C. M., and Lee, J. H. (2022). Deep learning for lung cancer nodal staging and real-world clinical practice. Radiology 302, 212–213. doi: 10.1148/radiol.2021211981
Park, H., and Monahan, C. (2019). Genetic deep learning for lung cancer screening. arXiv preprint arXiv:1907.11849.
Pati, P., Kumari, P., Kumari, N., Mahto, K. S., Marandi, S., Naaz, S., et al. (2022). Current advances in computational biology of lung cancer.
Perez, L., and Wang, J. (2017). The effectiveness of data augmentation in image classification using deep learning. arXiv preprint arXiv:1712.04621.
Rezaei, S. R., and Ahmadi, A. (2023). A gan-based method for 3D lung tumor reconstruction boosted by a knowledge transfer approach. Multimed. Tools Appl. 82, 44359–44385. doi: 10.1007/s11042-023-15232-0
Riaz, Z., Khan, B., Abdullah, S., Khan, S., and Islam, M. S. (2023). Lung tumor image segmentation from computer tomography images using mobilenetv2 and transfer learning. Bioengineering 10:981. doi: 10.3390/bioengineering10080981
Saha, A., Ganie, S. M., Pramanik, P. K. D., Yadav, R. K., Mallik, S., and Zhao, Z. (2024). Ver-net: a hybrid transfer learning model for lung cancer detection using CT scan images. BMC Med. Imag. 24:120. doi: 10.1186/s12880-024-01315-3
Said, Y., Alsheikhy, A. A., Shawly, T., and Lahza, H. (2023). Medical images segmentation for lung cancer diagnosis based on deep learning architectures. Diagnostics 13:546. doi: 10.3390/diagnostics13030546
Salama, W. M., Shokry, A., and Aly, M. H. (2022). A generalized framework for lung cancer classification based on deep generative models. Multimed. Tools Appl. 81, 32705–32722. doi: 10.1007/s11042-022-13005-9
Sasikala, S., Bharathi, M., and Sowmiya, B. (2019). “Lung cancer detection and classification using deep CNN,” in International Journal of Innovative Technology and Exploring Engineering (IJITEE).
Serj, M. F., Lavi, B., Hoff, G., and Valls, D. P. (2018). A deep convolutional neural network for lung cancer diagnostic. arXiv preprint arXiv:1804.08170.
Setio, A. A. A., Traverso, A., De Bel, T., Berens, M. S., Van Den Bogaard, C., Cerello, P., et al. (2017). Validation, comparison, and combination of algorithms for automatic detection of pulmonary nodules in computed tomography images: the luna16 challenge. Med. Image Anal. 42, 1–13. doi: 10.1016/j.media.2017.06.015
Shimazaki, A., Ueda, D., Choppin, A., Yamamoto, A., Honjo, T., Shimahara, Y., et al. (2022). Deep learning-based algorithm for lung cancer detection on chest radiographs using the segmentation method. Sci. Rep. 12:727. doi: 10.1038/s41598-021-04667-w
Song, J., Huang, S.-C., Kelly, B., Liao, G., Shi, J., Wu, N., et al. (2021). Automatic lung nodule segmentation and intra-nodular heterogeneity image generation. IEEE J. Biomed. Health Inform. 26, 2570–2581. doi: 10.1109/JBHI.2021.3135647
Stack Abuse (2023). Optimizing models: Cross-validation and hyperparameter tuning guide. Available at: https://stackabuse.com/optimizing-models-cross-validation-and-hyperparameter-tuning-guide/ (accessed August 28, 2024).
Svoboda, E. (2020). Deep learning delivers early detection. Nature 587, S20–S22. doi: 10.1038/d41586-020-03157-9
Thanoon, M. A., Zulkifley, M. A., Mohd Zainuri, M. A. A., and Abdani, S. R. (2023). A review of deep learning techniques for lung cancer screening and diagnosis based on CT images. Diagnostics 13:2617. doi: 10.3390/diagnostics13162617
UrRehman, Z., Qiang, Y., Wang, L., Shi, Y., Yang, Q., Khattak, S. U., et al. (2024). Effective lung nodule detection using deep CNN with dual attention mechanisms. Sci. Rep. 14:3934. doi: 10.1038/s41598-024-51833-x
Vijayaraj, J. (2021). Various segmentation techniques for lung cancer detection using CT images: a review. Turkish J. Comput. Mathem. Educ. 12, 918–928. doi: 10.17762/turcomat.v12i2.1102
Wang, L. (2022). Deep learning techniques to diagnose lung cancer. Cancers 14:5569. doi: 10.3390/cancers14225569
Wang, S., Yang, D. M., Rong, R., Zhan, X., Fujimoto, J., Liu, H., et al. (2019). Artificial intelligence in lung cancer pathology image analysis. Cancers 11:1673. doi: 10.3390/cancers11111673
Wang, Y., Zhou, C., Ying, L., Chan, H.-P., Lee, E., Chughtai, A., et al. (2024). Enhancing early lung cancer diagnosis: predicting lung nodule progression in follow-up low-dose CT scan with deep generative model. Cancers 16:2229. doi: 10.3390/cancers16122229
WebMD (2022). Lung cancer diagnosis - exams and tests. Available at: https://www.webmd.com/lung-cancer/lung-cancer-diagnosis (accessed April 27, 2022).
XenonStack (2023). Generative ai in medical imaging benefits and its application. Available at: https://www.xenonstack.com/blog/generative-ai-medical-imaging (accessed August 29, 2024).
Yan, S., Huang, Q., Yu, S., and Liu, Z. (2022). Computed tomography images under deep learning algorithm in the diagnosis of perioperative rehabilitation nursing for patients with lung cancer. Sci. Program. 2022:8685604. doi: 10.1155/2022/8685604
Yang, H., Yu, H., and Wang, G. (2016). Deep learning for the classification of lung nodules. arXiv preprint arXiv:1611.06651.
Zhang, F., Wang, Q., and Li, H. (2020). Automatic segmentation of the gross target volume in non-small cell lung cancer using a modified version of resnet. Technol. Cancer Res. Treat. 19:1533033820947484. doi: 10.1177/1533033820947484
Keywords: lung cancer, lung tumor segmentation, deep learning, review, survey
Citation: Hiraman A, Viriri S and Gwetu M (2024) Lung tumor segmentation: a review of the state of the art. Front. Comput. Sci. 6:1423693. doi: 10.3389/fcomp.2024.1423693
Received: 26 April 2024; Accepted: 08 October 2024;
Published: 05 November 2024.
Edited by:
Yi-Zhe Song, University of Surrey, United KingdomReviewed by:
Xi Wang, The Chinese University of Hong Kong, ChinaShailesh Tripathi, University of Applied Sciences Upper Austria, Austria
Copyright © 2024 Hiraman, Viriri and Gwetu. This is an open-access article distributed under the terms of the Creative Commons Attribution License (CC BY). The use, distribution or reproduction in other forums is permitted, provided the original author(s) and the copyright owner(s) are credited and that the original publication in this journal is cited, in accordance with accepted academic practice. No use, distribution or reproduction is permitted which does not comply with these terms.
*Correspondence: Serestina Viriri, dmlyaXJpcyYjeDAwMDQwO3Vrem4uYWMuemE=