- 1The Center for Immersive Experiences, The Pennsylvania State University, University Park, PA, United States
- 2Department of Nutritional Science, The Pennsylvania State University, University Park, PA, United States
- 3Chair of Cognitive Science, ETH Zürich, Zürich, Switzerland
- 4Department of Environmental Sciences, Wageningen University and Research, Wageningen, Netherlands
While different crowdsourcing platforms promote remote data collection, experiments in the immersive Virtual Reality (iVR) research community are predominantly performed in person. The COVID-19 pandemic, however, has forced researchers in different disciplines, including iVR, to seriously consider remote studies. In this paper, we present a remote study using the Immersive Virtual Alimentation and Nutrition (IVAN) application, designed to educate users about food-energy density and portion size control. We report on the results of a remote experiment with 45 users using the IVAN app. In IVAN, users actively construct knowledge about energy density by manipulating virtual food items, and explore the concept of portion size control through hypothesis testing and assembling virtual meals in iVR. To explore the feasibility of conducting remote iVR studies using an interactive health-related application for nutrition education, two conditions were devised (interactive vs. passive). The results demonstrate the feasibility of conducting remote iVR studies using health-related applications. Furthermore, the results also indicate that regardless of level of interactivity learners significantly improved their knowledge about portion size control after using the IVAN (p < 0.0001). Adding interactivity, however, suggests that the perceived learning experience of users could be partially affected. Learners reported significantly higher scores for immediacy of control in the interactive condition compared to those in the passive condition (p < 0.05). This study demonstrates the feasibility of conducting an unsupervised remote iVR experiment using a complex and interactive health-related iVR app.
1. Introduction
Over the past years eXtended Reality (i.e., XR; Virtual/Augmented/Mixed Reality) has demonstrated potential in research (Wilson and Soranzo, 2015), education (Radianti et al., 2020), and industry (Damiani et al., 2018). With the emergence of cost-effective Head-Mounted Displays (HMDs), immersive Virtual Reality (iVR) in particular has gained popularity over the past few years. One of the key distinguishing affordances of iVR is its ability to simulate realistic experiences (Blascovich et al., 2002). As argued by Bailenson (2018), with iVR the gap between a real and a mediated experience is very small, making what the user experiences very close to that of the real-world “A VR experience is often better understood not as a media experience, but as an actual experience” (Bailenson, 2018, p. 46). The naturalness of the interaction is significantly more pronounced with iVR compared to any other form of media to this day (Bailenson, 2018). Therefore, when learning in iVR people can have a similar experience to learning in the real world, leading, in theory, to a more seamless transfer of knowledge and skills to a real environment outside of iVR. There is a growing body of evidence demonstrating the efficacy of iVR in training for transferable skills in the contexts of, for example, military (Liu et al., 2018), healthcare (Siu et al., 2016), training astronauts (Garcia et al., 2020), and football quarterbacks (Bailenson, 2018).
Almost two decades ago, Reips (2000) argued for the advantages of conducting remote experiments. His arguments include access to demographically diverse populations, larger sample sizes with high statistical power, and savings costs. While we have witnessed a shift to remote experiments in numerous Human-Compuer Interaction (HCI) fields (Henze et al., 2011; Gustarini et al., 2013; Reinecke and Gajos, 2015; Mottelson and Hornbæk, 2016), iVR studies are still often performed in the lab with an experimenter present. As noted by Mottelson and Hornbæk (2017), a tremendous amount of effort goes into conducting iVR studies, from recruiting participants, managing schedules, preparing lab environment (physical space)/equipment (iVR-ready PCs), to conducting the actual study.
These challenges are of course not exclusive to iVR studies, but these studies often present additional logistical barriers, including the need for high-end hardware and dedicated open space (Ma et al., 2018; Kourtesis et al., 2020; Saffo et al., 2020). This has led to most research performed in this domain to be limited to in-person experiments with a homogeneous population of participants (mostly University students) and small sample sizes. These limitations often cause a lack of external validity of research findings, and contradictory results produced by different research. Wu et al. (2020), for example, stated that iVR is more effective in relation to learning performance than non-immersive counterparts. However, iVR has a greater effect on K-12 compared to post-secondary students, and prolonged exposure can diminish learning due to fatigue or simulation sickness; and Makransky et al. (2019) showed iVR improves presence but decreases learning.
The COVID-19 pandemic, however, has forced researchers in the XR community to seriously consider remote studies. With the emergence of modern HMDs, XR and in particular iVR, is no longer an expensive technology only available to researchers and industry. Over the past few years, we have witnessed astonishing growth in the population of iVR consumers1 as the technology is becoming affordable, easy to set up, and standalone (i.e., not requiring powerful PCs). In addition, the COVID-19 pandemic has also increased the iVR user-base and the amount of time spent in iVR (Rivu et al., 2021). This provides researchers in the iVR community with an opportunity to conduct experiments remotely. Remote iVR studies, however, introduce new design and methodological challenges. These challenges include recruiting participants who own iVR devices, designing robust virtual experiences that are user-friendly and self-explanatory, and experimental setups that do not require the intervention of an experimenter (unsupervised) while assuring high data quality. Leveraging the power of the crowd by performing remote studies on crowdsourcing platforms such as the Amazon Mechanical Turk (Mturk2), Prolific3, or CrowdFlower4, have shown to be an effective way in obtaining a higher diversity of participants, capturing reliable data, and saving costs (Buhrmester et al., 2011; Mason and Suri, 2012; Paolacci and Chandler, 2014; Rouse, 2015). As such, more attention has been given to this data collection paradigm in the community, particularly since the COVID-19 pandemic.
In 2017, Mottelson and Hornbæk (2017) reported that from a sample of 250 Mturk crowd workers only 3% owned devices capable of running iVR, and 2.2% owned Google Cardboard. Based on an estimation of the active MTurk population on 2015 (Stewart et al., 2015), the authors estimated that at most 226 Mturk workers would own an iVR device at the time of reporting their findings. Such a low number of iVR device owners makes it rather difficult or unrealistic to conduct remote iVR experiments. However, this situation has changed rapidly in recent years due to the more widespread adoption of advanced consumer iVR headsets such as the Oculus Quest5. A growing number of researchers in the iVR community have now experimented with remote studies, and have demonstrated the feasibility of this shift in paradigm (see Section 3.1 for an overview of remote iVR studies). Remote studies have become so in demand in the XR community, that in 2021 a crowdsourcing platform called XRDRN6 specifically for XR studies was introduced.
In light of this, we present and report on the evaluation of the Immersive Virtual Alimentation and Nutrition (IVAN) app, a health-related iVR application using a remote study paradigm. The IVAN app is designed to educate users about food-energy density and portion size control in iVR. In IVAN, users learn from a virtual Registered Dietitian Nutritionist (RDN), to actively construct knowledge about energy density by manipulating virtual food items and explore the concept of portion size control through hypothesis testing and assembling of virtual meals in iVR.
In the context of this research, it is important to understand why nutrition education matters, and how iVR can transform it. Obesity is one of the biggest health challenges facing the United States. Rates of overweight and obesity in the year 2020 have reached a new peak and are projected to rise for years to come (Fryar et al., 2020). The major contributing factor to weight gain is energy consumption in excess of the body's daily requirements. For an individual to correctly consume the amount of energy for their energy needs, there is a need to consider the amount of food and the amount of energy consumed. Therefore, educating individuals about the energy content of food (energy density/calorie density or the number of calories per gram of food), and the amount of these foods consumed (the portion size), has been shown to lead to a reduction in dietary energy intake (Zuraikat et al., 2018). Typically the goal of these education programs is to help individuals understand that they can reduce their intake of higher energy foods while increasing their intake of lower energy foods to reduce energy intake while simultaneously reducing feelings of hunger (Raynor and Champagne, 2016).
However, current methods for creating awareness and educating the public are primarily based on didactic methods, such as the use of pamphlets, handouts, and PowerPoint presentations, typically in consultation with a Registered Dietitian Nutritionists (RDN). Albeit effective, this form of education is not scalable as it usually involves multiple “intervention” sessions with a RDN, making them costly and exclusive to people of specific socioeconomic demographics. Moreover, there are limitations in practicing nutrition-related skills such as portion size control in the real-world. For obvious reasons, practicing with real food in terms of manipulating different items to understand the energy density associated with specific quantities can become costly and involves food waste, albeit being a great learning activity. However, many of these limitations can be potentially mitigated through the use of iVR solutions which provide a way to simulate realistic and ecologically relevant interactions and environments related to food. Immersive VR can afford learners the opportunity to perceive and interact with virtual objects and environments in a natural way. Learners can physically move around, pickup objects, and manipulate them. Indeed, interactivity along with presence and immersion are considered as core characteristics and unique affordances of iVR (Radianti et al., 2020). Additionally, researchers can control and manipulate environmental factors that can potentially influence decision making within the iVR environment, holding these variables constant across individuals (Blascovich et al., 2002; Cheah et al., 2019, 2020) and thereby offering researchers opportunities for a more nuanced understanding of factors that make controlling portion size challenging. With recent computational advancements (improvements to frame-rate, field of view, etc.), iVR devices can provide a sense of immersion and realism (i.e., presence, a feeling of being there) not possible previously, improving the ecological relevance of the learning experience itself as well as the data that are collected in scientific experiments (Parsons and Rizzo, 2008).
Given the numerous advantages of unsupervised remote iVR studies, we will be able to reach a more diverse population of users (compared to University students conventionally used for in-lab experiments) and thus, improve the external validity of findings. This is of utmost importance for health related topics such as nutrition that are not just about theoretical knowledge gain, but also practical skills that can be repeatedly used in everyday life. In light of this, we investigate the following research question: RQ1: Can a health-related iVR application be successfully evaluated in an unsupervised remote experiment?
While it is argued that the immersivity of iVR leads to a more motivating and perceptually effective learning experience, (e.g., Makransky et al., 2019; Zhao et al., 2020), bringing an experience to a Head Mounted Display (HMD) does not by default guarantee an improvement to learning experience or outcome. The design of the experience is still at the very core of what makes an iVR experience engaging and effective. The look and feel (aesthetics) of an experience are undoubtedly important design choices. However, the actions and events inside an experience are the most influential instruments in attaining the ultimate objectives of an immersive experience, having a profound and lasting change in users (Bailenson, 2018). On the one hand, these actions and events can be passive, as seen in many examples of iVR experience based on 360-degrees images and videos and other types, where users are practically observers and do not directly interact with the content. On the other hand, the actions and events can be tied to mechanics of interaction that are utilized by the user to actively interact with the content and explore the environment. For instance, watching a video on how deforestation can negatively affect climate change is not as visceral of an experience compared to an iVR one in which the users can walk around in the nature, cut down a tree and concretely experience the consequences of this action (Ahn et al., 2014; Huang et al., 2021). We argue that the interactivity of an iVR experience may affect the extent to which it can leave a lasting behavioral change in users. In the context of this research, this means inducing a change in the behavior of people in relation to their ability to identify proper portions of foods, and to apply the concept of energy density to their food choices. Furthermore, as it is demonstrated in Section 3.1, the majority of immersive experiences used in remote studies are either lack or have very limited interactivity. Considering that one of the most significant and powerful features of modern iVR HMDs is rich, intuitive, and natural interaction, it is important to understand the feasibility of conducting remote iVR studies using experiences that afford rich interactions.
As a first step to explore the role of interactivity to this end, we sought to investigate the feasibility of conducting a remote iVR study using a highly interactive experience, and to investigate whether interactivity would affect perceptions of learning and learning experience between groups of users who experienced an interactive or a passive version of our learning app. More precisely, we sought to investigate the research question: RQ2: Does an interactive iVR experience cause a more positive perceived learning experience for learners than a passive iVR experience? Therefore, we designed two versions of the IVAN app (one interactive and one passive) were designed and compared. The interactive version of the application required learners to interact with and manipulate virtual food items by for example using a virtual knife to cut them and their virtual hands to grab them and assemble meals based on set goal calorie amounts while listening to instructions from a virtual RDN. In the passive version, the learners only listened to typical, validated, educational materials related to energy density and portion size delivered by the virtual RDN within the immersive environment.
The rest of this article is organized as follows: Section 2 provides the necessary background on understanding the importance of nutrition education. Section 3 provides an overview of the literature on remote iVR studies, and the use of digital technology for nutrition education. Section 4 presents the remote iVR experiment performed using the IVAN app. Last, Section 5 concludes the article, while addressing its limitations and the future directions for this research.
2. Background on Nutrition Education
One of the key strategies in battling weight gain and obesity is controlling portion size. There is a direct correlation between the portion of food that is placed in front of an individual and the amount they consume, with larger portions leading to higher consumption (Rolls et al., 2002; Kral and Rolls, 2004; Roe et al., 2016). This is a phenomena referred to as the “portion size effect” and is shown to be related to individuals' weight and other aberrant eating behaviors (Rolls et al., 2002; Fisher et al., 2007; Brunstrom et al., 2008). Learning to manage the portion size of foods has shown to help individuals lose weight and maintain their weight loss (Raynor and Champagne, 2016; U.S. Department of Agriculture and U.S. Department of Health and Human Services, 2020). Nevertheless, managing portion size and developing the competencies to identity the energy density of portions remains a long-term challenge for individuals (Rolls et al., 2017).
Traditional didactic methods of educating individuals on the tenants of nutrition behavior change are typically done by RDNs in clinical settings. Access to this care can be costly, and is infrequently and inconsistently covered by insurance. If clinical sessions can be completed with these nutrition professionals the current methods of educating individuals about proper portion size and energy density, while proven to be effective, leave much to be desired. Specifically, the use of paper handouts, PowerPoint presentations, and plastic food models as a part of didactic education leave little to no opportunity for interaction with actual foods, which is the skill that precisely needs to be learned and practiced. Immersive VR can provide such an opportunity to patients allowing for immediate application and reinforcement of the hands on skills that are being taught.
It is important to note that technology has long been used in nutrition education. There is a plethora of commercial web and mobile applications as well as research endeavors in this domain. For instance, West et al. (2017) has provided an overview on this topic based on a survey study with 217 participants. Their findings suggest that users generally consider nutrition apps to be effective in increasing their health diet motivation and behavior, self-efficacy and consistency in eating healthy, and desire to set and achieve dietary goals. The work of Tallon et al. (2021) also provides an overview of technology-based interventions in schools for nutrition education. While Tallon and colleagues note the feasibility of this approach, they conclude that technology improvements are needed before we can observe any long-lasting effects.
The ubiquity of smartphones gave rise to the idea of mobile health (mHealth), and consequently the use of phones for nutrition intervention/education. The work of Hingle and Patrick (2016) for instance, shows that only in 1 month of 2016, around 46 million US adults have used health related apps on their smartphones, including nutrition. Most of these apps were related to calorie counters and diet trackers. While beneficial, Hingle et al. argue that there is a lack of rigorous scientific evaluation of commercially available nutrition apps. On similar grounds, Chen et al. (2018) have shown that mobile nutrition apps can be prescribed as supplementary material by dietitians, and have the capacity to streamline nutrition assessments while considering the dietary records of users. Moreover, based on a systematic review of the literature by DiFilippo et al. (2015), it is suggested that mobile nutrition apps are in general advantageous, but more rigorous research is needed to establish their efficacy. DiFlilippo et al. note that while some studies have shown that nutrition apps can help with weight loss, improved adherence to diet monitoring, and decrease the effort in diet continuation without an app, few studies have also shown inconsistent results in terms of their efficacy.
Personalization has also been researched in the context of nutrition education. In a review of the literature by Brug et al. (1999), it is shown that considering the behavior, needs, and beliefs of individuals are important factors in the efficacy of educational content in nutrition interventions. In their work, Brug et al. (1999) conclude that personalized nutrition education is more likely to be read and remembered compared to non-personalized standard materials. These findings are also corroborated by Oenema et al. (2001) and Brug et al. (2003), who have shown that tailoring web-based nutrition interventions provides a more personalized experience and impacts users' motivaation and intention to change significantly more compared to a generic intervention.
This brief overview of the literature on the use of technology for nutrition education is only a snapshot of the vast available literature. Notwithstanding, websites and mobile apps are technologically incapable of enabling users to meaningfully and naturally interact and practice with food items in a scalable way. Virtual Reality (both desktop and immersive) can potentially bridge this gap.
3. Related Work
3.1. Remote iVR Experiments
It is widely argued that remote iVR studies provide tremendous opportunities to recruit participants more effectively and efficiently (Mottelson and Hornbæk, 2017), reach a more diverse study population (Rivu et al., 2021), and conduct scientific research particularly at times when it is not possible to perform in-lab experiments such as during the COVID-19 pandemic (Steed et al., 2020). Gustarini et al. (2013) however, have discussed the challenges involved with using user-owned smartphone devices for in-the-wild experiments, including collection and storage of data, user device heterogeneity, recruitment (self-selection) biases, participants' motivation, privacy, and cheating. Notwithstanding, numerous studies have demonstrated the feasibility and validity of this approach in both conventional HCI domains, (e.g., Andreasen et al., 2007; Germine et al., 2012) as well as iVR, (e.g., Steed et al., 2016; Mottelson and Hornbæk, 2017; Saffo et al., 2020; Zhao et al., 2021).
3.1.1. Content Production and Dissemination
According to Rivu et al. (2021) there are two main approaches for producing content for remote experiments. The first method is developing applications from scratch using game engines such as Unity3D. While this approach provides developers and researchers with autonomy over the research-specific content they would like to produce, it requires tremendous efforts and costs on the production side. The second method is to use existing social iVR platforms such as VRChat7, Rec Room8, or AltspaceVR9 to directly use their existing virtual environments, or their software development kit (SDK) to create a customized one. While this approach is significantly cheaper to produce and promotes scalability, it limits the researchers by the functionalities and features afforded by these platforms. In addition, mechanisms for obtaining user data on these social iVR platforms are very limited (Saffo et al., 2020). Distribution of apps and recruitment of participants, however, seem to be significantly more convenient in this method. Following this analysis, Rivu et al. (2021) have suggested four main approaches for conducting remote iVR studies: (1) distributing a standalone app directly among participants. (2) distributing an app through existing platforms such as Steam, Oculus Store, etc. (3) building an app using the SDK/API of social iVR platforms (e.g., VRChat, Rec Room, etc.) to be directly used on these platforms. (4) using the existing environments of social iVR platforms.
3.1.2. Participants
One of the most widely addressed topics in the literature of remote XR, and in particular iVR, pertains to understanding who device owners are and whether they are suitable as participants in scientific remote studies, (e.g., Mottelson and Hornbæk, 2017; Ma et al., 2018; Rivu et al., 2021). In relation to iVR, Previous work has shown that headset owners that are recruited for remote experiments are not radically different from participants used in in-lab experimental settings at universities, and the data obtained in remote experiments are generally comparable to in-lab counterparts (Mottelson and Hornbæk, 2017). While this seems to be a general theme reported in the literature, some researchers, (e.g., Zhao et al., 2021), have noted that the spatial abilities, gender distribution, and gaming frequency of iVR device owner population are very different than the general public.
Similar observations were made by Rivu et al. (2021), stating that 91% of the iVR device owners in their survey identified as gamers and were predominantly male (77%). Ma et al. (2018), based on a curated panel of 242 Mturk users with access to iVR headsets, have also shown that the demographics of this panel were 60% male, 70% white, and 90% from the U.S.A. Furthermore, it was reported that at the time of this research (2018), only 15% of the curated population had access to advanced iVR headsets with 6 degrees of freedom (DOF). Such lack of diversity is also prevalent in social iVR platform users. As noted by Rivu et al. (2021), during the recruitment process for an experiment, they struggled with finding enough female participants on Rec Room. Therefore, the external validity of any findings reported based on remote iVR studies are questionable. This however, does not mean that in the future when iVR headsets become more ubiquitous, such limitations cannot be mitigated.
From a theoretical perspective, Steed et al. (2020) have motivate the need for a more distributed model of iVR participant recruitment in light of generalizability of findings, and potential access to specific and hard to reach user groups. For a long term solution, the author suggests using governmental scientific funding bodies to purchase and distribute headsets among participants who are willing to participate in multiple studies. They argue that this approach may enable us to reach unrepresented samples and thus, improve external validity. Ratcliffe et al. (2021a) also propose three different approaches for recruiting remote participants for better sample quality. The first is to establish a dedicated XR crowdsourcing community. Recently, such a dedicated community has emerged (see text footnote 6) and is being used by numerous researchers. The second is to leverage existing consumer communities on the Internet, such as the popular iVR groups on social media. The third is to create a hardware lending scheme to give access to a wide and broad range of potential participants, similar to what is proposed by Steed et al. (2020).
3.1.3. Feasibility and Reliability
Another theme of remote iVR pertains to the feasibility of performing unsupervised studies, and consequently, the reliability of the obtained data (Ma et al., 2018; Rivu et al., 2021). For instance, the work of Steed et al. (2016) showed that a remote data collection paradigm is a feasible method for running experiments. However, the types of iVR devices used in this study are already outdated and do not afford any sophisticated form of interaction. On similar grounds, Mottelson and Hornbæk (2017) have compared in-lab (using HTC Vive) and remote (using Cardboard) iVR studies. The authors have concluded that conducting remote iVR studies using user-owned smartphones is affordable and ecologically valid. Notwithstanding, these conclusions should be interpreted under the light of smartphone ubiquity. The population of smartphone owners these days are a much better representation of the general population than the ones who own iVR devices.
From the the population of 242 Mturk iVR users curated by Ma et al. (2018), it was reported that 144 own a Samsung Gear headset, and as such, this device was chosen as the experimental platform for a crowdsourced iVR experiment. Three different experiences were used in this experiment, focusing on place (55 participants), embodiment (59 participants), and plausibility (56 participants) illusions. The results of their study revealed that 98% of their participants performed the iVR task at home, 84% reported that they were alone during the experiment, and 81% reported having enough space to walk around in iVR. These results further support the feasibility of conducting remote iVR studies, even with sophisticated apps that require physical movements. While insightful, the iVR experiences used in the work of Ma et al. lack rich interactivity, while most modern iVR experiences utilize complex interaction mechanics that require advanced headsets (Saffo et al., 2020).
Rivu et al. (2021) report on two case studies that demonstrate the feasibility of performing remote iVR experiments. In the first case study, the authors demonstrate the feasibility of sharing a download link to an independent app among participants, and using this method for recruiting and conducting an iVR experiment. In the second case study, the authors have used Rec Room, a famous social iVR platform, and built their own custom environment for users to perform different collaborative tasks as part of the experiment.
On similar grounds, Saffo et al. (2020) have discussed remote studies from the perspective of social iVR. Their work demonstrates the potential of using VRChat, a massive social VR platform to conduct a remote iVR experiments. Ten participants were recruited from the public room VRChat and were invited to a private experiment room created by the researchers. While the authors conclude that VRChat is a feasible platform for conveniently recruiting participants for remote iVR experiments, it has limitations in terms of what can be implemented with the available SDK. In addition, Social iVR platforms are great for collaborative tasks, and provide the experimenters with the opportunity to be “there” in the virtual space. However, they suffer from limitations such as unprofessional behaviors by participants, and lack of program communication with external servers for user data logging. Furthermore, Saffo et al. (2020) have noted that a variety of iVR headsets are used by users in VRChat which could affect the validity and reliability of experimental data.
In a more recent work, Saffo et al. (2021) reported on their latest experience with using VRChat for remote iVR studies. They report on a collaborative qualitative study (tabletop collaborative coupling) and a quantitative study (Fitt's law). The authors report that they have successfully replicated the findings reported in in-lab studies, and as such, argue for the feasibility and validity of using social iVR for conducting remote experiments. Despite the convenience of social iVR platform for recruiting large participants, the authors only managed to recruit 23 for the first study and 8 for the second study. Furthermore, due to a lack of logging functionality, the authors used video recordings and in-game screenshots from the screen of the experimenter for post-intervention analysis. This approach, however, would not scale with more complex forms of data such as headset/controller tracking, eye-tracking, physiological data, etc. In addition, Saffo et al. (2021) have noted that in order for an experimenter to monitor the participants in VRChat, they need to be virtually present in the room using their avatar, which can potentially influence the behavior of participants, and thus the reliability of the data.
Huber and Gajos (2020) have also shown that using a remote iVR experiment they were able to replicate findings from an in-lab study. However, they note that in the remote study the effect sizes were smaller and the gender distribution was skewed toward male participants. Similar observations were made by Mottelson et al. (2021), reporting on the validity and reliability of remote iVR data while admitting to sampling issues (predominantly male). These findings are also corroborated by Zhao et al. (2021). Their research showed that their sample is male dominated, and remote participants exhibit higher sense of direction compared to an in-lab sample previously recruited for the same stimuli. However, their results indicate that the reliability of the data obtained from a remote experiments (42 participants—using the Oculus Quest) was as good as the in-lab (22 participants—using the HTC Vive) counterpart. The authors conclude that remote iVR studies are a feasible and effective alternative to University participant pools, while admitting that the lack of diversity in the demographics of remote participants impedes researchers from generalizing their results.
3.1.4. Challenges and Opportunities
Ratcliffe et al. (2021a) performed a survey study with 46 iVR researchers to gain a deeper understanding of the perceived challenges and benefits of conducting remote experiments. The results of a thematic analysis revealed that the participants generally think recruitment for remote studies would be easier than in-lab and a better option for cross cultural investigations. It was also noted that performing in-lab experiments requires extensive organization and set up time, whereas remote studies are potentially less time consuming, especially if they are encapsulated (experience and data collection in the same app) and unsupervised.
The respondents have also highlighted three major challenges. First is about hardware (e.g., VR-ready PC, Electroencephalography, eye-tracking, etc.). Second is about monitoring or sensing challenges (data such as facial expressions are lost, qualitative feedback is difficult to obtain). Third is about data transmission and storage (issues with transmission and collection of user data, specifically sensitive ones such as medical data). Another concern is related to the environment in which participants use iVR. Laboratory settings are controlled, whereas unsupervised experiments at homes are prone to interruptions, environmental noise, and lack of adequate and safe space.
On similar grounds, Rivu et al. (2021) report on a conceptual framework for remote iVR studies based on the results of an online survey with 227 participants who are iVR headset owners, assessing their demographics, iVR setup, and attitude toward remote participation. Rivu et al. note that the average time people who own iVR headsets spent in iVR has increased during the COVID-19 pandemic. Furthermore, more than 73% of their respondents have indicated preference for participating in remote studies during the pandemic, while 67% have indicated that they will still prefer remote studies post-pandemic. As such, Rivu and colleagues argue that there is a substantial demand for remote studies, and thus, there is great potential in running studies using this paradigm.
3.1.5. Summary
The state of the art shows a general belief that performing remote studies will help with the heterogeneity of the sample, and increasing the external validity of findings, defined as the extent to which findings can be generalized to a broader population. While this may be true for other domains in Human-Computer Interaction, in the domain of iVR there is still an inherent bias in the population of users who own HMDs. The literature shows that the majority of this population are gamers and predominantly male, and therefore not a very good Representative of the population. This is also corroborated by findings from Peck et al. (2020) showing that females are underrepresented in iVR research and as such there is a systematic bias in the population of iVR participants.
The state of the art also shows that remote iVR is already a feasible data collection paradigm. While the sample size in most of the reviewed research are still rather small (compared to other HCI studies), with the emergence of more cost-effective HMDs such as the Oculus Quest, we expect to see a much larger population of potential remote iVR participants in the near future. It is estimated that by 2023, 18.81 million high-end augmented and virtual reality HMDs will be sold10). We also see that most researchers are in the early phases of testing the applicability of remote iVR studies, and most often they test the migration from in-lab to remote studies with simple iVR experience and with very limited interaction. To the best of our knowledge, the IVAN iVR experience we present in this article is the first health-related iVR app with complex interaction mechanics that has been used in an unsupervised remote iVR study.
3.2. Virtual Reality and Nutrition Education
Studies about the implications of iVR for general education have suggested that the immersivity and interactivity of iVR experiences can potentially improve the performance and self-efficacy of learners (Markowitz et al., 2018). Since the emergence of modern cost-effective iVR headsets, more scientific findings are being reported on the effect of iVR on promoting motivational outcomes and offering superior learning experiences compared to traditional desktops and real-world experiences (Makransky et al., 2019; Zhao et al., 2020). Moreover, there is an abundance of iVR studies that demonstrate the efficacy of iVR in improving the learning outcome of individuals (Chittaro and Buttussi, 2015; Alhalabi, 2016; Webster, 2016; Lamb et al., 2018; Krokos et al., 2019; Makransky et al., 2019).
Numerous examples can be found in the literature on the use of desktop VR applications for the delivery of nutrition-related educational topics. For example, work by Majumdar et al. (2012) demonstrated that VR games may be able to improve dietary behaviors with middle-school aged youth. Others have even shown that VR programs can also improve longer term health outcomes such as weight loss and weight maintenance (Sullivan et al., 2013). These trials demonstrate the far reaching potential of VR applications to improve eating and health behaviors.
In a scoping review by McGuirt et al. (2020), the authors provide an overview of nutrition education and behavior change facilitated by virtual and augmented reality. Most studies evaluated used developmental testing in lab settings, employed descriptive or observational methods, and focus on momentary behavior change such as food purchasing behaviors rather than education. Of note, there appears to be a gap in the current use of iVR technologies. This is relevant as studies have demonstrated the ecological validity of iVR in education about nutrition-related topics. In the context of food buffets for example, it is shown that food selections made in iVR are similar to those made in real and fake food buffets (Persky et al., 2018; Ung et al., 2018; Cheah et al., 2020). In addition to making similar food choices to real world situations, participants in these studies also report that the iVR environment feels natural and decisions made during these paradigms represent their real-world behavior (Ruppert, 2011; Lombart et al., 2019; Siegrist et al., 2019).
Recent work has also shown that physiological responses to food in iVR environments are similar to experiencing real-world food cues such as food pictures, advertisements, or actual food (Gorini et al., 2010; Ledoux et al., 2013; van der Waal et al., 2021). In contrast to other potential desktop or smartphone based VR applications, iVR allows food to be presented on a 1:1 scale identical to that of the real-world, which is likely important for training skills that specifically rely on visual perception, such as the estimation of food portion sizes. Our review of the state of the art applications indicates that iVR applications specifically designed to teach about specific nutritional topics such as energy density and portion control are to the best of our knowledge nonexistent. Therefore, The overarching goals of this project is to investigate the applicability and efficacy of iVR in teaching about these topics using the IVAN application.
4. Immersive Virtual Alimentation and Nutrition
The design of the Immersive Virtual Alimentation and Nutrition (IVAN) app (Edwards et al., 2022) is based on established best practices in the fields of nutrition education. Two of the members of the authoring team are experts in nutritional sciences, while one is also a RDN. These domain experts developed the educational content of the app using evidence-based practices and Academy of Nutrition and Dietetics Standard Operating Procedures (Vermeer et al., 2014; Raynor and Champagne, 2016; Rolls et al., 2017; Zuraikat et al., 2018), which have demonstrated effectiveness in improving portion size control and the understanding of energy density of foods (Zuraikat et al., 2018). These practices and procedures were translated into interaction mechanics and actionable tasks in an interactive, immersive environment using the constructivism learning theory (Hein, 1991).
The IVAN app was developed using the Unity3D game engine and optimized for the Oculus Quest head-mounted displays (HMDs). The experience could be player in a stationary iVR setup, and as such, the participants did not require a significant physical space in order to complete the experience. There are eight learning modules integrated into the IVAN app that take the learner through the steps necessary for learning about the concepts of calorie density (CD) and portion size control (PSC). These modules allow the individual to directly apply, practice, and receive feedback on the CD and PSC techniques being taught. After putting on the HMD, and before going through the learning modules, the learner first enters a training area in which they receive guidance on the iVR headset, the device controllers, and navigating the iVR environment. Furthermore, the learner has the option to indicate their dominant hand and adjust the height of their virtual self in proportion to the virtual environment to the degree they feel comfortable.
After the training, an introduction scene is loaded, during which the learner finds themselves in the virtual office of a digital RDN who is sitting on a chair and in front of a large TV screen on the wall. The digital RDN (Figure 1) is represented by a Caucasian female embodied conversational agent (Sajjadi et al., 2019), equipped with verbal (speech synthesis) and non-verbal behavioral cues (gestures, lip-syncing, and blinking). The digital RDN introduces herself, as well as the activities in the iVR app to the learner. This is followed by the first two learning modules on the introductions to the theories of PSC and CD. In the PSC introduction module, the digital RDN explains to the learner the ways portions have grown over time and how they can impact weight status. The verbal explanations of the digital RDN are complemented with graphical and textual information presented on the TV screen. In the CD introduction module, the concept of CD is explained by the digital RDN, complemented with pictorial examples of high and low-calorie foods on the TV screen.
The remaining six learning modules are hands-on activities and are designed based on the learning theory of constructivism. The constructivist approaches to learning advocates the idea that we learn best when actively engaged in the learning experience to construct knowledge, as opposed to passively receiving information. Learners connect information with previous knowledge and experiences to create new knowledge (i.e., assimilation), and continuously redevelop/revise their knowledge space based on new information and experiences (i.e., accommodation) (Huang et al., 2010; Onyesolo et al., 2013). Within this approach to learning, the actual acquisition of knowledge occurs through performing hands-on activities in a learner-centered environment (Onyesolo et al., 2013), and the instructor (in this case a digital RDN) is only a facilitator/guide. The third learning module focuses on teaching the learners how the preparation of food can impact CD through an interactive activity where they are required to prepare steamed and fried potatoes and then compare their CD. During this activity, using their virtual hands the learners will grab potatoes and throw them in a pot of boiling water and a deep fryer. The resulting dishes (a plate of boiled potatoes and a plate of fries) are then placed on the table in front of the learners, and the corresponding CD values are visualized on the TV screen on the wall. This activity requires exploration and direct activation from the learner to construct the knowledge that how food preparation method (boiled vs. Deep fried) affects its CD (Figure 2).
The acquired knowledge through an interactive activity about CD is then incrementally completed in the forthcoming learning modules. In the fourth learning module for instance, the learners are taught about the interaction between CD and portion size through an interactive activity that requires them to practice serving specified calorie amounts of foods of varying CD using a virtual knife and scale (Figure 3). In this activity, the learners are presented with a target CD goal displayed on the TV screen. Their task is to cut different food items using a virtual knife and then place the food item on a virtual scale that shows its CD value. They have unlimited number of tries to learn what portion size of a food item corresponds to what calorie. The mechanism for virtually cutting food items using natural hand gestures and then measuring their CD is a good example of using iVR to train for a skill (i.e., PSC) transferable to real-world contexts, and is also a clear example for how knowledge can be constructed through experimentation. This activity is followed with the fifth learning module that shows nine food items for the learners to be identified as low or high CD. When combined with the previous and forthcoming activities, this learning module helps learners gain practical experience on how to effectively control their portion size by first identifying the CD of various food items. The sixth learning module presents another activity that reinforces the interactions between CD and portion size. The learners are required to assemble two dishes, one of high and one of low CD. They will then have to use the virtual scale to contrast the calories, CD, and visual components of these two meals, visualized on the TV screen (Figure 4). This module is aptly named “eating more to eat less”, helping learners train for PSC by assembling dishes with higher quantity of food but with lower CD to construct the knowledge that you can eat more food and at the same time consume less calories.
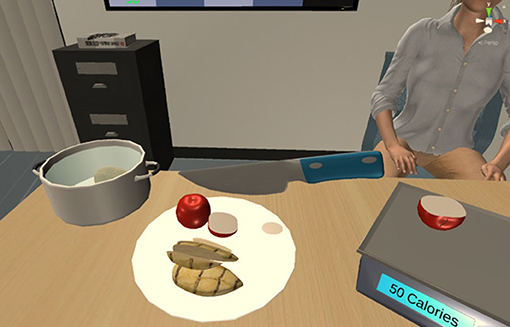
Figure 3. Cutting food items with the virtual knife to meet a set calorie goal (interactive condition).
The last two learning modules help the learners redevelop/revise the knowledge they have constructed so far, through experimentation and practice. As such, the seventh learning module provides the learners with the opportunity to assemble their own plate to reinforce the interaction between CD and portion size. In this module, the learners will explore and learn the extent to which low quantities of high CD food adds to the overall calorie of their dish. Lastly, the eighth learning module enables the learners to actively refine their understanding of CD in relation to different types of food through a cutting exercise. The learners are presented with a series of single food and meal options. They are asked to serve themselves single food items of a certain calorie goal and assemble meals at a certain calorie goal.
To inform the size of objects within the iVR environment, virtual food models were designed based on the NASCO11 food models used by RDNs in point of care settings (Thompson and Subar, 2017). Therefore, the 3D models of food items are realistic in size and in visual fidelity of appearance to both clinically appropriate portion sizes and what individuals would be shown in a real-world clinical setting. The following nine food models used: potatoes, French fries, steamed potatoes, chicken breast, broccoli, apple, carrots, hamburger, and cookies. The food models were systematically designed to ensure their caloric value correlates to their gram weight. This allowed for the models to be cut and maintain a real-world weight and caloric estimate based on the volume of the food model.
To investigate the second research question formulated in the introduction (RQ2: Does an interactive iVR experience cause a more positive perceived learning experience for learners than a passive iVR experience?), two versions of the IVAN app were created. As was explained in this section, the interactive version required the learners to interact with and manipulate virtual food items by using a virtual knife to cut them and then moving their virtual hands to grab them and assemble meals based on set goal calorie amounts while listening to instructions from a virtual RDN. Therefore, the IVAN app in the interactive condition fully utilized the functionalities afforded by an iVR system. The passive version of IVAN is similar in content, but in it, the learners only had to listen to the virtual RDN and observe the environment to go through the modules. Therefore, no interactions in the form of cutting virtual food items or assembling meals were performed by the learners. The passive version allowed the learners to grab virtual food items placed on a table in front of them for demonstration purposes, but they were not required nor able to perform any meaningful actions with them in order to finish the experience. As such, the IVAN app in the passive condition did not fully utilize the functionalities of an iVR system.
Both conditions were devised in iVR to eliminate the potential effect of any confounding variables as a result of comparing iVR with a non-immersive intervention. Notwithstanding, the passive condition of the IVAN closely and realistically (because of the pronounced media equation effect of iVR discussed in Section 1) resembles a didactic method for learning about calorie density and portion control, such as presentation from a RDN. As such, this enables us to compare learning about these nutrition-related topics in a common way with a constructive and exploratory way that fully utilizes the power of iVR.
As part of an iterative design process, the interactive version of IVAN was evaluated in a heuristic study with seven experts in the field of nutrition studies to assess its usefulness, applicability, and usability. The result of the heuristic evaluation, details of which are not reported here, revealed an overall positive attitude toward the IVAN. Experts found the tool to be impressive and demonstrating potential in disseminating nutritional information in an engaging way and as supplementary material.
5. Remote iVR Experiment With IVAN
In this exploratory remote study, the two versions of the IVAN app were evaluated using a between-subject design with N = 45 remote participants, where each participant was randomly assigned to either the interactive or the passive version of the IVAN app.
5.1. Methodology
5.1.1. Participants
Participants who own an Oculus Quest device were recruited remotely from academic mailing lists (Gamesnetwork, 3dui), social networks (Reddit), and crowdsourcing platforms (Amazon MTurk, and Prolific). These participants were recruited previously for another remote iVR study conducted by the authoring team of this paper, and showed interest in participating in future remote studies. All participants were invited to take part in this study through an email. In total, 45 participants were recruited, all of whom having prior experience with both iVR and participating in remote research studies. From our remote sample, 22 participants (17 males, average age of 31.09) were randomly assigned to the interactive version (i.e., experimental condition), and 23 (21 males, average age of 31.39) to the passive version (i.e., control condition) of the IVAN app. The participants were compensated with a $20 Amazon gift card for their participation time.
One limitation of this study was the relatively limited sample size of 45 participants. However, given that this was an exploratory pilot study we feel that the sample was still sufficient to explore the potential of fully remote data collection processes using iVR. We do note that in this study we offered significantly more financial incentive to ensure compliance of participants with study procedures. This is due to the fact that participants had arguably more burden up front with the need to follow study instructions to download and install our app on their HMDs prior to completing all other study procedures. This download and installation procedure is both a technical and to some degree time consuming process. The need to pay a higher cost contributed to our small sample size. However, we expect that in the near future with the emergence of XR crowdsourcing platforms as well as the ability to provide custom applications through widely accessible digital store fronts the associated costs of participant recruitment would be lowered.
5.1.2. Measures
The general and demographic information of participants was measured using fifteen questions related to age, height, weight, sex, student status, highest level of education obtained, dominant hand, color blindness status, using glasses/contact lenses status, race/ethnicity, household income, last time caffeine was used, last time a meal or a snack was eaten, and the last time exercised. The behavior, learning experience, and learning outcome of participants were measured using a combination of instruments. The participants' nutrition related behavior was measured using the adult eating behavior questionnaire (Hunot et al., 2016), the shortened three factor eating questionnaire (Stunkard and Messick, 1985), and the current portion control behavior questionnaire (Spence et al., 2015). Furthermore, participants' competencies in relation to the educational topic offered in the iVR app was measured using the portion control self-efficacy (PCSE) questionnaire (Fast et al., 2015), and the portion control and energy density subscales of the weight management nutrition knowledge questionnaire (Mikhail et al., 2020). The perceived learning experience of the participants was measured based on a variety of questions assessing the participants' perception of the immediacy of control, perceived usefulness, ease of use, presence, motivation, cognitive benefits, control and active learning, reflective thinking, perceived learning effectiveness, confidence, and satisfaction of the application. Definitions of these measured variables can be found in (Lee et al., 2010).
5.1.3. Procedure
The study was approved as meeting the requirement of ethical treatment of human subjects by the University's Institutional Review Board (IRB) before the commencement of data collection.
As the data collection process was done remotely, participants were sent an APK file (either passive or active depending on the condition they were randomly assigned to) along with detailed email instructions on how to install an APK on their Oculus Quest HMD. Prior to using the iVR app, the participants were asked to open the link to an online survey in the web browser of their personal computer and fill in the pre-test questionnaire. The pre-test started with the informed consent form followed by the demographics and general information questions. Afterwards, the participants had to fill in the eating behavior, the shortened three factor eating, the current portion control behavior, the portion control self-efficacy (PCSE), and the portion control and energy density metrics. At this point, a message was shown on the online survey, stating that the pre-test section of the survey is finished. This message also included instructions on how to run the APK previously installed, along with a start code that was required to run the IVAN app. This mechanism allowed us to make sure that the participants finish the pre-test questionnaire before they could run the app.
After going through the IVAN app, and upon finishing all the modules, a message inside the iVR app along with a finish code was shown to the participants that instructed them to take the HMD off, return to the online survey, type in the code and continue with the post-test section of the survey. this mechanism allowed us to make sure that the participants actually finished going through the IVAN app before they could proceed with the post-test. In the post-test, the perceived learning experience of the participants were measured using the immediacy of control, perceived usefulness, ease of use, presence, motivation, cognitive benefits, control and active learning, reflective thinking, perceived learning effectiveness, confidence, and satisfaction of the application measures. These measures were followed by the post-test repetition of the two competency metrics of PCSE and weight management nutrition knowledge questionnaire. The entire process approximately took 40 min (the average time the survey was active; i.e., from the pre to the post-test) to finish.
The code input mechanism we devised for getting into the app and back into the survey assured that the participants could not skip any of the steps we intended them to go through as part of the procedure. All 45 participants completed the entire study, and none spent a significantly different amount of time than average to finish (less than 1/3 of the median completion time, or more than three standard deviations of the mean complete time), indicating that all the steps in the procedure were experienced continuously, without any interruptions or breaks.
5.2. Analysis
Demographic variables were analyzed using the t-test (age) or Chi-square tests (gender, income, education, race, ethnicity) to examine if there were any differences in these variables between the interactive and passive experience groups.
To assess if there were differences in the learning outcome of participants in light of portion size self-efficacy (PCSE) and weight management nutrition knowledge scores pre- and post-test, repeated measure analyses of variance (ANOVAs) were performed with time (pre-test/post-test) as the within-subject factor, and condition (interactive/passive) as the between-subject factor.
To assess differences in the perceived learning experience metrics between the two conditions, independent sample t-tests were conducted for the overall group average scores as well as the group average of each subscale. For exploratory purposes, average scores for each individual question of the perceived learning experiences and outcome questionnaire were compared between the two groups using independent sample t-tests.
For the knowledge measure, a total value was calculated by summing the scores for individual items comprising each measure. For the experience measures, individual items were averaged and collapsed into the final metric. A priori p-value of p < 0.05 was used to indicate significance in all cases. All the assumptions for a repeated measure ANOVA were met in the analyses. All the analyses were performed with IBM SPSS.
5.3. Results and Discussion
The analysis of the general and demographic information indicates a rather homogeneous sample population in terms of income, education, race, and ethnicity (Table 1). While we have managed to recruit a relatively diverse populating of remote participants in terms of education and income, similar to what is reported in the remote iVR studies literature, our sample is also predominantly male dominated and Caucasian.
To explore the effect of IVAN on the learning outcome, two repeated measure ANOVAs were conduced on the influence of two independent variables (condition: interactive, passive; and time: pre-test, post-test) on the learning outcome measures of participants (portion size self-efficacy, and weight management nutrition knowledge, Table 2).
With respect to the portion size self-efficacy (PCSE), The results showed a significant main effect for time (pre-test/post-test): F(1,43) = 20.71, p < 0.0001, R2 = 0.325, indicating an improvement in portion size self-efficacy from the pre to post-test. However, no significant main effect for condition (interactive/passive) [F(1,43) = 1.29, p = 0.26, R2 = 0.029] or interaction between time and condition [F(1,43) = 0.17, p = 0.68, R2 = 0.004] was shown (Figure 5). The PCSE measures the learners competencies in relation to knowledge about portion control using items such as one's ability to control portion size when eating out with others, the extent to which others can influence how much one eats, the ability to handle eating the right food portion regardless of the food type, etc. Our findings indicate that the IVAN app has significantly improved the portion size self-efficacy of participants, which was the main topic the interactions were designed around. As such, our findings support that learning materials in the IVAN app were specific to portion size and energy density, exactly as we intended them to be.
With respect to the second measure of learning outcome, the weight management nutrition knowledge scores, our results did not indicate any significant main effects or interaction. This was to some degree expected, as the design of the IVAN app was primarily focused on portion size and energy density, and not on weight management.
To discuss the implications of IVAN on knowledge acquisition, our results suggest that iVR has the potential to educate people about food-energy density and portion control regardless of its interactivity. While we do see an increase in the portion size self-efficacy of people from pre to post intervention, it is important to note that the type of knowledge gain measured in this experiment is perceptions of self-competence. The ultimate objective of educating people about nutrition-related concepts using iVR is to leave a lasting effect that would manifest itself as a change in their behavior. Although this objective goes beyond the scope of the current study, our results suggest a very interesting and promising finding. In classical settings where topics such as calorie density and portion control are taught to people with the help of a RDN (resembling the passive condition in this experiment), multiple sessions are recommended to see increases in the competencies of people (Raynor and Champagne, 2016). Our results suggest that iVR may be able to improve food-related competencies in a much more scalable way.
Analyzing our results in light of perceived learning experience, however, provides preliminary evidence that manipulating interactivity could lead to different perceptions of learning experience. The results suggest that the perceived learning experiences of participants were marginally better in the interactive condition (M = 4.57, SD = 0.49) than the passive condition (M = 4.11, SD = 0.85) with respect to the immediacy of control metric [t(41) = 2.18, p < 0.05] (Table 3), Although we do highlight the exploratory nature of this study and the need for future confirmatory studies. The comprising items for the interactivity metric were as follows:
1. The ability to change the view position of the 3-D objects allows me to learn better.
2. The ability to change the view position of the 3-D objects makes learning more motivating and interesting.
3. The ability to manipulate the objects (e.g., pick up, cut, change the size) within the virtual environment makes learning more motivating and interesting.
4. The ability to manipulate objects in real time helps to enhance my understanding.
The comparison of the individual question items for the immediacy of control measure of perceived learning experience is depicted in Table 4. We see that participants scored marginally higher for two of the questions related to how immediacy of control helps them with learning and understanding. However, we do highlight that these results are exploratory. As this metric is directly related to the interaction with and manipulation of the virtual objects, we can consider this as promising preliminary support of our manipulation check. Furthermore, this finding seem to suggest a potential effect of interactivity affordance on perceived learning experience in iVR which should be explored further in future studies.
No other differences between the interactive and passive versions for the measured metrics were shown to be statistically significant.
To offer an answer to RQ1: Can a health-related iVR application be successfully evaluated in an unsupervised remote experiment?, our results demonstrate the feasibility of conducting a remote health-related iVR study. In light of this, we argue that the paradigm of remote iVR experiments is a viable approach for disseminating and evaluating educational iVR apps that go beyond fundamental research questions pertaining to iVR (e.g., place illusion, embodiment illusion, presence, etc.), and instead focus on developing knowledge or competencies in users that can be ultimately transferred to and used in the real-world.
Furthermore, our experience with conducting a remote iVR experiment shows that it is perfectly feasible to reach a population of users with access to advanced iVR HMDs, capable of running complex iVR apps. Our results also indicate that it is feasible to conduct a remote iVR study with a complex and highly interactive (in one condition) application. Thus, providing evidence that unsupervised remote iVR studies can be performed with immersive experiences that require extensive physical movements from the users (i.e., cutting virtual food items and assembling meals). The majority of the reviewed remote iVR research works in Section 3.1 have focused on experiences with no to minimal interactivity. However, our study provides evidence that remote iVR studies do not have to be limited in interactivity, and more complex applications can be disseminated and evaluated using the remote experiment paradigm if they are carefully designed.
However, we are still limited to biases in the demographics of iVR device owners, as they still seem to be predominantly male, Caucasian, and belonging to a certain age group, similar to what is reported in Ma et al. (2018); Huber and Gajos (2020); Zhao et al. (2021). Notwithstanding, the characteristics of participants presented in Table 1 indicate that using a remote experiment paradigm, we have managed to reach out to a diverse population of users in terms of income and education level. We predict that in the near future, and with the emergence of concepts such as the Metaverse (Lee et al., 2021), consumer-grade iVR devices would reach a level of ubiquity similar to smartphones. This will undoubtedly alter the design, dissemination, and evaluation of iVR experiences and help researchers in gaining access to a more diverse population of remote participants.
To offer an answer to RQ2: Does an interactive iVR experience cause a more positive perceived learning experience for learners than a passive iVR experience?, our results indicate that iVR has the potential to educate people about food-energy density and portion size control regardless of its interactivity. However, learners could potentially have a better perceived learning experience, at least in relation to immediacy of control, when they can interact with and control the 3D objects around them in a meaningful way. As such, interactivity seems to be partially affecting how people perceive and react to an educational iVR experience, although future studies are needed to further investigate this relationship.
It is important to note that remote iVR data collection methods pose specific challenges that can affect different aspects of studies focusing on behavioral outcome of users, such as the one performed in this paper. In laboratory settings, researchers can assess many different aspects of users such as physiological data, skin conductance, heart-rate, blood pressure, Electroencephalography, eye-tracking, positional tracking data, and more, that can prove to be vital and revealing in understanding the behavioral outcomes of users (Ratcliffe et al., 2021b). Some of such data can be extracted from some of the available HMDs in the market (e.g., HTC Vive Pro Eye12, HP Omnicept Reverb13), but these devices are often more expensive and not really of interest to the population of users who purchase iVR devices for entertainment purposes. We are however, capable of logging performance as well as positional and orientational tracking data from users' headsets and controllers and post them to a server for analysis. While such data can potentially pose privacy issues (Miller et al., 2020), they can reveal useful behavioral tendencies in participants and help us better understand the implications of our educational intervention on their behavior while playing.
We have learned valuable lesson from our experience with conducting a remote iVR experiment. First, the dissemination of iVR apps for standalone HMDs are not as easy and user friendly compared to social iVR platforms or desktop experiences. Participants need to install a third-party APK on their HMDs before they can access the virtual experience. While there are software and tools available that facilitate this process, it is still an extra burden placed on the participant and could cause privacy concerns. In contrast, based on our past experiences, web-based, social, or desktop iVR apps could be directly executed without any additional installations. However, they come at a cost (e.g., requiring tethered HMDs—for desktop iVR apps, limited functionality and development freedom—for social iVR, limited visual fidelity—for web iVR).
Second, while conducting a remote iVR experience is more efficient and cost effective in terms of equipment costs, time, and dedicated lab space, we had to offer significantly higher financial incentive to our remote participants. Given the length of our experiment, and the required efforts for downloading and installing the app, increasing the financial compensation was the only option to attract participants.
Third, similar to other remote iVR research, we also found it difficult to curate a representative sample with more females. Our experience with conducting this remote study show that there is a serious need for alternative ways to reach a larger female population of iVR users for remote studies. Perhaps using online communities that focus on apps specifically designed for a certain gender or age-rage would alleviate this problem to some extent, but it could also be a source of sampling biases. We are hoping that emerging crowdsourcing platforms such as XRDRN, the increasing popularity of low-cost HMDs, and hopefully the emergece of the Metaverse would be an effective way to address sample homogeneity issues.
Fourth, the IVAN app required participants to perform extensive physical movements. While these movements did not necessarily require them to physically walk, it required them to move their hands and manipulate virtual objects. We chose the Oculus Quest HMD as the platform for running our app, and this headset requires the users to define a so called “play area” in which they can physically but safely move. In light of this, we believe that the iVR technology as matured to a point where experiments that require extensive physical movements could be safely conducted without the supervision of an experimenter. This opens the door for numerous opportunities for future remote iVR experiments.
Despite some of the challenges we faced during this exercise, we are still in agreement with other researchers in the community that overall, the advantages of remote iVR experiments outweigh their shortcomings.
6. Conclusions, Limitations, and Future Work
In this paper, we presented and remotely evaluated an immersive VR app called IVAN aimed at educating people about the concepts of calorie density and portion control. To explore the applicability of iVR in nutrition-related education, two conditions were devised where one fully utilized the features of iVR (i.e., interactive), and the other resembled a classical way of learning about this topics with the help of a RDN and did not utilize the full potential of iVR (i.e., passive). The two unique contributions of this work, based on the results of an evaluation with 45 participants are that health-related iVR applications can be effectively disseminated and evaluated using a remote study paradigm, and that regardless of interactivity, experiencing the IVAN app increases the knowledge gain of learners in light of portion control self-efficacy.
For iVR applications with health-related topics such as nutrition, sample size is of utmost importance for the external validity of results. It is well-established that conducing in-lab iVR studies are challenging due to the involved logistics (Mottelson and Hornbæk, 2017). Consequently, studies often suffer from samples with small sizes. Remote iVR, indeed seems to be a viable approach to bridge this gap. We have demonstrated the feasibility and convenience of recruiting 45 remote participants with access to advanced iVR HMDs (i.e., Oculus Quest). While our sample is comparable to other remote iVR studies reviewed in Section 3.1, it can still be larger and more diverse to further increase the external validity of our findings. The study presented in this paper was in a ways both a pilot and a feasibility study. However, our results have enabled us to perform a (currently underway) more informed and rigorous larger trial based on these findings.
Immersive Virtual Reality has shown to be able to cause an increase in nutrition knowledge in a short time and an scalable way using remote data collection paradigm. In light of this, we conclude that our results suggest iVR to have the potential to be used for nutrition-related education. It is important to note however, that our study design does not compare iVR against conventional in-person interventions with RDNs or non-immersive apps, but rather only focuses on different versions of iVR (manipulating interactivity) in a remote study context. As such, our work is limited in the conclusions we can draw in relation to whether iVR matches or statistically outperforms in-person interventions with RDNs, or desktop/mobile nutrition apps. As part of the future direction of this research, however, we are currently comparing the interactive version of IVAN against conventional interventions with a RDN to gain a better understanding of the efficacy of iVR in nutrition-related education. It is also our aim to compare our current iVR version of the IVAN against a non-immersive version to investigate the effect of immersion on the learning and experience of users.
Furthermore, the form of knowledge gain assessed in this research pertains to only perceptions of competence assessed using self-reported questionnaires. The IVAN app, on the other hand, enables learners to explore and construct practical knowledge and skills through hands-on activities in the interaction condition. As such one of the limitations of the current research is the lack of an assessment that focuses on the development of practical skill in relation to calorie density and portion control. Therefore, as part of the future direction of this research, we will be using hands-on tests prior to and after interaction with the IVAN to explore the effect of interactivity on practical knowledge gain of individuals.
Moreover, our results suggest that the interactivity of an educational iVR app could have potential partial effect on the perceived learning experience of people in light of the immediacy of control metric. In light of this, our results seem to suggest that the ability to perform meaningful and decisive interactions in an educational iVR experience could be an important design consideration that can contribute to a more positive perceived learning experience. While interactivity adds theoretical value, for example, from a constructivist perspective to the learning experience, it might unfold its real value through longer terms effects that were not part of the current study. In addition, high interactivity in iVR applications could also manifest itself in physical movements in the virtual world. As was reported in the study conducted by Ma et al. (2018), 81% of their respondents have reported access to enough space to walk around in iVR. In addition, a large number of current iVR apps available on popular consumer HMDs such as the Oculus Quest require the users to setup an empty physical space as their “play area” to be able to physically move around within that space, as required by the mechanics of the app. As such, future design of remote iVR experience could include richer forms of interactions that go beyond a stationary mode. In the context of nutrition education, this could be enabling users to physically browse a virtual buffet or supermarket and naturally interact with virtual food items.
Our study, albeit preliminary, suggests that interactivity only affects one measure of perceived learning experience. The comparisons reported in Table 3 show an interesting but somewhat contradictory trend. While the self-reported mean score for most of the measures are higher in the interactive condition, we see that perceived ease of use, presence, perceived learning effectiveness, and motivation are marginally higher in the passive condition (albeit statistically non-significant). A larger sample size would help future studies in teasing apart these trends. However, interactivity was the only dimension of iVR that was manipulated in this study. While it could show a strong effect in relation to immediacy of control, it may not drastically affect other measures of the perceived learning experience. Other fundamental characteristics of iVR, such as presence and immersion could potentially be better predictors for perceived ease of use, presence, perceived learning effectiveness, and motivation. As such, more research is needed to gain a deeper understanding of what affordances and design choices in educational iVR applications predict the learning outcomes and experiences of users.
It is also important to note that the measures we have used for perceived learning experience in the context of this research, only reflect the subjective opinion of the participants in terms of how they feel or think their experience is affected. As such, further research, with more objective measures of learning experience should be performed in the future. Furthermore, as the nature of this research was exploratory, subsequent studies with pre-planned hypotheses should be conducted to confirm the suggested effect of interactivity on learning experience. In addition, to better understand the implications of our findings, qualitative analyses of post-experiment interviews and open-ended questions could prove to be useful. In light of this and as part of the future direction of this research, we are planning on utilizing a mixed-methods approach.
Furthermore, while the current study suggests the efficacy of iVR for nutrition-related education, and the suggestive partial role of interactivity on perceived learning experience, it falls short in investigating the ultimate desired effect of iVR—leaving a long lasting effect. Therefore, as part of the future direction of this research, using a remote longitudinal study we will be examining the extent to which learning in iVR is transferable to the real-world as well as its potential in inducing a long lasting change in the behavior of people. Such a study could also include physical behavioral data such as positional and orientations tracking of the headset and controllers, as well as performance data such as frequency of interaction with different virtual objects, success rate, repetitions, etc. to better understand the experiences of users in interactive educational iVR experiences.
Data Availability Statement
The raw data supporting the conclusions of this article will be made available by the authors, without undue reservation.
Ethics Statement
This study was reviewed and approved by the IRB of Penn State. The participants provided their written informed consent to participate in this study.
Author Contributions
PS, CE, AK, and TM: design and methodology. PS, CE, and TM: formal analysis and investigation. CE: resources. JZ, CE, PS, and TM: data collection. AF, PS, CE, AK, and TM: conceptualization. AF: software. JL, JZ, CE, PS, AK, and TM: writing. All authors contributed to the article and approved the submitted version.
Conflict of Interest
The authors declare that the research was conducted in the absence of any commercial or financial relationships that could be construed as a potential conflict of interest.
Publisher's Note
All claims expressed in this article are solely those of the authors and do not necessarily represent those of their affiliated organizations, or those of the publisher, the editors and the reviewers. Any product that may be evaluated in this article, or claim that may be made by its manufacturer, is not guaranteed or endorsed by the publisher.
Footnotes
1. ^https://www.xrtoday.com/virtual-reality/virtual-reality-statistics-2022/
5. ^https://www.oculus.com/quest-2/
12. ^https://www.vive.com/us/product/vive-pro-eye/overview/
13. ^https://www.hp.com/us-en/vr/reverb-g2-vr-headset-omnicept-edition.html
References
Ahn, S. J. G., Bailenson, J. N., and Park, D. (2014). Short-and long-term effects of embodied experiences in immersive virtual environments on environmental locus of control and behavior. Comput. Hum. Behav. 39, 235–245. doi: 10.1016/j.chb.2014.07.025
Alhalabi, W. (2016). Virtual reality systems enhance students achievements in engineering education. Behav. Inform. Technol. 35, 919–925. doi: 10.1080/0144929X.2016.1212931
Andreasen, M. S., Nielsen, H. V., Schrøder, S. O., and Stage, J. (2007). “What happened to remote usability testing? An empirical study of three methods,” in Proceedings of the SIGCHI Conference on Human Factors in Computing Systems (New York, NY), 1405–1414. doi: 10.1145/1240624.1240838
Bailenson, J. (2018). Experience on Demand: What Virtual Reality Is, How It Works, and What It Can Do. New York City, NY: WW Norton & Company.
Blascovich, J., Loomis, J., Beall, A. C., Swinth, K. R., Hoyt, C. L., and Bailenson, J. N. (2002). Immersive virtual environment technology as a methodological tool for social psychology. Psychol. Inq. 13, 103–124. doi: 10.1207/S15327965PLI1302_01
Brug, J., Campbell, M., and van Assema, P. (1999). The application and impact of computer-generated personalized nutrition education: a review of the literature. Patient Educ. Counsel. 36, 145–156. doi: 10.1016/S0738-3991(98)00131-1
Brug, J., Oenema, A., and Campbell, M. (2003). Past, present, and future of computer-tailored nutrition education. Am. J. Clin. Nutr. 77, 1028S–1034S. doi: 10.1093/ajcn/77.4.1028S
Brunstrom, J. M., Rogers, P. J., Pothos, E. M., Calitri, R., and Tapper, K. (2008). Estimating everyday portion size using a “method of constant stimuli”: in a student sample, portion size is predicted by gender, dietary behaviour, and hunger, but not bmi. Appetite 51, 296–301. doi: 10.1016/j.appet.2008.03.005
Buhrmester, M., Kwang, T., and Gosling, S. D. (2011). Amazon's mechanical turk: A new source of inexpensive, yet high-quality, data? Pers. Psychol. Sci. 6, 3–5. doi: 10.1177/1745691610393980
Cheah, C. S., Barman, S., Vu, K. T., Jung, S. E., Mandalapu, V., Masterson, T. D., et al. (2020). Validation of a virtual reality buffet environment to assess food selection processes among emerging adults. Appetite 153, 104741. doi: 10.1016/j.appet.2020.104741
Cheah, C. S., Kaputsos, S. P., Mandalapu, V., Tran, T., Barman, S., Jung, S. E., et al. (2019). “Neurophysiological variations in food decision-making within virtual and real environments,” in 2019 IEEE EMBS International Conference on Biomedical & Health Informatics (BHI) (IEEE), 1–4. doi: 10.1109/BHI.2019.8834497
Chen, J., Gemming, L., Hanning, R., and Allman-Farinelli, M. (2018). Smartphone apps and the nutrition care process: current perspectives and future considerations. Patient Educ. Counsel. 101, 750–757. doi: 10.1016/j.pec.2017.11.011
Chittaro, L., and Buttussi, F. (2015). Assessing knowledge retention of an immersive serious game vs. a traditional education method in aviation safety. IEEE Trans. Visual. Comput. Graph. 21, 529–538. doi: 10.1109/TVCG.2015.2391853
Damiani, L., Demartini, M., Guizzi, G., Revetria, R., and Tonelli, F. (2018). Augmented and virtual reality applications in industrial systems: a qualitative review towards the industry 4.0 era. IFAC Pap. Online 51, 624–630. doi: 10.1016/j.ifacol.2018.08.388
DiFilippo, K. N., Huang, W.-H., Andrade, J. E., and Chapman-Novakofski, K. M. (2015). The use of mobile apps to improve nutrition outcomes: a systematic literature review. J. Telemed. Telecare 21, 243–253. doi: 10.1177/1357633X15572203
Edwards, C. G., Sajjadi, P., Fatemi, A., Krieger, E. N., Klippel, A., and Masterson, T. D. (2022). The immersive virtual alimentation and nutrition application: an interactive digital dietitian. J. Nutr. Educ. Behav. 54, 481–484. doi: 10.1016/j.jneb.2022.02.014
Fast, L. C., Harman, J. J., Maertens, J. A., Burnette, J. L., and Dreith, F. (2015). Creating a measure of portion control self-efficacy. Eat. Behav. 16, 23–30. doi: 10.1016/j.eatbeh.2014.10.009
Fisher, J. O., Arreola, A., Birch, L. L., and Rolls, B. J. (2007). Portion size effects on daily energy intake in low-income hispanic and African American children and their mothers. Am. J. Clin. Nutr. 86, 1709–1716. doi: 10.1093/ajcn/86.5.1709
Fryar, C. D., Carroll, M. D., and Afful, J. (2020). Prevalence of Overweight, Obesity, and Severe Obesity Among Adults Aged 20 and Over: United states, 1960-1962 Through 2017-2018. NCHS Health E-Stats.
Garcia, A. D., Schlueter, J., and Paddock, E. (2020). “Training astronauts using hardware-in-the-loop simulations and virtual reality,” in AIAA Scitech 2020 Forum, 0167. doi: 10.2514/6.2020-0167
Germine, L., Nakayama, K., Duchaine, B. C., Chabris, C. F., Chatterjee, G., and Wilmer, J. B. (2012). Is the web as good as the lab? Comparable performance from web and lab in cognitive/perceptual experiments. Psychon. Bull. Rev. 19, 847–857. doi: 10.3758/s13423-012-0296-9
Gorini, A., Griez, E., Petrova, A., and Riva, G. (2010). Assessment of the emotional responses produced by exposure to real food, virtual food and photographs of food in patients affected by eating disorders. Ann. Gen. Psychiatry 9, 1–10. doi: 10.1186/1744-859X-9-30
Gustarini, M., Ickin, S., and Wac, K. (2013). “Evaluation of challenges in human subject studies “in-the-wild” Using subjects' personal smartphones,” in Proceedings of the 2013 ACM Conference on Pervasive and Ubiquitous Computing Adjunct Publication (New York, NY), 1447–1456. doi: 10.1145/2494091.2496041
Hein, G. (1991). Constructivist Learning Theory. Institute for Inquiry. Available online at: https://www.exploratorium.edu/ifi/resources/constructivistlearning.html.
Henze, N., Pielot, M., Poppinga, B., Schinke, T., and Boll, S. (2011). My app is an experiment: Experience from user studies in mobile app stores. Int. J. Mobile Hum. Comput. Interact. 3, 71–91. doi: 10.4018/jmhci.2011100105
Hingle, M., and Patrick, H. (2016). There are thousands of apps for that: navigating mobile technology for nutrition education and behavior. J. Nutr. Educ. Behav. 48, 213–218. doi: 10.1016/j.jneb.2015.12.009
Huang, H.-M., Rauch, U., and Liaw, S.-S. (2010). Investigating learners? attitudes toward virtual reality learning environments: based on a constructivist approach. Comput. Educ. 55, 1171–1182. doi: 10.1016/j.compedu.2010.05.014
Huang, J., Lucash, M. S., Scheller, R. M., and Klippel, A. (2021). Walking through the forests of the future: using data-driven virtual reality to visualize forests under climate change. Int. J. Geograph. Inform. Sci. 35, 1155–1178. doi: 10.1080/13658816.2020.1830997
Huber, B., and Gajos, K. Z. (2020). Conducting online virtual environment experiments with uncompensated, unsupervised samples. PLoS ONE 15, e0227629. doi: 10.1371/journal.pone.0227629
Hunot, C., Fildes, A., Croker, H., Llewellyn, C. H., Wardle, J., and Beeken, R. J. (2016). Appetitive traits and relationships with BMI in adults: development of the adult eating behaviour questionnaire. Appetite 105, 356–363. doi: 10.1016/j.appet.2016.05.024
Kourtesis, P., Korre, D., Collina, S., Doumas, L. A., and MacPherson, S. E. (2020). Guidelines for the development of immersive virtual reality software for cognitive neuroscience and neuropsychology: the development of virtual reality everyday assessment lab (VR-EAL), a neuropsychological test battery in immersive virtual reality. Front. Comput. Sci. 1, 12. doi: 10.3389/fcomp.2019.00012
Kral, T. V., and Rolls, B. J. (2004). Energy density and portion size: their independent and combined effects on energy intake. Physiol. Behav. 82, 131–138. doi: 10.1016/j.physbeh.2004.04.063
Krokos, E., Plaisant, C., and Varshney, A. (2019). Virtual memory palaces: immersion aids recall. Virtual Real. 23, 1–15. doi: 10.1007/s10055-018-0346-3
Lamb, R., Antonenko, P., Etopio, E., and Seccia, A. (2018). Comparison of virtual reality and hands on activities in science education via functional near infrared spectroscopy. Comput. Educ. 124, 14–26. doi: 10.1016/j.compedu.2018.05.014
Ledoux, T., Nguyen, A. S., Bakos-Block, C., and Bordnick, P. (2013). Using virtual reality to study food cravings. Appetite 71, 396–402. doi: 10.1016/j.appet.2013.09.006
Lee, E. A.-L., Wong, K. W., and Fung, C. C. (2010). How does desktop virtual reality enhance learning outcomes? A structural equation modeling approach. Comput. Educ. 55, 1424–1442. doi: 10.1016/j.compedu.2010.06.006
Lee, L. -H., Braud, T., Zhou, P., Wang, L., Xu, D., Lin, Z., et al. (2021). All one needs to know about metaverse: A complete survey on technological singularity, virtual ecosystem, and research agenda. arXiv [Preprint]. arXiv: 2110.05352. doi: 10.48550/arxiv.2110.05352
Liu, X., Zhang, J., Hou, G., and Wang, Z. (2018). “Virtual reality and its application in military,” in IOP Conference Series: Earth and Environmental Science (IOP Publishing), 032155. doi: 10.1088/1755-1315/170/3/032155
Lombart, C., Millan, E., Normand, J.-M., Verhulst, A., Labbé-Pinlon, B., and Moreau, G. (2019). Consumer perceptions and purchase behavior toward imperfect fruits and vegetables in an immersive virtual reality grocery store. J. Retail. Consumer Serv. 48, 28–40. doi: 10.1016/j.jretconser.2019.01.010
Ma, X., Cackett, M., Park, L., Chien, E., and Naaman, M. (2018). “Web-based VR experiments powered by the crowd,” in Proceedings of the 2018 World Wide Web Conference. doi: 10.1145/3178876.3186034
Majumdar, D., Koch, P., Lee, H., Contento, I., Islas, A., and Fu, D. (2012). “Creature-101”: using a virtual reality, serious game to promote healthy eating and physical activity behaviors among middle school students. J. Nutr. Educ. Behav. 44, S38. doi: 10.1016/j.jneb.2012.03.078
Makransky, G., Terkildsen, T. S., and Mayer, R. E. (2019). Adding immersive virtual reality to a science lab simulation causes more presence but less learning. Learn. Instruct. 60, 225–236. doi: 10.1016/j.learninstruc.2017.12.007
Markowitz, D. M., Laha, R., Perone, B. P., Pea, R. D., and Bailenson, J. N. (2018). Immersive virtual reality field trips facilitate learning about climate change. Front. Psychol. 9, 2364. doi: 10.3389/fpsyg.2018.02364
Mason, W., and Suri, S. (2012). Conducting behavioral research on Amazon?s mechanical turk. Behav. Res. Methods 44, 1–23. doi: 10.3758/s13428-011-0124-6
McGuirt, J. T., Cooke, N. K., Burgermaster, M., Enahora, B., Huebner, G., Meng, Y., et al. (2020). Extended reality technologies in nutrition education and behavior: comprehensive scoping review and future directions. Nutrients 12, 2899. doi: 10.3390/nu12092899
Mikhail, D., Rolls, B., Yost, K., Balls-Berry, J., Gall, M., Blixt, K., et al. (2020). Development and validation testing of a weight management nutrition knowledge questionnaire for adults. Int. J. Obesity 44, 579–589. doi: 10.1038/s41366-019-0510-1
Miller, M. R., Herrera, F., Jun, H., Landay, J. A., and Bailenson, J. N. (2020). Personal identifiability of user tracking data during observation of 360-degree VR video. Sci. Rep. 10, 1–10. doi: 10.1038/s41598-020-74486-y
Mottelson, A., and Hornbæk, K. (2016). “An affect detection technique using mobile commodity sensors in the wild,” in Proceedings of the 2016 ACM International Joint Conference on Pervasive and Ubiquitous Computing (New York, NY), 781–792. doi: 10.1145/2971648.2971654
Mottelson, A., and Hornbæk, K. (2017). “Virtual reality studies outside the laboratory,” in Proceedings of the 23rd ACM Symposium on Virtual Reality Software and Technology (New York, NY), 1–10. doi: 10.1145/3139131.3139141
Mottelson, A., Petersen, G. B., Lilija, K., and Makransky, G. (2021). Conducting unsupervised virtual reality user studies online. Front. Virtual Real. 2, 66. doi: 10.3389/frvir.2021.681482
Oenema, A., Brug, J., and Lechner, L. (2001). Web-based tailored nutrition education: results of a randomized controlled trial. Health Educ. Res. 16, 647–660. doi: 10.1093/her/16.6.647
Onyesolo, M., Nwasor, V., Ositanwosu, O., and Iwegbuna, O. (2013). Pedagogy: Instructivism to socio-constructivism through virtual reality. Int. J. Adv. Comput. Sci. Appl. 4. doi: 10.14569/IJACSA.2013.040907
Paolacci, G., and Chandler, J. (2014). Inside the turk: understanding mechanical turk as a participant pool. Curr. Direct. Psychol. Sci. 23, 184–188. doi: 10.1177/0963721414531598
Parsons, T. D., and Rizzo, A. A. (2008). Affective outcomes of virtual reality exposure therapy for anxiety and specific phobias: a meta-analysis. J. Behav. Therapy Exp. Psychiatry 39, 250–261. doi: 10.1016/j.jbtep.2007.07.007
Peck, T. C., Sockol, L. E., and Hancock, S. M. (2020). Mind the gap: the underrepresentation of female participants and authors in virtual reality research. IEEE Trans. Visual. Comput. Graph. 26, 1945–1954. doi: 10.1109/TVCG.2020.2973498
Persky, S., Goldring, M. R., Turner, S. A., Cohen, R. W., and Kistler, W. D. (2018). Validity of assessing child feeding with virtual reality. Appetite 123, 201–207. doi: 10.1016/j.appet.2017.12.007
Radianti, J., Majchrzak, T. A., Fromm, J., and Wohlgenannt, I. (2020). A systematic review of immersive virtual reality applications for higher education: design elements, lessons learned, and research agenda. Comput. Educ. 147, 103778. doi: 10.1016/j.compedu.2019.103778
Ratcliffe, J., Soave, F., Bryan-Kinns, N., Tokarchuk, L., and Farkhatdinov, I. (2021a). “Extended reality (XR) remote research: a survey of drawbacks and opportunities,” in Proceedings of the 2021 CHI Conference on Human Factors in Computing Systems (New York, NY), 1–13. doi: 10.1145/3411764.3445170
Ratcliffe, J., Soave, F., Hoover, M., Ortega, F. R., Bryan-Kinns, N., Tokarchuk, L., et al. (2021b). “Remote XR studies: exploring three key challenges of remote XR experimentation,” in Extended Abstracts of the 2021 CHI Conference on Human Factors in Computing Systems (New York, NY), 1–4. doi: 10.1145/3411763.3442472
Raynor, H. A., and Champagne, C. M. (2016). Position of the academy of nutrition and dietetics: interventions for the treatment of overweight and obesity in adults. J. Acad. Nutr. Diet. 116, 129–147. doi: 10.1016/j.jand.2015.10.031
Reinecke, K., and Gajos, K. Z. (2015). “Labinthewild: conducting large-scale online experiments with uncompensated samples,” in Proceedings of the 18th ACM Conference on Computer Supported Cooperative Work & Social Computing (New York, NY), 1364–1378. doi: 10.1145/2675133.2675246
Reips, U. D. (2000). “The web experiment method: Advantages, disadvantages, and solutions,” in Psychological Experiments on the Internet, ed M. H. Birnbaum (San Diego, CA: Academic Press), 89–118. doi: 10.5167/uzh-19760
Rivu, R., Mäkelä, V., Prange, S., Rodriguez, S. D., Piening, R., Zhou, Y., et al. (2021). Remote VR Studies: A Framework for Running Virtual Reality Studies Remotely Via Participant-Owned HMDs. New York, NY: Association for Computing Machinery. doi: 10.1145/3472617
Roe, L. S., Kling, S. M., and Rolls, B. J. (2016). What is eaten when all of the foods at a meal are served in large portions? Appetite 99, 1–9. doi: 10.1016/j.appet.2016.01.001
Rolls, B. J., Morris, E. L., and Roe, L. S. (2002). Portion size of food affects energy intake in normal-weight and overweight men and women. Am. J. Clin. Nutr. 76, 1207–1213. doi: 10.1093/ajcn/76.6.1207
Rolls, B. J., Roe, L. S., James, B. L., and Sanchez, C. E. (2017). Does the incorporation of portion-control strategies in a behavioral program improve weight loss in a 1-year randomized controlled trial? Int. J. Obesity 41, 434–442. doi: 10.1038/ijo.2016.217
Rouse, S. V. (2015). A reliability analysis of mechanical turk data. Comput. Hum. Behav. 43, 304–307. doi: 10.1016/j.chb.2014.11.004
Ruppert, B. (2011). New directions in the use of virtual reality for food shopping: marketing and education perspectives. J. Diabetes Sci. Technol. 5, 315–318. doi: 10.1177/193229681100500217
Saffo, D., Di Bartolomeo, S., Yildirim, C., and Dunne, C. (2021). “Remote and collaborative virtual reality experiments via social VR platforms,” in Proceedings of the 2021 CHI Conference on Human Factors in Computing Systems, 1–15. doi: 10.1145/3411764.3445426
Saffo, D., Yildirim, C., Di Bartolomeo, S., and Dunne, C. (2020). “Crowdsourcing virtual reality experiments using VRchat,” in Extended Abstracts of the 2020 CHI Conference on Human Factors in Computing Systems (New York, NY), 1–8. doi: 10.1145/3334480.3382829
Sajjadi, P., Hoffmann, L., Cimiano, P., and Kopp, S. (2019). A personality-based emotional model for embodied conversational agents: effects on perceived social presence and game experience of users. Entertain. Comput. 32, 100313. doi: 10.1016/j.entcom.2019.100313
Siegrist, M., Ung, C.-Y., Zank, M., Marinello, M., Kunz, A., Hartmann, C., et al. (2019). Consumers' food selection behaviors in three-dimensional (3D) virtual reality. Food Res. Int. 117, 50–59. doi: 10.1016/j.foodres.2018.02.033
Siu, K.-C., Best, B. J., Kim, J. W., Oleynikov, D., and Ritter, F. E. (2016). Adaptive virtual reality training to optimize military medical skills acquisition and retention. Military Med. 181(Suppl_5), 214–220. doi: 10.7205/MILMED-D-15-00164
Spence, M., Lähteenmäki, L., Stefan, V., Livingstone, M., Gibney, E., and Dean, M. (2015). Quantifying consumer portion control practices. A cross-sectional study. Appetite 92, 240–246. doi: 10.1016/j.appet.2015.05.035
Steed, A., Frlston, S., Lopez, M. M., Drummond, J., Pan, Y., and Swapp, D. (2016). An “in the wild” experiment on presence and embodiment using consumer virtual reality equipment. IEEE Trans. Visual. Comput. Graph. 22, 1406–1414. doi: 10.1109/TVCG.2016.2518135
Steed, A., Ortega, F. R., Williams, A. S., Kruijff, E., Stuerzlinger, W., Batmaz, A. U., et al. (2020). Evaluating immersive experiences during COVID-19 and beyond. Interactions 27, 62–67. doi: 10.1145/3406098
Stewart, N., Ungemach, C., Harris, A. J., Bartels, D. M., Newell, B. R., Paolacci, G., et al. (2015). The average laboratory samples a population of 7,300 Amazon mechanical turk workers. J. Decis. Mak. 10, 479–491.
Stunkard, A. J., and Messick, S. (1985). The three-factor eating questionnaire to measure dietary restraint, disinhibition and hunger. J. Psychosom. Res. 29, 71–83. doi: 10.1016/0022-3999(85)90010-8
Sullivan, D. K., Goetz, J. R., Gibson, C. A., Washburn, R. A., Smith, B. K., Lee, J., et al. (2013). Improving weight maintenance using virtual reality (second life). J. Nutr. Educ. Behav. 45, 264–268. doi: 10.1016/j.jneb.2012.10.007
Tallon, J. M., Saavedra Dias, R., Costa, A. M., Leitao, J., Barros, A., Rodrigues, V., et al. (2021). Impact of technology and school-based nutrition education programs on nutrition knowledge and behavior during adolescence–a systematic review. Scand. J. Educ. Res. 65, 169–180. doi: 10.1080/00313831.2019.1659408
Thompson, F. E., and Subar, A. F. (2017). “Dietary assessment methodology,” in Nutrition in the Prevention and Treatment of Disease, 5–48. doi: 10.1016/B978-0-12-802928-2.00001-1
U.S. Department of Agriculture and U.S. Department of Health and Human Services (2020). Dietary Guidelines for Americans, 2020-2025. Available online at: dietaryguidelines.gov
Ung, C.-Y., Menozzi, M., Hartmann, C., and Siegrist, M. (2018). Innovations in consumer research: the virtual food buffet. Food Qual. Prefer. 63, 12–17. doi: 10.1016/j.foodqual.2017.07.007
van der Waal, N. E., Janssen, L., Antheunis, M., Culleton, E., and van der Laan, L. N. (2021). The appeal of virtual chocolate: a systematic comparison of psychological and physiological food cue responses to virtual and real food. Food Qual. Prefer. 90, 104167. doi: 10.1016/j.foodqual.2020.104167
Vermeer, W. M., Steenhuis, I., and Poelman, M. (2014). Small, medium, large or supersize? The development and evaluation of interventions targeted at portion size. Int. J. Obesity 38, S13?S18. doi: 10.1038/ijo.2014.84
Webster, R. (2016). Declarative knowledge acquisition in immersive virtual learning environments. Int. Learn. Environ. 24, 1319–1333. doi: 10.1080/10494820.2014.994533
West, J. H., Belvedere, L. M., Andreasen, R., Frandsen, C., Hall, P. C., and Crookston, B. T. (2017). Controlling your “app” etite: how diet and nutrition-related mobile apps lead to behavior change. JMIR mHealth uHealth 5, e7410. doi: 10.2196/mhealth.7410
Wilson, C. J., and Soranzo, A. (2015). The use of virtual reality in psychology: a case study in visual perception. Comput. Math. Methods Med. 2015, 151702. doi: 10.1155/2015/151702
Wu, B., Yu, X., and Gu, X. (2020). Effectiveness of immersive virtual reality using head-mounted displays on learning performance: a meta-analysis. Brit. J. Educ. Technol. 51, 1991–2005. doi: 10.1111/bjet.13023
Zhao, J., LaFemina, P., Carr, J., Sajjadi, P., Wallgrün, J. O., and Klippel, A. (2020). “Learning in the field: comparison of desktop, immersive virtual reality, and actual field trips for place-based stem education,” in 2020 IEEE Conference on Virtual Reality and 3D User Interfaces (VR) (IEEE), 893–902. doi: 10.1109/VR46266.2020.00012
Zhao, J., Simpson, M., Sajjadi, P., Wallgrün, J. O., Li, P., Bagher, M. M., et al. (2021). “CrowdXR-pitfalls and potentials of experiments with remote participants,” in 2021 IEEE International Symposium on Mixed and Augmented Reality (ISMAR) (IEEE), 450–459. doi: 10.1109/ISMAR52148.2021.00062
Keywords: Virtual Reality, remote study, nutrition, education, interactivity, remote iVR
Citation: Sajjadi P, Edwards CG, Zhao J, Fatemi A, Long JW, Klippel A and Masterson TD (2022) Remote iVR for Nutrition Education: From Design to Evaluation. Front. Comput. Sci. 4:927161. doi: 10.3389/fcomp.2022.927161
Received: 23 April 2022; Accepted: 09 June 2022;
Published: 29 June 2022.
Edited by:
Liang Men, Liverpool John Moores University, United KingdomReviewed by:
Yue Li, Xi'an Jiaotong-Liverpool University, ChinaNathaniel D. Porter, Virginia Tech, United States
Copyright © 2022 Sajjadi, Edwards, Zhao, Fatemi, Long, Klippel and Masterson. This is an open-access article distributed under the terms of the Creative Commons Attribution License (CC BY). The use, distribution or reproduction in other forums is permitted, provided the original author(s) and the copyright owner(s) are credited and that the original publication in this journal is cited, in accordance with accepted academic practice. No use, distribution or reproduction is permitted which does not comply with these terms.
*Correspondence: Pejman Sajjadi, c2ZzNTkxOUBwc3UuZWR1