- 1Wearable Technologies Lab, University of Sussex, Brighton, United Kingdom
- 2Deutsches Forschungszentrum für Künstliche Intelligenz, University of Kaiserslautern, Kaiserslautern, Germany
- 3Lucerne University of Applied Sciences and Arts, Luzern, Switzerland
1 Introduction
Opportunity++ is a precisely annotated dataset designed to support AI and machine learning research focused on the multimodal perception and learning of human activities (e.g., short actions, gestures, modes of locomotion, higher-level behavior).
This is an important area of research in wearable, mobile and ubiquitous computing Kim et al. (2010); Bulling et al. (2014); San-Segundo et al. (2018); Plotz and Guan (2018) systems able to model human behaviour and automatically recognise specific activities and situations enable new forms of implicit activity-driven interactions Lukowicz et al. (2010), which can be used to provide industrial assistance Stiefmeier et al. (2008), to support independent living or assist in sports and healthcare Avci et al. (2010); Patel et al. (2012); Feuz et al. (2015); Lee and Eskofier (2018) — see Demrozi et al. (2020) and Chen et al. (2021) for recent reviews.
Opportunity++ is a significant multimodal extension of a previous dataset called OPPORTUNITY—including previously unreleased videos and video-based skeleton tracking.
The former OPPORTUNITY dataset Roggen et al. (2010) was used in a machine learning challenge in 2010 Sagha et al. (2011), which led to a meta-analysis of competing approaches and the establishment of baseline performance measures Chavarriaga et al. (2013), and was then publicly released in 20121.
Over the years, OPPORTUNITY became a well established dataset. For instance, it was used in one of the seminal work on deep learning for human activity recognition from wearable sensors Ordóñez and Roggen (2016); Morales and Roggen (2016), and it has been used recently in fields as varied as machine learning model compression for embedded systems Thakker et al. (2021), transfer learning research Kalabakov et al. (2021), ontology-based activity recognition Noor et al. (2018), unsupervised domain adaptation Chang et al. (2020), zero-shot learning Wu et al. (2020), convolutional feature optimisation Hiremath and Ploetz (2021) and deep network architecture optimisation Bock et al. (2021); Pellatt and Roggen (2021).
In recent years, there has been a growing interest in the combination of data obtained from video-based systems together with the time-series data originating from on-body sensors Rey et al. (2019); Kwon et al. (2020); Fortes Rey et al. (2021). Several reasons underpin this type of research: video-based activity recognition is naturally complementary to other sensing modalities Zhang et al. (2019); there is a wide availability of public videos that can be used as additional training source (e.g., YouTube) Kwon et al. (2020); and it makes sense to opportunistically use whichever sensing modalities are available around the user at any point in time Roggen et al. (2013). The original OPPORTUNITY dataset, however, did not contain any video camera data, nor data derived from Supplementary Video data. A similar limitation is seen with video-focused datasets, which generally do not include other sensor modalities Chaquet et al. (2013).
Opportunity++ addresses these limitations by enhancing the OPPORTUNITY dataset with previously unreleased video footage and video-based skeleton tracking. This will allow researchers to further build on this well-recognized dataset, while at the same time opening new research opportunities in multimodal sensor fusion.
2 Data Collection Method
Opportunity++ contains the recordings of 4 people engaging in a early-morning routine in a naturalistic home environment.
We designed the activity recognition environment and scenario to generate many activity primitives, yet in a realistic manner. Participants were instructed to prepare a coffee and sandwich as part of their breakfast, have the breakfast, and finally clean the kitchen putting utensils and foodstuffs back in place or in the dishwasher. This scenario allows to generates a large number of elementary actions (interaction between the hands and objects or hands and the environment) in a natural manner.
The environment, shown in Figure 1C, was set up to resemble a common kitchen fitted with all the required appliances (i.e., dish washer, fridge, etc.). The appliances as well as the object used to complete the activities were instrumented with a wide variety of motion and switch sensors. An inertial motion capture system and an UWB localization system was embedded in a jacket worn by the users performing the activities.
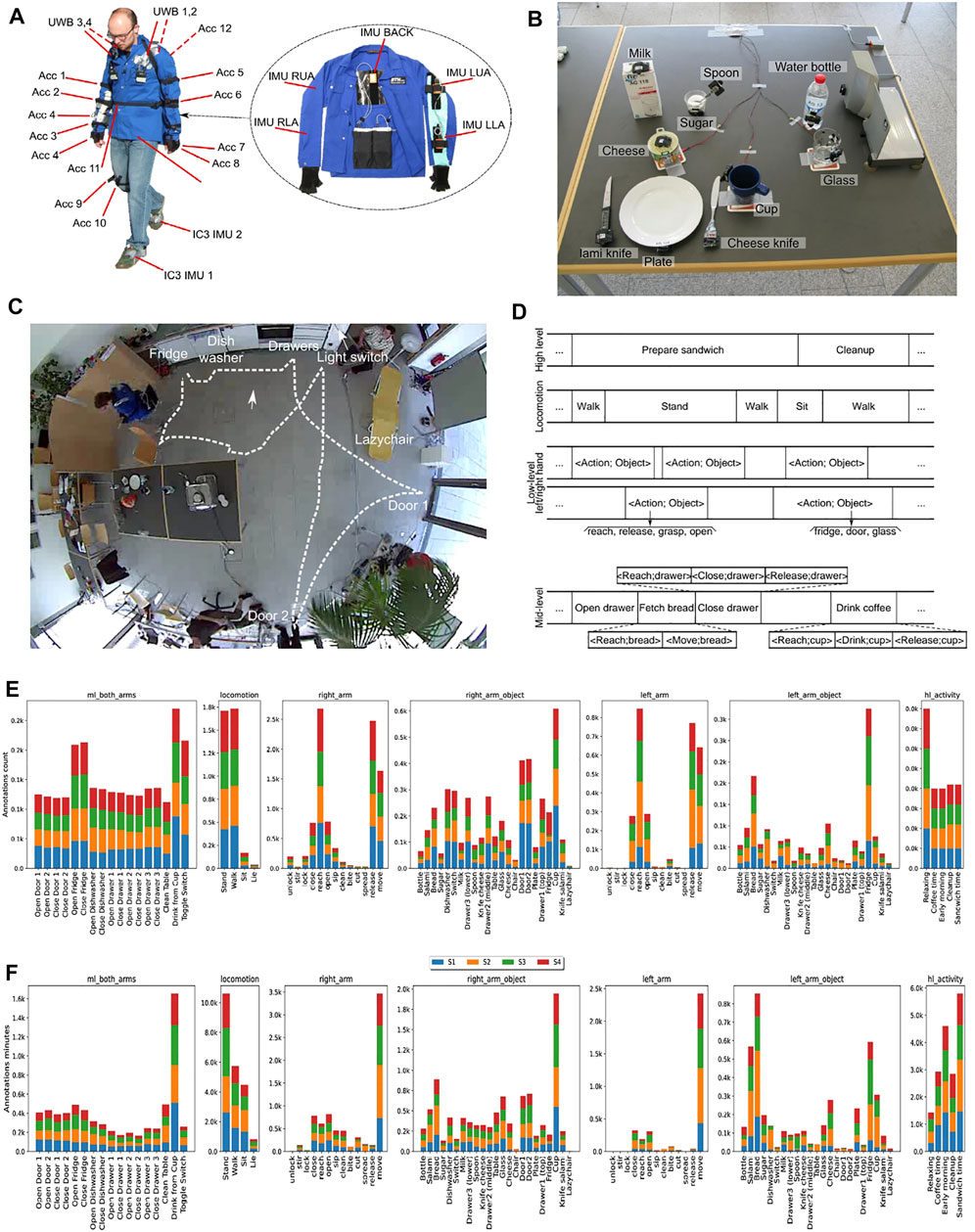
FIGURE 1. (A): placement of on-body sensors. Acc are 3D accelerometers; UWB are ultra-wideband localization tags; IMU are inertial measurement units (InertiaCube3 on the shoe and Xsens MT9 in the jacket on the upper body). (B): instrumented objects (except the bread and salami). (C): top view of the environment with key instrumented environmental objects. (D): annotation through independent tracks indicating the high-level activities, the modes of locomotion, the left and right hand action primitives, and finally the “mid-level” activities. (E, F): annotation counts and total time for each annotation, spanned by each annotation type, per-user.
2.1 Activity Scenario
The dataset included data from 4 users performing everyday living activities in a kitchen environment. For each user the dataset includes 6 different runs. Five runs, termed Activity of Daily Living (ADL), followed a given scenario as detailed below. The sixth termed Drill Run, was designed to generate a large number of activity instances in a more constrained scenario. The ADL run consists of temporally unfolding situations. In each situation (e.g., preparing sandwich), a large number of action primitives occur (e.g., reach for bread, move to bread cutter, operate bread cutter).
2.1.1 ADL Run
The ADL run consists of sequence of activities:
• Start: lying on the deckchair, get up
• Groom: move in the room, check that all the objects are in the right places in the drawers and on shelves
• Relax: go outside and have a walk around the building
• Prepare coffee: prepare a coffee with milk and sugar using the coffee machine
• Drink coffee: take coffee sips, move around in the environment
• Prepare sandwich: include bread, cheese and salami, using the bread cutter and various knifes and plates
• Eat sandwich
• Cleanup: put objects used to original place or dish washer, cleanup the table
• Break: lie on the deckchair
The users were given complete liberty in executing these activities in the most natural way for them. This makes these runs particularly challenging for the recognition of human activities.
2.1.2 Drill Run
The drill run consists of 20 repetitions of the following sequence of activities:
• Open then close the fridge
• Open then close the dishwasher
• Open then close 3 drawers (at different heights)
• Open then close door 1
• Open then close door 2
• Toggle the lights on then off
• Clean the table
• Drink while standing, then while seated
The dashed line in Figure 1C represents the typical trajectory of users during the Drill run, which performed the indicated activities in the same sequence throughout.
2.2 Annotations Track
The dataset was accurately annotated a posteriori from video recordings. The annotations include high level activities as well as lower level actions. They are divided in multiple tracks:
• Left/Right arm: this annotation track indicates the single action perform by the left and right hand. The annotated actions are: stir, lock, unlock, open, close, reach, release, move, sip, clean, bite, cut, spread.
• Left/Right arm object: these annotations refer to the object used while performing a corresponding action from the previous annotation track. The annotated objects are: bottle, salami, bread, sugar, dishwasher, switch, milk, spoon, knife cheese, knife salami, table, glass, cheese, chair, door (1 and 2), plate, fridge, cup, lazychair, drawer (lower, middle and top),
• ML Both Arm (“mid-level” annotations): these are actions performed with any of the two hands such as Open/Close door, Open/Close drawer, etc. These actions might be performed with either the right or the left arm, although the majority of people used the right hand.
There are a total of 17 unique such activities: they correspond to the actions “open”, “close” applied to the fridge, dishwasher, each of the two doors, and each of the 3 drawers, and to the actions “toggle” the light switch, “clean” the table, and “drink”. These 17 types of mid-level activities are the ones that have been most commonly used for activity recognition research.
• Locomotion: these annotations indicate the locomotion status of the user during the session (stand, walk, sit, lie).
The list of labels composing each annotation track can be found in Figure 1E,F, as x-axis of each histogram.
2.3 Sensor Data
The dataset comprises the readings of motion sensors recorded while the users executed the ADL and Drill runs. The detailed format is described in the package. The attributes correspond to raw sensor readings. There is a total of 242 attributes.
2.3.1 Body-Worn Sensors
The body-worn motion sensors are the 7 inertial measurement unitsand 12 3D acceleration sensors displayed in Figure 1A.
The inertial measurement units provide readings of: 3D acceleration, 3D rate of turn, 3D magnetic field, and orientation of the sensor with respect to a world coordinate system in quaternions. Five sensors are on the upper body and two are mounted on the user’s shoes.
The acceleration sensors provide 3D acceleration. They are mounted on the upper body, hip and leg. Four tags for an ultra-wideband localization system are placed on the left/right front/back side of the shoulder.
There is a total of 145 channels originating from the on-body sensors.
2.3.2 Object Sensors
12 objects (cup, salami, water bottle, cheese, bread, salami knife, cheese knife, milk bottle, spoon, sugar, plate, glass) are instrumented with wireless sensors measuring 3D acceleration and 2D rate of turn, for a total of 60 channels. This allows to detect which objects are used, and possibly also the kind of usage that is made of them.
2.3.3 Ambient Sensors
Ambient sensors include 13 switches and 8 3D acceleration sensors in drawers, kitchen appliances and doors. They corresponds to 37 channels.
The reed switches are placed in triplets on the fridge, dishwasher and drawer 2 and drawer 3. They may be used to detect three states of the furniture element: closed, half open, and fully open.
The acceleration sensors may allow to assess if an element of furniture is used, and whether it may be opened or closed.
2.4 Videos
This new release includes the videos recorded during the data collection from a side view. The videos are recorded at 640 × 480 pixels and 10 fps. For every video, the dataset annotations are included in form of subtitles (SRT files). These can be useful to better understand which annotated activities take place in the video.
The videos have been anonymized to preserve the identity of the subjects. However, in order to make the videos interesting for researchers, we also provide the pose tracking of all the people in the scene generated with OpenPose (Cao et al. (2021)).
2.4.1 People Tracking
The tracking of the people is performed using the OpenPose framework. OpenPose can detect people in a frame and generate a skeleton of body parts for each person detected. We used the BODY_25 model to generate the skeletons. As the name suggests, this model generate the x,y coordinates of 25 body points:
“Nose”, “Neck”, “RShoulder”, “RElbow”, “RWrist",
“LShoulder”, “LElbow”, “LWrist”, “MidHip",
“RHip”, “RKnee”, “RAnkle”, “LHip”, “LKnee”, “LAnkle",
“REye”, “LEye”, “REar”, “LEar”, “LBigToe",
“LSmallToe”, “LHeel”, “RBigToe”, “RSmallToe”, “RHeel",
“Background"
For each body point, the model also provides a confidence level in the interval [0–1).
Each frame can have several people in it, generally a single participant performing the data collection protocol and one or more bystanders. However, OpenPose does not provide tracking of the same person across multiple frames. Therefore, we implemented an additional post-processing step in order to assess whether a tracked person is a participant or just a bystander during the data collection.
The tracking of the participant is obtained through the color analysis of the arms of all the people detected in a frame. The participant is the only person wearing a blue jacket during the data collection. Our method extracts the level of blue in the area of tracked arms: if the level of blue is above 50%, then that and only that person is labelled as participant, while all the others are labelled as bystanders.
Due to the low resolution of the videos, we also defined a custom heuristic for values of detected blue between 20 and 50%. In this case, the person is labelled as participant only if the coordinates of her neck and hips in the frame are no more than 10 pixels a part from the position of a person detected as participant with a confidence higher than 80%.
2.4.2 Anonymisation
In order to avoid possible privacy issue with the release of the videos, we anonymise the faces of the people present in each frame through pixelation. This technique takes a subset of a frame, divides this area in blocks and then substitute each pixel in each block with the average color of that block.
In our dataset, we pixelate an area of 15 × 15 pixels using a 3 × 3 grid of blocks corresponding to the area of the face of every person in the frame. For every frame, the area was selected using the x-y coordinates of the Neck generated by OpenPose. This body point was selected as it has the highest confidence value (see Figure 2D).
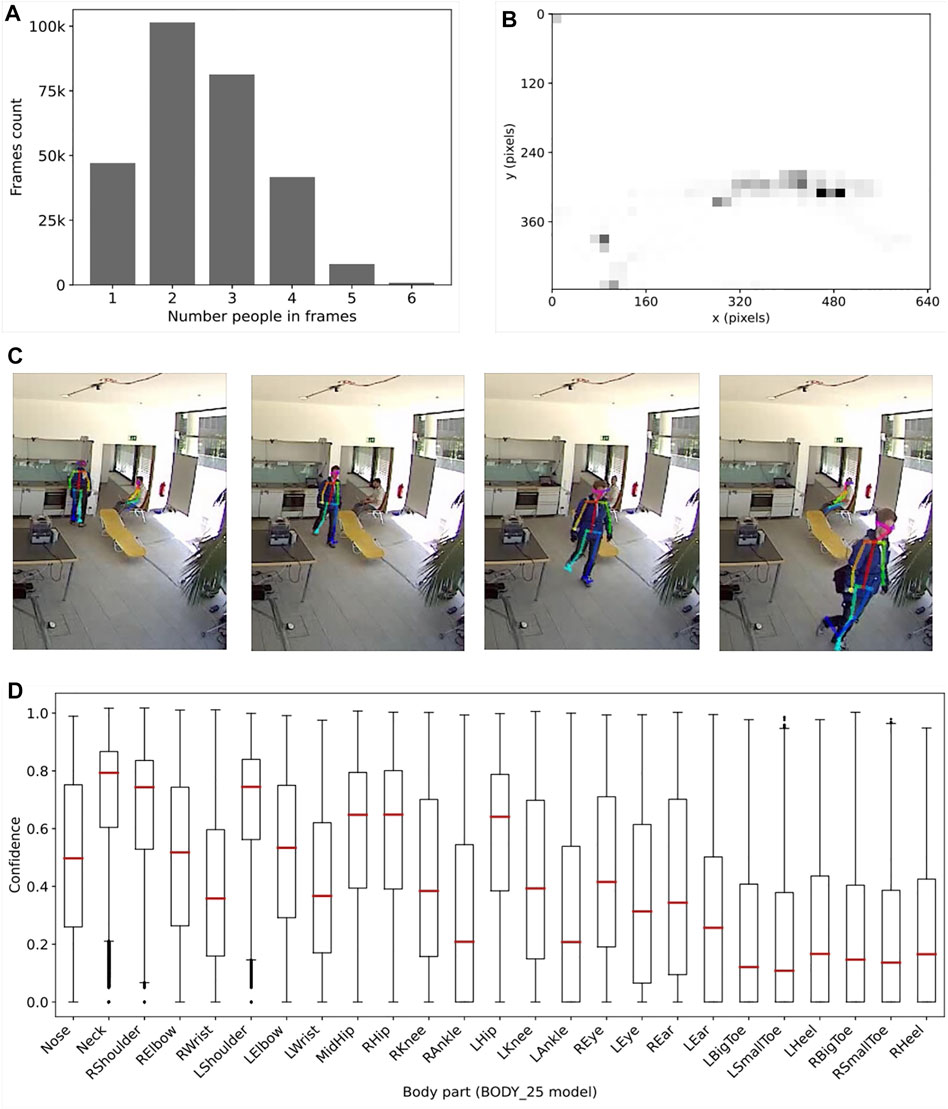
FIGURE 2. OpenPose tracking analysis. (A) plots the counting of people in all the frames of all the videos. (B) presents the distribution of the people in the frames in all the videos, calculated using the Neck coordinates. (C) shows a four consecutive frames with the skeleton produced by the people tracking. Finally, (D) indicates the distribution of the confidence values for all the body parts of the BODY_25 model.
3 Dataset Summary
The final release of OPPORTUNITY++ includes the data of 4 users, performing 5 ADL runs and 1 Drill run each. The dataset includes a total of 19.75 h of sensor data annotated with multiple tracks: 1.88 h of actions performed with any of the two hands (ML Both Hands), 6.01 h of locomotion status (Locomotion), 3.02 h of annotated data for action performed with a specific hand and 4.89 h of high level activities. Moreover, the sensors placed on the objects produced a total of 3.92 h of annotated data.
Overall, the dataset comprise of more than 24,000 unique annotations, divided in 2,551 activity instances for ML both arm, 3,653 activity instances of locomotion, 12,242 action instances performed with a single specific hand, 122 instances of high level activities and 6,103 instances of interaction with the object in the kitchen.
Due to the common high-level scenario, the data is quite balanced amongst the 4 users, as visible from Figure 1.
4 Applications of the Dataset
Opportunity++ can be used in to address a wide range of research questions. We indicate here a few potential uses of the dataset:
• Multimodal fusion: Fusion between multiple distinct sensor modalities can be explored, notably between skeleton data obtained from the videos and the wearable sensors to evaluate the potential improvements of a multimodal activity recognition approach (Chen et al. (2016)). This can be interesting when designing activity recognition which aim at obtaining the highest performance. For instance, in assisted-living scenarios occupants may wear a few wearable devices but also be observed by cameras.
• Dynamic modality selection: Research may focus on how to dynamically select the most suitable modalities at any point in time, such as wearable sensors when there are video occlusions, or Supplementary Video data when there are wireless sensor data loss, or when battery usage of wireless devices must be minimized.
• Data imputation: Wireless sensors may suffer from data loss, especially in congested radio environments. This is the case in this dataset with some of the wearable sensors indicated by “Acc” in Figure 1A. Data imputation strategies could be be explored to recover virtual inertial measurement data from the Supplementary Video data. Similarly, the inertial measurement units may be used to impute the skeleton tracking data during occlusions. As we have synchronised sensor and pose data, the dataset can also be used as a benchmark for generating virtual sensor data from poses.
• Re-annotation: The publication of the videos will allow the community to re-annotate the dataset with new or distinct activities, and as well correct potential annotation mistakes. While significant efforts were made to annotate the OPPORTUNITY datasets, some minor annotation issues may nevertheless exist. In order to ensure the comparability of results, we recommend researchers exploring re-annotation for activity recognition to report their results based on the annotations as provided in this dataset, to allow for comparison to prior work, as well as to their new re-annotations.
• Annotation crowd-sourcing: Dataset annotation is particularly time consuming: this dataset required 7–10 h of annotation time for a 30-min video segment. Crowd-sourcing can help overcome this challenge, while at the same time opening multiples areas of research: developing suitable tools for annotation; comparing the variability between “expert” raters and lay person; exploring whether common “ontologies” of annotations emerge naturally, or how they can be influenced through instructions provided to the annotators; etc.
• Inter-rater reliability: Data annotation of activities of daily life is particularly challenging. Identifying the “start” and “end” of an activity may be interpreted differently between annotators. This dataset could be used to explore the inter-rater reliability of annotators. This may be particularly interesting for annotation crowd-sourcing (Nowak and Rüger (2010)).
• Privacy-preserving annotation: While the videos here are anonymized, the use of video-based skeleton tracking and motion capture from wearable inertial measurement data allows to explore how datasets could be annotated without ever sharing the original video, thus further minimising risks to privacy, for instance by rendering a user model in a virtual reality environment. Early work to that end showed a surprising human ability to recognise characteristic activities from such a virtual model Ciliberto et al. (2016).
Data Availability Statement
The datasets presented in this study can be found in online repositories. The names of the repository/repositories and accession number(s) can be found below: https://ieee-dataport.org/open-access/opportunity-multimodal-dataset-video-and-wearable-object-and-ambient-sensors-based-human.
Author Contributions
AC, DR, and PL organised and performed the original data collection. They also provided invaluable insight on the data useful for the data analysis and processing performed by MC and VF. MC and VF generated the new data as well as participating in the writing of this manuscript. DR also contributed to the writing.
Funding
This work received support from the EU H2020-ICT-2019-3 project “HumanE AI Net” (grant agreement number 952 026).
Conflict of Interest
The authors declare that the research was conducted in the absence of any commercial or financial relationships that could be construed as a potential conflict of interest.
Publisher’s Note
All claims expressed in this article are solely those of the authors and do not necessarily represent those of their affiliated organizations, or those of the publisher, the editors and the reviewers. Any product that may be evaluated in this article, or claim that may be made by its manufacturer, is not guaranteed or endorsed by the publisher.
Supplementary Material
The Supplementary Material for this article can be found online at: https://www.frontiersin.org/articles/10.3389/fcomp.2021.792065/full#supplementary-material
Footnotes
1https://archive.ics.uci.edu/ml/datasets/opportunity+activity+recognition.
References
Avci, A., Bosch, S., Marin-Perianu, M., Marin-Perianu, R., and Havinga, P. (2010). “Activity Recognition Using Inertial Sensing for Healthcare, Wellbeing and Sports Applications: A Survey,” in Proc Int Conf on Architecture of Computing Systems (ARCS), 1–10.
Bock, M., Hölzemann, A., Moeller, M., and Van Laerhoven, K. (2021). “Improving Deep Learning for HAR With Shallow LSTMs,” in 2021 International Symposium on Wearable Computers (ACM). doi:10.1145/3460421.3480419
Bulling, A., Blanke, U., and Schiele, B. (2014). A Tutorial on Human Activity Recognition Using Body-Worn Inertial Sensors. ACM Comput. Surv. 46, 1–33. doi:10.1145/2499621
Cao, Z., Hidalgo, G., Simon, T., Wei, S.-E., and Sheikh, Y. (2021). OpenPose: Realtime Multi-Person 2d Pose Estimation Using Part Affinity Fields. IEEE Trans. Pattern Anal. Mach. Intell. 43, 172–186. doi:10.1109/tpami.2019.2929257
Chang, Y., Mathur, A., Isopoussu, A., Song, J., and Kawsar, F. (2020). A Systematic Study of Unsupervised Domain Adaptation for Robust Human-Activity Recognition. Proc. ACM Interact. Mob. Wearable Ubiquitous Technol. 4, 1–30. doi:10.1145/3380985
Chaquet, J. M., Carmona, E. J., and Fernández-Caballero, A. (2013). A Survey of Video Datasets for Human Action and Activity Recognition. Computer Vis. Image Understanding. 117, 633–659. doi:10.1016/j.cviu.2013.01.013
Chavarriaga, R., Sagha, H., Calatroni, A., Digumarti, S. T., Tröster, G., MillánMillán, J. d. R. J., et al. (2013). The Opportunity Challenge: A Benchmark Database for On-Body Sensor-Based Activity Recognition. Pattern Recognition Lett. 34, 2033–2042. doi:10.1016/j.patrec.2012.12.014
Chen, C., Jafari, R., and Kehtarnavaz, N. (2016). “Fusion of Depth, Skeleton, and Inertial Data for Human Action Recognition,” in 2016 IEEE International Conference on Acoustics, Speech and Signal Processing (ICASSP) (IEEE). doi:10.1109/icassp.2016.7472170
Chen, K., Zhang, D., Yao, L., Guo, B., Yu, Z., and Liu, Y. (2021). Deep Learning for Sensor-Based Human Activity Recognition. ACM Comput. Surv. 54, 1–40. doi:10.1145/3447744
Ciliberto, M., Roggen, D., and Morales, F. J. O. (2016). “Exploring Human Activity Annotation Using a Privacy Preserving 3d Model,” in Proceedings of the 2016 ACM International Joint Conference on Pervasive and Ubiquitous Computing: Adjunct (ACM). doi:10.1145/2968219.2968290
Demrozi, F., Pravadelli, G., Bihorac, A., and Rashidi, P. (2020). Human Activity Recognition Using Inertial, Physiological and Environmental Sensors: A Comprehensive Survey. IEEE Access. 8, 210816–210836. doi:10.1109/access.2020.3037715
Feuz, K. D., Cook, D. J., Rosasco, C., Robertson, K., and Schmitter-Edgecombe, M. (2015). Automated Detection of Activity Transitions for Prompting. IEEE Trans. Human-mach. Syst. 45, 575–585. doi:10.1109/thms.2014.2362529
Fortes Rey, V., Garewal, K. K., and Lukowicz, P. (2021). Translating Videos into Synthetic Training Data for Wearable Sensor-Based Activity Recognition Systems Using Residual Deep Convolutional Networks. Appl. Sci. 11, 3094. doi:10.3390/app11073094
Hiremath, S. K., and Ploetz, T. (2021). “On the Role of Context Length for Feature Extraction and Sequence Modeling in Human Activity Recognition,” in 2021 International Symposium on Wearable Computers (ACM). doi:10.1145/3460421.3478825
Kalabakov, S., Gjoreski, M., Gjoreski, H., and Gams, M. (2021). Analysis of Deep Transfer Learning Using DeepConvLSTM for Human Activity Recognition from Wearable Sensors. Ijcai. 45. doi:10.31449/inf.v45i2.3648
Kim, E., Helal, S., and Cook, D. (2010). Human Activity Recognition and Pattern Discovery. IEEE Pervasive Comput. 9, 48–53. doi:10.1109/mprv.2010.7
Kwon, H., Tong, C., Haresamudram, H., Gao, Y., Abowd, G. D., Lane, N. D., et al. (2020). IMUTube. Proc. ACM Interact. Mob. Wearable Ubiquitous Technol. 4, 1–29. doi:10.1145/3411841
Lee, S., and Eskofier, B. (2018). Special Issue on Wearable Computing and Machine Learning for Applications in Sports, Health, and Medical Engineering. Appl. Sci. 8, 167. doi:10.3390/app8020167
Lukowicz, P., Amft, O., Roggen, D., and Cheng, J. (2010). On-Body Sensing: From Gesture-Based Input to Activity-Driven Interaction. Computer. 43, 92–96. doi:10.1109/mc.2010.294
Morales, F. J. O., and Roggen, D. (2016). “Deep Convolutional Feature Transfer Across Mobile Activity Recognition Domains, Sensor Modalities and Locations,” in Proceedings of the 2016 ACM International Symposium on Wearable Computers (ACM). doi:10.1145/2971763.2971764
Noor, M. H. M., Salcic, Z., and Wang, K. I.-K. (2018). Ontology-Based Sensor Fusion Activity Recognition. J. Ambient Intell. Hum. Comput. 11, 3073–3087. doi:10.1007/s12652-017-0668-0
Nowak, S., and Rüger, S. (2010). “How Reliable Are Annotations via Crowdsourcing,” in Proceedings of the international conference on Multimedia information retrieval - MIR ’10 (New York, NY: ACM Press). doi:10.1145/1743384.1743478
Ordóñez, F., and Roggen, D. (2016). Deep Convolutional and LSTM Recurrent Neural Networks for Multimodal Wearable Activity Recognition. Sensors. 16, 115. doi:10.3390/s16010115
Patel, S., Park, H., Bonato, P., Chan, L., and Rodgers, M. (2012). A Review of Wearable Sensors and Systems With Application in Rehabilitation. J. Neuroengineering Rehabil. 9, 1–17. doi:10.1186/1743-0003-9-21
Pellatt, L., and Roggen, D. (2021). “Fast Deep Neural Architecture Search for Wearable Activity Recognition by Early Prediction of Converged Performance,” in 2021 International Symposium on Wearable Computers (ACM). doi:10.1145/3460421.3478813
Plotz, T., and Guan, Y. (2018). Deep Learning for Human Activity Recognition in Mobile Computing. Computer. 51, 50–59. doi:10.1109/mc.2018.2381112
Rey, V. F., Hevesi, P., Kovalenko, O., and Lukowicz, P. (2019). “Let There Be IMU Data,” in Adjunct Proceedings of the 2019 ACM International Joint Conference on Pervasive and Ubiquitous Computing and Proceedings of the 2019 ACM International Symposium on Wearable Computers (ACM). doi:10.1145/3341162.3345590
Roggen, D., Calatroni, A., Rossi, M., Holleczek, T., Forster, K., Troster, G., et al. (2010). “Collecting Complex Activity Datasets in Highly Rich Networked Sensor Environments,” in 2010 Seventh International Conference on Networked Sensing Systems (INSS) (IEEE). doi:10.1109/inss.2010.5573462
Roggen, D., Troster, G., Lukowicz, P., Ferscha, A., del R. MillanMillan, J. J., and Chavarriaga, R. (2013). Opportunistic Human Activity and Context Recognition. Computer. 46, 36–45. doi:10.1109/mc.2012.393
Sagha, H., Digumarti, S. T., delMillán, R. J., Calatroni, A., Roggen, D., Tröster, G., et al. (2011). “SMC 2011 Workshop on Robust Machine Learning Techniques for Human Activity Recognition: Activity Recognition challenge,” in Presented at IEEE International Conference on Systems, Man, and Cybernetics.
San-Segundo, R., Blunck, H., Moreno-Pimentel, J., Stisen, A., and Gil-Martín, M. (2018). Robust Human Activity Recognition Using Smartwatches and Smartphones. Eng. Appl. Artif. Intelligence. 72, 190–202. doi:10.1016/j.engappai.2018.04.002
Stiefmeier, T., Roggen, D., Ogris, G., Lukowicz, P., and Tr, G. (2008). Wearable Activity Tracking in Car Manufacturing. IEEE Pervasive Comput. 7, 42–50. doi:10.1109/mprv.2008.40
Thakker, U., Fedorov, I., Zhou, C., Gope, D., Mattina, M., Dasika, G., et al. (2021). Compressing RNNs to Kilobyte Budget for IoT Devices Using Kronecker Products. J. Emerg. Technol. Comput. Syst. 17, 1–18. doi:10.1145/3440016
Wu, T., Chen, Y., Gu, Y., Wang, J., Zhang, S., and Zhechen, Z. (2020). “Multi-layer Cross Loss Model for Zero-Shot Human Activity Recognition,” in Advances in Knowledge Discovery and Data Mining (Springer International Publishing), 210–221. doi:10.1007/978-3-030-47426-3_17
Keywords: multimodal dataset, wearble sensors, video dataset, human activity recognition, body tracking and recognition
Citation: Ciliberto M, Fortes Rey V, Calatroni A, Lukowicz P and Roggen D (2021) Opportunity++: A Multimodal Dataset for Video- and Wearable, Object and Ambient Sensors-Based Human Activity Recognition. Front. Comput. Sci. 3:792065. doi: 10.3389/fcomp.2021.792065
Received: 09 October 2021; Accepted: 19 November 2021;
Published: 20 December 2021.
Edited by:
Jean-Paul Calbimonte, University of Applied Sciences and Arts of Western Switzerland, SwitzerlandReviewed by:
Nadia Elouali, École supérieure en informatique de Sidi Bel Abbès, AlgeriaDalin Zhang, Aalborg University, Denmark
Copyright © 2021 Ciliberto, Fortes Rey, Calatroni, Lukowicz and Roggen. This is an open-access article distributed under the terms of the Creative Commons Attribution License (CC BY). The use, distribution or reproduction in other forums is permitted, provided the original author(s) and the copyright owner(s) are credited and that the original publication in this journal is cited, in accordance with accepted academic practice. No use, distribution or reproduction is permitted which does not comply with these terms.
*Correspondence: Mathias Ciliberto, bWNpbGliZXJ0b0BhY20ub3Jn