- 1Department of Computer Science, University of Rochester, Rochester, NY, United States
- 2Faculty of Economics and Management, East China Normal University, Shanghai, China
While the long-term effects of the COVID-19 pandemic have yet to be determined, its immediate impact on crowdfunding is nonetheless significant. This study adopts a computational approach to better understanding this consequence. We aim to gain insight into whether and how the COVID-19 pandemic has changed crowdfunding. Using a unique dataset of all GoFundMe campaigns published over the past 2 years, we explore the factors that have led to successfully funded crowdfunding projects. In particular, we study a corpus of 36,370 projects from November 2018 to December 2020 by analyzing cover images and other attributes commonly found on crowdfunding sites. We first construct a classifier and a regression model to assess the importance of features based on XGBoost. Next, we employ counterfactual analysis to investigate the causality between features and the success of crowdfunding. Furthermore, sentiment analysis and paired sample t-tests are performed to examine differences in crowdfunding campaigns before and after the COVID-19 outbreak in March 2020. Findings suggest a significant racial disparity in crowdfunding success. In addition, sad emotions expressed in a campaign's description became significant after the COVID-19 outbreak. This study enriches our understanding of the impact of the COVID-19 pandemic on crowdfunding as well as the prevalence of discrimination in crowdfunding.
1. Introduction
The development of the Internet has introduced more ways to raise money online in recent years. GoFundMe, an American for-profit crowdfunding platform that encourages people to create online crowdfunding projects for life events such as illnesses and accidents, is a prime example. Despite the increasing convenience of crowdfunding, campaigns' success rates remain low. Yet little is known about the “recipe” for a successful campaign; as such, uncovering the factors contributing to successful crowdfunding constitutes a key research aim (Kaartemo, 2017).
Amid the COVID-19 pandemic, GoFundMe has become a powerful online platform through which people can raise or donate money. This digitally enabled process has largely replaced offline crowdfunding. Researchers have thus explored the factors influencing crowdfunding success during the COVID-19 pandemic. The number of coronavirus-related campaigns soared on platforms such as GoFundMe between early and mid of March 2020 (Cadogan, 2021). Saleh et al. (2021) found that COVID-19 related campaigns received more donations than non-COVID-19 related campaigns. These campaigns had a longer description and were more likely to be shared on social media platforms such as Facebook than other campaigns. Rajwa et al. (2020) considered the responsiveness of online crowdfunding during the pandemic. However, neither study compared features contributing to campaigns' success before and after the outbreak. The authors also did not investigate the potential causality and associations among relevant features.
In this paper, we define a crowdfunding project as successful when the amount raised is greater than the target amount. We employ XGBoost to assess features' importance. Moreover, target amounts are classified and regressed. We use XGBoost to resolve regression or classification problems and provide a sequence of important factors. Finally, we perform a counterfactual experiment to analyze the influence of each factor and the impact of the COVID-19 pandemic on these features. To the best of our knowledge, this study is the first to investigate the impact of the COVID-19 pandemic on crowdfunding campaigns.
2. Literature Review
Studies have begun to address the impact of the COVID-19 pandemic on crowdfunding. Farhoud et al. (2021) sought to understand the effect of the COVID-19 pandemic on social enterprise crowdfunding and outlined implications for crowdfunding platforms. The authors discovered that social entrepreneurs' crowdfunding success rates reflected the nature of the campaigns and innovative ideas. Song et al. (2020) compared patient and campaign characteristics between 250 users of complementary and alternative medicine (CAM) and 250 non-CAM users. They observed that CAM users were more likely to be women and to report more stage IV cancer. In addition, Elmer et al. (2020) noted that campaigns related to COVID-19 were likely to raise more money and had more attractive descriptions than other types of campaigns.
Most empirical studies on GoFundMe have involved the medical setting. For example, Mattingly et al. (2021) applied descriptive statistics about campaign categories and features to uncover potential associations among features. Notably, they found that disclosing the virus source contributes to higher donations. Radu and McManus (2018) determined that victims of intimate partner violence preferred to seek assistance from informal social ties rather than official organizations. Their findings conveyed the challenges of obtaining help through traditional avenues. In order to build a more accurate prediction model, some researchers apply deep learning and machine learning methods to analyze latent relationship between potential factors and goals in detail (Valaskova et al., 2021a,b).
Much of the literature on crowdfunding suffers from several limitations. For example, most studies have referred to visible website features when determining crowdfunding success. More importantly, to our best knowledge, no study has examined the impact of the COVID-19 pandemic on crowdfunding using large-scale data.
3. Hypothesis
Life course theory explains individuals' development over time as a function of internal forces (agency) and external influences (e.g., time and place) with an emphasis on the social and historical trajectories that influence one's life course (Giele and Elder, 1998). The life course refers to social patterns in the timing, duration, spacing, and order of events and roles. Individual time, also called ontogenetic time, is based on a person's chronological age; this concept also assumes that periods of life (including childhood, adolescence, adulthood, and old age) affect a person's social positions, roles, and rights (Binstock et al., 2011). In contrast, generational time draws attention to the experiences of groups or cohorts of people based on age. For instance, many countries experienced a “baby boom”— a faster-than-expected increase in birth rates between 1946 and 1964 after World War II (Rice et al., 2011). Whereas, Baby Boomers' consumer behavior has been researched extensively over the last 70 years, scholars have paid less attention to other cohorts.
Another principle of the life course theory posits that individuals' behavior can change due to geopolitical events (e.g., war), geopolitical events (e.g., war), and economic cycles (e.g., recessions) because people and families interact within sociohistorical time. For instance, consumers' attitudes are likely to be affected by economic up- and down-swings (Katona, 1974). Also, Igra et al. (2021) examined increasing inequities on crowdfunding platforms during the first months of the COVID-19 pandemic. They found that wealthier counties receive more donations than less wealthy counties. We argue that the COVID-19 pandemic has altered people's decisions and behavior and has formed some social disparities (create logical motivations for H3).
In the current study, we analyze GoFundMe campaigns to extract critical aspects contributing to crowdfunding success. We also consider whether relevant influencing factors have changed against the backdrop of the pandemic. Some scholars have contended that emotional elements conveyed through text and facial expressions are likely to attract donors (Rhue and Robert, 2018). We hence extract faces from pictures and judge the displayed emotion using Baidu's Application Programming Interface (API)1. In addition, we extract and infer individual campaign-level features such as gender, race, age, beauty, target, location, followers, shares, distinct donors, family status, facial attractiveness, and crowdfunding duration. Text is a fundamental element of information transfer; research suggests that textual features, including descriptions, reviews, and emotion, heavily mold crowdfunding's success (Koch and Siering, 2019). Therefore, we incorporate text emotion into models through a text scoring model that produces the scores as a feature. In-depth analysis can then be performed based on the aforementioned characteristics. If a campaign page visitor sympathizes with certain project aspects, a longer visit duration increases the probability of a personal donation and hence successful crowdfunding (Koch and Siering, 2019). The aesthetic and technical scores of the cover image are also thought to affect campaigns' success (Zhang et al., 2020). In light of the preceding discussion, we propose three hypotheses:
• Hypothesis 1: The basic features of a crowdfunding project and description significantly affect fund-raising success.
• Hypothesis 2: Crowdfunding differs significantly between before and after the COVID-19 outbreak.
• Hypothesis 3: Social disparities in crowdfunding success (in terms of race and compound factors) reflect the impacts of the COVID-19 pandemic and other social factors.
4. Methodology
4.1. Data Sets
We focus on GoFundMe to analyze crucial factors contributing to campaigns' success. Specifically, we crawl the 36,370 crowdfunding campaigns on GoFundMe and divide them into two parts: those collected before August 2019 and after August 2020. This division was intended to indicate whether the COVID-19 pandemic has affected people's attitudes toward crowdfunding along with whether and how influential factors have changed. The dataset features are summarized below and shown in Table 1.
4.2. Basic Features
The following campaign features can be directly extracted from the GoFundMe website: launch date, cover image, description, category, current amount, target amount, number of followers, number of shares, and number of donors. We refer to these features as the basic features.
4.3. Inferred Features
• Quality Scores: We use the pre-trained model of neural image assessment (NIMA) to obtain the aesthetic and technical scores of each cover image (Talebi and Milanfar, 2018).
• Text Features: First, we merge campaigns' titles and descriptions and use them as text data. Next, we employ the Valence Aware Dictionary for Sentiment Reasoning (VADER) (Hutto and Gilbert, 2014) to evaluate individual text data and obtain three predicted emotion scores: positive, negative, and neutral. Finally, we examine the text using the Linguistic Inquiry and Word Count (LIWC) program to obtain more detailed text sentiment scores (i.e., sadness, anger, and anxiety). Both campaign descriptions are essential to successful crowdfunding. These potential effects are difficult to measure directly; therefore, we train an XLNet model to predict a campaign's success and discern its potential effects (Yang et al., 2019).
• Image Features: In terms of image features, we compare the DeepFace API (Serengil and Ozpinar, 2020) with the Baidu API and consider research comparing the Baidu API with competing APIs (Yang et al., 2020). We eventually opt to use Baidu's API, which provides reliable face recognition services. This API returns the facial attractiveness, age, race, emotion, and gender of each face in a cover image (also referred to as the profile image). For simplicity, we calculate the mean attractiveness of faces when an image contains more than one person. In terms of age, we calculate the mean age as well as the number of children and number of older adults among pictured people. People under age 15 are considered children for this purpose, whereas those over age 60 are older adults. We regard the number of people of different races as the characteristics variable. Baidu's API recognizes four races: Black, White, Asian, and Other. We obtain the number of men and women to classify gender. We also extract the emotion of each face (happy, sad, grimace, neutral, or angry).
4.4. Campaign Category
We identified 21 categories of campaigns and their distributions before and after COVID-19, depicted in Figure 1. Some categories are related to campaigns' success rates, while others are not. Specifically, we analyze categories with the most significant effects on crowdfunding success via t-tests. The impacts of certain categories on crowdfunding success have changed over the past 2 years. As it would be illogical to compare p-values directly, we compare whether categories' significance levels have changed (Table 2); for example, those of the Travel & Adventure, Non-Profits & Charities, Medical, Illness & Healing, Celebrations & Events, Creative Arts, Music & Film, Accidents & Emergencies, and Dreams, Hops & Wishes categories have shifted. These categories' success ratios have also increased, except for Travel & Adventure category. People appear even more reluctant to donate to campaigns in the Travel & Adventure category since the COVID-19 outbreak. In particular, the Medical, Illness & Healing and Non-Profits & Charities categories were not significant before the COVID-19 outbreak but have become highly significant thereafter. Regarding the Dreams, Hops & Wishes category, people who had been more willing to donate are now reluctant. For the other declining categories, among most of those to which people were unwilling to donate before, people have become even more hesitant to contribute(e.g, Sports, Teams & Clubs; Missions, Faith & Church; Weddings & Honeymoons; Competitions & Pageants). Of note, every category's success rate has declined since the COVID-19 outbreak.
4.5. Prediction
To analyze the contributions of influential factors to campaigns' success, we employ the XGBoost method and divide features among basic features, text features, and image features. We feed (1) basic features, (2) basic features plus text features, and (3) all features into an XGBoost model to obtain the accuracy, F1 score, precision, and recall, respectively. We also train an XGBoost model to construct a regression model with the ratio/percentage of success as output. The inferred features significantly improve the model's performance (Table 3).
To extract statistically significant features, we construct a logistic regression model for the classification problem; results appear in Table 4 and lend support to Hypothesis 1. The #Donors feature may be highly correlated with success, but here we kept the #Donors feature, because it has less impact on other features. To further analyze the impact of each feature on the success of crowdfunding, we conduct several counterfactual analysis experiments. Statistically significant features are examined in a separate analysis due to our high number of features.
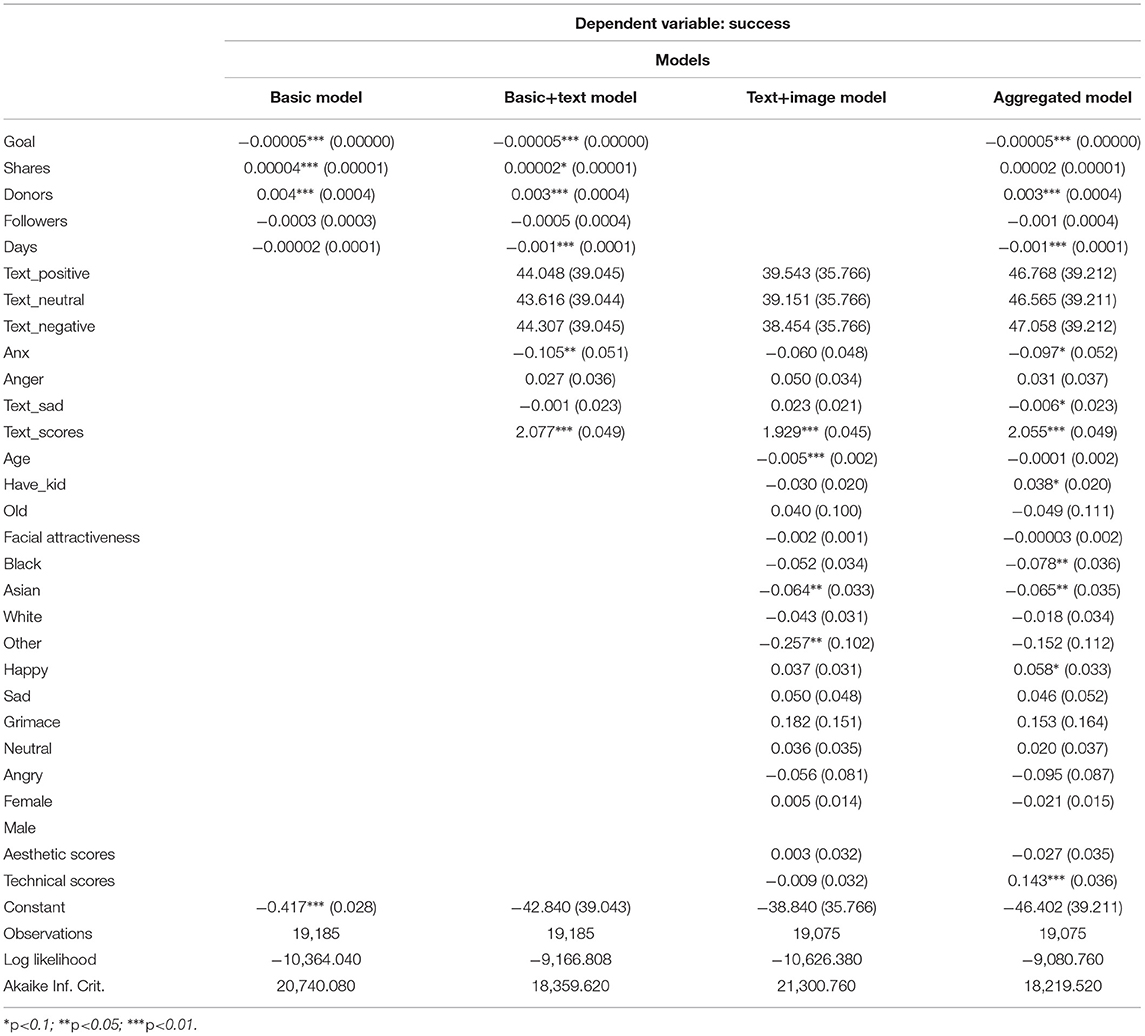
Table 4. Logistic regression model results for the relationship between campaigns' features and success.
4.6. Counterfactual Analysis
We compare the effects of the abovementioned features on crowdfunding success before and after the COVID-19 outbreak and further analyze whether the pandemic has altered people's priorities. To better understand the causality between these features and crowdfunding success, we employ counterfactual analysis, a popular means of comparative inquiry (Chang et al., 2021). We test our remaining two hypotheses based on findings from the counterfactual analysis experiments.
4.6.1. Removing the Category Factors to Reduce the Impact
In counterfactual analysis, we turn the factor indicator of each category into 0 in each experiment as shown in Table 5. We find that Creative Arts, Music & Film and Dreams Hopes & Wishes had positive causal impacts on the success of crowdfunding before the COVID-19 outbreak but a negative causal effect after the outbreak. The positive effects of Medical, Illness & Healing, Accidents & Emergencies, and Non-Profits & Charities on the success of crowdfunding have increased dramatically since the COVID-19 outbreak. The significance of these three categories in Table 5 has changed, suggesting the pandemic's profound effects on each. The COVID-19 pandemic has accordingly informed individuals' campaign preferences, with people focusing more on medical or charity topics than dreams, arts, and charity topics. These results validate Hypothesis 2. Notably, the ratio in the table changes negligibly across multiple categories; eliminating the impact of one category does not significantly change the overall success rate, but this outcome does not mean that these categories are not significant.
4.6.2. Improving the Effect of Features
First, we divide the remaining features into basic features, text features, and image features after removing category features. Combined with the counterfactual experiment, we analyze only statistically significant features. Table 6 presents the results of counterfactual analyses of such features using logistic regression. Campaign success rates increase significantly when increasing these features with their mean values.
• Goal Amount The counterfactual experiment reveals that a campaign's goal amount has the most significant causal relationship with success. The displayed funding goal signals the project's complexity. In general, the higher the crowdfunding goal, the lower the project's success rate (Barbi and Bigelli, 2017). Our counterfactual experiment results are also consistent with our previous findings.
• Duration The experimental results indicate that the duration of crowdfunding campaigns positively influences their success: the longer a project lasts, the more likely people are to find and share it (p < 0.001). Campaigns with a greater number of shares typically receive more donations (p < 0.001).
• Donors The relationship between the number of donors and success is significant (p < 0.001); that is, the number of donors positively affects crowdfunding success.
• Emotion of text Text scores obtained by XLNet have had significant positive impacts on crowdfunding success since the COVID-19 outbreak, implying a statistically significant relationship between text and success. Further analysis reveals a significant relationship between emotion and campaign success rate. For example, the anxiety (p < 0.1) and sadness (p < 0.1) conveyed in text each negatively influence success. This pattern corroborates our counterfactual experiment.
• Technical Score Technical scores obtained via the pretrained NIMA model have had a significant positive impact on crowdfunding success since the COVID-19 outbreak (p < 0.01). A statistically significant relationship thus seems to exist between image quality and campaign success.
• Race Among image features, race appears to have differentially affected crowdfunding success since the COVID-19 outbreak. Of all races, Black has the most significant impact on success (p < 0.05). While Black people are historically less likely to be funded than people of other races, this effect was less significant before COVID-19 (p < 0.1). In other words, Blacks are even less likely to be funded during the pandemic. Asian ethnicity also has a statistically significant impact on the success of crowdfunding (p < 0.05) after the COVID-19 outbreak in the full dataset; no statistically significant impact applies before the outbreak. These results support Hypothesis 3. We suspect that the former finding might be related to the root cause of the #BlackLivesMatter movement with the latter being tied to the root cause of the #StopAsianHate movement. These two directions would be interesting to investigate in future work.
• Emotion of cover image We examine the relationship between the emotion of the cover image and campaign success, identifying a significant difference between them. The counterfactual experiments show that sadness adversely affects success whereas happiness has a positive impact (p < 0.1). People are more willing to donate to campaigns whose cover images carry positive valence than to those whose images carry negative valence. This discovery is somewhat surprising, as donors seem to prefer giving to individuals who are optimistic about life.
• Children The logistic regression model indicates that the impact of children on crowdfunding success tends to be significantly positive if the campaign is related to children. In addition, we confirm that the effect is positive and consistent with the regression model.
4.7. LDA Topic Generation and Analysis
We further analyze the relationships among crowdfunding success and the topics in each category using latent Dirichlet allocation (LDA) topic modeling (Blei et al., 2003). We specifically employ LDA to divide each category into five topics. We then build a regression model to analyze which topics have the most positive impacts on the success of crowdfunding and which have the most negative effects. We focus on categories featuring significant changes before and after the COVID-19 outbreak: Accidents & Emergencies, Travel & Adventure, Medical, Illness & Healing, Celebrations & Events, Creative Arts, Music & Film and Dreams, and Hopes & Wishes. The results are shown in Figure 2, where c denotes the correlation coefficient between success and topics. The first value represents the correlation coefficient before the COVID-19 outbreak, while the second value represents the coefficient after the outbreak. The frequencies of some words in the same category are high, leading to substantial overlap between topics. We therefore add the words whose frequencies are in the top 10 of all topics to the stop words list. We next apply an LDA model again to generate topics and repeat this process until no such words remain. In the end, the coherence of the topic model is 0.34803. In addition, we observe some significant changes in the Other category before and after the COVID-19 outbreak. Because this category includes various topics, we construct separate topic models for this category to analyze the impact of each topic on crowdfunding success.
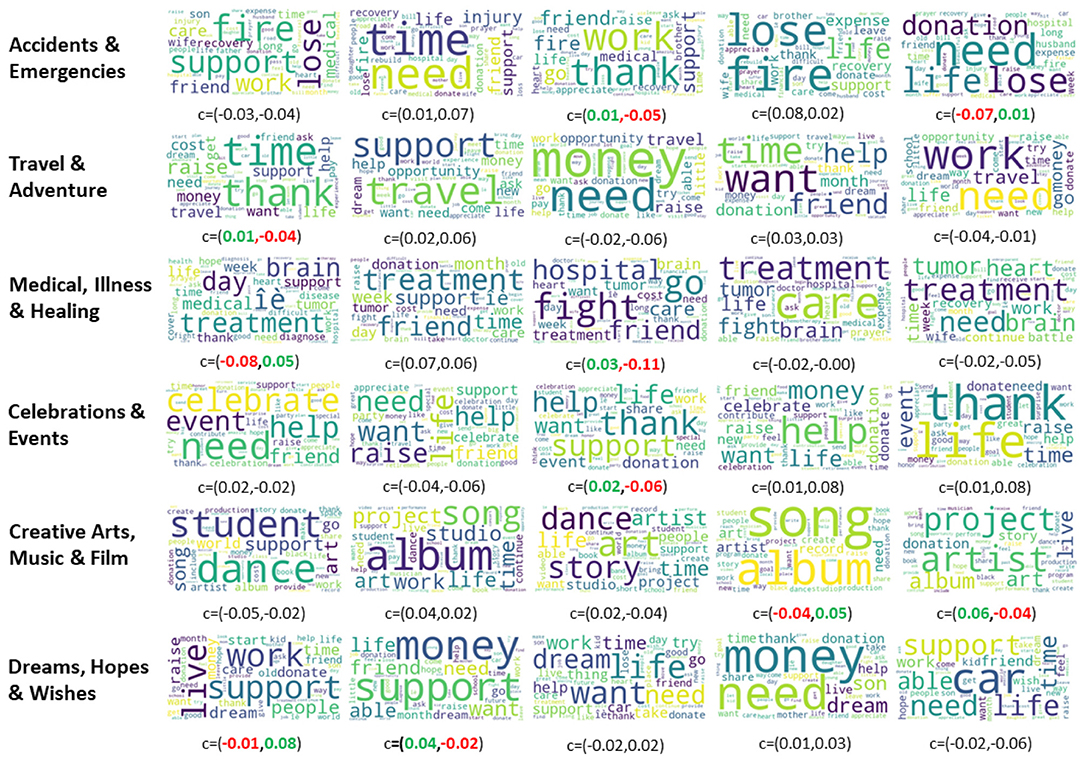
Figure 2. Topics under different categories. The first value represents the correlation coefficient before the COVID-19 outbreak, while the second value represents the coefficient after the outbreak. A green value indicates that the topic has a positive impact on success, while a red value indicates that the topic has a negative impact on success. Note those topics with changes in the polarity of the impact before and after the COVID-19 pandemic.
We find significant changes in the Other category before and after the COVID-19 outbreak and analyze them individually. We start with a topic number with the best coherence value. Topics in this category were relatively scattered before the COVID-19 outbreak; we divide them into four topics (coherence score: 0.35192; see Figure 3). The topics are relatively concentrated after the COVID-19 outbreak and are thus only divided into two topics (coherence score: 0.32625; see Figure 4). Before the COVID-19 outbreak, the Other category included dreams, gifts, children, travel, honeymoons & weddings, and other topics. Combined with the above results, we find that most of these topics negatively affect the success of crowdfunding. By contrast, after the COVID-19 outbreak, most topics focus on family, children, friends, and medical care; these topics positively influence campaigns' success. The significance of the Other category appears to have changed given these differences before and after the outbreak.

Figure 3. Topics under the Other category that had significant effect on success before the COVID-19 outbreak. We generate four topics with the best coherence value: 0.35192.
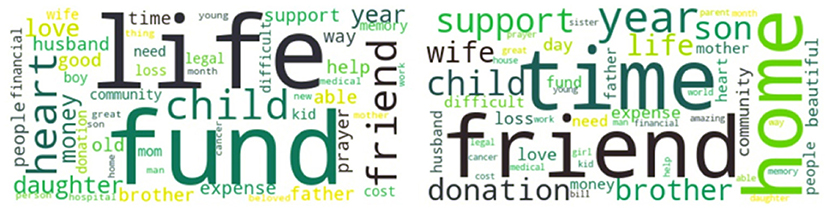
Figure 4. Topics under the Other category that had significant effect on success during the COVID-19 pandemic. We generate two topics with the best coherence value: 0.32625.
5. Discussion
Our study analyzes the changes in significant features influencing crowdfunding success before and after the COVID-19 outbreak and validates three hypotheses. The results suggest a substantial difference in certain categories before and after the outbreak. Although dreams, travel, or other topics were less likely to be funded before the COVID-19 outbreak, people have begun to donate to these campaigns thereafter. People have also started to pay more attention to medical, accident, and charity projects. Some categories have not changed before or after the outbreak. For instance, campaigns including babies, family, funerals, and memorials have always attracted donations relatively easily. Conversely, campaigns involving sports, weddings, and missions have received fewer donations. We also observe significant differences in crowdfunding success by race. The COVID-19 pandemic has made it more challenging for Black and Asians to raise money because the pandemic has exacerbated existing social disparities.
Consistent with research demonstrating that image attributes influence the success of crowdfunding campaigns (Bretschneider and Leimeister, 2017; Hou et al., 2020), sadness, anxiety, and anger are found to have either negative or no effects on crowdfunding in this study. Yet campaigns with positive emotions conveyed through the cover image are more likely to be funded than those showing negative emotions. These findings suggest that fundraisers should express optimistic attitudes toward life in addition to describing their misfortune.
6. Conclusion
Our study supports the prevalence of discrimination in online marketplaces (Edelman et al., 2017; Farmaki and Kladou, 2020). To the best of our knowledge, this study is the first to reveal the presence of racial discrimination on crowdfunding platforms.
Several limitations of this study merit attention. The first limitation is that our findings may not generalize to other countries because we only use U.S. data on GoFundMe. However, the COVID-19 pandemic may have a considerable impact on crowdfunding campaigns throughout the world. Data collection is required in other countries to understand the extent to which our findings are generalizable. Second, we only consider stable features on the GoFundMe website. We will account for other dynamic factors in the future, such as the characteristics of each donation. Finally, we do not differentiate between categories of crowdfunding campaigns. Different categories may distinctly influence the success of crowdfunding (Zhang et al., 2020), which is worth investigating as well.
Data Availability Statement
The raw data supporting the conclusions of this article will be made available by the authors, without undue reservation.
Author Contributions
JL designed the model and the computational framework. XZ and JW analyzed the data, carried out the implementation, and wrote the manuscript with input from all authors. JW performed the calculations. XZ and JL conceived the study and were in charge of overall direction and planning. All authors contributed to the article and approved the submitted version.
Conflict of Interest
The authors declare that the research was conducted in the absence of any commercial or financial relationships that could be construed as a potential conflict of interest.
Publisher's Note
All claims expressed in this article are solely those of the authors and do not necessarily represent those of their affiliated organizations, or those of the publisher, the editors and the reviewers. Any product that may be evaluated in this article, or claim that may be made by its manufacturer, is not guaranteed or endorsed by the publisher.
Footnotes
References
Barbi, M., and Bigelli, M. (2017). Crowdfunding practices in and outside the US. Res. Int. Bus. Fin. 42, 208–223. doi: 10.1016/j.ribaf.2017.05.013
Binstock, R. H., George, L. K., Cutler, S. J., Hendricks, J., and Schulz, J. H. (2011). Handbook of Aging and the Social Sciences. Elsevier.
Blei, D. M., Ng, A. Y., and Jordan, M. I. (2003). Latent Dirichl et allocation. J. Mach. Learn. Res. 3, 993–1022. doi: 10.5555/944919.944937
Bretschneider, U., and Leimeister, J. M. (2017). Not just an ego-trip: exploring backers' motivation for funding in incentive-based crowdfunding. J. Strat. Inform. Syst. 26, 246–260. doi: 10.1016/j.jsis.2017.02.002
Cadogan, T.. (2021). Gofundme CEO: Hello Congress, Americans Need Help and We Can't Do Your Job for You. USA Today, 11.
Chang, S., Pierson, E., Koh, P. W., Gerardin, J., Redbird, B., Grusky, D., et al. (2021). Mobility network models of covid-19 explain inequities and inform reopening. Nature 589, 82–87. doi: 10.1038/s41586-020-2923-3
Edelman, B., Luca, M., and Svirsky, D. (2017). Racial discrimination in the sharing economy: evidence from a field experiment. Am. Econ. J. 9, 1–22. doi: 10.1257/app.20160213
Elmer, G., Ward-Kimola, S., and Burton, A. G. (2020). Crowdfunding during COVID-19: An international comparison of online fundraising. First Monday. 25. doi: 10.5210/fm.v25i11.10869
Farhoud, M., Shah, S., Stenholm, P., Kibler, E., Renko, M., and Terjesen, S. (2021). Social enterprise crowdfunding in an acute crisis. J. Bus. Vent. Insights 15:e00211. doi: 10.1016/j.jbvi.2020.e00211
Farmaki, A., and Kladou, S. (2020). Why do Airbnb hosts discriminate? Examining the sources and manifestations of discrimination in host practice. J. Hosp. Tour. Manage. 42, 181–189. doi: 10.1016/j.jhtm.2020.01.005
Giele, J. Z., and Elder, G. H. (1998). “Life course research: Development of a field,” in Methods of Life Course Research: Qualitative and Quantitative Approaches (Sage Publications), 5–27. doi: 10.4135/9781483348919
Hou, J.-R., Zhang, J. J., and Zhang, K. (2020). Pictures that are worth a thousand donations: How emotions in project images drive the success of crowdfunding campaigns? An image design perspective. PsychRN: Psychological Applications of Technology and Media.
Hutto, C., and Gilbert, E. (2014). “Vader: a parsimonious rule-based model for sentiment analysis of social media text,” in Proceedings of the International AAAI Conference on Web and Social Media, Vol. 8.
Igra, M., Kenworthy, N., Luchsinger, C., and Jung, J.-K. (2021). Crowdfunding as a response to covid-19: increasing inequities at a time of crisis. Soc. Sci. Med. 282:114105. doi: 10.1016/j.socscimed.2021.114105
Kaartemo, V.. (2017). The elements of a successful crowdfunding campaign: a systematic literature review of crowdfunding performance. Int. Rev. Entrepreneur. 15, 291–318.
Koch, J.-A., and Siering, M. (2019). The recipe of successful crowdfunding campaigns. Electron. Markets 29, 661–679. doi: 10.1007/s12525-019-00357-8
Mattingly, T. J., Li, K., Ng, A., Ton-Nu, T.-L., and Owens, J. (2021). Exploring patient-reported costs related to hepatitis c on the medical crowdfunding page gofundme®. PharmacoEcon. Open 5, 245–250. doi: 10.1007/s41669-020-00232-9
Radu, M. B., and McManus, L. (2018). A qualitative analysis of requests for financial help via gofundme by victims of intimate partner violence. Sociol. Spectr. 38, 312–325. doi: 10.1080/02732173.2018.1502105
Rajwa, P., Hopen, P., Mu, L., Paradysz, A., Wojnarowicz, J., Gross, C. P., et al. (2020). Online crowdfunding response to coronavirus disease 2019. J. Gen. Intern. Med. 35, 2482–2484. doi: 10.1007/s11606-020-05896-x
Rhue, L., and Robert, L. P. (2018). “Emotional delivery in pro-social crowdfunding success,” in Extended Abstracts of the 2018 CHI Conference on Human Factors in Computing Systems (Montreal, QC), 1–6. doi: 10.1145/3170427.3188534
Rice, N. E., Lang, I. A., Henley, W., and Melzer, D. (2011). Common health predictors of early retirement: findings from the English longitudinal study of ageing. Age ageing 40, 54–61. doi: 10.1093/ageing/afq153
Saleh, S. N., Lehmann, C. U., and Medford, R. J. (2021). Early crowdfunding response to the covid-19 pandemic: CROSS-sectional study. J. Med. Intern. Res. 23:e25429. doi: 10.2196/25429
Serengil, S. I., and Ozpinar, A. (2020). “Lightface: a hybrid deep face recognition framework,” in 2020 Innovations in Intelligent Systems and Applications Conference (Istanbul), 23–27. doi: 10.1109/ASYU50717.2020.9259802
Song, S., Cohen, A. J., Lui, H., Mmonu, N. A., Brody, H., Patino, G., et al. (2020). Use of GoFundMe®to crowdfund complementary and alternative medicine treatments for cancer. J. Cancer Res. Clin. Oncol. 146, 1857–1865. doi: 10.1007/s00432-020-03191-0
Talebi, H., and Milanfar, P. (2018). Nima: neural image assessment. IEEE Trans. Image Process. 27, 3998–4011. doi: 10.1109/TIP.2018.2831899
Valaskova, K., Kliestik, T., and Gajdosikova, D. (2021a). Distinctive determinants of financial indebtedness: evidence from Slovak and Czech enterprises. Equilibr. Q. J. Econ. Econ. Policy 16, 639–659. doi: 10.24136/eq.2021.023
Valaskova, K., Ward, P., and Svabova, L. (2021b). Deep learning-assisted smart process planning, cognitive automation, and industrial big data analytics in sustainable cyber-physical production systems. J. Self-Govern. Manage. Econ. 9, 9–20. doi: 10.22381/jsme9220211
Yang, K., Wang, C., Sarsenbayeva, Z., Tag, B., Dingler, T., Wadley, G., et al. (2020). Benchmarking commercial emotion detection systems using realistic distortions of facial image datasets. The Visual Comput. 37, 1447–1466. doi: 10.5555/3454287.3454804
Yang, Z., Dai, Z., Yang, Y., Carbonell, J., Salakhutdinov, R., and Le, Q. V. (2019). Xlnet: generalized autoregressive pretraining for language understanding. arXiv preprint arXiv:1906.08237.
Keywords: crowdfunding, GoFundMe, counterfactual, topic model, XGBoost
Citation: Wang J, Luo J and Zhang X (2022) How COVID-19 Has Changed Crowdfunding: Evidence From GoFundMe. Front. Comput. Sci. 4:893338. doi: 10.3389/fcomp.2022.893338
Received: 11 March 2022; Accepted: 13 April 2022;
Published: 20 May 2022.
Edited by:
Luca Romeo, Marche Polytechnic University, ItalyReviewed by:
Alessia Pedrazzoli, University of Modena and Reggio Emilia, ItalyMaria Kovacova, University of Žilina, Slovakia
Copyright © 2022 Wang, Luo and Zhang. This is an open-access article distributed under the terms of the Creative Commons Attribution License (CC BY). The use, distribution or reproduction in other forums is permitted, provided the original author(s) and the copyright owner(s) are credited and that the original publication in this journal is cited, in accordance with accepted academic practice. No use, distribution or reproduction is permitted which does not comply with these terms.
*Correspondence: Xupin Zhang, eHB6aGFuZ0BmZW0uZWNudS5lZHUuY24=