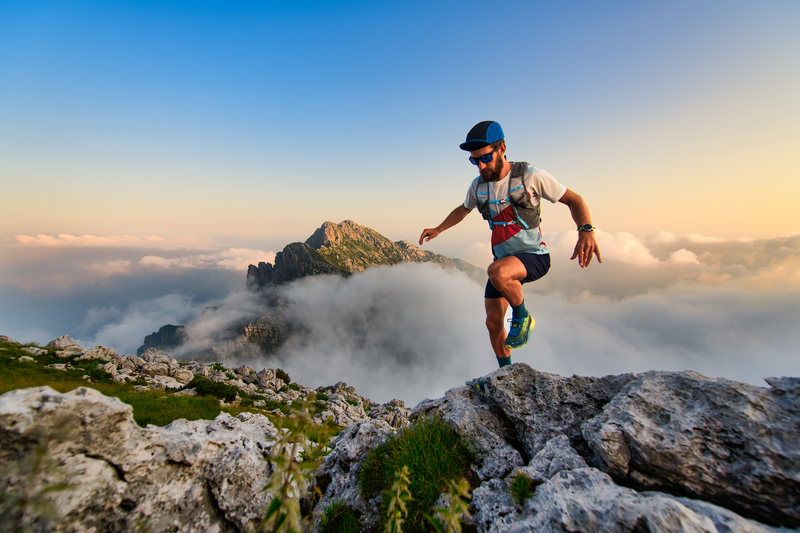
95% of researchers rate our articles as excellent or good
Learn more about the work of our research integrity team to safeguard the quality of each article we publish.
Find out more
SYSTEMATIC REVIEW article
Front. Sustain. Food Syst. , 25 February 2025
Sec. Agroecology and Ecosystem Services
Volume 9 - 2025 | https://doi.org/10.3389/fsufs.2025.1472109
Measuring the performance of food and agricultural systems is critical for their transformation towards a sustainable, healthy, and resilient future. To guide decisions and ensure agrifood systems deliver multiple functions, a holistic systems perspective is needed. Previous reviews of assessment approaches have focused primarily on the farm level and have been limited in their scope and definition of what it means to be holistic. In this review, we describe and evaluate 206 approaches based on four key characteristics of holistic systems assessment: (1) measuring multiple dimensions of performance, (2) integrating multiple stakeholder perspectives, (3) evaluating emergent system properties, and (4) collecting and presenting data in ways which reveal interactions, synergies, and trade-offs, so that they can be understood and considered when designing solutions. We find that there is recognition of the need for holistic assessment and a growing number of assessments are published each year. However, many assessments limit themselves to examining multiple dimensions of performance, neglecting the remaining three key characteristics of holistic assessment. While a systemic perspective is often acknowledged as important, only 14% of assessments considered synergies and trade-offs between metrics and 26% addressed emergent system properties. There is a trend toward more systemic framings such as agroecology and the inclusion of emergent properties. We conclude that there will never be one assessment approach that will work for everyone, can measure everything, and be used everywhere because of the diversity of agrifood systems and assessment objectives. Improving holistic assessment of agrifood systems is not a question of improving existing assessments. The gap to be addressed is the lack of methods for designing effective holistic systems assessments. This gap can be closed by providing clear guidance on how to navigate the abundance of existing approaches and develop assessments that meet specific needs. A meta-framework for guiding the development of holistic systems assessments, proposed in this review, can offer such guidance.
Food and agricultural (agrifood) systems—the ways we produce, distribute and consume food—are among the leading drivers of human impact on our environment (Rockström et al., 2020). Agriculture occupies 38% of the earth’s land area (Ramankutty et al., 2008), while our global food system is responsible for 21–37% of global greenhouse gases (Mbow et al., 2019), contributes to the loss of natural habitats and biodiversity (Zabel et al., 2019), and uses more water than any other human activity (FAO, 2011), yet still often fails to provide a healthy diet for all (Nature Food, 2023). Transforming our food systems globally offers powerful routes to addressing multiple global challenges simultaneously and contributing to the achievement of the United Nations Sustainable Development Goals (SDGs), both those directly related to food and others (Fanzo et al., 2021; Schneider et al., 2023).
The central role of agrifood systems in global challenges has prompted the development of innovative approaches such as regenerative agriculture, sustainable intensification, organic agriculture, and agroecology, which are gaining traction. Each of these approaches articulate and promote different principles and practices but share the common goal of fostering transitions towards more sustainable agrifood systems that can deliver both human and planetary health (HLPE, 2019). Currently these approaches account for only a small proportion of total agricultural production worldwide and questions remain whether such approaches can feed a growing population whilst preserving nature (Giller et al., 2021). There is particular scepticism about the productivity and profitability of approaches such as agroecology and regenerative agriculture, and consequently their potential to contribute to poverty alleviation for smallholder farmers and rural communities (Muhumuza, 2023). There are also questions as to whether some principles behind these approaches, such as input reduction, are universally applicable, especially for smallholder farmers in sub-Saharan Africa (Falconnier et al., 2023). We thus need better evidence on how agrifood systems can support better diets and resilient livelihoods while maintaining biodiversity, avoiding climate change and land degradation, and the tradeoffs between them (Geck et al., 2023).
Measuring the multifunctional performance of food and agricultural systems is widely seen as a necessary step in enabling agrifood systems transformation (Namirembe et al., 2022; Pope et al., 2004; Yakovleva, 2007; Zou et al., 2022). However, the complexity of agrifood systems means that measuring their performance is not easy, and common practice has been to measure a narrow set of indicators that are mainly focused on productivity and economic performance (Ali and Perna, 2021). Yet, sustainable approaches to agrifood systems, such as agroecology, aim to provide environmental and social benefits not just economic ones thus “to assess them only in short-term production or economic terms misses the whole point of the approach. Likewise, assessing conventionally intensified systems without including their longer-term social and environmental effects leads to degradation of those aspects” (Lamanna et al., 2024:1). Through conducting holistic assessments of agrifood systems, a level playing field for comparing the performance of alternative approaches can be created, helping policymakers, donors, development actors, and producers to make informed decisions.
The need for measuring multifunctional performance of agrifood systems has led to a proliferation of indicator-based assessment methods, particularly in relation to sustainability (Riley, 2001; Soulé et al., 2021). These assessment methods have the common aim of seeking to measure the multiple effects of food production, not just economic impacts, and typically use the concept of the ‘triple bottom line’ as their conceptual foundation. This concept refers to “the intersection between environment, society and economy” (Chopin et al., 2021; Giddings et al., 2002:187) or “planet, people, profit” (Miller, 2020), typically articulated in a combination and distinction of environmental, economic, and social domains or dimensions. To help people navigate the diversity and abundance of assessment methods, several authors have attempted to describe, classify and compare available methods and tools based on characteristics such as their purpose, dimensions addressed, methods used, complexity, and stakeholder involvement (Binder et al., 2010; Chopin et al., 2021; Coteur et al., 2020; de Olde et al., 2016; Douxchamps et al., 2017). These past reviews reveal that:
1. While assessments used to focus on a single dimension of performance in isolation, there has been a shift towards a more holistic and integrated approach. Multiple dimensions are now considered, including social and economic dimensions alongside the environmental (Ali and Perna, 2021; Binder et al., 2010; Coteur et al., 2020; Song et al., 2020).
2. Many assessments lack the involvement of those with an interest in using the resulting data in their development and application (Gharsallah et al., 2021; Zou et al., 2022).
3. Assessment approaches and tools are diverse. They differ in the dimensions and themes addressed along with their objectives, metrics, and intended users, reflecting the diverse perspectives on agrifood systems sustainability (Binder et al., 2010). A major conclusion from past reviews is thus that there is no one-size-fits-all assessment tool (Alrøe et al., 2016; Bonisoli et al., 2018; Marchand et al., 2014; Nadaraja et al., 2021; Schader et al., 2014).
In this review, we build on this literature and evaluate agrifood systems assessment methods to identify the gaps and opportunities for more holistic evaluation so as to better support food systems transformation. As far as we are aware, ours is the most extensive review of holistic systems assessments conducted in terms of the number of assessments, dimensions covered, scales considered and geographic scope. Although past reviews provide useful guidance on the strengths and weaknesses of different approaches, the majority of reviews have focused on a limited number of popular and widely used tools such as RISE (Häni et al., 2003), SAFA (FAO, 2014), IDEA (Zahm et al., 2006) and PG Tool (Gerrard et al., 2011). Chopin et al. (2021) have argued this risks overlooking the local development of assessment tools and those described within the academic literature. Further, most reviews focus on farm-level assessment tools (Alaoui et al., 2022; Arulnathan et al., 2020; Bonisoli et al., 2018; Coteur et al., 2020; de Olde et al., 2016; i.e., Marchand et al., 2014; Röös et al., 2019; Slätmo et al., 2017) or those developed for a specific geography or farming system (e.g., Streimikis and Baležentis, 2020 focuses on the European context). We aim to evaluate the extent to which current assessments adequately capture the holistic performance of agrifood systems based on a much broader definition of holism than past reviews.
It is widely acknowledged that integrated, holistic systems views are needed to understand the nature, functioning, and performance of food and agriculture systems (Slätmo et al., 2017). Many reviews state the importance of agrifood assessments being ‘holistic’ or ‘integrated’, yet most authors fail to clearly define what they mean by these terms (e.g., Talukder et al., 2020; Streimikis and Baležentis, 2020). When authors do define ‘holistic’, it is typically in reference to an assessment that considers multiple dimensions of sustainability, namely economic, social and environment (Coteur et al., 2020; de Olde et al., 2016; Marchand et al., 2014; Pope et al., 2004). Others may refer to holism and holistic assessment yet do not define them and it is implicit from their reviews that they refer to holism in terms of multidimensionality (Binder et al., 2010; Wohlenberg et al., 2020; Zou et al., 2022).
Multi-dimensionality is one important characteristic of holistic assessment. Recognising that a holistic approach is more than just measuring multiple dimensions in isolation, Lamanna et al. (2024) propose three additional characteristics that are important to include in a holistic assessment of agrifood systems. Below we describe these additional characteristics and their rationale.
1. The assessment “is conducted from multiple perspectives – as different actors in the system and users of the data are likely to assess system performance differently” (Lamanna et al., 2024:7). While most reviews frame holism in terms of measuring multiple dimensions, some consider holism to include the consideration of multiple stakeholder perspectives or performance at multiple levels of the system (Song et al., 2020; Talukder et al., 2020). Including multiple perspectives acknowledges that different actors possess unique connections to and understandings of agrifood systems, which in turn influence their decision-making and behaviors (Song et al., 2020). Moreover, some aspects of system performance are inherently subjective and depend on the observer. A claim to holism should therefore include the assessments of different people. While it is impossible to include all people and perspectives, an assessment claiming to be holistic must go beyond a single viewpoint. By doing so, holistic assessments can better account for the various ways in which different actors perceive and value system performance. This multi-perspective approach is most relevant for developing effective and inclusive policies that reflect the complex interplay between human values, behavior, and agrifood system performance (Song et al., 2020).
2. The assessment “generates insights into synergies and trade-offs in the system” (Lamanna et al., 2024:7). Agrifood systems are complex adaptive systems with interdependencies and interactions between social, economic, environmental, and political factors with feedback loops and nonlinearities (Prosperi et al., 2016). Working with complex adaptive systems where multiple components or parts interact and influence each other needs a holistic approach that considers these multiple aspects and dimensions. Yet what distinguishes a system from a collection of parts are the interactions and interdependencies between parts (Betley et al., 2021; Tittonell, 2023). This is why we cannot just look at multiple dimensions in isolation, instead we must also consider the interactions between them. As defined by Lamanna et al. (2024), a holistic assessment of system performance uses metrics and presents data in ways that reveal complexity, nuance, and trade-offs, so that they can be understood and considered when using results. Lamanna et al. (2024:7) also note, “synergy and trade-offs are not usually measured directly, but inferred during data analysis and interpretation. However, the intention to interpret data in this way has implications for the ways data are collected”.
3. The assessment “includes assessment of emergent properties that only appear at, or are defined at, the level of the system as a whole” (Lamanna et al., 2024:7). This characteristic, like the one before, is rooted in systems thinking and the understanding that agrifood systems are complex and adaptive, comprising numerous interconnected elements across various scales. As defined by Lamanna et al. (2024:7), “emergent properties of a complex system are properties of the system as a whole that are not properties of the constituent parts.” An example of an emergent system property is resilience (Prosperi et al., 2016)—“the ability of a system and its component parts to anticipate, absorb, accommodate, or escape from unacceptable standards of living due to the effects of a hazardous event, in a timely and efficient manner” (Douxchamps et al., 2017:11). Other examples include circularity, justice and sustainability. Systems theory highlights the importance of assessing such properties because they influence the overall functionality, adaptability, and stability of the system (Meadows, 2008). By assessing emergent properties such as resilience, we can better understand system-level outcomes and how agrifood systems adapt to disturbances and maintain their essential functions in the face of change. As Lamanna et al. (2024:7) note, “evidence on these aspects may be assembled from evidence on each of the separate dimensions but will need some additional assessments that would not be included if we were only interested in some of those individual dimensions”.
These additional characteristics were based on the idea that, while being ‘holistic’ implies assessing the whole system, it is never possible to measure ‘everything’, and choices must be made. However, a holistic assessment is not only one that measures a lot of different indicators, any of which might be measured in a more narrow assessment. These were identified by Lamanna et al. (2024) as three characteristics that extend the scope of an assessment beyond one that simply includes many indicators.
Taking a holistic systems perspective is necessary for the management of systems so that they provide all services expected and or better avoid negative consequences. Adaptive system management (for improved outcomes) is often the reason why people collect data and assess systems. It is said in relation to agrifood systems assessment, “only what gets measured gets management” (Linder et al., 2017; Rocchi et al., 2021). Primary purposes of collecting data are to determine if things are improving or getting worse, to help formulate policies, and to inform management decisions. Consequently, only those aspects that are measured, and thus managed, will improve over time—those that are not measured and thus remain unmanaged, may either improve or decline, irrespective of our goals (Stiglitz et al., 2018). Using a clear definition of holistic will move systems assessors closer to their goals and clearly stating the characteristics of holism means there is a greater chance of achieving holistic assessment.
In this review, we extend and apply a framework developed by Binder et al. (2010) and adapted by Chopin et al. (2021) to describe and evaluate 206 holistic systems assessments. This framework was originally developed for evaluating sustainability assessments and has since been widely used and adapted (Bonisoli et al., 2018; Chopin et al., 2021; de Olde et al., 2016; Marchand et al., 2014). Here, we extend this framework to include all four characteristics of holism outlined by Lamanna et al. (2024) – multiple dimensions, multiple perspectives, synergies and trade-offs, and emergent properties. While Lamanna et al. (2024) aims to provide a stepwise approach and design principles for developing a holistic systems assessment, in this review we use their framing of holism as an additional lens through which to evaluate existing assessments.
To identify existing holistic assessments, we searched two online databases: Web of Science (WoS) and CAB Abstracts. These two databases were chosen because they are large, high-quality, relevant databases and accessible to the research team. We explored these databases using a search string with three main components. The first component focused on terms relating to different sustainable agrifood systems approaches (building on approaches identified in HLPE, 2019), the second concerned their application to agrifood systems, and the third focused on terms relating to some form of assessment: TI = (Holistic OR Socio-ecological OR Social-ecological OR Sustainab* OR Agroecolog* OR Agro-ecolog* OR Regenerat* OR Organic OR Resilien* OR Climate-smart OR “Climate smart” OR Diversified OR Ecological OR Agrobiodivers* OR Agro-biodivers* OR Inclusi*) AND (Agro-ecosystem* OR Agricultur* OR farm* OR “Food systems” OR “Food system”) AND (Framework* OR Tool* OR Indicator* OR Metric* OR Assessment* OR Evaluat* OR Measur* OR Monitor* OR Index* OR Indices).
To limit the number of articles retrieved and improve article relevance, this search string was applied to the article title only. Article language was set to English and document type was set to article, review article, book or chapter. No restriction of year of publication was set. All searches were conducted on the 28th of September 2022. The database searches yielded 3,525 articles: 1,601 articles from WoS and 1,924 articles from CAB Abstracts. These retrieved articles were supplemented with holistic assessment methods already known to the research team, resulting in an additional 13 assessments being added to the review. Retrieved article data were imported into Mendeley reference management software (Mendeley, 2022) and duplicates removed before being exported to Rayyan (Ouzzani et al., 2016) – an online platform for systematic literature reviews—for further screening. Figure 1 provides an overview of the selection process using a PRISMA (Preferred Reporting Items for Systematic reviews and Meta-Analyses) flow diagram (Page et al., 2021). A total of 1,261 duplicate articles were removed and following the screening of abstracts and full texts, a further 2,071 articles were excluded at various stages, resulting in a final set of 206 assessments included in the review.
Given our focus on holistic assessment, we excluded assessments that did not assess all three dimensions – economic, environment and social – thus failing to meet our first characteristic of holistic assessment. In this review, we define ‘assessment’ or ‘assessment method’ to include “the diversity of approaches used in the literature (referred to as “approach,” “method,” “tool” or “framework”)” (Chopin et al., 2021:4) to assess the multifunctional performance of food and agricultural systems. This includes one-off and routine monitoring and performance data.
We discarded articles that were of poor quality or lacked sufficient information on their methodology, were reviews of assessment approaches, were irrelevant to agrifood systems, or were not deemed to be performance assessments (e.g., papers focused on the adoption rates of practices or suitability mapping). We also excluded ex ante evaluations using simulation models. Further, given that our unit of analysis was the assessment method, several articles referring to the same assessment approach (e.g., three articles referred to the use of the same approach) were treated as one entry in the final review. We included assessments irrespective of the geographies (e.g., low- to high-income countries, temperate, tropics), scale (plot, farm, landscape, national etc.) and farming systems (small-scale, commercial, pastoral etc.) for which they were developed.
The search and evaluation process used for the review was effective in that it generated a large number of diverse assessments. Importantly, the diversity of ways concepts such as sustainability and social components have been included in assessments are represented in our results and were not pre-determined by the search terms used. Such a review can never be complete and we acknowledge that the results are dependent on the decisions we made. For example, assessments for which there are no references in English will not have been captured. Likewise, assessments that aim to be holistic and systems oriented but that do not use any of our search terms to describe themselves will have been missed. As in most research projects, there is a lag between collecting data and publishing results. Our results should be complete up to time of data collection, but new assessments or new versions of existing assessments published since September 2022 have not been included.
Several frameworks have been developed for characterising and evaluating agrifood systems assessments, each with their own focus. While many focus on evaluating the characteristics of tools (e.g., Gasparatos and Scolobig, 2012; Schader et al., 2014), others have focused on the process of tool development (e.g., de Olde et al., 2016) or the implementation of assessment tools in terms of their ability to inform strategic decision-making (e.g., Coteur et al., 2020). In our review we extend and apply a framework developed by Binder et al. (2010) and adapted by Chopin et al. (2021) (see Supplementary Table S1 for details of the variables used in this review).
The framework originally outlined by Binder et al. (2010) has been widely adapted and used to review a range of characteristics and assessment tools (Bonisoli et al., 2018; e.g., de Olde et al., 2016; Linder et al., 2017). It was developed for evaluating sustainability assessments based on three interlinked dimensions: normative, systemic, and procedural (Binder et al., 2010). The normative dimension refers to “how to assess whether the studied system is sustainable” and covers aspects such as the goal of an assessment and underlying conceptual framing and theory (Binder et al., 2010:73). The systemic dimension refers to “whether a system is properly described by means of the set of indicators used” (Binder et al., 2010:73). This includes aspects such as the dimensions, themes and metrics used, which also fall under the normative dimension, but also whether an assessment captures relationships between metrics and potential trade-offs and synergies. Lastly, the procedural dimension covers “how the assessment was carried out” (Binder et al., 2010:73). This includes the methods used to collect data, the types of data collected, resource requirements and the user-friendliness of the tool. A more detailed description of these three dimensions and the rationale behind their associated variables can be found in Binder et al. (2010) and Chopin et al. (2021).
Given our focus on holistic systems assessment, we drew on the framework outlined by Binder et al. (2010) and its adaptation by Chopin et al. (2021) and extended it to include the four characteristics of holistic assessment proposed by Lamanna et al. (2024). In addition, we collected general information regarding each assessment, such as year of first publication, geographic focus, and whether they have been widely promoted.
In the following section we structure our results around four main sections. First, we provide an overview of the reviewed assessments in terms of their year of first publication, geographic focus and whether they have been widely promoted. This is followed by sections presenting findings on each of the three interlinked dimensions: normative, systemic and procedural. The dataset produced by this review is available online (see Crossland et al., 2024).
Over the past three decades, there has been an increase in the number of holistic assessments published annually, with notable growth after 2010 and peaks in 2020 and 2021 (Figure 2). Possible explanations for this trend include an increasing recognition of the role agrifood systems play in addressing multiple, interconnected global challenges, and achieving global goals such as the Millennium Development Goals (2000–2015) and Sustainable Development Goals (2015–2030). This recognition is underscored by the prominence of agrifood systems in high-level discussions, including the first-ever United Nations Food Systems Summit (UNFSS) held in 2021 and the 26th and 27th United Nations Climate Change Conference (COP) held in 2021 and 2022, respectively (Schneider et al., 2023).
Figure 2. Number of holistic agrifood systems assessments published annually between 1990 and 2022 (n = 205). Articles published in 2023 (n = 1) were excluded as they do not represent a full year of publications and thus do not allow for fair comparison between years.
Most assessments (87%) stated the geographic locations they had either been developed for or conducted in.1 A total of 73 individual countries were mentioned (Figure 3). The top five most mentioned countries were India (27%), Italy (22%), China (21%), Spain (16%) and Brazil (12%). India, China, and Brazil are among the most populous countries in the world (World Bank Group, 2023), potentially explaining the high numbers of assessments from these countries (i.e., more people, more research, more output). The high level of output from these countries could also reflect high levels of public interest in and government support for sustainable agrifood systems. For instance, India has a strong organic farming movement, and its government has launched several initiatives, such as Paramparagat Krishi Vikas Yojana (national organic agriculture scheme), to promote organic and sustainable farming practices.
Figure 3. Global heatmap of countries the reviewed assessments had either been developed for and/or deployed in. The table to the right of the map shows the top 15 most frequently mentioned countries (some assessments had been deployed in multiple countries).
Of the 206 assessments reviewed, 13% (26) were developed with the explicit intention of being globally relevant, albeit some of these assessments focused on a specific type of farming systems. For example, the Sustainable Intensification Assessment (SIA) developed by Musumba et al. (2017) despite having been developed to be widely applicable, focuses on smallholder farming systems in low-and middle-income countries.
When reviewing assessments, we made a distinction between two types of assessment: (1) promoted tools, that is, assessments developed with the explicit intention of being used by other users, and (2) non-promoted tools, that is, assessments developed for a specific research objective or study without the explicit intention of developing a tool for others to use, albeit we recognise that many researchers may well hope others will employ their approach once published.2 We chose to make this distinction based on the hypothesis that whether a framework is taken up depends not only on its inherent strengths but also on whether it is actively promoted and the influence of those promoting and supporting its use.
Of the 206 assessments reviewed, 30% (62) were classified as promoted tools. Several of these tools, for example, RISE, SAFA, PG Tool, IDEA and TAPE, regularly appear in past reviews of sustainable and holistic assessment approaches (e.g., Binder et al., 2010; Chopin et al., 2021; Röös et al., 2019). Of these 62 promoted tools, 77% (49) had a formal name by which they were known (see Supplementary Table S2). The most common tool developer was the United Nations Food and Agricultural Organisation (FAO) with eight named tools having been developed by the organisation, followed by Biovision Foundation for Ecological Development (Biovision) with three tools.
The conceptual framing of an assessment is crucial as it informs the choice of dimensions, themes, and metrics. It clarifies and helps identify what matters and what to measure. Based on our reading of assessment documentation, we deduced the main overarching concepts on which each assessment was framed and recorded whether authors explicitly used an existing conceptual framework from which to design their assessment.
The main concepts used to frame assessments were that of sustainability, followed by resilience, agroecology, and sustainable livelihoods (Figure 4). In terms of trends over time, we see an increase in the diversity of concepts and the emergence of more systemic framings (i.e., resilience, agroecology, vulnerability) from around 2014 (Figure 4).
Figure 4. Main framing concepts used by assessments over time. Articles published in 2023 (n = 1) were excluded as they do not represent a full year of publications and thus do not allow for a fair comparison between years. (Assessments could have multiple framings. Seven conceptual framings with only one occurrence are removed from the plot. These include: ecosystem services, soil health, stewardship, public goods, nutrition security, multifunctionality and food systems transformation).
Many assessments, although framed around the concept of sustainability, did not explicitly define sustainability in terms of the system they were evaluating. Few provided definitions beyond the simple ‘triple bottom line’ or generational view as stated in the Brundtland Report (WCED, 1987) (i.e., meeting long-term environmental, social, and economic needs without compromising the ability of future generations to meet their own needs). Similar to Binder et al. (2010) we found the most common definition of sustainability was in reference to the Brundtland Commission statement (WCED, 1987).
Of the 206 assessments, 24% (49) used or adapted an existing conceptual framework. The rest either developed their own (36%) or did not explicitly outline a framework (41%). Of those that adopted or adapted an existing framework, the most common frameworks were SAFA (7), MESMIS (5), Sustainable Development Goals (5), DPSIR (4), SAFE (3), Sustainable Livelihood Security Index (SLSI) (3), Sustainable livelihoods framework (3), ACT (2), and IDEA (2). Many of these frameworks are also included in our review as assessments themselves since they provide both a conceptual framework for developing an assessment (i.e., providing an analytical structure from which to develop an assessment) and an associated assessment with defined or suggested metrics. For example, SAFA provides a conceptual framework for developing an assessment in addition to an assessment and recommended metrics.
Given the need to address multiple dimensions, holistic assessments often use hierarchical or nested structures. These usually start with three main dimensions or pillars of sustainability – economic, environmental, and social (de Olde et al., 2016). Within each of these broad dimensions, there are usually several sub-dimensions or themes of interest, each with associated metrics for measuring performance. While this structure is common, not all assessments fall neatly into this three-tier structure. Some may only have one level of organisation while others may have many more layers of nesting. Similarly, although they may address social, economic, and environmental dimensions, they may use their own set of overarching dimensions. For example, the five capitals of sustainable livelihoods (i.e., physical, financial, human, natural and social). Given this variation in structure, categorising holistic systems assessments based on their dimensions and themes can be challenging. As noted by other authors (e.g., Chopin et al., 2021; Lamanna et al., 2024), these three dimensions are not discrete and not all themes fall neatly into this framing. For example, it is unclear in which dimension the theme ‘animal health’ falls in. Nevertheless, we attempted to classify the reviewed assessments using this three-tiered structure of dimensions, themes, and associated metrics.
Of the 116 (56%) assessments that included a second level of organisation, we see a huge number and diversity of the themes addressed (Figure 5), with 1,273 themes found across the 116 assessments, and a median of nine themes and a range of 2–41 themes per assessment. Each of the three dimensions had a similar number of associated themes with a total of 488 themes for the social dimension, 501 for the environmental dimension and 409 for the economic dimension. Nevertheless, based on the frequency of words used within each theme, we see that the environmental dimension has a higher number of commonly used terms within themes compared to the social and economic dimensions (Figure 5). This could suggest greater consensus among assessment developers over key environmental aspects compared to the social and economic dimensions.
Figure 5. Word clouds of the themes under each dimension—environment (A), social (B); economic (C). The size and color of words denote their frequency.
To further explore the thematic focus of the reviewed assessments, we mapped each assessment to the SDGs which they addressed (Figure 6). From this analysis, we see a greater number of assessments focusing on SDGs more directly related to food systems such “Zero hunger” (SDG 2), “No poverty” (SDG 1), “Life on land” (SDG 15), and “Climate action” (SDG 13), and less so to more social and institution-related goals such as “Reduced inequalities” (SDG 10), “Gender equality” (SDG 5), “Peace, justice and strong institutions” (SDG 16) and “Partnerships for the goals” (SDG 17).
Figure 6. The number of assessments that address each of the United Nations Sustainable Development Goals through their themes and metrics.
Of the 206 assessments reviewed, 89% (184) provided details of the metrics they used. The total number of metrics included in these assessments was 5,735 metrics.3 The median number of metrics per assessment was 24 with a range of 3 to 237 metrics per assessment. Each of the three dimensions had a similar number of metrics with a total of 2,087 metrics for the social dimension, 2,209 for the environmental dimension and 1,806 for the economic dimension. Most assessments (58%) stated that data availability and convenience were considered when selecting indicators. For example, metrics were chosen that could be extracted from existing secondary sources or information that farmers would be able to easily provide.
Co-designing assessments with those who have an interest in the results can increase the relevance and utility of an assessment and its outcomes. Various types of stakeholders may have an interest in the results of an assessment. These include: (1) the assessment users, that is, those who will use the results of the assessment; (2) those who may be influenced by actions taken because of the assessment findings, and (3) those who are part of the system being assessed, that is, the subjects of the assessment (Belebema et al., 2020).
Most assessments however did not explicitly distinguish between these different groups when referring to the level of stakeholder participation in their development. We therefore defined stakeholders in broad terms as groups other than the developers of the assessment, and classified assessments based on three levels of participation: “no participation” for assessments where the goal and metrics of the assessment were determined solely by the assessment developer/researcher, “consultation” for assessments where the assessment goal and metrics were initially designed by the developer/researcher and then stakeholders were consulted and their feedback incorporated, and “co-design” for assessments where stakeholders were engaged from the beginning and in determining the goal and metrics of the assessment.
Of the reviewed assessments, only 6% (13) were co-designed with stakeholders from the beginning. Most were either designed solely by developers (47%) or sought stakeholder feedback after the initial design of the assessment (47%). We also see that over time the frequency of assessments which have been co-designed has remained low (Figure 7). The most common stakeholders involved in the development of the reviewed assessments (be it through co-design or consultation) were farmers or producers (67), researchers (63), government representatives (31), unspecified multi-stakeholder groups (26) and agricultural advisors and extensionists (25) (Table 1). Fifty-six percent (115) of assessments selected metrics based on literature review, 41% (84) were researcher selected, and 36% (74) in consultation with experts. Only 30% (61) of assessments took a participatory approach to selecting indicators, including target users and other actors in the selection process. Most relied on reviewing literature (56%), followed by expert consultation (35%). While both promoted and unpromoted had a similar percentage of co-designed (6%), a higher percentage of promoted tools (60%) consulted users in the design and development of the assessment and tool compared to non-promoted tools (41%). Of the 206 assessments, 48% (98) incorporated locally defined indicators.
Figure 7. Number of assessments published each year by the approach taken to their design and development.
Table 1. Stakeholders involved in the development of assessments either through co-design or consultation (n = 108).
Of the 186 (90%) assessments that presented results, the main methods used for evaluating assessment output included the use of a scoring system (115), composite indices (41), indicator ranges, thresholds or reference values (22), stakeholder or self-assessment (4) and various statistical approaches (76), varying from simple descriptive statistics to more complex analyses such as cluster analysis and multivariate analyses.
Many assessments (73%) aggregated metrics to form either a score or composite indices. Across these assessments, there were two main approaches used for weighting. Most of these assessments (65%) weighted each dimension equally when calculating an overall score or index. Those who did not use equal weighting (34%) mostly relied on consultation with researchers or experts to develop weights.
Of the reviewed assessments, 123 (60%) presented results visually (Table 2). The most common types of visual display were radar plots – also known as spiderweb plots – and bar plots. Radar plots are used to display multivariate data, where each variable is represented on axes originating from the same point and were commonly used when an assessment used a scoring system or composite indices. Such assessments include the PG Tool developed by Gerrard et al. (2011).
Despite many of the reviewed assessments emphasising the importance of considering the multifunctionality of agrifood systems and the need to consider the complex relationships between metrics, the number of assessments that evaluate interactions has remained low over time (Figure 8). Only 29 (14%) of assessments considered interactions and relationships between metrics. Those that did, did so using correlation or regression analysis or multivariate methods such as Principal Component Analysis (PCA).
Figure 8. Number of assessments published each year that consider interactions, trade-offs and synergies between metrics in their analysis (A). Number of assessments published each year that included themes related to emergent system properties such as resilience, adaptability, stability, equality, equity, fairness, empowerment, peace, and security (B).
Emergent properties such as resilience, equity, and empowerment, arise from the complex interactions amongst components within systems. Although some measures have been developed for some of these properties, they can be difficult to measure directly. Of the reviewed assessments, 53 (26%) assessments included themes that used terms related to emergent system properties. These terms included resilience, adaptability, stability, equality, equity, fairness, empowerment, peace, and security. However, the number of assessments that include themes related to emergent system properties has increased over time (Figure 8). While assessments including these themes were few before 2013, they have consistently featured in assessments since then. Nevertheless, as a proportion of total assessments published the number of assessments including such themes has remained relatively low.
Looking at changes in system performance over time rather than a one-off snapshot can provide important insight into a system’s trajectory and response to shocks, stresses, and management changes. Measuring performance over time is critical for evaluating emergent system properties such as resilience. Yet, most assessments (73%) were used to provide one-off snapshots of performance or to compare the performance of two or more cases at a single point in time. Only 21% (44) of the assessments had been used multiple times and looked at change over time (11 assessments were classed as ‘unknown’ due to lack of detail on their methodology).
The most common intended users of the reviewed assessments (i.e., those that will use the results of the assessment), regardless of whether the assessment was classified as a promoted tool or not, were researchers (66%, 135), policymakers (63%, 121), and farmers or producers (53%, 109) (Figure 9). The three most common combinations of intended users were researchers and policymakers (14%, 28), researchers (11%, 22), policymakers (10%, 21) and researchers and farmers (9%, 19).
Figure 9. Upset plot of target users of assessments. Upset plots use a matrix-based layout to show data from multiple response questions (Conway et al., 2017). The bottom left bar chart shows the total number of assessments targeting each user group (set). Given that assessments could specify multiple target users the dot plot displays the various answer combinations (intersections), and the upper bar chart shows the number of assessments using each answer combination (intersection size).
In terms of the scale of measurement (i.e., the scale at which most measurements are taken) and scale of reporting (i.e., the scale at which assessment results are analysed and reported) of assessments, we see a greater focus on spatially-and geographical-related scales, such as field, farm, landscape, and nation, rather than more systems-related scales such as value chain, business, project/programme, and food system (Table 3). We also see a greater number of assessments focused on the farm scale and production stage of agrifood systems, with a limited number of assessments focusing on the retail and consumption stages.
The most common data collection method used by assessments was questionnaires administered to farmers, producers, and other actors such as business owners (Figure 10). This was followed by data from secondary sources such as national censuses or existing, publicly available household survey data (e.g., European Union’s Farm Structure Survey data). The dominance of questionnaires as the main data collection instrument is reflected by the types of data collected with quantitative data estimated by those administering the questionnaire or provided by the questionnaire respondent being used in 61% (123) of reviewed assessments (Table 4). Only 21% (44) of assessments required direct field data collection and 4% (9) utilised remote sensing data. The most common combinations of methods were questionnaire and secondary data (11), questionnaire and field measurements (10) and questionnaire and interviews (9) (Figure 10).
We deduced the methodological complexity of assessments based on categories outlined by Kaufmann et al. (2023:22): (1) “basic complexity” – being assessments that are “generally applicable for professionals working in the environmental or agricultural sector” and “can immediately be applied with no or very little additional training” and “no or limited interdisciplinary knowledge and expertise is necessary”; (2) “intermediate complexity” – being assessments that are “generally applicable for professionals working in the environmental or agricultural sector with advanced experience” and where “some additional training is necessary to become familiar with these methods” and “interdisciplinary knowledge and expertise are favourable”; and (3) “high complexity” – assessments that require “extensive training or the use of an external expert is necessary” and where “interdisciplinary knowledge and expertise are necessary.” The review team categorised assessments based on their own interpretation and judgement of this criteria.
Of the reviewed assessments 48% (98) were classified as having intermediate complexity, 31% (63) as having basic complexity, and 18% (38) as having high complexity. Seven assessments (3%) were classified as “unknown” in terms of their complexity due to a lack of details regarding the assessment methodology.
The time required for data collection was rarely stated in the documentation of the reviewed assessments. Instead, we estimated the required time to collect data per unit of observation (e.g., the time required to administer a questionnaire to a single household or respondent) based on the methods used and types of data collected. The categories used were those outlined by Chopin et al. (2021): “low” (<2 h), “medium” (2–7 h or one working day) and “high” (>1 day of data collection).” Of the reviewed assessments, 21% (44) were thought to have high collection time, 32% (65) as having a medium collection time, and 25% (52) as having a low collection time. 22% (45) of assessments were unclassified due to a lack of detail requiring data collection methods.
In addition to the collection methods and data types collected, we evaluated assessments in terms of the reproducibility of their results. Here, we defined an assessment as reproducible by judging whether one would expect to get the same result if the assessment were repeated by more than one person (e.g., would you get the same results if the same farm was assessed by two different enumerators). Assessments likely to be classified as non-reproducible were those whose metrics were dependent on the value judgement of the person conducting the assessment and likely to vary depending on the perceptions of the individual carrying it out. For example, TAPE relies on several subjective metrics such as whether a farm has many trees or not. Based on this definition of reproducibility, 39% (81) of assessments were categorised as being reproducible, and 35% (72) were not. 26% (53) were classified as “unknown” due to lack of detail regarding metrics used.
For promoted tools, we evaluated their accessibility and the availability of guidance information for potential users. Of the 62 promoted tools, 86% (53) were open access, in that they could be used by anyone and without payment, 10% (6) had restricted access (i.e., users needed to be a member or part of a project to access the tool), and 5% (3) required payment to access and use them. In terms of available guidance, 24% (15) had an online platform for tool users, 79% (49) provided guidance for potential users on how to carry out the assessment, 71% (44) on approaches to data analysis and 63% (39) on how to interpret assessment results. Only 11% (7) provided potential users with guidance on data governance (i.e., considerations on how data is used, who owns data and who has access).
There has been a surge in assessments of food and agriculture systems since 2010, likely due to the growing awareness of agrifood systems as both a source of and solution to many of the complex challenges we face globally. For such assessments to effectively guide decisions and enable agrifood systems transformation, a holistic systems perspective is needed. This includes: (1) measuring multiple dimensions, (2) integrating multiple perspectives, (3) collecting and presenting data in ways which reveal complexity, nuance, and trade-offs, and (4) capturing emergent system properties. The following discussion is structured around these four characteristics of holistic systems assessment and discusses the implications of our findings for future frameworks and assessment of agrifood systems.
Although there is nothing wrong with an assessment focusing on a particular issue of interest and selecting themes and metrics based on what matters to them, certain dimensions may receive more attention than others. This risks important aspects of agrifood systems performance receiving less attention and contributes to the ‘level playfield’ problem described earlier. Selective measurement of a system opens the possibility of the designer of the assessment influencing the results.
It is widely claimed that social dimensions of holistic assessments remain relatively underdeveloped compared to the economic and environmental dimensions (de Olde et al., 2016; Röös et al., 2019; Schader et al., 2014; Slätmo et al., 2017; Springer et al., 2015). Springer et al. (2015) and Nadaraja et al. (2021) found that assessments contain higher numbers of metrics related to the environmental dimension compared to the social and economic dimensions. Of the 47 indicators reviewed by Nadaraja et al. (2021), 29% pertained to social, 20% to economic, and 5% to governance aspects. In contrast, 47% of indicators were related to the environmental dimension.
We found no substantial differences in the number of metrics for each dimension but observed greater diversity in the themes associated with the social dimension. This finding aligns with other studies and suggests lack of consensus over how and what to measure within this dimension (Chopin et al., 2021; Janker and Mann, 2020; Springer et al., 2015; Wohlenberg et al., 2020). This reflects the diverse and subjective nature of many social dimensions of sustainability or a lack of consensus on how to define ‘social sustainability’ and difficulties in developing quantifiable metrics (Janker and Mann, 2020; Latruffe et al., 2016; Springer et al., 2015). What social sustainability means and entails differs around the world and across agricultural systems, geographies, and scales (Janker and Mann, 2020). An illustrative example is Röös et al. (2019) who reported that two popular farm-level assessment tools (SAFA and IDEA) struggled to capture the social conditions of Swedish farmers and had limited relevance to the social context of farming in Sweden.
Using social components of SDGs as a guide, our analysis uncovered several social themes that have received less attention by existing assessments. We found that far fewer assessments focused on the more social and institutional-related goals such as “Reduced Inequalities” (SDG 10), “Gender Equality” (SDG 5), “Peace, Justice, and Strong Institutions” (SDG 16), and “Partnerships for the Goals” (SDG 17), compared to those directly related to environmental and economic outcomes, such as “No Poverty” (SDG 1), “Life on Land” (SDG 15), and “Climate Action” (SDG 13). Additionally, a common reason for the exclusion of assessments from our review was their failure to incorporate aspects of the social dimension (see Figure 1).
It is possible that designers of holistic assessments of agrifood systems do not see such social issues as characteristics of the system they are able to influence and change. Aspects such as peace, justice and equity are determined by socio-political systems larger than those which agricultural researchers tend to focus on and are able to influence and thus such aspects may be deemed beyond the scope of their assessments.
These findings echo those of Zou et al. (2022), who identified ‘governance’ as the most neglected dimension at the food systems level. Similarly, at the farm level, Chopin et al. (2021) noted that few sustainability assessments used a ‘governance-oriented’ framing. Nevertheless, we speculate that the consideration of social and governance related aspects such as equity, peace, food sovereignty, and the intrinsic and relational values of nature will become more prevalent in the assessment of agrifood systems as more systemic framings which include a greater emphasis on social dimensions, such as agroecology, continue to gain traction.
It is widely claimed that the development of sustainability assessments should involve those who will use or be affected by the results and that they should be involved early and throughout, from the assessment design to the interpretation of results (Alrøe et al., 2016; Chopin et al., 2021; Schindler et al., 2015). Yet, our findings indicate that the stated intended users of assessments—farmers, researchers and policy makers—are seldom involved from the beginning. Instead, their input is often solicited after the goals and metrics of an assessment have been established, raising questions about the extent to which an assessment can inform and impact their decisions. In line with Arulnathan et al. (2020), we also found a severe lack of information on how users were engaged in the development of the reviewed assessments and whether their views were taken on-board. Most descriptions of how assessments were developed simply listed the types of actors consulted.
For holistic assessment to support transitions to sustainable agrifood systems, they need to be used and inform people’s decisions and actions. Yet, at least in the case of farm-level sustainability assessment, the uptake and use of tools by end users has been limited (Binder et al., 2010; de Olde et al., 2016; Triste et al., 2014). Triste et al. (2014) emphasise the importance of how a tool is developed rather than the content of the tool itself. They claim that the limited uptake of the MOTIFS tool by farmers is due to failings in the design process and insufficient engagement with end users (Triste et al., 2014). Farmers interviewed by de Olde et al. (2016), despite seeing relevance in the farm-level assessment tools, were sceptical as to whether the results were useful for informing their decisions. The farmers questioned whether the results provided new knowledge (i.e., the assessment did not tell them anything they did not already know) and reported that they “felt restricted in their opportunities to improve their sustainability due to the complexity of the system they are a part of” (de Olde et al., 2016:396).
Co-designing assessments with the intended users of the resulting data is likely to increase the relevance and utility of an assessment and its outcomes (Alrøe et al., 2016). Such assessments are more likely to meet the needs of their intended users, increasing the likelihood of wider uptake and use. Anyone organising and promoting an assessment needs to be realistic about whose needs are really being served by it, and the fact that farmers, while at the heart of an agricultural system, may not see the need for additional data. Participation can also lead to greater sense of ownership and allow for negotiating multiple user needs and interests (Namirembe et al., 2022; Schindler et al., 2015).
Care is needed when deciding who, how and when to engage different interest groups in the development of a holistic assessment. Such decisions determine the quality of participation and its outcomes (Reed, 2008). For example, evidence from co-production research indicates that stakeholders do not necessarily want to be included in every single step of the research process, rather they prefer to be consulted in strategic ways (Bieluch et al., 2017). Others warn of “consultation fatigue” among stakeholders who are “increasingly asked to take part in participatory processes that are not always well run, and as they perceive that their involvement gains them little reward or capacity to influence decisions that affect them” (Reed, 2008). Whether to engage those who are affected by the outcome of an assessment is another key decision (Reed, 2008). On the one hand, involving such actors is likely to increase their trust in assessment results and subsequent decisions. On the other, it may be impractical to involve stakeholders at this level. For example, involving everyone who may be impacted by the outcome of tracking progress on the UN SDGs in assessment design is neither feasible – since it includes everyone in the world – nor necessarily desirable as not everyone who is part of a system wants or needs data about its performance.
Assessing from multiple perspectives does not only imply involving stakeholders in assessment design. Data on many of the indicators used in holistic assessments are collected as subjective responses from individuals and depend on those individual experiences and values. The levels of these indicators will depend on who is answering. Taking an example from TAPE, rating of the amount of stress animals experience will be done differently by farmers and an animal welfare expert, and access to new knowledge rated differently by a farmer and extension officer. A holistic assessment that takes account of multiple perspectives would measure these different perspectives. Although many of the assessments collected self-reported data from farmers and used local indicators (indicators based on what local actors use to evaluate their system), we did not explicitly score for whether they captured multiple perspectives on the same aspect of system performance. Nevertheless, none of the reviewed assessments stood out as consistently generating data from multiple perspectives or stated this as an explicit aim.
A system is distinguished from a collection of parts by the interactions and interdependencies between those parts (Betley et al., 2021). If we are to understand and manage a system as a whole, it is inadequate to examine multiple dimensions in isolation. Instead, we must understand their interactions, trade-offs and synergies, as well as their associated themes and metrics (Binder et al., 2010; Capello and Nijkamp, 2002; Van Passel et al., 2007). Understanding synergies and tradeoffs is in part a function of how the data are analysed and presented. However, there are also implications for the design and implementation of data collection. For example, it will usually be necessary to ensure that all variables used in a tradeoff analysis have been collected on the same set of units (e.g., farms) at the same time. If understanding is to go beyond correlation, then evidence on the functional connection between system components will also need to be collected. Like Soulé et al. (2021), Binder et al. (2010), and Bonisoli et al. (2018), we found that, despite acknowledging the importance of interactions, few assessments analysed interactions. It is common practice to use numerical and visual integration in the form of composite indices and graphs such as radar charts. Both approaches allow for easy communication of assessment results. Yet, they can also hide the complexities and interactions between indicators. For example, the metrics displayed using a radar chart are not linked together and trade-offs and synergies are not shown despite being displayed next to each other.
Similarly, composite indices involve the integration of multiple sub-indicators into one single numerical indicator and are thought to be attractive to policy makers because they can be easily and quickly communicated and are interpretable by a wide audience (i.e., a higher score is ‘better’ than a lower score) (Siva Muthuprakash and Damani, 2017; Van Passel and Meul, 2012). Yet, composite indices are unable to provide an understanding of system dynamics as they do not reveal anything about the interactions between metrics (Roy et al., 2019). They can hide important details regarding the complexity of an issue and lack transparency on what sub-indicators may be driving an overall score (Latruffe et al., 2016; Van Passel et al., 2007). Further, they can obscure the differences in scores between systems. For example, two systems may have the same score, yet it is unclear if the systems are fundamentally similar or simply happen to have the same values of composite indicator (Peano et al., 2015).
The methods used to compute such composite indices are often subjective or arbitrary (Siva Muthuprakash and Damani, 2017). As highlighted by de Olde et al. (2016), even experts in each field can disagree markedly on the importance of different indicators. The use of composite indices thus requires transparency in how they are developed and if composite indices are used, they should not be presented in isolation from their more detailed sub-indicators (Magrini and Giambona, 2022; Van Passel and Meul, 2012).
Of the assessments we reviewed that did consider interactions, most used some form of correlation analysis between different indicators and dimensions (e.g., Rodrigues et al., 2010). To ensure such analysis is possible, data must be collected in ways that allow for interactions to be considered. In addition to the co-measurement requirement described above, there needs to be some basis for assuming that a correlation describes interaction and is not spurious. At the same time, given that assessment results need to be understandable and relatable to those making the decisions if they are to influence decisions, we need to find simple ways of communicating interactions and their complexity (Shields et al., 2002; Van Passel and Meul, 2012).
What happens after the assessment is perhaps more important than the assessment design itself in terms of changing practice and moving towards sustainability. The need for support in interpreting results to inform action has been clearly articulated in the case of farm-level tools and farmers decision making (Marchand et al., 2014). Coteur et al. (2020) reported that despite an awareness among tool developers of the importance of supporting farmers in interpreting assessment results and providing advice, only eight of the 18 tools that they reviewed provided support in interpreting results and recommendations based on results. This gap is also noted by de Olde et al. (2018), with many developers focusing on the methodology of a tool rather than how assessment results will be used by users and influence decision making. Ensuring that the presentation of results is interpretable by the assessment audience is key for action and influencing decision making. Given that different audiences will often have different preferences on how data is presented (Bourne et al., 2021) it is crucial for assessments to consider options in data representation (Lamanna et al., 2024). The use of online platforms for automating the analysis and visualisation of data collected using promoted tools could help increase the usability and interpretation of assessment results by those who lack data analysis skills. Yet we found that online platforms are uncommon. Most promoted tools we reviewed were open access and provide guidance on their use for generating data, but less so on interpretation and how to use the results of the assessment.
The design of a holistic assessment is challenged by the number of system components and processes that could be included in the measurement scheme. However, a holistic view includes understanding the system as a whole—its emergent properties—not only each of its components. Emergent properties of agrifood systems, such as resilience, equity, and empowerment, result from complex interactions among system components across scales. Prosperi et al. (2016) argue that concepts like resilience and vulnerability offer a more effective framework for assessing food systems, and hence determining what should be measured and monitored, than system components alone. Only 26% of the assessments that we reviewed addressed themes related to such emergent system properties, perhaps because it is difficult to do so. For instance, despite increasing interest in resilience and development of several indicators for its measurement (Douxchamps et al., 2017), measuring resilience remains challenging given its varied definitions, multidimensional nature and need for the inclusion of multi-spatial and temporal scales (Prosperi et al., 2016). Resilience first requires clear definition in the context of the system being assessed, the measurement at multiple levels and scales, yet most assessments of resilience focus on one scale (Douxchamps et al., 2017).
Evaluating changes in system performance over time, rather than through a single snapshot, can provide important insight into a system’s trajectory and response to shocks, stresses, and management changes (Chopin et al., 2021). Measuring resilience necessitates the measurement of a system over time (Douxchamps et al., 2017). Nevertheless, we found that most assessments are used to provide one-off snapshots of performance or to compare the performance of two or more cases at a single point in time, even if the authors hope their measurement scheme will be taken up and used repeatedly. In their review of farm-level assessment tools, Marchand et al. (2014) present a potential trade-off between how comprehensive or complex an assessment is and how easy or quick it is to use. On one end, there are full assessments aimed at providing high accuracy and often complex evaluations, and on the other end, there are rapid assessments aimed at providing a quick picture and overview with lower complexity and accuracy. Neither end point is strictly defined since it is never possible to assess everything and unclear when a simplified assessment stops being holistic. However, there are real choices to make. This trade-off closely relates to other trade-offs identified in the literature, including scope versus precision (Schader et al., 2014) and breadth versus depth (Namirembe et al., 2022). Complexity of an assessment tool has implications for the cost, time, and skill requirements for carrying out an assessment. This, in turn, has implications for using a method repeatedly or routinely to monitor change and data collection by non-specialists such as activists or communities themselves. For instance, the more complex a tool is the less likely it is to be widely taken up (Marchand et al., 2014).
In our review, we classed most assessments as having ‘medium complexity’ and having a ‘medium’ time requirement, in that they are “generally applicable for professionals working in the environmental or agricultural sector with advanced experience” and where “some additional training is necessary to become familiar with these methods” (Kaufmann et al., 2023:22) and take 2 to 7 h to complete. This prevalence of medium complexity and time requirement could reflect a recognition of this trade-off between complexity and useability among tool developers and efforts to find a middle ground.
Nevertheless, this compromise can come at the expense of assessment reliability and validity. Of the assessments we reviewed, 34% were classed as being non-reproducible, in that you might not get the same result if they were to be repeated by more than one person, and over half of the assessments we reviewed relied on questionnaires and recall data rather than field measurements. Subjective data is liable to biases and limits the utility of a metric for evaluating agrifood systems approaches on a level playing field. There are potential sources of bias in objective measurements too, but they are usually easier to manage by design.
Assessments that were classified as non-reproducible were often those where metrics used were dependent on value judgements and likely to vary depending on the perceptions of those carrying out the assessment. For example, TAPE relies on several subjective metrics such as whether a farm has many trees or not. As noted by Marchand et al. (2014), more objective measures also allow for greater comparability across cases and the potential development of benchmarks. Yet tools that are quick and rapid to apply often rely on respondent recall and perception which may reduce the accuracy and correctness of an assessment (Coteur et al., 2020; Marchand et al., 2014).
Coteur et al. (2020) and Payraudeau and van der Werf (2005) note that there is often a trade-off between the use of performance-based indicators and feasibility. System indicators can often be described as either (1) practice-based indicators that describe what is done in the system, such as practices used by a farmer, and (2) performance-based indicators that measure what the system is doing in social, economic or environmental dimensions. The latter provides direct evidence of the system function. The former are often interpreted as indicators of performance based on an assumption that use of a practice will lead to specific performance (Coteur et al., 2020; FAO, 2014). FAO (2014) proposes that performance-based indicators, given their closeness to reality and the impact of interest, should be prioritised over practice-based indicators.
We note that one person’s practice-based indicator may be another’s performance-based indicator. For example, consider the level and diversity of tree cover. It is a description of a system and not its performance. However, for someone interested in the biodiversity value of an agricultural landscape and aiming to increase tree cover, the level of tree cover could well be a performance indicator. The tensions between assessment scope and accuracy, breadth and depth, and complexity and use ability, mean trade-offs will need to be made. These should be done with the assessment objectives and intended users clearly in mind and involved in the development of the assessment.
The 206 holistic agrifood system assessment frameworks, methods, and examples that we identified and reviewed display a wide diversity of approaches and practices. The reason is simple: there is no one assessment tool that will work for everyone and new concepts and purposes of assessment are continually emerging. Previous, less extensive reviews have reached similar conclusions (Alaoui et al., 2022; Arulnathan et al., 2020; Bonisoli et al., 2018; Marchand et al., 2014; Nadaraja et al., 2021; Schader et al., 2014; Van Passel and Meul, 2012). The diversity of themes and metrics employed by assessments is to be expected since agrifood systems encompass so much. For instance, while one assessment may focus on measuring soil health as the key environmental dimension, another may focus on measuring biodiversity. Neither of these two assessments is right or wrong in their selection of themes and metrics. They are different because they were developed for different users, each with different objectives and perspectives on what is important to measure and how to measure it (Alaoui et al., 2022; Chopin et al., 2021; Coteur et al., 2020; Gasparatos and Scolobig, 2012; Janker and Mann, 2020; Marchand et al., 2014). As Alaoui et al. (2022) note, different groups have different reasons for using farm-level assessment tools. Farmers may use them for evaluating the performance of their own farm; agricultural advisors for advising farmers on how to improve their sustainability; and researchers for comparing farm performance across different cases, regions, or countries (Alaoui et al., 2022). The same will be true for any other scale of assessment.
The development of conceptual bases for assessments is one driver of development of new methods. Among the assessments we reviewed, holism first appeared as a frame in 1997, agroecology in 2004, resilience in 2008, ecosystem services in 2011, climate adaptation and sustainable intensification in 2015, and vulnerability in 2019 (see Figure 4). This trend of conceptual development will inevitably continue, resulting in further innovation in assessment methods.
The objectives of an assessment also influence the level at which it is conducted. For instance, farmers and agricultural advisors may have a greater interest in assessments conducted at the field or farm level, while national governments and international bodies may have greater interest in assessments conducted at the regional, national, or sectoral level. We found that most assessments focused on the farm scale and production stage of agrifood systems. This focus on farm-level assessment could reflect a potential bias in our search terms. It could also reflect the fact that many decisions over agricultural production are made by farmers at the farm level and thus the farm level is often identified as an entry point for influencing system change (Marchand et al., 2014). Further, measuring performance at the field and farm level is often easier than measurement at higher levels (e.g., landscape, regional, national) making it a likely focus for research projects trying new ideas with limited resources. In addition, many metrics collected at farm level can be aggregated to provide a view at higher levels but the converse is not true. Nevertheless, as Gharsallah et al. (2021) note, there are important aspects of agrifood systems performance that cannot be scaled in this way such as ecosystem services that emerge at landscape scale, water regulation, or biodiversity protection. IDEA4 (Zahm et al., 2024) is an example of an assessment that is based explicitly on a multiple scales, with a framework that merges farmers’ self-centred goals with wider interests of the community, country and rest of the world.
The appeal of universal system indicators is obvious. We have extensive databases of conventional production and economic data about food and agriculture systems that are global in extent and aim to be globally comparable (e.g., FAOStat). Recognising that these are inadequate for giving a holistic picture of systems leads to demands for assessments and indicators that will fill the gap (Béné et al., 2019; Schneider et al., 2023). However, the utility of a globally relevant assessment tool is limited given the diversity of assessment objectives and varied nature of agrifood systems (Binder et al., 2010; Dong et al., 2016; Hayati et al., 2010). We identified numerous promoted or ‘readymade’ tools developed with the intention of being globally applicable and meeting the needs of multiple audiences (e.g., farmers, researchers, and policy makers). Despite many of these tools being co-designed, they are unlikely to meet all users’ aims and need context specificity. For instance, TAPE (FAO, 2019) was developed with the intention of being a global tool, yet Namirembe et al. (2022) in their evaluation of using TAPE across multiple projects and contexts conclude, that “it is not a readymade approach or set of tools to use in every situation” and that “the same will be true for any other ‘readymade’ assessment tool that involves processes, indicators, and tools that have been defined without reference to the specific context and objectives.” In recognition that no one tool fits all, several authors have argued for combining tools or using them sequentially to address different scales and user needs, thus catering to the various “layers and players” involved in an assessment (Alrøe et al., 2016; Van Passel and Meul, 2012).
Wide scale adoption of new standards for system measurement depends on the power of people demanding it. UN agencies, for example, have power and can influence what data is collected—for example, most countries report national progress on sustainable development goals and international assistance is available to help in that effort. In our review we noted most of the ‘promoted’ assessment approaches and tools (those behind which there is a concerted effort to persuade others to use them) are developed by international bodies and NGOs. The most common tool developer was the United Nations Food and Agricultural Organisation (FAO) with eight named tools having been developed by the organisation, followed by Biovision Foundation with three tools. Whether a framework is taken up depends not only on its inherent strengths but also on who is promoting and supporting it and the influence of those groups. This applies at national as well as international scales, with states having the authority to impose adoption of an approach and provide resources to implement it.
The ideas of technology lock-in (Foxon, 2013) also play a role in whether a new assessment tool will be used. There are always reasons to keep doing what we are doing rather than something new, even when the new approach is demonstrably superior. Foxon (2013) identifies four sources of technology lock-in: economies of scale, learning and skills, perceptions of success and network effects. All these will apply to assessment approaches and hence we see a method such as TAPE being widely used even if it is not necessarily the most appropriate in each case.
Many past reviews aimed at identifying deficiencies and gaps to be filled and call for the development of new assessment approaches and metrics. This thinking—that we or anyone else will be able to fill such gaps—is flawed. There are and always will be gaps to holistic agrifood systems assessment. While the appeal of universal methods and indicators is obvious, creating a new standard that everyone will use is unlikely given the diversity of assessment objectives, and varied and unlimited nature of agrifood systems. There will never be one tool or approach that will work for everyone, can measure everything and be used everywhere. There is large diversity and continuous innovation and adaptation in the assessment of agrifood systems. There will always be new frameworks, concepts and perspectives on agrifood systems assessment emerging. Improving the holistic assessment of agrifood systems is therefore not a question of improving or combining existing tools to meet the vast needs and interests of different users. Instead, the gap to be addressed is the lack of guidance for setting up and designing effective holistic systems assessments.
There are two broad options for someone wanting to conduct a holistic systems assessment: (1) to select or adapt an existing tool or combination of tools to best fit their needs; or (2) to develop their own tool. Several past reviews have aimed to provide guidance on how to select the right assessment tool based on needs (e.g., Bonisoli et al., 2018). Yet, there is no guarantee that such a tool already exists given the diversity of global agrifood systems and user objectives.
Lamanna et al. (2024) describe the second approach. While they include the design principle of “do not reinvent the wheel” in their guidance (i.e., if there is already a tool that meets the assessment objectives, then use it), they also outline a flexible stepwise approach to developing holistic systems assessments that meet specific user needs. This stepwise approach has several advantages, one of which is the emphasis on clearly articulating the purpose of the assessment and identifying an appropriate conceptual framing. Objectives and conceptual framing drive the effective design of assessments (Namirembe et al., 2022). Yet, like Siva Muthuprakash and Damani, 2017 and Janker and Mann (2020), we found that many assessments lack a clearly articulated conceptual framework on which their selection of themes and metrics are based, with the risk that indicators are selected because they ‘might be interesting’ rather than because they contribute to a consistent view. Following a stepwise approach to developing a holistic systems assessment, with a strong emphasis on theoretical framing and clearly articulated goals, can help ensure that what matters is measured (i.e., the choice of what to measure is determined by what users care about) and that context-specific aspects are not overlooked.
An argument against designing individual context-specific assessment frameworks is that resulting datasets will not be comparable across time and contexts. However, if that is a requirement or aim, then it becomes part of the design criteria (e.g., monitoring progress towards SDGs where coherence is needed) (Rosenstock et al., 2017). There may also be a tension and balance to be made between measuring what a user cares about (i.e., “what matters”), which is often value-based, and selecting a holistic conceptual frame that is logical, coherent and complete. Nevertheless, those interested in holistic assessment need clear guidance to navigate the abundance of existing frameworks and develop assessments that meet their needs while avoiding the extremes of narrowly focused metrics or attempting to measure everything simultaneously. Frameworks for selecting and developing assessments, such as that outlined by Lamanna et al. (2024), could offer such guidance.
The datasets presented in this study can be found in online repositories. The names of the repository/repositories and accession number(s) can be found below: https://doi.org/10.34725/DVN/BVVKE2.
MC: Conceptualization, Data curation, Formal analysis, Investigation, Methodology, Visualization, Writing – original draft, Writing – review & editing. RC: Conceptualization, Formal analysis, Investigation, Methodology, Writing – original draft, Writing – review & editing. CL: Conceptualization, Formal analysis, Investigation, Methodology, Writing – review & editing. BC: Conceptualization, Data curation, Formal analysis, Investigation, Methodology, Writing – review & editing. LO: Conceptualization, Data curation, Formal analysis, Investigation, Methodology, Visualization, Writing – review & editing. BA: Conceptualization, Data curation, Formal analysis, Investigation, Methodology, Writing – review & editing. SK: Formal analysis, Writing – review & editing. VM: Conceptualization, Data curation, Formal analysis, Investigation, Methodology, Writing – review & editing. EA: Data curation, Formal analysis, Investigation, Writing – review & editing. LF: Conceptualization, Formal analysis, Investigation, Methodology, Writing – review & editing. AK: Data curation, Formal analysis, Investigation, Writing – review & editing. MG: Conceptualization, Formal analysis, Investigation, Methodology, Writing – review & editing.
The author(s) declare financial support was received for the research, authorship, and/or publication of this article. The project on Holistic Metrics for Food and Agricultural Systems Performance (Metrics) under the Agroecological Transitions Program for Building Resilient and Inclusive Agricultural and Food Systems (TRANSITIONS), is funded by the European Commission through its DeSIRA initiative and managed by the International Fund for Agricultural Development (IFAD). This research was conducted by the Metrics project under the European Commission’s grant agreement 2000003774.
The authors would like to thank Jaika Gaylord for his assistance in data collection. Additionally, we appreciate the thoughtful input on initial findings provided by Dave Mills and Carlos Barahona.
The authors declare that the research was conducted in the absence of any commercial or financial relationships that could be construed as a potential conflict of interest.
All claims expressed in this article are solely those of the authors and do not necessarily represent those of their affiliated organizations, or those of the publisher, the editors and the reviewers. Any product that may be evaluated in this article, or claim that may be made by its manufacturer, is not guaranteed or endorsed by the publisher.
The Supplementary material for this article can be found online at: https://www.frontiersin.org/articles/10.3389/fsufs.2025.1472109/full#supplementary-material
1. ^Although some assessments were developed for use within a specific country (e.g., Brazilian Multidimensional Index for Sustainable Food Systems developed by de Carvalho et al., 2021), we recognise that many developers hope for broad, perhaps universal, relevance and application of their assessments, yet may test them in only one or two places.
2. ^Assessments are likely to go through a process of development, from identifying an issue, developing concepts, to piloting methods and mainstreaming approaches. The reviewed assessments fall at different points along this continuum, with our classification of ‘promoted tools’ representing assessments at the later end of this spectrum.
3. ^This does not necessarily equate to 5,735 unique metrics since assessments may have used different terms and wording for the same or similar metrics.
Alaoui, A., Barão, L., Ferreira, C. S. S., and Hessel, R. (2022). An overview of sustainability assessment frameworks in agriculture. Land 11:537. doi: 10.3390/land11040537
Ali, A., and Perna, S. (2021). Sustainability indicators in agriculture: a review and bibliometric analysis using Scopus database. J. Agric. Environ. Int. Dev. 115, 5–21. doi: 10.36253/jaeid-12083
Alrøe, H. F., Moller, H., Læssøe, J., and Noe, E. (2016). Opportunities and challenges for multicriteria assessment of food system sustainability. Ecol. Soc. 21:art38. doi: 10.5751/ES-08394-210138
Arulnathan, V., Heidari, M. D., Doyon, M., Li, E., and Pelletier, N. (2020). Farm-level decision support tools: a review of methodological choices and their consistency with principles of sustainability assessment. J. Clean. Prod. 256:120410. doi: 10.1016/j.jclepro.2020.120410
Belebema, M., Jekums, A., Mcleod, R., Obst, C., and Sharma, K. (2020). Applying the Teebagrifood evaluation framework (issue September) : Global Alliance for the Future of Food.
Béné, C., Prager, S. D., Achicanoy, H. A. E., Toro, P. A., Lamotte, L., Bonilla, C., et al. (2019). Global map and indicators of food system sustainability. Sci. Data 6:279. doi: 10.1038/s41597-019-0301-5
Betley, E., Sterling, E. J., Akabas, S., Paxton, A., and Frost, L. (2021). Introduction to systems and systems thinking, vol. 11. Network of Conservation Educators and Practitioners, Center for Biodiversity and Conservation, American Museum of Natural History.
Bieluch, K. H., Bell, K. P., Teisl, M. F., Lindenfeld, L. A., Leahy, J., and Silka, L. (2017). Transdisciplinary research partnerships in sustainability science: an examination of stakeholder participation preferences. Sustain. Sci. 12, 87–104. doi: 10.1007/s11625-016-0360-x
Binder, C. R., Feola, G., and Steinberger, J. K. (2010). Considering the normative, systemic and procedural dimensions in indicator-based sustainability assessments in agriculture. Environ. Impact Assess. Rev. 30, 71–81. doi: 10.1016/j.eiar.2009.06.002
Bonisoli, L., Galdeano-Gómez, E., and Piedra-Muñoz, L. (2018). Deconstructing criteria and assessment tools to build Agri-sustainability indicators and support farmers’ decision-making process. J. Clean. Prod. 182, 1080–1094. doi: 10.1016/j.jclepro.2018.02.055
Bourne, M., Neely, C., Magaju, C., Lamanna, C., Peterson, N., Wanyama, R., et al. (2021). Enhancing policy and strategy planning: How to tailor data visualisation and evidence sharing for improved stakeholder uptake and application. CIFOR-ICRAF. Available at: https://www.cifor-icraf.org/knowledge/publication/25864/
Capello, R., and Nijkamp, P. (2002). In search of sustainable human settlements: prefatory remarks. Ecol. Econ. 40, 151–155. doi: 10.1016/S0921-8009(01)00251-8
Chopin, P., Mubaya, C. P., Descheemaeker, K., Öborn, I., and Bergkvist, G. (2021). Avenues for improving farming sustainability assessment with upgraded tools, sustainability framing and indicators. A review. Agron. Sustain. Dev. 41:19. doi: 10.1007/s13593-021-00674-3
Conway, J. R., Lex, A., and Gehlenborg, N. (2017). UpSetR: an R package for the visualization of intersecting sets and their properties. Bioinformatics (Oxford, England) 33, 2938–2940. doi: 10.1093/bioinformatics/btx364
Coteur, I., Wustenberghs, H., Debruyne, L., Lauwers, L., and Marchand, F. (2020). How do current sustainability assessment tools support farmers’ strategic decision making? Ecol. Indic. 114:106298. doi: 10.1016/j.ecolind.2020.106298
Crossland, M., Orero, L., Adoyo, B., Mwangi, V., Anyango, E., Gaylord, J., et al. (2024). Dataset for measuring the holistic performance of food and agricultural systems: A systematic review : World agroforestry (ICRAF).
de Carvalho, A. M., Verly, E., Marchioni, D. M., and Jones, A. D. (2021). Measuring sustainable food systems in Brazil: a framework and multidimensional index to evaluate socioeconomic, nutritional, and environmental aspects. World Dev. 143:105470. doi: 10.1016/j.worlddev.2021.105470
de Olde, E. M., Oudshoorn, F. W., Sørensen, C. A. G., Bokkers, E. A. M., and de Boer, I. J. M. (2016). Assessing sustainability at farm-level: lessons learned from a comparison of tools in practice. Ecol. Indic. 66, 391–404. doi: 10.1016/j.ecolind.2016.01.047
de Olde, E. M., Sautier, M., and Whitehead, J. (2018). Comprehensiveness or implementation: Challenges in translating farm-level sustainability assessments into action for sustainable development. Ecol. Indic. 85, 1107–1112. doi: 10.1016/j.ecolind.2017.11.058
Dong, F., Mitchell, P. D., Knuteson, D., Wyman, J., Bussan, A. J., and Conley, S. (2016). Assessing sustainability and improvements in US Midwestern soybean production systems using a PCA–DEA approach. Renew. Agric. Food Syst. 31, 524–539. doi: 10.1017/S1742170515000460
Douxchamps, S., Debevec, L., Giordano, M., and Barron, J. (2017). Monitoring and evaluation of climate resilience for agricultural development – a review of currently available tools. World Dev. Perspect. 5, 10–23. doi: 10.1016/j.wdp.2017.02.001
Falconnier, G. N., Cardinael, R., Corbeels, M., Baudron, F., Chivenge, P., Couëdel, A., et al. (2023). The input reduction principle of agroecology is wrong when it comes to mineral fertilizer use in sub-Saharan Africa. Outlook Agric. 52, 311–326. doi: 10.1177/00307270231199795
Fanzo, J., Haddad, L., Schneider, K. R., Béné, C., Covic, N. M., Guarin, A., et al. (2021). Viewpoint: rigorous monitoring is necessary to guide food system transformation in the countdown to the 2030 global goals. Food Policy 104:102163. doi: 10.1016/j.foodpol.2021.102163
FAO (2011). The state of the world’s land and water resources for food and agriculture (SOLAW) – Managing systems at risk. Earthscan: Food and Agriculture Organization.
FAO. (2014). SAFA guidelines: sustainability assessment of food and agriculture systems version 3.0. Food and Agriculture Organization.
FAO. (2019). Tool for Agroecology performance evaluation (TAPE)—test version. Food and Agriculture Organization. Available at: https://openknowledge.fao.org/handle/20.500.14283/ca7407en
Foxon, T. J. (2013). “Technological lock-in” in Encyclopedia of energy, natural resource, and environmental economics. ed. J. F. Shogren (Elsevier Science), 123–127.
Gasparatos, A., and Scolobig, A. (2012). Choosing the most appropriate sustainability assessment tool. Ecol. Econ. 80, 1–7. doi: 10.1016/j.ecolecon.2012.05.005
Geck, M. S., Crossland, M., and Lamanna, C. (2023). Measuring agroecology and its performance: an overview and critical discussion of existing tools and approaches. Outlook Agric. 52, 349–359. doi: 10.1177/00307270231196309
Gerrard, C., Smith, L., Padel, S., Hitchings, R., Measures, M., and Cooper, N. (2011). OCIS public goods tool development : Organic Research Centre.
Gharsallah, O., Gandolfi, C., and Facchi, A. (2021). Methodologies for the sustainability assessment of agricultural production systems, with a focus on Rice: a review. Sustain. For. 13:11123. doi: 10.3390/su131911123
Giddings, B., Hopwood, B., and O’Brien, G. (2002). Environment, economy and society: fitting them together into sustainable development. Sustain. Dev. 10, 187–196. doi: 10.1002/sd.199
Giller, K. E., Hijbeek, R., Andersson, J. A., and Sumberg, J. (2021). Regenerative Agriculture: An agronomic perspective. Outlook Agric. 50, 13–25. doi: 10.1177/0030727021998063
Häni, F., Braga, F., Stämpfli, A., Keller, T., and Porsche, H. (2003). RISE, a tool for holistic sustainability assessment at the farm level. International Food and Agribusiness Management Review. 6, 78–90. doi: 10.22004/ag.econ.34379
Hayati, D., Ranjbar, Z., and Karami, E. (2010). “Measuring agricultural sustainability” in Biodiversity, Biofuels, Agroforestry and Conservation Agriculture eds. E. Lichtfouse Sustainable Agriculture Reviews, vol 5. Springer, Dordrecht.
HLPE (2019). Agroecological and other innovative approaches for sustainable agriculture and food systems that enhance food security and nutrition : High Level Panel of Experts on Food Security and Nutrition of the Committee on World Food Security.
Janker, J., and Mann, S. (2020). Understanding the social dimension of sustainability in agriculture: a critical review of sustainability assessment tools. Environ. Dev. Sustain. 22, 1671–1691. doi: 10.1007/s10668-018-0282-0
Kaufmann, J., Cartsburg, M., and Staubach, L. (2023). “Analyses of socio-economic and environmental effects of agroecological practices” Deutsche Gesellschaft für Internationale Zusammenarbeit (GIZ). Available at: https://www.giz.de/en/downloads/giz2023-en-measuring-socio-economic-effects-of-agroecology.pdf
Lamanna, C., Coe, R., Crossland, M., Fuchs, L. E., Barahona, C., Chiputwa, B., et al. (2024). Developing holistic assessments of food and agricultural systems: A meta-framework for metrics users : CIFOR-ICRAF.
Latruffe, L., Diazabakana, A., Bockstaller, C., Desjeux, Y., Finn, J., Kelly, E., et al. (2016). Measurement of sustainability in agriculture: a review of indicators. Stud Agric Econ 118, 123–130. doi: 10.7896/j.1624
Linder, M., Sarasini, S., and van Loon, P. (2017). A metric for quantifying product-level circularity. J. Ind. Ecol. 21, 545–558. doi: 10.1111/jiec.12552
Magrini, A., and Giambona, F. (2022). A composite Indicator to assess sustainability of agriculture in European Union countries. Soc. Indic. Res. 163, 1003–1036. doi: 10.1007/s11205-022-02925-6
Marchand, F., Debruyne, L., Triste, L., Gerrard, C., Padel, S., and Lauwers, L. (2014). Key characteristics for tool choice in indicator-based sustainability assessment at farm level. Ecol. Soc. 19:art46. doi: 10.5751/ES-06876-190346
Mbow, C., Rosenzweig, C., Barioni, L. G., Benton, T. G., Herrero, M., Krishnapillai, M., et al. (2019). “Food security” in Climate change and land: An IPCC special report on climate change, desertification, land degradation, sustainable land management, food security, and greenhouse gas fluxes in terrestrial ecosystems. eds. P. R. Shukla, J. Skea, E. Calvo Buendia, V. Masson-Delmotte, and H. O. Pörtner (IPCC).
Mendeley. (2022). Mendeley desktop (version 1.19.8) [computer software]. Available at: https://www.mendeley.com
Miller. (2020). The triple bottom line: What it is & why It’s important. Business insights blog. Available at: https://online.hbs.edu/blog/post/what-is-the-triple-bottom-line
Muhumuza, J. R. (2023). Why current agroecology rhetoric stands to protract farmer poverty in the developing world. Outlook Agric. 52, 303–310. doi: 10.1177/00307270231195381
Musumba, M., Grabowski, P., Palm, C., and Snapp, S. (2017). Guide for the sustainable intensification assessment framework (SSRN scholarly paper 3906994).
Nadaraja, D., Lu, C., and Islam, M. M. (2021). The sustainability assessment of plantation agriculture—a systematic review of sustainability indicators. Sustain. Prod. Consump. 26, 892–910. doi: 10.1016/j.spc.2020.12.042
Namirembe, S., Mhango, W., Njoroge, R., Tchuwa, F., Wellard, K., and Coe, R. (2022). Grounding a global tool—principles and practice for agroecological assessments inspired by TAPE. Elementa 10:00022. doi: 10.1525/elementa.2022.00022
Nature Food (2023). The triple burden of malnutrition. Nat. Food 4:925. doi: 10.1038/s43016-023-00886-8
Ouzzani, M., Hammady, H., Fedorowicz, Z., and Elmagarmid, A. (2016). Rayyan—a web and mobile app for systematic reviews. Syst. Rev. 5:210. doi: 10.1186/s13643-016-0384-4
Page, M. J., McKenzie, J. E., Bossuyt, P. M., Boutron, I., Hoffmann, T. C., Mulrow, C. D., et al. (2021). The PRISMA 2020 statement: an updated guideline for reporting systematic reviews. BMJ 372:n71. doi: 10.1136/bmj.n71
Payraudeau, S., and van der Werf, H. M. G. (2005). Environmental impact assessment for a farming region: a review of methods. Agric. Ecosyst. Environ. 107, 1–19. doi: 10.1016/j.agee.2004.12.012
Peano, C., Tecco, N., Dansero, E., Girgenti, V., and Sottile, F. (2015). Evaluating the sustainability in complex Agri-food systems: the SAEMETH framework. Sustain. For. 7, 6721–6741. doi: 10.3390/su7066721
Pope, J., Annandale, D., and Morrison-Saunders, A. (2004). Conceptualising sustainability assessment. Environ. Impact Assess. Rev. 24, 595–616. doi: 10.1016/j.eiar.2004.03.001
Prosperi, P., Allen, T., Cogill, B., Padilla, M., and Peri, I. (2016). Towards metrics of sustainable food systems: a review of the resilience and vulnerability literature. Environ. Syst. Decis. 36, 3–19. doi: 10.1007/s10669-016-9584-7
Ramankutty, N., Evan, A. T., Monfreda, C., and Foley, J. A. (2008). Farming the planet: 1. Geographic distribution of global agricultural lands in the year 2000. Glob. Biogeochem. Cycles 22:2007GB002952. doi: 10.1029/2007GB002952
Reed, M. S. (2008). Stakeholder participation for environmental management: a literature review. Biol. Conserv. 141, 2417–2431. doi: 10.1016/j.biocon.2008.07.014
Riley, J. (2001). The indicator explosion: local needs and international challenges. Agric. Ecosyst. Environ. 87, 119–120. doi: 10.1016/S0167-8809(01)00271-7
Rocchi, L., Paolotti, L., Cortina, C., Fagioli, F. F., and Boggia, A. (2021). Measuring circularity: an application of modified material circularity Indicator to agricultural systems. Agric. Food Econ. 9:9. doi: 10.1186/s40100-021-00182-8
Rockström, J., Edenhofer, O., Gaertner, J., and DeClerck, F. (2020). Planet-proofing the global food system. Nat. Food 1, 3–5. doi: 10.1038/s43016-019-0010-4
Rodrigues, G. S., Rodrigues, I. A., Buschinelli, C. C. d. A., and de Barros, I. (2010). Integrated farm sustainability assessment for the environmental management of rural activities. Environ. Impact Assess. Rev. 30, 229–239. doi: 10.1016/j.eiar.2009.10.002
Röös, E., Fischer, K., Tidåker, P., and Nordström Källström, H. (2019). How well is farmers’ social situation captured by sustainability assessment tools? A Swedish case study. Int. J. Sustain. Dev. World Ecol. 26, 268–281. doi: 10.1080/13504509.2018.1560371
Rosenstock, T. S., Lamanna, C., Chesterman, S., Hammond, J., Kadiyala, S., Luedeling, E., et al. (2017). When less is more: innovations for tracking progress toward global targets. Curr. Opin. Environ. Sustain. 26-27, 54–61. doi: 10.1016/j.cosust.2017.02.010
Roy, R., Gain, A. K., Samat, N., Hurlbert, M., Tan, M. L., and Chan, N. W. (2019). Resilience of coastal agricultural systems in Bangladesh: assessment for agroecosystem stewardship strategies. Ecol. Indic. 106:105525. doi: 10.1016/j.ecolind.2019.105525
Schader, C., Grenz, J., Meier, M. S., and Stolze, M. (2014). Scope and precision of sustainability assessment approaches to food systems. Ecol. Soc. 19:art42. doi: 10.5751/ES-06866-190342
Schindler, J., Graef, F., and König, H. J. (2015). Methods to assess farming sustainability in developing countries. A review. Agron. Sustain. Dev. 35, 1043–1057. doi: 10.1007/s13593-015-0305-2
Schneider, K. R., Fanzo, J., Haddad, L., Herrero, M., Moncayo, J. R., Herforth, A., et al. (2023). The state of food systems worldwide in the countdown to 2030. Nat. Food 4, 1090–1110. doi: 10.1038/s43016-023-00885-9
Shields, D. J., Šolar, S. V., and Martin, W. E. (2002). The role of values and objectives in communicating indicators of sustainability. Ecol. Indic. 2, 149–160. doi: 10.1016/S1470-160X(02)00042-0
Siva Muthuprakash, K. M., and Damani, O. P. (2017). A stock and flow based framework to identify indicators for a holistic comparison of farming practices. Agric. Res. 6, 248–258. doi: 10.1007/s40003-017-0266-6
Slätmo, E., Fischer, K., and Röös, E. (2017). The framing of sustainability in sustainability assessment frameworks for agriculture. Sociol. Rural. 57, 378–395. doi: 10.1111/soru.12156
Song, B., Robinson, G., and Bardsley, D. (2020). Measuring multifunctional agricultural landscapes. Land 9:260. doi: 10.3390/land9080260
Soulé, E., Michonneau, P., Michel, N., and Bockstaller, C. (2021). Environmental sustainability assessment in agricultural systems: a conceptual and methodological review. J. Clean. Prod. 325:129291. doi: 10.1016/j.jclepro.2021.129291
Springer, N. P., Garbach, K., Guillozet, K., Haden, V. R., Hedao, P., Hollander, A. D., et al. (2015). Sustainable sourcing of global agricultural raw materials: assessing gaps in key impact and vulnerability issues and indicators. PLoS One 10:e0128752. doi: 10.1371/journal.pone.0128752
Stiglitz, J., Fitoussi, J., and Durand, M. (Eds.). (2018). For good measure: Advancing research on well-being metrics beyond GDP. OECD Publishing. Available at: https://www.oecd-ilibrary.org/content/publication/9789264307278-en
Streimikis, J., and Baležentis, T. (2020). Agricultural sustainability assessment framework integrating sustainable development goals and interlinked priorities of environmental, climate and agriculture policies. Sustain. Dev. 28, 1702–1712. doi: 10.1002/sd.2118
Talukder, B., Blay-Palmer, A., van Loon, G. W., and Hipel, K. W. (2020). Towards complexity of agricultural sustainability assessment: Main issues and concerns. Environ. Sustain. Indicat. 6:100038. doi: 10.1016/j.indic.2020.100038
Triste, L., Marchand, F., Debruyne, L., Meul, M., and Lauwers, L. (2014). Reflection on the development process of a sustainability assessment tool: learning from a Flemish case. Ecol. Soc. 19. doi: 10.5751/ES-06789-190347
Van Passel, S., and Meul, M. (2012). Multilevel and multi-user sustainability assessment of farming systems. Environ. Impact Assess. Rev. 32, 170–180. doi: 10.1016/j.eiar.2011.08.005
Van Passel, S., Nevens, F., Mathijs, E., and Van Huylenbroeck, G. (2007). Measuring farm sustainability and explaining differences in sustainable efficiency. Ecol. Econ. 62, 149–161. doi: 10.1016/j.ecolecon.2006.06.008
WCED (1987). World commission on environment and development: Our common future : Oxford University Press, World Commission on Environment and Development.
Wohlenberg, J., Schneider, R. C. S., and Hoeltz, M. (2020). Sustainability indicators in the context of family farming: a systematic and bibliometric approach. Environ. Eng. Res. 27:200545, –200540. doi: 10.4491/eer.2020.545
World Bank Group (2023). Population, total. Available at: https://data.worldbank.org/indicator/SP.POP.TOTL
Yakovleva, N. (2007). Editorial introduction: measuring the sustainability of the food system. J. Environ. Policy Plan. 9, 1–3. doi: 10.1080/15239080701254842
Zabel, F., Delzeit, R., Schneider, J. M., Seppelt, R., Mauser, W., and Václavík, T. (2019). Global impacts of future cropland expansion and intensification on agricultural markets and biodiversity. Nat. Commun. 10:2844. doi: 10.1038/s41467-019-10775-z
Zahm, F., Ugaglia, A. A., Barbier, J. M., Carayon, D., Delhomme, B., Gafsi, M., et al. (2024). Assessing farm sustainability: the IDEA4 method, a conceptual framework combining dimensions and properties of sustainability. Cahiers Agric. 33:10. doi: 10.1051/cagri/2024001
Zahm, F., Viaux, P., Girardin, P., Vilain, L., and Mouchet, C. (2006). Farm sustainability assessment using the IDEA method. INFASA Symposium.
Keywords: metrics, indicators, multidimensional, farming systems, sustainability assessment, complex systems assessment, integrated assessment, emergent system properties
Citation: Crossland M, Coe R, Lamanna C, Chiputwa B, Orero L, Adoyo B, Kumar S, Mwangi VM, Anyango E, Fuchs LE, Kuria A and Geck M (2025) Measuring the holistic performance of food and agricultural systems: a systematic review. Front. Sustain. Food Syst. 9:1472109. doi: 10.3389/fsufs.2025.1472109
Received: 28 July 2024; Accepted: 06 February 2025;
Published: 25 February 2025.
Edited by:
Vassilios D. Litskas, Independent Researcher, Lefkosia, CyprusReviewed by:
Raquel Ajates, Universidad Nacional de Educación a Distancia (UNED), SpainCopyright © 2025 Crossland, Coe, Lamanna, Chiputwa, Orero, Adoyo, Kumar, Mwangi, Anyango, Fuchs, Kuria and Geck. This is an open-access article distributed under the terms of the Creative Commons Attribution License (CC BY). The use, distribution or reproduction in other forums is permitted, provided the original author(s) and the copyright owner(s) are credited and that the original publication in this journal is cited, in accordance with accepted academic practice. No use, distribution or reproduction is permitted which does not comply with these terms.
*Correspondence: Mary Crossland, bS5jcm9zc2xhbmRAY2lmb3ItaWNyYWYub3Jn
Disclaimer: All claims expressed in this article are solely those of the authors and do not necessarily represent those of their affiliated organizations, or those of the publisher, the editors and the reviewers. Any product that may be evaluated in this article or claim that may be made by its manufacturer is not guaranteed or endorsed by the publisher.
Research integrity at Frontiers
Learn more about the work of our research integrity team to safeguard the quality of each article we publish.