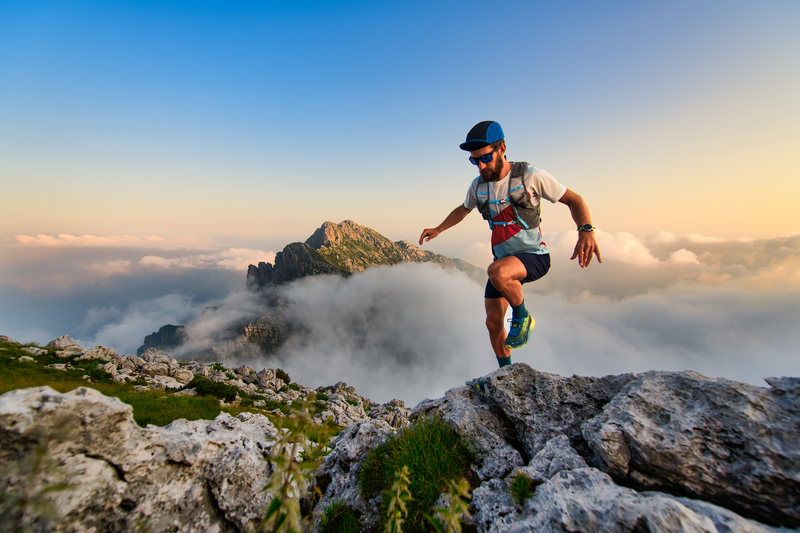
95% of researchers rate our articles as excellent or good
Learn more about the work of our research integrity team to safeguard the quality of each article we publish.
Find out more
ORIGINAL RESEARCH article
Front. Sustain. Food Syst. , 27 November 2024
Sec. Agricultural and Food Economics
Volume 8 - 2024 | https://doi.org/10.3389/fsufs.2024.1488747
Artificial meat is increasingly recognized as a crucial innovation for addressing global food security challenges and reducing environmental pressures. This study aims to understand the practicability of promoting artificial meat consumption to achieve the dual goals of improving food security and reducing resource-environment pressure by evaluating Chinese consumers’ willingness to pay (WTP) for artificial meat. A discrete choice experiment was conducted via an online survey involving 998 consumers from five representative Chinese cities. The experiment was designed to elicit preferences and WTP for artificial meat. A random information intervention providing positive information about artificial meat was included to examine its impact on consumer choices. The estimation results indicate significantly lower WTP for both cultured and plant-based meat compared to farm-raised meat, with plant-based meat receiving higher WTP than cultured meat. The information intervention notably reduced the WTP gap between artificial meat and farm-raised meat, suggesting that consumer education can positively influence acceptance. The findings suggest that the market introduction of artificial meat in China faces considerable challenges, particularly regarding consumer acceptance and perceived value. While positive information can reduce the disparity in WTP, the practicability of promoting artificial meat to achieve food security and environmental sustainability goals is currently limited. This study contributes to the literature on consumer attitudes towards artificial meat in China and provides critical insights for policymakers aiming to promote the consumption of artificial meat.
The rising demand for protein-rich foods like meat, eggs, and dairy products in developing countries poses significant challenges to global food security and environmental resource protection (Tilman and Clark, 2014; Fan and Brzeska, 2016; Sharma et al., 2018; Humpenöder et al., 2022). According to the statistics of the Food and Agriculture Organization of the United Nations (FAO), per capita meat consumption globally, on average, increased from 38.07 kg in 2000 to 43.64 kg in 2019. It is expected that, by 2050, the meat demand of about 10 billion people globally will require too much land, water and energy, and lead to unacceptable greenhouse gas and other pollutant emissions (Godfray, 2019). Currently, food production and consumption contribute to roughly one-third of the world’s household environmental impact (Simeone and Scarpato, 2020). This may significantly impede the achievement of the sustainable development goals (SDGs) (Halkos and Gkampoura, 2021; Brumm and Fukushi, 2023). Demand for natural resource exploitation will continue to increase, while the environmental constraints on resource usage will become increasingly evident.
Artificial meat has been proposed as a potential alternative to meet the increasing meat demand and ensure food security (Hamlin et al., 2022; Bhat et al., 2015). Artificial meat usually includes cultured meat, plant-based meat, and fermented meat. Cultured meat is produced through in vitro cultivation of stem cells, replicating the growth and repair mechanisms of animal muscle (Post, 2012). Plant-based meat is synthesized from vegetable proteins and other plant-derived components (Vanhonacker et al., 2013; Rubio et al., 2020). Fermented meat is produced by microorganisms that form proteins using engineered microbes to generate recombinant proteins and various compounds. The final product contains no host cells (Ong et al., 2020). Early proponents of artificial meat, particularly animal conservationists, argue that it protects animal welfare by eliminating the need for traditional farming and slaughtering practices (Hopkins and Dacey, 2008; Chriki et al., 2022). Additionally, proponents believe that expanding artificial meat production can increase meat supply, thereby enhancing food and nutrition security (Lee et al., 2020).
Numerous studies indicate that promoting artificial meat can alleviate water and land resource pressures and reduce greenhouse gas emissions (Simeone and Scarpato, 2020; Mattick et al., 2015; Tuomisto and Teixeira de Mattos, 2011; Tuomisto et al., 2014). Plant-based meat production consumes less water and land than traditional methods and provides sufficient plant protein to meet basic human nutritional needs (Sheng et al., 2020). Similarly, cultured meat meets human meat demand and ensures food security while mitigating environmental pollution, positioning it as an ideal alternative (Hamlin et al., 2022; Post, 2012). Compared with traditional farm-raised meat, the production of cultured meat does not need feed and water, does not generate waste and harmful gases. It only needs nutritional factors to maintain cell tissue growth (Bhat et al., 2015). Previous studies have demonstrated that cultured meat production consumes less energy, emits fewer greenhouse gas emissions, requires less land, and uses less water compared to traditional farm-raised meat (Tuomisto and Teixeira de Mattos, 2011; Tuomisto et al., 2014). These findings highlight the potential of cultured meat in significantly improving the eco-environment.
The extent to which artificial meat can improve food security and reduce resource-environment pressure largely depends on consumer acceptance. Many studies have explored consumer preferences and attitudes toward artificial meat (e.g., Hocquette et al., 2015; Wilks and Phillips, 2017; Mancini and Antonioli, 2019), primarily focusing on developed countries (e.g., Slade, 2018; Mancini and Antonioli, 2020; Asioli et al., 2022). However, consumer attitudes toward artificial meat in developed countries are inconsistent. For instance, many European consumers, such as Italians, highly accept cultured meat (Mancini and Antonioli, 2019), whereas few Americans believe cultured meat will replace traditional meat in their diets (Wilks and Phillips, 2017). In developing countries, consumers’ attitudes toward artificial meat are also mixed according to only a few studies (Zhang et al., 2020; Chen and Zhang, 2022; Chriki et al., 2021; Li et al., 2022; Kombolo Ngah et al., 2023). Bryant et al. (2019) showed that Indian and Chinese consumers have higher acceptance of artificial meat than those in the United States, while Liu et al. (2021) reported resistance among Chinese consumers. Chen and Zhang (2022) revealed that over half of Chinese social media comments on artificial meat were negative or neutral. Kombolo Ngah et al. (2023) reported that 32.1% of consumers from 12 African countries held strong negative attitudes toward artificial meat, believing it would detrimentally impact their lives. In Brazil, Chriki et al. (2021) discovered that over 46.6% of participants considered artificial meat acceptable. Given these ambiguous attitudes of consumers toward artificial meat, further ex-ante analyses and empirical studies on consumers’ attitudes toward artificial meat are needed.
This study aims to better understand the practicability of promoting artificial meat consumption to achieve the dual goals of improving food security and reducing resource-environment pressure by taking a case of Chinese consumers’ WTP for artificial meat. There are three reasons to focus on China. First, China is the largest developing country with a rapid increase in meat demand (Wang, 2022). A change in Chinese meat demand will remarkably affect the global meat market. Second, China is currently encountering significant environmental and resource pressures in order to meet the escalating demand for meat. Third, a deep understanding of the practicability of promoting artificial meat in China would provide a reference for other developing countries with rapid economic growth such as Vietnam or India.
To meet the goal, a choice experiment was conducted in an online survey of 1,000 consumers in five representative cities in China (Beijing, Shenyang, Wuhan, Chengdu, and Guangzhou) in October 2020 to examine consumers’ preferences and WTP for artificial meat including plant-based meat and cultured meat. A random information intervention was also incorporated into the experiment to detect the effects of providing positive information related to artificial meat on consumers’ choice.
The estimation results of a random parameter logit model show that consumers have significantly lower preferences and WTP for both cultured and plant-based meat compared to farm-raised meat. Furthermore, consumers exhibit relatively higher preferences and WTP for plant-based meat over cultured meat. Positive information interventions narrow the preference gap between artificial and farm-raised meat. Additionally, the production location influences consumer choice. The relatively negative attitudes of Chinese consumers challenge the practicability of promoting artificial meat to ensure food security and protect environment and resource sustainability.
The rest of this study proceeds as follows. Section 2 presents the methods, including a discrete choice modeling framework, a random parameters logit model, and choice experiment design. Section 3 introduces the data. Section 4 presents the estimation results and discusses the findings. Section 5 summarizes and concludes.
This study employs a discrete choice experiment (DCE) framework and a random parameters logit (RPL) model to state consumers’ preferences and WTP for artificial meat (Slade, 2018; Wang et al., 2022). DCE and contingent valuation method (CVM) have been widely used for non-market valuation (e.g., Halkos and Matsiori, 2016; Hu et al., 2012; Wang et al., 2018; Lin and Nayga, 2022). DCE offers a more realistic and flexible approach for estimating consumer preferences and WTP (Hensher et al., 2005) and serves a comprehensive understanding of consumer behavior by incorporating the trade-offs individuals make when selecting among various product attributes (Louviere et al., 2000). This framework provides valuable insights into consumer valuation, enabling a thorough assessment of consumer preferences (Hensher et al., 2007). To analyze DCE data, RPL model and conditional Logit model (CLM) are applied in previous studies (e.g., Bai et al., 2013; Lusk and Tonsor, 2016; Ortega et al., 2022). Compared to CLM, RPL model facilitates the capture of heterogeneity in consumer preferences by incorporating individual-specific random parameters (Colombo et al., 2009). These parameters serve to accommodate variations in individual characteristics within the population, allowing for a more comprehensive analysis of consumer behavior (Train, 1998). Hence, following previous studies (e.g., Van Loo et al., 2020; Ortega et al., 2022), we designed a choice experiment to collect stated preference data and incorporated an information intervention into the choice experiment.
Based on the Lancaster’s consumer theory (Lancaster, 1966) and random utility theory (McFadden, 1974), this study employs an attribute-based choice method. A beef burger is assumed to contain a bundle of attributes, while corresponding utilities can be derived from these attributes. Meanwhile, consumers’ utility typically comprises of a discernible deterministic component and an indiscernible random component. Thus, the utility of consumer n choosing alternative i in scenario t can be specified as follows:
where is the deterministic part of the utility and can be defined as:
where is a vector of observed attributes of the alternative in scenario t as faced by consumer n; is a vector of random coefficients representing consumer n’s taste; is the stochastic component.
Theoretically, a rational consumer chooses a meat product with a specific set of attributes that can yield the highest utility. Thus, according to McFadden (1974), the probability of choosing a particular meat product is greater if the utility it provides is the highest among the various options in a set of choices.
The RPL model is further employed to estimate the consumers’ preferences and WTP for a burger product with specific attributes, such as cultured meat burger and plant-based meat burger. The RPL model provides enhanced flexibility by relaxing the Independence of Irrelevant Alternatives (IIA) assumption, incorporating preference heterogeneity, and accounting for correlations between multiple choice observations made by individual respondents (Train, 2009; Campbell et al., 2018). This allows for a more accurate representation of consumer preferences and decision-making processes, particularly in complex choice scenarios. Thus, the probability of consumer n choosing alternative i in scenario t can be expressed as follows:
where j represents the total number of alternatives in the choice set. A vector of coefficients ( ) are further defined as random variables following density function , given the distributional parameter .These coefficients can be obtained by approximating the likelihood function using simulated maximum likelihood approach (Train, 2009). By using this approach, we can capture the inherent uncertainty and variability within the coefficients, considering them as random variables rather than fixed values. This allows for a more comprehensive understanding of the relationships between the variables in the model and provides a more accurate representation of the underlying data.
In order to examine consumers’ preferences for artificial meat, we took beef burger as a case in the choice experiment. As a set of attributes are employed, the deterministic component of utility in our econometric models can be further defined as follows:
where captures no choice option, which is an alternative specific constant. is a continuous variable characterized with three price levels in the design, representing the price of alternative i in scenario t for consumer n. and represent the dummy variables for the patties of burger made from cultured and plant-based meat, respectively (the farm-raised meat is the baseline). is a dummy variable equal to one if the burger patties originated from China and zero from foreign countries. The non-price attribute coefficients are assumed to be independent with normal distributions. Additionally, following Shi et al. (2023), we assumed that, first, the coefficient for no choice is fixed to represent the baseline utility level, providing a consistent reference point across respondents and ensuring accurate comparison of alternatives and capture of relative preferences within the RPL model (Hensher and Greene, 2003); and second, the price coefficient is fixed to facilitate straightforward derivation and interpretation of WTP distributions, and to prevent the irrational occurrence of positive price coefficients in any subsample (Revelt and Train, 1998; Layton and Brown, 2000).
As noted by Hensher and Greene (2003), interaction terms can be valuable in exploring preference heterogeneity in mean parameter estimates. Accordingly, we include interaction terms between the type of meat used in burger patties and various consumer familiarity levels regarding artificial meat, along with their socio-demographic characteristics. Consistent with previous studies (Liu et al., 2019; Shi et al., 2023), the coefficients for these interaction terms are assumed to be fixed. The utility function with interactions is specified as follows:
where represents a vector of consumers’ socio-demographic characteristics.
From the estimated coefficients in the RPL model, the WTP measures for attribute k is calculated by dividing the coefficient estimate for the attribute by the negative of the price coefficient:
where is the specific attribute coefficient, and is the price coefficient. Moreover, following Hole (2007), all WTP standard deviations and 95% confidence intervals are estimated using the delta method. The models’ estimates were derived from a simulated maximum likelihood using 1,000 Halton draws.
We take beef burger consumption in China as a case study. First, although traditionally not as popular, beef burger consumption in China has been rising, particularly in urban areas and among younger consumers influenced by Western dietary habits (Nam et al., 2010; Wang, 2022). This emerging trend offers a unique opportunity to study the potential market for artificial meat products. Second, beef production is recognized for its significant environmental impact, which includes greater greenhouse gas emissions, water consumption, and land use compared to other meats (Gerber et al., 2015; Wang et al., 2024). China’s beef production has shown a consistent growth trend since 2013 and is now among the world’s leading beef-producing countries (NBSC, 2022). Therefore, focusing on beef burgers in China, our study emphasizes the significant environmental benefits that artificial meat could provide, which is a crucial aspect of our research. Third, beef consumption and imports in China are increasing, and this trend is expected to persist in the foreseeable future (Ortega et al., 2016). The choice of beef burgers enables a comparative analysis with other regions where they are more popular, thus offering a broader understanding of consumer behavior and market dynamics.
In the choice experiment design for a beef burger, the product attributes varied based on three specific attributes: (a) the type of meat as burger patties, (b) the production location of patties, and (c) the price of burger. Table 1 presents the product attributes of beef burgers and their respective levels. Figure A1 presents a sample of the choice experiment card along with its description. As observed in Table 1, the meat utilized for burger patties includes three levels: cultured meat, plant-based meat, and farm-raised meat. Cultured meat and plant-based meat are the main types of artificial meat (Zhang et al., 2021). Cultured meat is made by cultivating animal cells in the laboratory, and plant-based meat is made by processing plant fiber. They have a similar appearance and taste to farm-raised meat. Country of origin have traditionally been used as a proxy to identify local foods, as the location of food production is a significant factor influencing consumers’ food choices (Ortega et al., 2014). Thus, we incorporated a “location of production origin” attribute, specifying the source of the burger patties. The origin is categorized into two levels: China or foreign. The prices employed in the choice experiment fell within the range reflective of observed market prices at fast food establishments including Burger King, McDonald’s, and KFC in China as of September 2020. The mean observed price was 30 Yuan ($4.39). The average observed price was 30 Yuan ($4.39), with the price range for beef burgers at these three fast food chains typically falling between 27 and 33 Yuan. Incorporating plus and minus one standard deviation from the mean observed price in a choice experiment is a methodological strategy that enhances the fidelity of the study’s design. This approach allows for a more nuanced examination of how consumers navigate their decision-making process when confronted with price fluctuations. By encompassing a range around the mean, it acknowledges the inherent variability in consumer preferences and sensitivity to price changes, offering a more comprehensive understanding of the complex interplay between pricing dynamics and consumer behavior, and illuminating the multifaceted nature of market responses. Therefore, we ultimately settled on three prices for the beef burger: 27 Yuan ($3.95), 30 Yuan ($4.39), and 33 Yuan ($4.83).
Based on the selected attributes and their levels, a full factorial design generates 18 possible beef burger profiles (three burger patties two locations of production three price levels). Randomly combining two different beef burger profiles creates a total of 153 choice sets, which is not realistic in a real-choice situation. Sufficient evidence indicates that participants who engage in selection tasks for more than 20 min will become fatigued, which will impact their judgments (Allenby and Rossi, 1998; Shi et al., 2023). It is not recommended to compel a respondent to assess the complete choice sets. As a result, we applied a D-efficient design using NGENE software to generate 18 choice sets, thereby maximizing the D-efficiency. To reduce the cognitive load of the participants, the 18 choice sets were randomly divided into six blocks, with three choice sets in each block. Each respondent was randomly assigned to assess one block of choice sets.
A pre-test was conducted with participants from Wuhan in June 2020. In our preliminary examination, we conducted on-site interviews with a cohort of 20 consumers, diligently recording the challenges they faced throughout the process of the choice experiment, aiming to assess the efficacy of its design. In each of the three choice sets, participants were asked to choose between two beef burger options and a third option of not purchasing either of the first two beef burgers (see Figure A1). Adding the option of “no choice” makes the subjects closer to the actual choice scenario in the choice process, avoiding being forced to choose the product they do not want, thus improving the overall quality of the data (Louviere et al., 2000). We randomly distributed the sequence of choice options, and the choice sets in each block to avoid order effects and respondent learning effects (Carson et al., 1994; Hamlin, 2016). A cheap talk technique was employed to mitigate the hypothetical bias (Murphy et al., 2005), demonstrating that the WTP measures derived from the choice experiment closely aligned with the actual choices made (Lusk, 2003). The respondents were also informed that, aside from the attributes presented in the choice set, products within each choice set do not differ in any other attributes.
In order to explore whether providing information related to cultured and plant-based meat can affect consumers’ choice and WTP, we randomly selected approximately half of the participants for an information intervention. This random assignment ensures that any observed differences in consumer behavior can be attributed to the informational content rather than pre-existing variations among participants. Before the choice experiment, the intervened participants received comprehensive introductory information about cultured meat and plant-based meat, while the remaining participants (the control group) were not provided with any specific information on these alternatives. The information mainly introduces the production process of cultured and plant-based meat and its internal and external benefits. Internal benefits mainly include benefits for health, while external benefits mainly include benefits for the environment and natural resources. The specific content of the information used in the intervention is in Figure A2, which outlines the key points and graphical representations provided to participants.
The data used in this study were collected through the online survey of Chinese urban consumers’ attitudes toward artificial meat conducted in October 2020. In order to ensure (urban) national representativeness economically and geographically, the survey was conducted in five major cities in China, including Beijing, Shenyang, Wuhan, Guangzhou, and Chengdu. Beijing, the capital of China, is a political and cultural hub located in the north. Shenyang, situated in the northeast, is a significant industrial center. Wuhan, centrally positioned, plays a pivotal role in the Yangtze River Economic Belt. In the south, Guangzhou stands out as a major business and trade center, boasting the fourth-highest GDP in the country. Chengdu, the core city of southwestern China, ranks eighth in GDP. Collectively, these five cities serve as major regional hubs and represent a substantial portion of the Chinese urban population. Their advanced economies make them ideal locations for studying consumer preferences and WTP for artificial meat. Given that artificial meat is an emerging concept and most rural consumers may not yet be familiar with it, this survey focused exclusively on urban adult meat eaters, as referenced in the study by Zhang et al. (2020).
To ensure data quality, we employed the Credamo platform,1 one of the top survey companies in China. Renowned for data collection, Credamo was widely used in previous studies (e.g., Zhang et al., 2022a; Gai and Puntoni, 2021; Huang and Sengupta, 2020). The Credamo sample library boasts an extensive database of more than 3 million registered users spanning all provincial-level administrative regions in China. Each participant must complete real-name and mobile number authentication to be included in the Credamo sample library, ensuring the authenticity of data collection. Currently, Credamo serves more than 3,000 universities globally, including prestigious institutions like the Massachusetts Institute of Technology, New York University, and Peking University. It is well regarded and has an outstanding reputation. To encourage participation, respondents were offered a reward of 8 Yuan ($1.17) upon completing the questionnaire. Moreover, to prevent multiple submissions, each ID was allowed to complete the questionnaire only once. Additionally, the Credamo platform incorporates intelligent human-machine verification, identity verification, and IP address restrictions to prevent participants from completing the questionnaire multiple times. Following Hauser and Schwarz (2016), we incorporated attention checks at the one-third and two-thirds of the way through the questionnaire to ensure data quality. If participants answered an attention check question incorrectly, the questionnaire was immediately terminated, and their responses were invalidated. Additionally, we analyzed completion times and discarded responses that were significantly faster than the average completion time, as these often indicate a lack of engagement or random answering (Meade and Craig, 2012). We collected the responses from 200 consumers in each city, resulting in a total of 1,000 questionnaires. However, due to missing values for key socio-demographic variables such as age and family income in two questionnaires, we used data from 998 valid responses.
Four modules of data collected from the survey questionnaire were utilized in this study: (1) individual and family socio-economic characteristics of interviewed consumers; (2) consumers’ familiarity of artificial meat, measured by the question, “Have you ever heard of artificial meat?.” The answer “Never heard” represents the low familiarity level of artificial meat, “I have heard, but I do not understand” represents the middle familiarity level of artificial meat, and “I have heard of it and know it well” represents the high familiarity level of artificial meat; (3) an information intervention was implemented by providing relatively positive information on cultured meat and plant-based meat for a group randomly selected by the survey platform; (4) consumers’ choice of a beef burger product with specific attributes based on the choice experiment.
Table 2 reports the individual and family socio-economic characteristics of 998 interviewed consumers. It is noticed that most sample consumers in this study are relatively young and have relatively high education levels. This is mainly because the survey was conducted through an online platform, which is typically favored by young and well-educated individuals. Similar to previous studies, the representativeness of the sample may be debatable (Zhang et al., 2020; Liu et al., 2021). Nevertheless, the survey data remains valuable in several aspects. First, these young and well-educated consumers are likely to constitute the majority of consumers in the near future and tend to be more concerned about sustainable development. Therefore, they may represent the initial group of potential consumers for artificial meat. Second, the unique and well-designed survey data collected from these five cities in China enable us to empirically compare consumers’ attitudes toward artificial meat, thereby contributing to the existing literature. Lastly, the dataset obtained from a survey of 1,000 respondents plays a pivotal role in shaping policies to advance the promotion of sustainable food practices. The demographic profile of the participants indicated a balanced gender distribution, with 53.4% male and 46.6% female respondents. Half of the participants fell into the middle-income bracket, with family incomes ranging from 4,001 Yuan to 8,000 Yuan ($585.53–$1170.77). Regarding family size, 63.8% of the surveyed participants had a household of 3–4 individuals, while 33.1% of the respondents had a household of 5 individuals or more. Furthermore, 80.8% of the respondents exhibited a moderate level of cognitive understanding concerning artificial meat, whereas only 14.7% of the participants demonstrated a high level of familiarity regarding artificial meat. In the context of the information intervention, 47.1% of the participants were assigned to the information group, while the remaining participants were allocated to the no-information group, resulting in nearly equal numbers in both groups. Additionally, Table A1 presents a comprehensive summary of the socio-demographic variables for both the information intervention and control groups. The t-test results indicated no significant differences in most variables between the two groups, thereby confirming the effectiveness of the random information intervention in ensuring comparable baseline characteristics across groups.
The results reported in Table 3 indicate that a significant Wald Chi-square statistic from the RPL model confirms the overall validity of the model. First, the mean estimated part-worth utility is negative and statistically significant for both cultured and plant-based meat. This finding indicates that beef burger products with cultured meat patties or plant-based meat patties are less likely to be selected compared to those with farm-raised meat patties. Moreover, the marginal effects of cultured and plant-based meat are −0.201 and −0.173, respectively. This indicates that compared with the reference group of “farm-raised meat,” Chinese consumers have a 20.1 and 17.3% lower likelihood of choosing cultured and plant-based meat. At the same time, consumers prefer plant-based meat over cultured meat. Second, the marginal effects of cultured and plant-based meat on the probability of choosing in the information intervention group are relatively higher than those in the group without information intervention. This result suggests that information interventions providing positive information on cultured and plant-based meat enhance consumers’ preferences toward these products, which is consistent with the findings of Chuah et al. (2024). Moreover, the production location and price of the given burger product also affect consumers’ choices. The estimation results show a 5% significantly higher probability of buying meat products made in China than those made in foreign countries. The coefficient estimates for price are all negative and statistically significant, as expected. The negative coefficients for no choice estimates suggest that, on average, consumers prefer purchasing the offered beef burger over the no choice option.
Based on the estimation results in Table 3, we further calculated consumers’ WTP premiums for artificial meat, including both cultured and plant-based meat (Table 4). Compared with farm-raised meat, consumers’ WTP premiums for cultured meat and plant-based meat are lower by 21.5 Yuan ($3.15 per burger) and 17.85 Yuan per burger ($2.61 per burger), respectively. Hence, most Chinese consumers are not willing to pay as much for artificial meat. Therefore, their WTP premiums for artificial meat, on average, are negative too. Nevertheless, consumers’ WTP premium for plant-based meat is relatively higher than that for cultured meat.
Figure 1 shows that consumers’ WTP premiums for artificial meat are obviously different between the groups with and without information intervention. For consumers who did not receive information intervention, the average WTP premiums for cultured meat and plant-based meat are the lowest, which carry a negative premium of 35.94 Yuan per burger ($5.26 per burger) and 28.44 Yuan per burger ($4.16 per burger), respectively. Consumers who received information intervention also exhibit negative WTP premiums for cultured meat (13.69 Yuan, $2.00 per burger) and plant-based meat (12.31 Yuan, $1.80 per burger). While the average WTP premiums of consumers who received information intervention for artificial meat remain negative, the information intervention appears to increase Chinese consumers’ WTP for artificial meat.
Figure 1. Consumers’ WTP premiums for cultured meat and plant-based meat as compared to farm-raised meat.
First, we examined the heterogeneity in consumers’ purchasing decisions for artificial meat based on various socio-demographic characteristics. To detect consumer preferences with different socio-demographic variables toward artificial meat, we re-estimated the RPL model, including the interaction terms between some socio-demographic variables and artificial meat. The demographic variables include age, gender, education, family size, and family income. Age and family size are treated as continuous variables, while education (1 = bachelor’s degree or higher), gender (1 = female), and family income (categorized into three groups, with the low-income group as the baseline) are treated as dummy variables. Table 5 presents the estimation results of the RPL model with interaction terms. The findings indicate that only a portion of the interaction terms are statistically significant. Notably, older consumers exhibit a higher probability of choosing plant-based meat, particularly when they are provided with positive information about artificial meat. Additionally, individuals holding a bachelor’s degree or higher are less likely to select artificial meat options. Interestingly, compared to low-income consumers, those in the middle and high-income groups demonstrate a more favorable preference for artificial meat. Despite these significant interactions, the coefficients for both cultured meat and plant-based meat remain significantly negative even after controlling for socio-demographic variables. This persistence suggests that the overall negative attitude of Chinese consumers toward artificial meat is not solely driven by their socio-economic characteristics.
Second, we explored the heterogeneity in consumers’ preferences based on their levels of familiarity with artificial meat. Table 6 presents the estimation results of the choice model, which includes interaction terms between artificial meat and consumers’ familiarity levels. The results suggest that consumers with a high familiarity with artificial meat demonstrate the strongest preferences for cultured and plant-based meat across all groups.
Based on the estimation results in Table 6, we subsequently calculated consumers’ WTP premiums for artificial meat at different levels of familiarity. Figures 2, 3 illustrate that consumers with higher familiarity levels of artificial meat exhibit greater WTP premiums for both cultured meat and plant-based meat compared to those with lower familiarity levels, irrespective of information intervention. These findings indicate that consumers’ WTP for artificial meat is significantly influenced by their understanding of it. The key factor behind these findings is that consumers who have a better understanding of artificial meat can fully comprehend its benefits, including its potential to promote environmental conservation, sustainable development, and food security. According to Milman and Pizam (1995), the level of consumers’ familiarity plays a crucial role in shaping their valuation of products. A deeper understanding of artificial meat’s attributes and benefits enhances perceived value and trust, thereby increasing their WTP. Consequently, their WTP for artificial meat tends to be higher (Figure 3).
A robustness check was further conducted to test that the main findings are not affected by the estimation models. In previous studies, the generalized multinomial logit model (G-MNL II) and the RPL model in WTP space have been utilized to analyze discrete choice experiment data (Liu et al., 2019; Harold et al., 2021). The G-MNL II model extends the flexibility of the conventional RPL model by allowing for scale heterogeneity and preference heterogeneity (Fiebig et al., 2010). This model can therefore accommodate variations in the relative importance of attributes across individuals, providing a more nuanced understanding of choice behavior (Hess and Train, 2017). Alternatively, the RPL model in WTP space directly estimates the WTP distributions, offering a more straightforward interpretation of the value consumers place on different attributes (Train and Weeks, 2005). This model simplifies the interpretation of coefficients and avoids potential biases associated with the conventional RPL model’s indirect estimation of WTP (Scarpa et al., 2008). Subsequently, the G-MNL II model and the RPL model in WTP space were used to re-examine consumer preferences and WTP for artificial meat.
Table A2 reports the estimation results of the G-MNL II model, while Table A3 presents the corresponding calculated WTP premiums. Table A2 indicates that consumers continue to exhibit significantly negative preferences for artificial meat compared to farm-raised meat. The marginal effects of cultured meat and plant-based meat in the G-MNL II model are relatively similar to those in the RPL model estimates presented in Table 3. Despite these preferences, Table A3 shows that consumers’ WTP premiums for cultured and plant-based meat, as estimated by the G-MNL II model, are consistent with the RPL model results in Table 4. Additionally, Table A4 presents the estimation results from the RPL model in WTP space, which also align with the WTP estimates results in Table 4. Overall, the results obtained from the G-MNL II model and RPL model in WTP space are consistent with those based on the RPL model. As a result, the consistency across different modeling approaches underscores the reliability of our conclusions regarding consumers’ preferences and WTP premiums for cultured and plant-based meat.
The active promotion of artificial meat may play a pivotal role in achieving the SDGs, which aim to address global challenges across social, economic, and environmental spheres, thereby advancing sustainable development (Halkos and Gkampoura, 2021). In this regard, understanding consumers’ attitudes toward artificial meat are essential (Sodano et al., 2016; Siegrist and Sütterlin, 2017). Our results show that consumers’ attitudes toward artificial meat are negative in China, the world’s largest meat consumption market. By eliciting consumers’ WTP premiums for artificial meat through a choice experiment, we found that, on average, Chinese consumers are unwilling to pay a premium for artificial meat compared to farm-raised meat. However, previous studies that directly queried consumers’ acceptance or WTP for artificial meat contribute to understanding consumers’ attitudes toward artificial meat (Zhang et al., 2020; Liu et al., 2021). In contrast, our study measured consumers’ WTP premiums for artificial meat compared to farm-raised meat, providing a clearer understanding of the variation in consumers’ attitudes toward artificial meat. Additionally, we found a relatively higher preference among Chinese consumers for plant-based meat compared to cultured meat; this finding is reasonable because some plant-based meats are soybean products that have been consumed by Chinese consumers for thousands of years.
The findings of this study reveal that the marketing of artificial meat in China remains a significant challenge. This situation suggests that the practicability of promoting artificial meat consumption to achieve the dual goals of improving food security and reducing resource-environment pressures is currently low. To enhance the future consumption of artificial meat, two key actions must be undertaken. First, enhancing consumers’ knowledge and understanding of artificial meat is essential, which can be achieved by providing them with comprehensive information about its positive aspects. Providing information to consumers has been found to be important in this regard (Verneau et al., 2014). This includes educating them about its benefits, such as sustainability, reduced environmental impact, and potential health advantages. Second, improving the price competitiveness of artificial meat is crucial, which can be achieved by reducing product costs through advancements in production processes, scaling up manufacturing capabilities, and optimizing the supply chain. By simultaneously increasing consumer awareness and improving price competitiveness, artificial meat can be positioned for higher consumption rates in the future.
In the context of resource-environmental constraints, trade uncertainty, public health event shocks, geopolitical conflict and interstate war increase, food security has been globally threatened (Nguyen et al., 2023). In response to the increasing demand for protein-rich foods, developing novel food technologies, such as artificial meat, becomes critically important. This advancement is especially vital for ensuring future food security, safety, and sustainability. The integration of these innovative solutions is imperative in addressing the nutritional needs of a burgeoning global population while simultaneously mitigating environmental impacts (Siegrist and Hartmann, 2020). This study investigates Chinese consumers’ attitudes toward artificial meat by using a choice experiment. The RPL model estimates consumers preferences for meat products with different attributes and elicits consumers’ WTP premiums for artificial meat. The estimation results suggest that Chinese consumers have a significantly lower probability of buying cultured and plant-based meat than farm-raised meat. Additionally, the results of consumers’ WTP premiums further reveal a negative attitude of Chinese consumers toward artificial meat; they are willing to pay less for plant-based meat and the least for cultured meat. These findings suggest that the current proposal to promote artificial meat to improve food security and reduce resource-environment pressure is premature and impracticable. Our study highlights that, at present, Chinese consumers are not ready to embrace artificial meat, which aligns with the broader literature indicating consumer skepticism and price sensitivity regarding novel food technologies (Siegrist and Hartmann, 2020). Given these insights, it is essential to focus on reducing the production costs of artificial meat by advancing related research and enhancing consumers’ familiarity and acceptance of artificial meat.
The findings of this study have important implications. First, this study evaluates the practicability of promoting artificial meat consumption to achieve the dual goals of improving food security and reducing resource-environment pressure in China. While many previous studies focused on the positive aspects of promoting artificial meat consumption (Post, 2012; Van Loo et al., 2020) and theoretically discussed its practicability as an alternative to conventional meat (Bonny et al., 2015; Verbeke et al., 2015; Pakseresht et al., 2022), there is limited empirical evidence on Chinese consumers’ preferences and WTP for artificial meat. Second, the findings supplement the literature about Chinese consumers’ attitudes toward artificial meat (Zhang et al., 2020; Wang et al., 2022; Zhang et al., 2022b). While previous studies show a portion of Chinese consumers accept artificial meat (Zhang et al., 2020; Liu et al., 2021), our study further reveals that Chinese consumers have a lower probability of buying artificial meat than farm-raised meat and are willing to pay less for artificial meat compared to farm-raised meat. This suggests that consumer acceptance is still a significant barrier. Third, this study has important policy implications for developing diverse food sources in China under the concept of Big Food. Our findings suggest that information interventions and increasing consumers’ familiarity about artificial meat can enhance their awareness and acceptance. Policymakers should consider implementing information dissemination campaigns through television and the internet to educate the public about the environmental and health benefits of artificial meat. Meanwhile, the government should encourage the adoption of sustainable practices within the artificial meat industry by offering subsidies or tax incentives for companies that invest in environmentally friendly technologies and processes. Artificial meat producers ought to participate in consumer education initiatives to foster trust and familiarity with artificial meat. This can be accomplished through clear communication regarding the safety, nutritional benefits, and environmental advantages of artificial meat, while also addressing common concerns and misconceptions. Additionally, providing financial incentives and support for research and development can help reduce the production costs of artificial meat, making it more competitive in the market. Lower production costs can lead to lower retail prices, increasing accessibility and attractiveness to consumers.
Even though our study provides several important insights, it is subject to some limitations. First, the range of meat types included in the experiment may have influenced consumers’ choices regarding artificial meat. To capture a more comprehensive spectrum of consumer preferences, future studies should incorporate a broader variety of meat forms. Second, the representativeness of our consumer sample may be questioned due to demographic imbalances. To enhance the generalizability of the findings, subsequent research should employ surveys with more diverse and representative samples that accurately reflect the broader population demographics. Third, data collection was conducted via an online survey, which may introduce selection bias. Consistent with many online consumer surveys in China (Hu et al., 2022; Shi et al., 2023), our sample does not fully represent the entire Chinese population. Although this limitation restricts the extent to which our findings can be generalized, the study still provides significant insights into consumer preferences and WTP for artificial meat. To mitigate selection bias, future studies should combine online surveys with field surveys, thereby ensuring a more balanced and representative sample.
The raw data supporting the conclusions of this article will be made available by the authors, without undue reservation.
MY: Data curation, Formal analysis, Investigation, Methodology, Visualization, Writing – original draft. SM: Data curation, Formal analysis, Investigation, Methodology, Writing – original draft, Conceptualization, Funding acquisition, Validation, Writing – review & editing. TN: Conceptualization, Funding acquisition, Writing – review & editing. PQ: Writing – review & editing.
The author(s) declare that financial support was received for the research, authorship, and/or publication of this article. This research is funded by the special research project titled “Research on Demand and Prediction for Medical Services among Farming Households in Hubei” under the Hubei Outstanding Young Social Sciences Talent Program. The publication of this article was funded by the Open Access Publishing Fund of Leibniz University Hannover.
The authors declare that the research was conducted in the absence of any commercial or financial relationships that could be construed as a potential conflict of interest.
The author(s) declared that they were an editorial board member of Frontiers, at the time of submission. This had no impact on the peer review process and the final decision.
All claims expressed in this article are solely those of the authors and do not necessarily represent those of their affiliated organizations, or those of the publisher, the editors and the reviewers. Any product that may be evaluated in this article, or claim that may be made by its manufacturer, is not guaranteed or endorsed by the publisher.
The Supplementary material for this article can be found online at: https://www.frontiersin.org/articles/10.3389/fsufs.2024.1488747/full#supplementary-material
Allenby, G. M., and Rossi, P. E. (1998). Marketing models of consumer heterogeneity. J. Econ. 89, 57–78. doi: 10.1016/S0304-4076(98)00055-4
Asioli, D., Bazzani, C., and Nayga, R. M. Jr. (2022). Are consumers willing to pay for in-vitro meat? An investigation of naming effects. J. Agric. Econ. 73, 356–375. doi: 10.1111/1477-9552.12467
Bai, J., Zhang, C., and Jiang, J. (2013). The role of certificate issuer on consumers’ willingness-to-pay for milk traceability in China. Agric. Econ. 44, 537–544. doi: 10.1111/agec.12037
Bhat, Z. F., Kumar, S., and Fayaz, H. (2015). In vitro meat production: challenges and benefits over conventional meat production. J. Integr. Agric. 14, 241–248. doi: 10.1016/S2095-3119(14)60887-X
Bonny, S. P., Gardner, G. E., Pethick, D. W., and Hocquette, J. F. (2015). What is artificial meat and what does it mean for the future of the meat industry? J. Integr. Agric. 14, 255–263. doi: 10.1016/S2095-3119(14)60888-1
Brumm, A., and Fukushi, K. (2023). Introducing the food value framework (FVF) to empower transdisciplinary research and unite stakeholders in their efforts of building a sustainable global food system. Environ. Dev. Sustain. 26, 25921–25943. doi: 10.1007/s10668-023-03713-z
Bryant, C., Szejda, K., Parekh, N., Deshpande, V., and Tse, B. (2019). A survey of consumer perceptions of plant-based and clean meat in the USA, India, and China. Front. Sust. Food Syst. 3:11. doi: 10.3389/fsufs.2019.00011
Campbell, D., Mørkbak, M. R., and Olsen, S. B. (2018). The link between response time and preference, variance and processing heterogeneity in stated choice experiments. J. Environ. Econ. Manag. 88, 18–34. doi: 10.1016/j.jeem.2017.10.003
Carson, R. T., Louviere, J. J., Anderson, D. A., Arabie, P., Bunch, D. S., Hensher, D. A., et al. (1994). Experimental analysis of choice. Mark. Lett. 5, 351–367. doi: 10.1007/BF00999210
Chen, Y., and Zhang, Z. (2022). Exploring public perceptions on alternative meat in China from social media data using transfer learning method. Food Qual. Prefer. 98:104530. doi: 10.1016/j.foodqual.2022.104530
Chriki, S., Ellies-Oury, M. P., and Hocquette, J. F. (2022). Is “cultured meat” a viable alternative to slaughtering animals and a good comprise between animal welfare and human expectations? Anim. Front. 12, 35–42. doi: 10.1093/af/vfac002
Chriki, S., Payet, V., Pflanzer, S. B., Ellies-Oury, M. P., Liu, J., Hocquette, É., et al. (2021). Brazilian consumers’ attitudes towards so-called “cell-based meat. Food Secur. 10:2588. doi: 10.3390/foods10112588
Chuah, S. X. Y., Gao, Z., Arnold, N. L., and Farzad, R. (2024). Cell-based seafood marketability: what influences United States consumers’ preferences and willingness-to-pay? Food Qual. Prefer. 113:105064. doi: 10.1016/j.foodqual.2023.105064
Colombo, S., Hanley, N., and Louviere, J. (2009). Modeling preference heterogeneity in stated choice data: an analysis for public goods generated by agriculture. Agric. Econ. 40, 307–322. doi: 10.1111/j.1574-0862.2009.00377.x
Fan, S., and Brzeska, J. (2016). Sustainable food security and nutrition: demystifying conventional beliefs. Glob. Food Sec. 11, 11–16. doi: 10.1016/j.gfs.2016.03.005
Fiebig, D. G., Keane, M. P., Louviere, J., and Wasi, N. (2010). The generalized multinomial logit model: accounting for scale and coefficient heterogeneity. Mark. Sci. 29, 393–421. doi: 10.1287/mksc.1090.0508
Gai, P. J., and Puntoni, S. (2021). Language and consumer dishonesty: a self-diagnosticity theory. J. Consum. Res. 48, 333–351. doi: 10.1093/jcr/ucab001
Gerber, P. J., Mottet, A., Opio, C. I., Falcucci, A., and Teillard, F. (2015). Environmental impacts of beef production: review of challenges and perspectives for durability. Meat Sci. 109, 2–12. doi: 10.1016/j.meatsci.2015.05.013
Halkos, G., and Gkampoura, E. C. (2021). Where do we stand on the 17 sustainable development goals? An overview on progress. Econ. Anal. Policy 70, 94–122. doi: 10.1016/j.eap.2021.02.001
Halkos, G., and Matsiori, S. (2016). Determining public attitudes and willingness-to-pay for artificial lakes protection. Water Res. Econ. 15, 15–27. doi: 10.1016/j.wre.2016.05.001
Hamlin, R. (2016). The consumer testing of food package graphic design. Br. Food J. 118, 379–395. doi: 10.1108/BFJ-03-2015-0105
Hamlin, R., McNeill, L., and Sim, J. (2022). Food neophobia, food choice and the details of cultured meat acceptance. Meat Sci. 194:108964. doi: 10.1016/j.meatsci.2022.108964
Harold, J., Bertsch, V., and Fell, H. (2021). Preferences for curtailable electricity contracts: can curtailment benefit consumers and the electricity system? Energy Econ. 102:105454. doi: 10.1016/j.eneco.2021.105454
Hauser, D. J., and Schwarz, N. (2016). Attentive Turkers: MTurk participants perform better on online attention checks than do subject pool participants. Behav. Res. Methods 48, 400–407. doi: 10.3758/s13428-015-0578-z
Hensher, D. A., and Greene, W. H. (2003). The mixed logit model: the state of practice. Transportation 30, 133–176. doi: 10.1023/A:1022558715350
Hensher, D. A., Rose, J., and Bertoia, T. (2007). The implications on willingness to pay of a stochastic treatment of attribute processing in stated choice studies. Transp. Res. Part E Logist. Transp. Rev. 43, 73–89. doi: 10.1016/j.tre.2005.07.006
Hensher, D. A., Rose, J. M., and Greene, W. H. (2005). Applied choice analysis: A primer. Cambridge: Cambridge University Press.
Hess, S., and Train, K. (2017). Correlation and scale in mixed logit models. J. Choice Modell. 23, 1–8. doi: 10.1016/j.jocm.2017.03.001
Hocquette, A., Lambert, C., Sinquin, C., Peterolff, L., Wagner, Z., Bonny, S. P., et al. (2015). Educated consumers don't believe artificial meat is the solution to the problems with the meat industry. J. Integr. Agric. 14, 273–284. doi: 10.1016/S2095-3119(14)60886-8
Hole, A. R. (2007). A comparison of approaches to estimating confidence intervals for willingness to pay measures. Health Econ. 16, 827–840. doi: 10.1002/hec.1197
Hopkins, P. D., and Dacey, A. (2008). Vegetarian meat: could technology save animals and satisfy meat eaters? J. Agric. Environ. Ethics 21, 579–596. doi: 10.1007/s10806-008-9110-0
Hu, W., Batte, M. T., Woods, T., and Ernst, S. (2012). Consumer preferences for local production and other value-added label claims for a processed food product. Eur. Rev. Agric. Econ. 39, 489–510. doi: 10.1093/erae/jbr039
Hu, W., Sun, S., Penn, J., and Qing, P. (2022). Dummy and effects coding variables in discrete choice analysis. Am. J. Agric. Econ. 104, 1770–1788. doi: 10.1111/ajae.12311
Huang, Y., and Sengupta, J. (2020). The influence of disease cues on preference for typical versus atypical products. J. Consum. Res. 47, 393–411. doi: 10.1093/jcr/ucaa029
Humpenöder, F., Bodirsky, B. L., Weindl, I., Lotze-Campen, H., Linder, T., and Popp, A. (2022). Projected environmental benefits of replacing beef with microbial protein. Nature 605, 90–96. doi: 10.1038/s41586-022-04629-w
Kombolo Ngah, M., Chriki, S., Ellies-Oury, M. P., Liu, J., and Hocquette, J. F. (2023). Consumer perception of “artificial meat” in the educated young and urban population of Africa. Front. Nutr. 10:1127655. doi: 10.3389/fnut.2023.1127655
Lancaster, K. J. (1966). A new approach to consumer theory. J. Polit. Econ. 74, 132–157. doi: 10.1086/259131
Layton, D. F., and Brown, G. (2000). Heterogeneous preferences regarding global climate change. Rev. Econ. Stat. 82, 616–624. doi: 10.1162/003465300559091
Lee, H. J., Yong, H. I., Kim, M., Choi, Y. S., and Jo, C. (2020). Status of meat alternatives and their potential role in the future meat market: a review. Asian Australas. J. Anim. Sci. 33, 1533–1543. doi: 10.5713/ajas.20.0419
Li, H., Van Loo, E. J., van Trijp, H., Chen, J., and Bai, J. (2022). Will cultured meat be served on Chinese tables? A study of consumer attitudes and intentions about cultured meat in China. Meat Sci. 197:109081. doi: 10.1016/j.meatsci.2022.109081
Lin, W., and Nayga, R. M. (2022). Green identity labeling, environmental information, and pro-environmental food choices. Food Policy 106:102187. doi: 10.1016/j.foodpol.2021.102187
Liu, R., Gao, Z., Nayga, R. M. Jr., Snell, H. A., and Ma, H. (2019). Consumers’ valuation for food traceability in China: does trust matter? Food Policy 88:101768. doi: 10.1016/j.foodpol.2019.101768
Liu, J., Hocquette, É., Ellies-Oury, M. P., Chriki, S., and Hocquette, J. F. (2021). Chinese consumers’ attitudes and potential acceptance toward artificial meat. Food Secur. 10:353. doi: 10.3390/foods10020353
Louviere, J. J., Hensher, D. A., and Swait, J. D. (2000). Stated choice methods: Analysis and applications. Cambridge: Cambridge University Press.
Lusk, J. L. (2003). Effects of cheap talk on consumer willingness-to-pay for golden rice. Am. J. Agric. Econ. 85, 840–856. doi: 10.1111/1467-8276.00492
Lusk, J. L., and Tonsor, G. T. (2016). How meat demand elasticities vary with price, income, and product category. Appl. Econ. Perspect. Policy 38, 673–711. doi: 10.1093/aepp/ppv050
Mancini, M. C., and Antonioli, F. (2019). Exploring consumers’ attitude towards cultured meat in Italy. Meat Sci. 150, 101–110. doi: 10.1016/j.meatsci.2018.12.014
Mancini, M. C., and Antonioli, F. (2020). To what extent are consumers’ perception and acceptance of alternative meat production systems affected by information? The case of cultured meat. Animals 10:656. doi: 10.3390/ani10040656
Mattick, C. S., Landis, A. E., and Allenby, B. R. (2015). A case for systemic environmental analysis of cultured meat. J. Integr. Agric. 14, 249–254. doi: 10.1016/S2095-3119(14)60885-6
McFadden, D. (1974). The measurement of urban travel demand. J. Public Econ. 3, 303–328. doi: 10.1016/0047-2727(74)90003-6
Meade, A. W., and Craig, S. B. (2012). Identifying careless responses in survey data. Psychol. Methods 17, 437–455. doi: 10.1037/a0028085
Milman, A., and Pizam, A. (1995). The role of awareness and familiarity with a destination: the Central Florida case. J. Travel Res. 33, 21–27. doi: 10.1177/004728759503300304
Murphy, J. J., Stevens, T., and Weatherhead, D. (2005). Is cheap talk effective at eliminating hypothetical bias in a provision point mechanism? Environ. Resour. Econ. 30, 327–343. doi: 10.1007/s10640-004-4224-y
Nam, K. C., Jo, C., and Lee, M. (2010). Meat products and consumption culture in the east. Meat Sci. 86, 95–102. doi: 10.1016/j.meatsci.2010.04.026
NBSC. (2022). National Bureau of Statistics of China. Available at: http://www.stats.gov.cn/sj/
Nguyen, T. T., Timilsina, R. R., Sonobe, T., and Rahut, D. B. (2023). Interstate war and food security: implications from Russia's invasion of Ukraine. Front. Sust. Food Syst. 7:1080696. doi: 10.3389/fsufs.2023.1080696
Ong, S., Choudhury, D., and Naing, M. W. (2020). Cell-based meat: current ambiguities with nomenclature. Trends Food Sci. Technol. 102, 223–231. doi: 10.1016/j.tifs.2020.02.010
Ortega, D. L., Hong, S. J., Wang, H. H., and Wu, L. (2016). Emerging markets for imported beef in China: results from a consumer choice experiment in Beijing. Meat Sci. 121, 317–323. doi: 10.1016/j.meatsci.2016.06.032
Ortega, D. L., Sun, J., and Lin, W. (2022). Identity labels as an instrument to reduce meat demand and encourage consumption of plant based and cultured meat alternatives in China. Food Policy 111:102307. doi: 10.1016/j.foodpol.2022.102307
Ortega, D. L., Wang, H. H., and Olynk Widmar, N. J. (2014). Aquaculture imports from Asia: an analysis of US consumer demand for select food quality attributes. Agric. Econ. 45, 625–634. doi: 10.1111/agec.12111
Pakseresht, A., Kaliji, S. A., and Canavari, M. (2022). Review of factors affecting consumer acceptance of cultured meat. Appetite 170:105829. doi: 10.1016/j.appet.2021.105829
Post, M. J. (2012). Cultured meat from stem cells: challenges and prospects. Meat Sci. 92, 297–301. doi: 10.1016/j.meatsci.2012.04.008
Revelt, D., and Train, K. (1998). Mixed logit with repeated choices: households' choices of appliance efficiency level. Rev. Econ. Stat. 80, 647–657. doi: 10.1162/003465398557735
Rubio, N. R., Xiang, N., and Kaplan, D. L. (2020). Plant-based and cell-based approaches to meat production. Nat. Commun. 11, 1–11. doi: 10.1038/s41467-020-20061-y
Scarpa, R., Thiene, M., and Train, K. (2008). Utility in willingness to pay space: a tool to address confounding random scale effects in destination choice to the Alps. Am. J. Agric. Econ. 90, 994–1010. doi: 10.1111/j.1467-8276.2008.01155.x
Sharma, R., Nguyen, T. T., and Grote, U. (2018). Changing consumption patterns - drivers and the environmental impact. Sustain. For. 10:4190. doi: 10.3390/su10114190
Sheng, J., Shi, H., Diao, M., and Liu, R. (2020). Will artificial meat be accepted by the public? A review of consumer research literature. Food Nutr. China 26, 39–43. doi: 10.19870/j.cnki.11-3716/ts.2020.06.010
Shi, L., Chen, X., and Chen, B. (2023). Covid-19-tested food labels. Can. J. Agric. Econ. 71, 203–230. doi: 10.1111/cjag.12327
Siegrist, M., and Hartmann, C. (2020). Consumer acceptance of novel food technologies. Nat. Food 1, 343–350. doi: 10.1038/s43016-020-0094-x
Siegrist, M., and Sütterlin, B. (2017). Importance of perceived naturalness for acceptance of food additives and cultured meat. Appetite 113, 320–326. doi: 10.1016/j.appet.2017.03.019
Simeone, M., and Scarpato, D. (2020). Sustainable consumption: how does social media affect food choices? J. Clean. Prod. 277:124036. doi: 10.1016/j.jclepro.2020.124036
Slade, P. (2018). If you build it, will they eat it? Consumer preferences for plant-based and cultured meat burgers. Appetite 125, 428–437. doi: 10.1016/j.appet.2018.02.030
Sodano, V., Gorgitano, M. T., Verneau, F., and Vitale, C. D. (2016). Consumer acceptance of food nanotechnology in Italy. Br. Food J. 118, 714–733. doi: 10.1108/BFJ-06-2015-0226
Tilman, D., and Clark, M. (2014). Global diets link environmental sustainability and human health. Nature 515, 518–522. doi: 10.1038/nature13959
Train, K. E. (1998). Recreation demand models with taste differences over people. Land Econ. 74, 230–239. doi: 10.2307/3147053
Train, K. E. (2009). Discrete choice methods with simulation. Cambridge: Cambridge University Press.
Train, K., and Weeks, M. (2005). Discrete choice models in preference space and willingness-to-pay space. Berlin: Springer Netherlands, 1–16.
Tuomisto, H. L., Ellis, M., and Haastrup, P. (2014). “Environmental impacts of cultured meat: alternative production scenario,” in 9th International Conference on Life Cycle Assessment in the Agri-Food Sector, San Francisco, CA.
Tuomisto, H. L., and Teixeira de Mattos, M. J. (2011). Environmental impacts of cultured meat production. Environ. Sci. Technol. 45, 6117–6123. doi: 10.1021/es200130u
Van Loo, E. J., Caputo, V., and Lusk, J. L. (2020). Consumer preferences for farm-raised meat, lab-grown meat, and plant-based meat alternatives: does information or brand matter? Food Policy 95:101931. doi: 10.1016/j.foodpol.2020.101931
Vanhonacker, F., Van Loo, E. J., Gellynck, X., and Verbeke, W. (2013). Flemish consumer attitudes towards more sustainable food choices. Appetite 62, 7–16. doi: 10.1016/j.appet.2012.11.003
Verbeke, W., Sans, P., and Van Loo, E. J. (2015). Challenges and prospects for consumer acceptance of cultured meat. J. Integr. Agric. 14, 285–294. doi: 10.1016/S2095-3119(14)60884-4
Verneau, F., Caracciolo, F., Coppola, A., and Lombardi, P. (2014). Consumer fears and familiarity of processed food. The value of information provided by the FTNS. Appetite 73, 140–146. doi: 10.1016/j.appet.2013.11.004
Wang, H. H. (2022). The perspective of meat and meat-alternative consumption in China. Meat Sci. 194:108982. doi: 10.1016/j.meatsci.2022.108982
Wang, H. H., Chen, J., Bai, J., and Lai, J. (2018). Meat packaging, preservation, and marketing implications: consumer preferences in an emerging economy. Meat Sci. 145, 300–307. doi: 10.1016/j.meatsci.2018.06.022
Wang, H., Chen, Q., Zhu, C., and Bao, J. (2022). Paying for the greater good? What information matters for Beijing consumers’ willingness to pay for plant-based meat? Food Secur. 11:2460. doi: 10.3390/foods11162460
Wang, R., Puillet, L., Pinsard, C., Lescoat, P., and Accatino, F. (2024). Regional-scale trade-offs among beef production, emissions and land use impacts: a cattle diet composition perspective. Agric. Syst. 220:104048. doi: 10.1016/j.agsy.2024.104048
Wilks, M., and Phillips, C. J. (2017). Attitudes to in vitro meat: a survey of potential consumers in the United States. PLoS One 12:e0171904. doi: 10.1371/journal.pone.0171904
Zhang, T., Chen, J., and Grunert, K. G. (2022a). Impact of consumer global–local identity on attitude towards and intention to buy local foods. Food Qual. Prefer. 96:104428. doi: 10.1016/j.foodqual.2021.104428
Zhang, L., Hu, Y., Badar, I. H., Xia, X., Kong, B., and Chen, Q. (2021). Prospects of artificial meat: opportunities and challenges around consumer acceptance. Trends Food Sci. Technol. 116, 434–444. doi: 10.1016/j.tifs.2021.07.010
Zhang, M., Li, L., and Bai, J. (2020). Consumer acceptance of cultured meat in urban areas of three cities in China. Food Control 118:107390. doi: 10.1016/j.foodcont.2020.107390
Keywords: artificial meat, willingness to pay, choice experiment, information intervention, China
Citation: Yang M, Min S, Nguyen TT and Qing P (2024) Promoting artificial meat to improve food security and reduce resource-environment pressure: is it practicable in China? Front. Sustain. Food Syst. 8:1488747. doi: 10.3389/fsufs.2024.1488747
Received: 30 August 2024; Accepted: 13 November 2024;
Published: 27 November 2024.
Edited by:
Haohao Wu, Ocean University of China, ChinaReviewed by:
Nicolas Merener, Torcuato di Tella University, ArgentinaCopyright © 2024 Yang, Min, Nguyen and Qing. This is an open-access article distributed under the terms of the Creative Commons Attribution License (CC BY). The use, distribution or reproduction in other forums is permitted, provided the original author(s) and the copyright owner(s) are credited and that the original publication in this journal is cited, in accordance with accepted academic practice. No use, distribution or reproduction is permitted which does not comply with these terms.
*Correspondence: Trung Thanh Nguyen, dGhhbmgubmd1eWVuQGl1dy51bmktaGFubm92ZXIuZGU=; Shi Min, bWluQG1haWwuaHphdS5lZHUuY24=
Disclaimer: All claims expressed in this article are solely those of the authors and do not necessarily represent those of their affiliated organizations, or those of the publisher, the editors and the reviewers. Any product that may be evaluated in this article or claim that may be made by its manufacturer is not guaranteed or endorsed by the publisher.
Research integrity at Frontiers
Learn more about the work of our research integrity team to safeguard the quality of each article we publish.