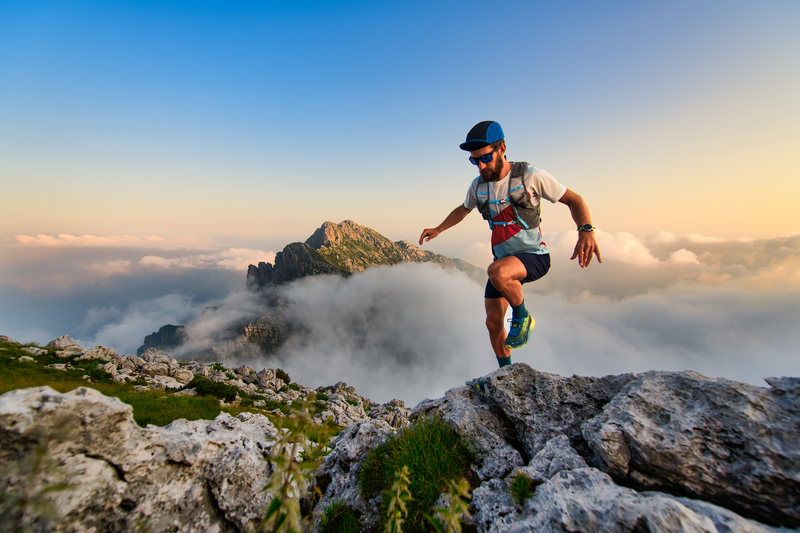
94% of researchers rate our articles as excellent or good
Learn more about the work of our research integrity team to safeguard the quality of each article we publish.
Find out more
ORIGINAL RESEARCH article
Front. Sustain. Food Syst. , 04 December 2024
Sec. Land, Livelihoods and Food Security
Volume 8 - 2024 | https://doi.org/10.3389/fsufs.2024.1466008
Cropland expansion, driven by increasing population pressure and economic demands, is a major contributor to accelerating deforestation. While most studies have focused on the spatial and temporal analysis of landscape changes using Geographic Information Systems (GIS) and remote sensing, there has been less emphasis on the socioeconomic analysis of these changes. Understanding the socioeconomic dynamics of affected areas is essential for implementing practical corrective actions. We apply linear programming and welfare maximization theory to examine how population growth impacts competition between agriculture and forest land use, per capita cash income, and subsistence consumption from privately owned farms. Focusing on the Tchamba district in Togo, we aim to determine the sustainability of farming systems to better inform the ongoing Forest Landscape Restoration (FLR) program. The findings show that the current agricultural systems can support the population growth rate (2.68% per year in the Tchamba district) and per capita income until at least 2063. However, the current agricultural systems cannot support one human generation, which is estimated to be 65 years. These results underscore the need to enhance farming technologies, boost land productivity, diversify income sources to meet the rising food demand and curb forest clearing for farmland expansion. By integrating these insights into the FLR program, this research highlights the importance of a comprehensive policy framework that supports sustainable agricultural practices, community engagement, enforcement of regulations, and economic diversification. This approach will guide restoration efforts and strengthen the resilience and productivity of agricultural systems under population and income pressures.
The world is experiencing rapid deforestation and land degradation, significantly exacerbating climate change and biodiversity loss, harming livelihoods, food security, and the ability to withstand challenges, putting the entire human population at risk (Yesuf et al., 2019). Currently a quarter of the world’s land is degraded, with mounting pressure from factors such as population growth, inappropriate agricultural practices leading to the conversion of forests and intact ecosystems into farmland. This results in an annual loss of 24 billion tonnes of arable land (CIFOR, 2021; Hosonuma et al., 2012; Stanturf et al., 2015). Such degradation contributes to the loss of crucial ecosystem services, impacting the lives of 3.2 billion people worldwide, particularly emphasizing the vulnerability of communities in developing regions like Sub-Saharan Africa (SSA) (CIFOR, 2021; IFAD, 2021). In SSA, where over 75% of the population lives in rural areas, relying on small-scale food production systems, land degradation and soil erosion are becoming more pronounced (ICRAF, 2022; Mapfumo et al., 2013). Tully et al. (2015) highlight that agricultural expansion and intensification, driven by population growth, are fundamental causes of soil degradation, leaving subsistence-based farming systems in SSA particularly vulnerable. As populations grow, agricultural extensification escalates, transforming forest lands into agriculture to meet rising food demand (Potapov et al., 2022). Extensive research across regions like Africa, Brazil, and Southeast Asia has shown that cropland expansion driven by population growth and economic pressures accelerates deforestation (Potapov et al., 2022; Qasim et al., 2013; Xu et al., 2021), highlighting the critical need for sustainable land-use management.
These global trends resonate strongly in the Tchamba district, where smallholder farmers are facing grapple similar challenges. In this area, mono-cropping and shifting agriculture practices have resulted in ongoing deforestation as farmers clear forest land in search of more fertile soil (Kombate et al., 2022). Since 2015, the Tchamba district, a significant agricultural region, has been transitioning toward cultivating high-value crops. The economic potential of soybean, yam, and cashew is a driving force behind this shift, as these crops have become increasingly popular in global markets (Bassan et al., 2020b). However, this change is intricately linked to deforestation in Tchamba, as extensive land clearing is undertaken to establish soybean plantations (Kombate et al., 2020). The rising demand for soybeans in animal feed, biodiesel, and food products has made them a lucrative crop in Tchamba. Consequently, soybean production has expanded significantly, contributing to deforestation in the Tchamba district (Kombate et al., 2023). According to Kombate et al. (2022), large areas of land have been cleared for soybean cultivation, resulting in the destruction of forests and natural habitats. This deforestation has negative environmental impacts, such as soil erosion, habitat destruction, and loss of biodiversity. To address these issues, the government and local communities in Tchamba must adopt and promote sustainable agricultural practices. This could include implementing reforestation programs, employing agroforestry techniques, and advocating for sustainable land use practices. Notably, there are ongoing Forest Landscape Restoration (FLR) activities in Tchamba initiated in September 2020 through the global Project on FLR and Governance in Ethiopia, Togo, Madagascar, Benin, and Cameroon of the Deutsche Gesellschaft für Internationale Zusammenarbeit (GIZ Forests4Future, F4F). By combining these ongoing initiatives with new measures, it may be possible to find a balance between the economic benefits of resource management and the need to protect the environment and preserve natural habitats. FLR emerges as a vital approach to address these issues (Eshetu et al., 2024; Mansourian et al., 2024). FLR aims to restore ecological integrity while enhancing human well-being (Mansourian et al., 2020), making it a comprehensive solution that aligns with sustainable agriculture and conservation goals in Tchamba. However, before implementing any restoration program, it is essential to evaluate the sustainability of current farming systems. Understanding the sustainability of these systems will guide how restoration efforts should proceed, ensuring that agricultural practices are sustained and improved to support both environmental and economic objectives.
Scholars have investigated the causes of forest degradation, deforestation and land use change. Evidence includes studies analyzing driving factors in Pakistani districts (Qasim et al., 2013); spatio-temporal changes in Greater Lomé, Togo (Blakime et al., 2024); conservation constraints in central Togo (Bassan et al., 2020a); the investigation of the current factors of cropland and forest degradation in the Kloto district in Togo (Koglo et al., 2018); factors influencing agricultural changes in Cambodia (Kong et al., 2019); the assessment of changes in the landscape in Rizal, Philippines (Olfato-Parojinog et al., 2023); the analysis of cost–benefit and assessment of farming returns in West Africa (Bassan et al., 2020b). Other research quantifies deforestation in Madagascar (Yesuf et al., 2019) and assesses land occupations in Brazil (Brown et al., 2016). However, many of these studies emphasize spatial and temporal analysis using geographic information systems (GIS) and remote sensing, with limited attention to socioeconomic drivers. Existing research focuses on how cost benefits and farming returns to farmers predict deforestation (Bassan et al., 2020b) and the analysis of land use and land cover (LULC) change in Tchamba Prefecture (Hounkpati et al., 2024).
In this context, examining the socioeconomic factors driving deforestation and forest management outcomes reveals a nuanced and context-specific interplay that complements understanding land use changes. For instance, Borrego and Skutsch (2019) found that both wealthier and poorer farmers in Western Mexico contribute to forest degradation through shifting cultivation, though wealthier farmers are more likely to engage in temporary land clearances, underscoring the importance of considering intra-community socio-economic disparities when designing REDD+ interventions. Similarly, Shrestha et al. (2018) revealed that Nepal’s community forestry program positively impacted forest cover retention, with districts having more community forests experiencing lower deforestation rates and higher tree cover recovery. In Northern Pakistan, Ullah et al. (2022) identified a range of socioeconomic factors—such as poverty, low literacy, and agricultural expansion—that exacerbate deforestation, suggesting that economic incentives could mitigate these pressures. The interaction between land use changes and socioeconomic conditions has also been linked to public health outcomes, as Andrade et al. (2024) demonstrated with dengue incidence in Brazil, where deforestation correlated with disease prevalence in areas undergoing significant vegetation loss. While these studies address various socioeconomic factors, there remains a need to further explore how population growth impacts competition between agriculture and forest land use, per capita cash income, and subsistence consumption from privately owned farms. The complex, long-term issue of land use requires case studies on local socioeconomic conditions. Few studies address this problem in Tchamba district from a socioeconomic perspective.
Therefore, this research investigates the correlation between deforestation, population growth, and the sustainability of current farming systems at the district level in Tchamba, Togo. It aims to determine the sustainability of farming systems at the district level of Tchamba, Togo, to better inform the ongoing restoration program. We apply mathematical programming, specifically linear programming (LP) and welfare maximization theory, to achieve this objective. Mathematical modelling is a powerful tool that can be used to analyze and optimize farming systems (Folefack et al., 2015; Groot et al., 2012; Jagoret et al., 2009; Norton and Hazell, 1986; Sali et al., 2016). This research aims to provide actionable insights to guide FLR initiatives by establishing the link between deforestation and population growth. By demonstrating how population pressures contribute to deforestation, the study seeks to inform strategies that mitigate the adverse impacts of deforestation, such as habitat loss and biodiversity decline. The findings will emphasize the importance of addressing population growth in the context of sustainable resource management. By integrating these insights within FLR projects, stakeholders can effectively tackle the underlying socio-economic challenges, fostering long-term benefits for ecosystems and local communities. This research underscores the need for a comprehensive policy framework that supports sustainable agricultural practices, community engagement, and economic diversification, ultimately contributing to the success of restoration efforts.
This study was conducted in the Tchamba district, in the central region of Togo (Figure 1). The region covers an area of 3,166 km2, accounting for 23.5% of the central region and 5.6% of the national territory. The district of Tchamba is located in a tropical semi-humid climate of the Sudano-Guinean type, receiving a monomodal type of rainfall characterized by a long rainy season that spans from April to October and a long dry season that lasts from November to March. The highest rainfall amounts are observed during the monsoon season, which typically takes place between July and September. They have one rainy season, and the average annual rainfall varies between 1,200 and 1,300 mm. This climatic characteristic does not favour the occurrence of dense forests, which require a minimum annual rainfall of 1,500 mm distributed throughout the year and a dry season of less than 3 months (Chevalier, 1933). However, dense forest patches exist within the savanna ecosystem, particularly at lower latitudes, where they predominate over the savannas. Tchamba is a part of the vegetation cover type that stretches from the savanna forests of the Upper Ivory Coast, encompassing both wooded savanna and forest (Bassan et al., 2020b). Agriculture serves as the primary economic activity in the district, with farmers practising small-scale farming through shifting cultivation and crop rotation. Farmers grow various crops, including maize, soybean, and yam, with an average of about 3 hectares (ha) of cropland per farmer (own estimation based on field data). Yam cultivation is particularly notable, as it thrives well on newly converted forest land or dense wooded savanna. Typically, the farming cycle begins with yam in the first year, followed by other food crops such as cereals, tubers, beans, and cash crops in subsequent years. Given the reliance on these practices, the annual land area dedicated to yam, soybeans, and maize were used as constraints in the linear programing to reflect land availability limits. Land conversion to agriculture often involves transforming wooded savanna areas as well.
Figure 1. Localization and land use land cover change (LULC 2022) (Hounkpati et al., 2024) of the study area.
The Tchamba district is an interesting case study because it is a region where particularly negative trends have been observed in forest resource management. Tchamba district is experiencing significant deforestation due to logging, agricultural expansion, and human settlement (Kombate et al., 2023). Due to these characteristics, the region has also become of interest to different international organizations and actors who aim to contribute toward landscape restoration, including Forests4Future (F4F). F4F is a global project by GIZ focused on Forest Landscape Restoration and Governance in Ethiopia, Togo, Madagascar, Benin, and Cameroon. Households participating in F4F were the source of the data collected for this study.
The farm household is a crucial decision-making unit in many contexts. It is distinguished by the fact that it is both a producer and a consumer of a set of products. Food and family time (used for work or leisure) are typical examples of these products (Gibreel, 2009). Thus, the farm household model considers this non-separability between production and consumption. In this study of the sustainability of farming systems, the hypotheses are guided by economic models. Most farm household models have been studied, but for simplicity, we assume the farmer’s strategy is to produce crops to meet his family’s basic needs, such as food and cultural requirements. This assumes that the labour used to plant crops cannot exceed the total amount of effective household family labour available for farming activities, as there may not be a perfect labour market where farmers can hire additional labour. According to Maslow’s hierarchy of needs, these physiological requirements must be met before the farmer can move on to higher needs, such as earning cash income, which was done by selling surplus crops. This approach ensured that the model was not only maximizing net cash income but also considering the household’s well-being.
As the population increases, so does the demand for food and other goods, increasing household consumption. Household consumption refers to the goods and services a household consumes or uses, such as food, clothing, housing, transportation, and so on. In this analysis, household consumption was estimated exogenously, which means that it was estimated from outside the modeled system. Specifically, the growth rate of the population was used as a proxy to estimate the increase in household consumption. The population growth rate is the rate at which the population is increasing over time. By using the population growth rate as a proxy for household consumption, the assumption is that the two are closely related: as the population grows, so does household consumption. It’s worth noting that this assumption may not be entirely realistic, as other factors beyond population growth can also affect household consumption, such as changes in income, technology, and preferences. Furthermore, it was assumed that per capita consumption would remain unchanged over time. The assumption is that as the population grows, each person will continue consuming the same goods and services as before. Based on this framework, household consumption over time was captured and estimated as follows.
Where Ct is household consumption in year t, Co is household consumption in the base year (kg per household), r is population growth rate (%), and t is time horizon, i.e., 10, 20, 30, or 40 years. The existing farm plan contained the base year values used to compute future household consumption for each crop.
According to Equation 1, an increase in the population growth rate (%) would necessitate an increase in household consumption in a given year, which consequently would increase deforestation through the expansion of cropland to meet the increasing food demand in the condition of Tchamba district where farming is in a significant part extensive (Bassan et al., 2020b). This is because extensive farming typically involves clearing large areas of forested land to create space for crops and livestock.
Building and analyzing mathematical models can help researchers and farmers identify optimal strategies for managing farming systems, such as choosing the best crops to plant in a given region or determining the most effective irrigation schedule. Usually, LP models are typically static. However, in this study, we introduced population growth as a dynamic element to capture temporal variations. This approach could serve as a foundation for developing strategies to enhance land-based resource utilization in the Tchamba district.
When farmers decide to diversify or specialize and allocate lands to different crops and choose crop management options, modelling the resultant multi-objective and multi-activity nature of their households becomes more complex. The decision-making process involves considering different goals, activities, and limitations to maximize overall welfare. For example, diversification is beneficial in spreading risk across various crops or income sources, while specialization may offer more efficient resource allocation and greater profit potential. In situations where econometric analysis within a single cropping year is not feasible using cross-sectional data, mathematical programming approaches are commonly employed (Ruben and van Ruijven, 2001). Mathematical programming involves the optimization of a mathematical function subject to a set of constraints. For agricultural contexts, a mathematical programming model may optimize resource allocation (e.g., labour, land, capital) across crops or activities to maximize household welfare or profits. This modelling approach is useful when assessing policy or intervention impacts on decision-making and welfare. It is not uncommon to use LP to analyze the relationship between deforestation, population growth, and the sustainability of the existing farming systems (Kaoneka and Solberg, 1997). Therefore, this study uses a LP model to capture the relationship between humans and the environment in Tchamba district, Togo.
We used a mixed-methods approach to gather data from primary and secondary sources to generate model coefficients. Concerning secondary data sources, we use the baseline data of the F4F project, country package Togo, to cross-check with the household data from the primary source. We obtained the region’s value chain data of the main crops grown in the baseline report. Data from the primary source were obtained through structured and semi-structured interviews alongside direct observations. We surveyed 456 farmers in Tchamba district, central region of Togo, to gather information on the yield of farm crops, population size, density, and growth rate. Socioeconomic data comprises the annual income of individuals or households, the income per capita, the availability of seasonal labour, the household size, and any off-farm activities that individuals or households engage in to understand their economic status and financial activities. Land use data comprise sources of land, which could be through inheritance, purchase, rent, or village allocation, the land tenure system and ownership, along with the size of the land holdings, average land size, range of land size, distribution of land, and spatial arrangement. Additionally, land use data covers homestead, crops, trees, and crop production, including details on main crops, land preparation, planting, inputs, weeding, harvesting, pesticide spraying, and storage methods. The aim is to comprehensively understand land-related practices and agriculture-related activities within the region under study.
The enumerated crops were maize, soybean, and yam. To standardize measurements, the bowl (for cereals) and calabash (for yams) were converted to bags, with one bag equal to 40 bowls or approximately 100 kilograms (kg). Each yam tuber weighed 3.33 kg, and a calabash held around 30 tubers, equivalent to about 100 kg1.
These conversion weights were based on market surveys conducted at Tchamba market during the fieldwork. We gathered information from various vendors and traders at the market to determine the most appropriate conversion weights for our analysis. Based on the results of our surveys, we determined that these particular conversion weights are the most appropriate and accurate for this study. We also used the data to develop the input–output matrix for the farming systems on a per-ha basis for an average farmer, as shown in Supplementary Table S1. The entries in Supplementary Table S1 are the required input in numerical form for each crop in a given farming system during the cropping season. 0 means no labour was needed or no fertilizer was bought or used. Supplementary Table S1 also shows the operating accounts of crops according to the different farming systems. Three major farming systems in the study area are monocropping, intercropping, and crop rotation. According to Power and Follett (1987), monocropping is repeatedly growing crops on the same land. Intercropping is a multiple-cropping system in which two or more crop species are planted simultaneously in a field during a growing season (Mousavi and Eskandari, 2011). Dury et al. (2012) defined Crop rotation as growing a series of different plant species on the same land.
The objective function in this linear programming model is designed to maximize the net cash income (NCI) generated by the farm. We achieved this by considering farm-gate prices as crop sales coefficients, representing the primary source of cash income. The model also includes other activities, like purchasing nitrogen, phosphorus and potassium (NPK), Urea 46 and Chloride of potassium (KCL) fertilizers, which are based on their respective market prices and used as coefficients in the objective function. The cost of working capital borrowing is also included in the objective function and is calculated using the prevailing interest rate. Land-clearing activities are also taken into account as part of the objective function.
The model also considers what the family needs to eat by setting a minimum subsistence requirement for each crop. The output allocation is based on the assumption that the farmer’s strategy is to produce crops that meet his family’s basic needs, including food and cultural requirements. This method ensures that the model maximizes the net cash income and considers the household’s well-being.
We assumed that farmers could access working capital from microfinance institutions, such as the Women’s Mutual Savings and Loan Fund of Tchamba (UCMECF-TO) and the Cooperative Action for Socioeconomic Development and Household Fulfilment (COOPEC ADESEM), whenever opportunities for borrowing were available. As of 2022, these institutions levy an interest rate of 18% per annum on such loans. It should be noted that we did consider the impact of inflation in this scenario. Therefore, the interest rate of 18% per annum is integrated into the model. According to Norton and Hazell (1986), linear programming problems aim to maximize an objective function (Equation 2) subject to constraints (Equation 3) such as the availabilities of land, labour, capital, and credit as well as, nutrient t and consumption requirements. The mathematical formulation of each activity and constraints is shown below. Linear programming tableau or aggregated tableau of the model in numerical form is also shown in Supplementary Table S2. The entries in Supplementary Table S2 are the model’s coefficients; a positive coefficient indicates the quantity of input required or activity demand for a resource, while a negative coefficient in the objective function is the amount of money spent to purchase a resource. A negative coefficient also represents the activity supply of a resource. “−1” indicates that the payment was made for fertilizer and manure. Fertilizers are used for some crop pair combinations and not others because crops such as legumes fix nitrogen, and farmers do not often apply fertilizer for those crops. The model is constructed for the representative household. We used Generalized algebraic modelling system (GAMS) to estimate the model.
Subject to constraints:
Where:
Xj = is the level of the jth decision variables; Cj = profit of a unit of the j decision variable or activity; aij = quantity of the ith resource to produce one unit of the jth decision variable; bi = amount of ith resource available; n = 16 decision variables grouped into 4 groups which are crop production activities, purchase activities, clearing activities, and crop sales activities; NCI = total profit aggregated from the 4 activities group; j = decision variables, i = resource.
The activities, also known as decision variables, include cropping, selling, purchasing, borrowing, land rental, and consumption. The major activities used in the model are presented below.
The production activities of the programming model, which Xi represents, are based on the number of ha of land allocated to each crop i, where i = 1, 2, … 7 (see the Appendix for the definition of Xi.). In the study area, three major farming systems for these crops are essential to the local population’s dietary habits. This region is particularly suitable for their cultivation due to the favourable soil conditions. The three major farming systems are monocropping, intercropping, and crop rotation (we defined the three farming systems in the data types and sources section). Thus, understanding the relationship between land allocation and crop production is essential to optimizing agricultural productivity in the region and ensuring an adequate food supply for the local population.
Purchasing activities include NPK, Urea 46 and KCL fertilizers based on their respective market prices. A borrowing activity is included as a purchase activity since the farmer might want to borrow at the existing interest rate to the extent that this is profitable. The sales activities represent the surplus of different crops produced (see the Appendix for the definition of purchase and sale activities).
The crop product markets are well-functioning compared to other markets in the study area. However, cultural (habitual) and social issues force households to grow and store their production for consumption purposes. When subsistence constraints are met, households often generate income by selling the available surplus. Thus, consumption is included as a separate activity.
Such activities are clearly defined to incorporate land-clearing activities into the model. This definition serves as the basis for including land-clearing activities and ensures their accurate representation in the model. We introduce population growth as a dynamic element in our model and make certain important assumptions. First, we assume that the same farming techniques will be used in the future. Secondly, we assume that new land will only be cleared through the use of family labour without the provision of hired labour. The model also considers the importance of avoiding unnecessary deforestation and only clearing new land when there is demand for it. It was recognized that clearing new land should not be done unless necessary under the model’s conditions. The model estimates that clearing forest land would require 16 person-days per ha, reflecting the demanding nature of the work. Additionally, an average working capital requirement of 30,000 FCFA per ha is assumed for the purchase of additional hand tools, such as cross-cut saws, axes, and pangas (see the Appendix for the definition of land clearing activities).
The core components of a household utility maximization function include income, leisure time, and essential food needs. Typically, decisions regarding leisure and income are interdependent. Households in Tchamba district, adhering to traditional mosque practices, strictly observe religious holidays, during which farm work is prohibited (Holden et al., 2004). Therefore, these holidays must be deducted to determine the actual number of working days available for farming. Any day not designated as a religious holiday is dedicated to farm work. Consequently, leisure time is confined to mosque holidays and can be considered fixed and separable from income in the utility function. The labour supply for any given month must not surpass the total number of effective working days. Additionally, the labour required to cultivate 1 ha of any crop must not exceed the effective household family labour available for farming activities, thereby eliminating the need for additional hired labour. The average household size, determined from a sample of 456 households, is 7.5 persons (based on field survey data). It is assumed that in each month, 80% of the days (approximately 24 days) are available for farming activities. Family members younger than 15 years old, who constitute about 10% of the household, are excluded from farming activities as they primarily attend school. Thus, the average household has about 6.8 adults available for farming, translating to 163 person-days per month. Therefore, the average household adult labour available for farming activities is 6.8 persons. It is presumed that each household member spends an equal amount of time working on the farm each day. Only peak farming months (April to October), which coincide with the rainy season, are considered for constraints when labour might be limited.
The total amount of land used for farming is not divided into groups based on how it was used. The field survey indicates that the land was used for planting crops such as maize, soybeans, and yams during the rainy season. In addition, as these crops are planted only once each year, there was no need to further classify the farmland. Based on an average farm size of 3 ha per household, the total soybeans and maize area is estimated to be 2 ha, and 1 ha for yam land per household (based on the field survey and experience). As soybeans, maize, and yams are planted once each year during the rainy season, the land area available in each season for planting these crops is taken to be 3 ha. In a mono-cropping system, on the other hand, once maize is planted, it stays on 1 ha of land for the whole year. Therefore, the land area available each season for planting maize, beans, and yam is 3 ha.
Phosphorus, an essential macronutrient for crop growth, is typically applied during planting. In Tchamba, the standard annual fertilization rate is 250 kg/ha of NPK 15-15-15, a commercial fertilizer containing 15% phosphorus by weight, resulting in 37.5 kg P/ha. This aligns with the phosphorus requirement of maize, which is also 37.5 kg P/ha. Therefore, the quantity of phosphorus fertilizer purchased must not be less than that required by the allocated agricultural systems.
Nitrogen (N), similar to phosphorus, is crucial for crop growth and is typically applied as a top dressing. The application rate in Tchamba is 100 kg/ha of Urea, which contains 46% N by weight, equating to 46 kg N/ha. This matches the nitrogen requirement for maize, which is also 46 kg N/ha. Therefore, the quantity of nitrogen fertilizer purchased must not be less than that required by the allocated farming systems.
Like phosphorus and nitrogen, potassium is an essential macronutrient required by plants for many physiological processes, such as regulating water balance, forming sugars and starches, and synthesizing proteins. In yam crops, potassium is vital in promoting root growth, tuber enlargement, and overall plant vigor. Additionally, potassium helps to improve the plant’s resistance to disease and environmental stresses, such as drought. The application rate is 125 kg/ha of KCL by weight of K (65.45 kg of K). Yam required 65.45 Kg of K/ha. The quantity of potassium fertilizer purchased must not be less than that required by the allocated farming systems.
Farmyard manure is regularly used as a way to take care of the land. It adds nutrients and organic matter, provides cation exchange sites, and changes the soil’s physical properties. The manure application rate in Tchamba district is 1.5 tonnes per ha (ITRA, 2021). Based on socioeconomic and agricultural surveys conducted by the Division of Agricultural Statistics, Informatics, and Documentation (DSID) of the Ministry of Agriculture, households may obtain a maximum of 5 t of manure per year. The total quantity of farmyard manure applied must not exceed the maximum amount a household can obtain annually.
In this context, working capital refers specifically to the funds allocated for purchasing seeds, hand tools, and pesticides. The required amount of working capital fluctuates depending on the crop type and agricultural system. A borrowing option is included, allowing farmers to take loans at the prevailing interest rate when profitable opportunities present themselves.
Credit limit constraints are incorporated into the model to cap the amount of working capital that can be borrowed. This maximum credit limit encompasses both working capital and fertilizer purchase costs. Based on field surveys, the capital limit is initially set at 2402500 FCFA, which represents the average expenditure on purchasing market inputs.
The total maize produced is estimated to be 1,630 kg per ha in the Tchamba area as of 2022, based on field surveys and personal experience. About 70% of this production is assumed to be used to meet household subsistence needs. For a household with an average size of 7.5 persons, the amount set aside for household subsistence requirements is taken as 150 kg per person, resulting in a total household requirement of 1,125 kg per year. It is necessary for the maize produced in all systems to at least meet these subsistence requirements. The value of subsistence requirements is used as the right-hand side (RHS) in the consumption requirement constraint equation.
Approximately 22% of the total soybean harvest is allocated to fulfill the household’s basic protein needs. Based on field surveys and practical experience, the average soybean yield is estimated at 1,213 kg/ha. Each person requires an average of 36 kg of soybeans annually, resulting in a total household consumption requirement of 270 kg per year. Consequently, the soybean production across all farming systems must meet or exceed these subsistence needs.
In the field survey data and based on experience, it is estimated that about 19% of the yams produced are used to satisfy household demand. The assumed crop yield is 9,691 kg/ha and the consumption rate is 245 kg per person annually, resulting in an estimated total household consumption of 1841 kg/year. This means the quantity of yams produced should meet the subsistence requirements of the household.
The LP models developed for farm household farming systems in this study draw inspiration from Dykstra (1984) and Sankhayan and Cheema (1991). The theoretical framework integrates general microeconomic theory relevant to farming systems, household economic theory as outlined by Low (1986), Nakajima (2012), and principles of linear programming. This comprehensive approach guided the design of the model, specification of parameters, and definition of underlying assumptions and logic, facilitating a robust analysis and discussion of the results.
According to Equation 1, an increase in the population growth rate (%) will necessitate an increase in household consumption in a given year, which will consequently increase deforestation in the Tchamba district, Togo, where farming is, in significant part, extensive (Bassan et al., 2020b). This is because extensive farming typically involves clearing large areas of forested land to create space for crops and livestock. Thus, in this study, we hypothesize that:
Hypothesis 1: Considering the trends in population growth, the existing farming systems are not sustainable.
Hypothesis 2: Population growth will lead to deforestation as farmlands expand for food and future production.
We analyze three scenarios to test these hypotheses and to capture how the situation varies over time. They are summarized as follows.
Scenario 1: We assume a population growth rate of 2.68% per year over 50 years, while maintaining per person cash income and food consumption from own farm non-declining.
Scenario 2: We assume a population growth rate of 3% per year over 50 years, while maintaining non-declining per person cash income and food consumption from own farm.
Scenario 3: We assume a population growth rate of 2.68% per year and an increase in net cash income per capita to 2% annually over 50 years while maintaining per capita food consumption non-declining.
In this section, we present the outcomes of the linear programming analysis, which explored three scenarios to evaluate the sustainability of farming systems in the Tchamba district over time. The results assess the impact of varying population growth rates, agricultural practices, and income levels on land use and deforestation, providing insights into the dynamics of resource utilization and the potential implications for sustainable development in the region. The LP model was used to optimize farm plans under each scenario by balancing the competing objectives of subsistence needs and economic sustainability. These findings directly relate to our hypotheses by illustrating the unsustainable nature of existing farming systems and the pressures of population growth on deforestation.
The results reveal a declining trend in net cash income over time, which may be linked to a decrease in soybean sales (one of the main cash crops sold over the period), corresponding to an increase in soybean land (Table 1). This trend supports Hypothesis 1, suggesting that current farming systems are not sustainable given the continuous population pressure leading to declining income. This trend could be ascribed to more family labour being diverted to cash crops, yams, and soybeans to satisfy the household demand for food and income. This seems logical as the model assumes that any rational farmer would secure subsistence requirements before selling farm crops. Further, as long as the farmer relies on family labour, any diversion of it to cash crop production will decrease the labour available for food crop production.
Table 1. Optimum farm plans generated over time for an average farmer assuming a population growth rate of 2.68% per year throughout 50 yearsa (all figures are per household).
A total of 1.33 ha of land was cleared in year 10 to compensate for the 2.33 ha allocated, with 1 ha each dedicated to soybean and maize production. This finding indicates that farmers may resort to clearing more land as population growth pressures increase, which aligns with Hypothesis 2. The rationale behind this land clearing is based on the principle that the combined area for maize and soybean cultivation should not exceed 2 ha (1 ha for each crop) in the Tchamba district. Therefore, any optimal farm plan that allocates more than 1 ha to either soybean or maize production will likely contribute to deforestation through land clearing. This approach stems from non-forest lands being deemed unsuitable for soybean production, which requires fertile, well-drained soil conditions. Non-forest lands are instead considered more suitable for yam cultivation, a crop better adapted to those soil types.
Given that soybean is currently one of the primary cash crops in the Tchamba district, the decision to clear more than 1 ha for soybean is driven by farmers’ desire to maximize their income. By increasing the land area available for soybean production, farmers aim to capitalize on the crop’s economic potential. Consequently, from year 20 onwards, there has been an exponential increase in the land cleared for soybean cultivation. This trend is expected considering soybean’s significant role as a cash crop in the region. The high demand for soybeans, driven by their versatile applications in animal feed, biodiesel, and food products, has incentivized farmers to expand their soybean cultivation areas. This expansion highlights the tension between economic benefits and environmental sustainability. While increasing soybean production can improve farmers’ incomes and support local economies, it also poses a threat to forested areas, leading to deforestation and the associated environmental impacts.
The LP model captures the economic rationale behind land clearing, where the marginal economic returns from expanding cash crop production outweigh the opportunity cost of leaving the forest intact. The model’s optimization of land allocation underscores how population-driven increases in food demand create pressure to convert forested land for agricultural purposes, emphasizing the need for integrated land-use policies that balance economic objectives with forest conservation.
In the second scenario, assuming a population growth rate of 3% per year over 50 years (as shown in Table 2), there is a more significant decrease in net cash income generated over time compared to the first scenario (shown in Table 1). This further supports Hypothesis 1, as the higher population growth rate exacerbates the decline in economic viability of farming systems. This decline can be attributed to the higher population growth rate of 3% per year, leading to an increased household demand for food. As a result, more family labour is allocated to cash crop production, specifically yam and soybeans, to meet the household’s consumption needs. The land dedicated to yam and soybean cultivation increased significantly to compensate for the quantity consumed by the household. This adjustment, however, resulted in a corresponding decrease in the quantity of yam and soybeans available for sale. As more of these crops were diverted for household consumption, the surplus available for the market diminished, leading to a decline in sales revenue. Consequently, the net cash income, which is derived from the sale of these cash crops, experienced a notable decline.
Table 2. Optimum farm plans generated over time for an average farmer with a population growth rate of 3% per year throughout 50 yearsa (all figures are per household).
The higher population growth rate exacerbates the pressure on agricultural resources, as more land and labour are required to satisfy the food needs of a growing population. This shift in resource allocation highlights the challenges of maintaining economic sustainability under conditions of rapid population growth. The increased demand for food forces households to prioritize subsistence over market-oriented production, thereby reducing their potential cash income. The LP model illustrates the intensifying competition for limited agricultural resources and the resulting socio-economic challenges under rapid population growth. This scenario highlights the need for alternative income sources and diversification of livelihoods to reduce reliance on expanding agricultural land as a means of economic adaptation.
The results representing scenario 3, which assumes an annual population growth rate of 2.68% but includes an intended cash income increase of 2% per year (summarized in Table 3), indicate a declining trend in actual cash income produced over time. This outcome further supports Hypothesis 1 by demonstrating that even with projected increases in income, the economic sustainability of farming systems remains challenged under growing population pressures. The persistent decline in cash income points to a deteriorating welfare situation for the average farmer, rather than an improvement. This trend highlights the inadequacy of existing measures to counterbalance the effects of population growth, leading to a devolutionary cycle of declining welfare. In this scenario, the inability to achieve the projected cash income increase underscores the challenges farmers face in maintaining economic stability amid rising population pressures. As the population grows, the demand for food and resources increases, placing additional strain on the already limited agricultural land and labour. These findings highlight a critical gap in the effectiveness of current policies and practices in addressing the economic needs of farmers, aligning with the hypotheses that current farming systems are unsustainable and that population growth will necessitate further deforestation.
Table 3. Optimum farm plans generated over time for an average farmer with a population growth rate of 2.68% per year and an increase in cash income of 2% per year over 50 yearsa (all figures are per household).
The LP outcomes in this scenario demonstrate that while increasing cash income could theoretically offset some of the negative impacts of population growth, the actual economic dynamics, including limited access to credit, technology, and market opportunities, constrain the potential for income growth. This reveals a gap in policy measures and highlights the importance of supporting farmers with resources that can enhance agricultural productivity without expanding agricultural land.
Based on the analysis, the following conclusions can be drawn: With a population growth rate of 2.68% per year in the Tchamba district (as of 2023), the average household’s farming systems remain economically viable for a period between 40 to 50 years at the current technological level. These conclusions underscore the urgency of addressing the challenges posed by population growth, reinforcing both hypotheses about the sustainability of farming systems and the link to deforestation. This timeframe shifts slightly with a population growth rate of 3% per year (close to the national average of 2.4% according to the 2022 census), sustaining economic viability for the same range of 40 to 50 years. Additionally, under a 2.68% annual population growth rate and a projected 2% annual increase in per capita cash income, the economic sustainability of these farming systems also falls between 40 and 50 years.
The scenarios analyzed appear quite restrictive for farmers. In reality, however, cash income is not typically their primary objective. More often, farmers prioritize food production and cultural needs before focusing on generating cash income. Additionally, in Togo’s rural sector, particularly in the Tchamba district, population growth rarely translates to an increase in per capita income. This disparity is evident in the declining living conditions of the rural population.
This study uses population growth rates as a proxy to establish a link between deforestation and population growth, aiming to determine the sustainability of farming systems over time in Tchamba district, Togo. The analyses reveal that the conflicts between forest land and farmland are very perceptible in the study area. The different scenarios (Tables 1–3) show that cash crop farming, particularly soybean and yam farming, significantly affect the loss of vegetation cover area as more land is cleared to compensate for the number of ha allocated to soybean production. This is explained by the fact that soybeans became the main cash crop in the study area. This implies that soybean production will lead to continuing deforestation if no action is taken. These findings are consistent with the results of Bassan et al. (2020b) in Togo, who show that an increase in the profitability of yam crops resulted in the expansion of farm size and the destruction of forests. This suggests that high-value crops are linked to deforestation as farmers prioritize economic returns over environmental preservation, a trend similarly observed in other regions like Nigeria (Indarto, 2016) and Togo (Kombate et al., 2022), where population growth has increased the demand for agricultural land, exacerbating deforestation.
Our results confirm that cash crops affect forest size, leading to deforestation aligning with the predetermined objective function. Bassan et al. (2020b) verify that external influences like trade policies and international markets for high value crop are drivers of deforestation. Therefore, demand for soybeans for export can result in deforestation in Tchamba. Additionally, an increase in trade coupled with non-sustainable farming systems will cause a general forest decline (Indarto, 2016). Similarly, studies at the global level indicate that cropland expansion has accelerated since 2003, and regions like Africa witnessing increased cropland expansion rates (Potapov et al., 2022). Furthermore, agriculturally high-potential areas such as Brazil have recently faced the conversion of natural lands and pastures into agricultural zones. Such demand has doubled the productivity of crops like soybeans at the expense of environmental conservation (Xu et al., 2021). These results suggest that cash crops like soybeans, if left unchecked, will continue to exacerbate deforestation in Tchamba.
The linear programming (LP) approach used in this study offers a unique perspective by simulating future land use under various scenarios, while considering economic drivers such as population growth and income dynamics. Unlike GIS and remote sensing studies, which focus on past trends, LP modelling allows for the exploration of alternative farm plans and highlights the economic motivations behind land-use decisions. This analysis offers insights into the economic trade-offs that drive land-use decisions, highlighting how increased population and income growth can exacerbate competition for land between agricultural and forest uses. This provides deeper insights into the link between economic incentives and land management, demonstrating how profitability rather than sustainable practices shape farmers’ choices. Furthermore, while GIS and remote sensing give spatial and temporal data on deforestation, LP modelling complements these tools by offering scenario-based exploration, allowing policymakers to predict and mitigate the consequences of future agricultural policies. By comparing these economic factors with spatial trends identified through GIS and remote sensing, the complementary nature of these methodologies becomes evident, as LP modelling incorporates economic factors into land-use analysis. This combined approach provides a more holistic understanding of the interplay between economic drivers and land management decisions, which can be invaluable in designing effective policies that balance economic development with environmental sustainability. The combined effects of an increase in population and income on deforestation are also demonstrated. Since 2000, for instance, more than 1.22 million ha, for instance, shifted annually in or out of US forest use and a large part of the forest is projected even to be converted into farmland by 28.33 million ha by 2030 (Alig and Plantinga, 2004). The results indicate that population expansion directly impacts the extent of forest cover, resulting in deforestation. This aligns with prior expectations and is optimized significantly, as shown by the model. This result is confirmed in the work of Oyetunji et al. (2020), who identify population growth and its resulting effect on deforestation in Nigeria. This is because, as the population grows, the need for the existing forest resources to support and ensure survival increases. Further, population growth increases demand for food, resulting in a general forest decline in Togo (Bassan et al., 2020b). Additionally, higher income levels can worsen this situation by leading to increased consumption, which in turn raises the demand for land for both cash crop cultivation and subsistence farming. As incomes increase, households may expand agricultural production, further encroaching on forested areas (Nurrochmat et al., 2023). This creates a harmful cycle where population and economic growth contribute to deforestation, putting additional pressure on forest ecosystems. Without effective interventions, this trend could jeopardize the ecological balance and diminish the ability of forests to provide crucial ecosystem services such as carbon sequestration and biodiversity conservation.
As population growth drives agricultural expansion, inadequate land-use planning and management practices often exacerbate the associated land-use changes. In regions like Togo, where resources for effective land governance are limited, these challenges can lead to significant pressure on forest resources, accelerating deforestation trends. Per capita cash income also has a negative relationship with forest size in the Tchamba district, aligning with the a priori expectation from the model. This is because cash crops usually result in increased land demand leading to forest decline. Cropland area constantly increases at the district level with a significant inter-period variation. For instance, at a population growth rate of 3%, there is an exponential increase in the conversion of forest land into cropland over time (Table 3). Under increasing population size, farmers can respond in two ways: they can expand their farm size or they can intensify cultivation on existing farms (Ouedraogo et al., 2010). In a situation where most farmers experience financial constraints to access farm inputs (fertilizer and seeds), they opt for expanding cropland size through the conversion of forests and woodlands. The prevailing pattern is exacerbated by limited access to agricultural technology and sustainable land-use practices, leading farmers to depend on traditional, land-intensive farming methods. Consequently, deforestation emerges as the most feasible solution for short-term agricultural productivity, despite its detrimental impact on long-term sustainability. The lack of effective policies to promote sustainable farming methods further perpetuates this trend, making it increasingly challenging to address the environmental consequences of population-driven land use. While enhancing agricultural productivity on existing land is critical, research shows that without clear measures to prevent land expansion, farmers may continue to seek additional land for production gains (Keith et al., 2024; Zabel et al., 2019). Policies such as enforced land-use designations or community agreements can play a key role in ensuring that increased productivity does not inadvertently lead to further deforestation.
These results indicate that the analyzed farming systems are not economically sustainable in the long term. A maximum period of 30 to 40 years is insufficient to support even one human generation, typically lasting around 65 years. This highlights the urgent need to improve farming technologies and practices in the study area. Addressing this issue requires a balanced approach that promotes sustainable agricultural practices, ensuring that economic gains do not come at the expense of the environment. Implementing strategies such as agroforestry, which integrates trees into crop and livestock systems, can help maintain soil health and biodiversity while supporting farmers’ livelihoods. To reverse this declining welfare trend, a multifaceted approach is essential, involving the combined efforts of the government, restoration initiatives or programs, extension workers, and farmers. In addition, allocating resources to education and training initiatives for farmers can enrich their comprehension of sustainable practices, empowering them to make well-informed decisions that support their economic well-being and the environment. Cooperative alliances among stakeholders can further promote the exchange of knowledge and the development of innovative solutions, ultimately resulting in more robust farming systems. Ultimately, nurturing an environment that prioritizes sustainable practices will enhance agricultural productivity and play a significant role in maintaining long-term ecological equilibrium.
Restoration initiatives, such as FLR programs, are crucial in addressing the interconnected challenges of deforestation and agricultural sustainability identified in this study. The research findings highlight how population growth and economic pressures contribute to unsustainable farming practices, leading to land degradation and reduced farm incomes. FLR programs can address these root causes by restoring degraded lands, improving soil fertility, and promoting sustainable agricultural practices that enhance the productivity and resilience of farming systems. The different scenarios modeled in the study demonstrate the potential for integrating agroforestry techniques and sustainable land-use practices to boost agricultural yields while reducing deforestation pressures. Extension workers can support this transition by providing training on sustainable practices, facilitating access to restoration resources, and guiding farmers toward diversified, resilient farming systems. This approach aligns with the broader FLR goals by restoring ecological integrity and improving local communities’ economic conditions, thereby fostering long-term sustainability in the Tchamba district.
The overarching strategy should focus on ensuring the long-term maintenance and improvement of the welfare of the local population. Addressing the root causes of declining cash income and implementing comprehensive, sustainable measures can create a more resilient and economically viable agricultural sector in the Tchamba district is possible. This approach will enhance the welfare of individual farmers and contribute to the broader goals of economic development and environmental sustainability in the region. This scenario underscores the importance of developing strategies to enhance agricultural productivity and ensure food security while maintaining economic viability. Addressing the decline in net cash income requires interventions to increase crop yields and optimize land use. Such measures might include adopting improved farming technologies, better crop management practices, and diversification of income sources. Implementing these strategies may mitigate the adverse effects of population growth on household income and ensure the long-term sustainability of farming systems in the Tchamba district. In summary, integrating LP analysis with GIS and remote sensing offers a holistic view of the factors driving deforestation, thus enabling more effective management and conservation strategies.
This study establishes a correlation between deforestation, population growth, and the viability of current agricultural systems at the district level in Tchamba, Togo. However, it is important to acknowledge certain limitations. Firstly, while the model offers valuable insights into farming practices and land use, it does not explicitly consider socio-economic factors such as trade dynamics, fluctuations in commodity prices, or the transition to cash crops like soybeans. In Tchamba, soybeans have become a primary cash crop, influencing farmers’ choices to prioritize cash income over household food self-sufficiency. The model does not fully capture this economic dynamic, where farmers opt to sell a significant portion of their harvest and use the proceeds to purchase other foods. Additionally, this analysis relies on self-reported data on crop production, which may introduce biases or inaccuracies. While the approach can be adapted to regions with similar socio-economic and climatic characteristics, caution should be exercised when generalizing these findings. Variations in environmental conditions, cultural factors, and governance structures may impact the model’s applicability, necessitating further research to validate its robustness in different contexts.
We apply mathematical programming, specifically linear programming and welfare maximization theory, to examine, at the district level of Tchamaba, the correlation between deforestation, population growth and the sustainability of the current farming systems. We analyze three scenarios to assess the potential impacts of varying population growth rates and income dynamics on deforestation and the economic sustainability of farming systems. The findings suggest that current farming systems in the Tchamba district can support the present population growth rate of 2.68% per year and per capita income for a maximum of 30–40 years. Given that life expectancy in Togo is 65 years (as per the 2022 population census), these systems cannot sustain even a single human generation. Furthermore, the results indicate that population growth is likely to accelerate deforestation at the current level of socioeconomic development and farming technology. Consequently, it can be concluded that the existing farming systems are not economically sustainable beyond the next 50 years. These findings underscore the need to enhance the economic sustainability of current farming practices. Improving farming technology to increase crop production through improved land productivity is important. Implementing this strategy would meet the increasing food demand resulting from population growth and limit farmlands’ expansion through forest clearing. Integrating these findings into the ongoing FLR program highlights the importance of adopting sustainable agricultural practices that are both economically viable and environmentally friendly. The outcome of this research raises awareness of these challenges among policymakers and practitioners and provides valuable insights for policy formulation and implementation. Moreover, it underscores the need for a comprehensive policy framework that embraces sustainable agricultural practices, community engagement, rigorous enforcement of government regulations, and promotion of economic diversification. By linking sustainable farming systems with FLR initiatives, this research offers actionable strategies to guide restoration efforts, ensuring the resilience and productivity of agricultural systems under the pressures of population growth and income constraints.
The raw data supporting the conclusions of this article will be made available upon reasonable request from the corresponding author S2F0aGFyaW5hLkxvZWhyQHphbGYuZGU=.
A verbal consent for publishing was obtained, and no identifying details of the participants is published.
HM: Conceptualization, Data curation, Investigation, Methodology, Software, Writing – original draft, Writing – review & editing, Formal analysis, Validation, Visualization. KH: Data curation, Investigation, Writing – review & editing, Validation, Visualization. KA: Supervision, Validation, Writing – review & editing, Visualization. KK: Resources, Supervision, Validation, Writing – review & editing, Visualization. SS: Funding acquisition, Project administration, Resources, Supervision, Validation, Visualization, Writing – review & editing. KL: Funding acquisition, Project administration, Resources, Software, Supervision, Validation, Visualization, Writing – review & editing.
The author(s) declare that financial support was received for the research, authorship, and/or publication of this article. The project received financial support from the German Federal Ministry for Economic Cooperation and Development (BMZ), commissioned and administered through the global project on forest landscape restoration and good governance in the forest sector (Forests4Future) of the Deutsche Gesellschaft für Internationale Zusammenarbeit (GIZ) (ref no: 81277142/19.0125.5-101.00). The contents of this publication are the sole responsibility of the authors of this publication and can under no circumstances be regarded as reflecting the position of GIZ/F4F or the Federal Ministry for Economic Cooperation and Development (BMZ).
We thank the Leibniz Centre for Agricultural Landscape Research (ZALF) and the University of Lomé (UL) for logistical support in conducting the research. We express our sincere thanks and appreciation to the experts and farmers who agreed to participate in this study.
The authors declare that the research was conducted in the absence of any commercial or financial relationships that could be construed as a potential conflict of interest.
All claims expressed in this article are solely those of the authors and do not necessarily represent those of their affiliated organizations, or those of the publisher, the editors and the reviewers. Any product that may be evaluated in this article, or claim that may be made by its manufacturer, is not guaranteed or endorsed by the publisher.
The Supplementary material for this article can be found online at: https://www.frontiersin.org/articles/10.3389/fsufs.2024.1466008/full#supplementary-material
Supplementary Table 1 | A summary of the input-output matrix for production activities on a per-hectare basis for an average farmer (per household).
Supplementary Table 2 | Linear programming tableau of the problem in its numerical form.
1. ^1 bag of Maize = 100 kg, 1 bag of soybeans = 100 kg, and 1 calabash of yam = 100 kg.
Alig, R. J., and Plantinga, A. J. (2004). Future forestland area: impacts from population growth and other factors that affect land values. J. For. 102, 19–24. doi: 10.1093/jof/102.8.19
Andrade, A. C., Falcão, L. A. D., Borges, M. A. Z., Leite, M. E., and Espírito Santo, M. M. D. (2024). Are land use and cover changes and socioeconomic factors associated with the occurrence of dengue fever? A case study in Minas Gerais state, Brazil. Resources 13:38. doi: 10.3390/resources13030038
Bassan, K. A., Kokou, K., and Sills, E. (2020a). Constraints to tropical forest conservation and successful monitoring and assessment of land uses cover and change: do the forest definition and administration really matter? Nat. Resour. 11, 1–19. doi: 10.4236/nr.2020.111001
Bassan, K. A., Sills, E. O., and Kokou, K. (2020b). Linear panel data and farming cash flow analyses to assess the causes of deforestation in the upper Guinean Forest: data and evidence from the prefectures of the central region in Togo. Nat. Resour. 11, 71–95. doi: 10.4236/nr.2020.113005
Blakime, T.-H., Adjonou, K., Komi, K., Hlovor, A. K. D., Gbafa, K. S., Zoungrana, J.-B. B., et al. (2024). Dynamics of built-up areas and challenges of planning and development of urban zone of greater Lomé in Togo, West Africa. Land 13:84. doi: 10.3390/land13010084
Borrego, A., and Skutsch, M. (2019). How socio-economic differences between farmers affect Forest degradation in Western Mexico. Forests 10:893. doi: 10.3390/f10100893
Brown, D. S., Brown, J. C., and Brown, C. (2016). Land occupations and deforestation in the Brazilian Amazon. Land Use Policy 54, 331–338. doi: 10.1016/j.landusepol.2016.02.003
Chevalier, A. (1933). Le territoire géo-botanique de l'Afrique tropicale nord-occidentale et ses subdivisions. Bulletin de la Société Botanique de France 80, 4–26. doi: 10.1080/00378941.1933.10833810
CIFOR. (2021). Restoration for resilience. Available at: https://www.cifor-icraf.org/restoration-for-resilience/ (accessed March 23, 2022).
Dury, J., Schaller, N., Garcia, F., Reynaud, A., and Bergez, J. E. (2012). Models to support cropping plan and crop rotation decisions. A review. Agron. Sustain. Dev. 32, 567–580. doi: 10.1007/s13593-011-0037-x
Dykstra, D. P. (1984). Mathematical programming for natural resource management. New York, USA: McGraw-Hill Book Company.
Eshetu, S. B., Kipkulei, H. K., Koepke, J., Kächele, H., Sieber, S., and Löhr, K. (2024). Impact of forest landscape restoration in combating soil erosion in the Lake Abaya catchment, southern Ethiopia. Environ. Monit. Assess. 196:228. doi: 10.1007/s10661-024-12378-8
Folefack, A. J. J., Eboutou, L. Y., Degrande, A., Moulende, T. F., Kamajou, F., and Bauer, S. (2015). Benefits from tree species’ diversification in cocoa agroforests in the Centre region of Cameroon. Russ. J. Agric. Soc. Econ. Sci. 47, 3–13. doi: 10.18551/rjoas.2015-11.01
Gibreel, T. M. (2009). Unfortunately, the location of the publisher is uncertain, but please find relevant information about the reference. Journal: Farming & Rural Systems Economics Vol.105 ISSN 1616-9808. Margraf Publishers.
Groot, J. C., Oomen, G. J., and Rossing, W. A. (2012). Multi-objective optimization and design of farming systems. Agric. Syst. 110, 63–77. doi: 10.1016/j.agsy.2012.03.012
Holden, S., Shiferaw, B., and Pender, J. (2004). Non-farm income, household welfare, and sustainable land management in a less-favoured area in the Ethiopian highlands. Food Policy 29, 369–392. doi: 10.1016/j.foodpol.2004.07.007
Hosonuma, N., Herold, M., De Sy, V., De Fries, R. S., Brockhaus, M., Verchot, L., et al. (2012). An assessment of deforestation and forest degradation drivers in developing countries. Environ. Res. Lett. 7:044009. doi: 10.1088/1748-9326/7/4/044009
Hounkpati, K., Adjonou, K., Moluh Njoya, H., Hlovor, A. K. D., Kipkulei, H. K., Sieber, S., et al. (2024). Strengthening Forest landscape restoration through understanding land use dynamics: case study of Tchamba prefecture (Togo). J. Indian Soc. Remote Sens. 52, 1117–1134. doi: 10.1007/s12524-024-01862-w
ICRAF. (2022). Establishing a soil spectral laboratory in Rwanda. Available at: https://www.worldagroforestry.org/blog/2022/04/07/establishing-soil-spectral-laboratory-rwanda (accessed May 17, 2023).
IFAD. (2021). Why invest in climate change adaptation? Your questions answered. Available at: https://www.ifad.org/en/web/latest/-/why-invest-in-climate-change-adaptation-your-questions-answered?p_l_back_url=%2Fen%2Fweb%2Flatest%2Fgallery (accessed April 24, 2022).
Indarto, J. (2016). An overview of theoretical and empirical studies on deforestation. J. Int. Dev. Coop. 14.
ITRA. (2021). Recommandations pour les principales cultures selon l’état de la fertilité des sols: Préfecture de Tchamba. Available at: www.itra.tg.
Jagoret, P., Ngnogue, H. T., Bouambi, E., Battini, J.-L., and Nyassé, S. (2009). Diversification des exploitations agricoles à base de cacaoyer au Centre Cameroun: mythe ou réalité? Available at: https://popups.uliege.be/1780-4507/index.php (Accessed August 17, 2023).
Kaoneka, A. R. S., and Solberg, B. (1997). Analysis of deforestation and economically sustainable farming systems under pressure of population growth and income constraints at the village level in Tanzania. Agric. Ecosyst. Environ. 62, 59–70. doi: 10.1016/s0167-8809(96)01118-8
Keith, F., Stephen, M., and Jeremy, J. (2024). Global changes in agricultural production, productivity, and resource use over six decades. USDA, Economic Research Service. Available at: https://www.ers.usda.gov/amber-waves/2024/september/global-changes-in-agricultural-production-productivity-and-resource-use-over-six-decades/ (accessed October 28, 2024).
Koglo, Y. S., Agyare, W. A., Diwediga, B., Sogbedji, J. M., Adden, A. K., and Gaiser, T. (2018). Remote sensing-based and participatory analysis of forests, agricultural land dynamics, and potential land conservation measures in Kloto District (Togo, West Africa). Soil Syst. 2:49. doi: 10.3390/soilsystems2030049
Kombate, B., Atakpama, W., Egbelou, H., Ahuide, K., Dourma, M., Folega, F., et al. (2023). Dynamique de l’occupation de sol et modélisation du carbone de la Forêt Communautaire d’Alibi-I. Annales de la Recherche Forestière en Algérie 13, 13–26.
Kombate, B., Dourma, M., Folega, F., Atakpama, W., Wala, K., and Akpagana, K. (2020). Spatio-temporal dynamics and habitat fragmentation within a central region of Togo. Agric. Sci. Res. J. 10, 291–305.
Kombate, A., Folega, F., Atakpama, W., Dourma, M., Wala, K., and Goïta, K. (2022). Characterization of land-cover changes and Forest-cover dynamics in Togo between 1985 and 2020 from Landsat images using Google earth engine. Land 11:1889. doi: 10.3390/land11111889
Kong, R., Diepart, J. C., Castella, J. C., Lestrelin, G., Tivet, F., Belmain, E., et al. (2019). Understanding the drivers of deforestation and agricultural transformations in the northwestern uplands of Cambodia. Appl. Geogr. 102, 84–98. doi: 10.1016/j.apgeog.2018.12.006
Low, A. (1986). Agricultural development in southern Africa: farm household-economics and the food crisis. London, UK: James Currey Publisher.
Mansourian, S., Derkyi, M., Djenontin, I., Elias, M., Oldekop, J., Pacheco, P., et al. (2024). Human dimensions of forest landscape restoration. IUFRO. Available at: https://www.iufro.org/publications/joint-publications/article/2024/04/24/human-dimensions-of-forest-landscape-restoration/ (Accessed April 24, 2024).
Mansourian, S., Parrotta, J., Balaji, P., Bellwood-Howard, I., Bhasme, S., Bixler, R. P., et al. (2020). Putting the pieces together: integration for forest landscape restoration implementation. Land Degrad. Dev. 31, 419–429. doi: 10.1002/ldr.3448
Mapfumo, P., Adjei-Nsiah, S., Mtambanengwe, F., Chikowo, R., and Giller, K. E. (2013). Participatory action research (PAR) as an entry point for supporting climate change adaptation by smallholder farmers in Africa. Environ. Dev. 5, 6–22. doi: 10.1016/j.envdev.2012.11.001
Mousavi, S. R., and Eskandari, H. (2011). A general overview on intercropping and its advantages in sustainable agriculture. J. Appl. Environ. Biol. Sci. 1, 482–486.
Nakajima, C. (2012). Elsevier Science Publishers B.V., Sara Burgerhartstraat 25, P.O. Box 211, 1000 AE Amsterdam. The Netherlands.
Norton, R. D., and Hazell, P. B. (1986). Mathematical programming for economic analysis in agriculture. Biometrics 43:1032. doi: 10.2307/2531573
Nurrochmat, D. R., Suryanto, N., Nurrochmat, N. A., Tarigan, S., Siregar, I. Z., Rizki, D. L. Y., et al. (2023). Indonesia's options in becoming a high-income country: accelerating the turning point in deforestation? Forest Policy Econ. 148:102905. doi: 10.1016/j.forpol.2022.102905
Olfato-Parojinog, A., Sobremonte-Maglipon, P. A., Limbo-Dizon, J. E., Almadrones-Reyes, K. J., and Dagamac, N. H. A. (2023). Land use/land cover changes (LULCC) using remote sensing analyses in Rizal, Philippines. GeoJournal 88, 6105–6118. doi: 10.1007/s10708-023-10959-7
Ouedraogo, I., Tigabu, M., Savadogo, P., Compaoré, H., Odén, P., and Ouadba, J. (2010). Land cover change and its relation with population dynamics in Burkina Faso, West Africa. Land Degrad. Dev. 21, 453–462. doi: 10.1002/ldr.981
Oyetunji, P., Ibitoye, O., Akinyemi, G., Fadele, O., and Oyediji, O. (2020). The effects of population growth on deforestation in Nigeria: 1991–2016. J. Appl. Sci. Environ. Manag. 24, 1329–1334. doi: 10.4314/jasem.v24i8.4
Potapov, P., Turubanova, S., Hansen, M. C., Tyukavina, A., Zalles, V., Khan, A., et al. (2022). Global maps of cropland extent and change show accelerated cropland expansion in the twenty-first century. Nat. Food 3, 19–28. doi: 10.1038/s43016-021-00429-z
Power, J. F., and Follett, R. F. (1987). Monoculture. Sci. Am. 256, 78–86. doi: 10.1038/scientificamerican0387-78
Qasim, M., Hubacek, K., and Termansen, M. (2013). Underlying and proximate driving causes of land use change in district swat, Pakistan. Land Use Policy 34, 146–157. doi: 10.1016/j.landusepol.2013.02.008
Ruben, R., and van Ruijven, A. (2001). Technical coefficients for bio-economic farm household models: a meta-modelling approach with applications for southern Mali. Ecol. Econ. 36, 427–441. doi: 10.1016/S0921-8009(00)00240-8
Sali, G., Monaco, F., Mazzocchi, C., and Corsi, S. (2016). Exploring land use scenarios in metropolitan areas: food balance in a local agricultural system by using a multi-objective optimization model. Agric. Agric. Sci. Procedia 8, 211–221. doi: 10.1016/j.aaspro.2016.02.095
Sankhayan, P., and Cheema, H. (1991). Using linear programming models for generating optimum farm plans-an expository analysis. Indian J. Agric. Econ. 46, 601–612.
Shrestha, S., Shrestha, U. B., and Bawa, K. (2018). Socio-economic factors and management regimes as drivers of tree cover change in Nepal. PeerJ 6:e4855. doi: 10.7717/peerj.4855
Stanturf, J. A., Kant, P., Lillesø, J.-P. B., Mansourian, S., Kleine, M., Graudal, L., et al. (2015). Forest landscape restoration as a key component of climate change mitigation and adaptation, vol. 34. Vienna, Austria: International Union of Forest Research Organizations (IUFRO).
Tully, K., Sullivan, C., Weil, R., and Sanchez, P. (2015). The state of soil degradation in sub-Saharan Africa: baselines, trajectories, and solutions. Sustain. For. 7, 6523–6552. doi: 10.3390/su7066523
Ullah, S. M. A., Tsuchiya, J., Asahiro, K., and Tani, M. (2022). Exploring the socioeconomic drivers of deforestation in Bangladesh: the case of Teknaf wildlife sanctuary and its surrounding community. Trees For. People 7:100167. doi: 10.1016/j.tfp.2021.100167
Xu, J., Gao, J., de Holanda, H. V., Rodríguez, L. F., Caixeta-Filho, J. V., Zhong, R., et al. (2021). Double cropping and cropland expansion boost grain production in Brazil. Nat. Food 2, 264–273. doi: 10.1038/s43016-021-00255-3
Yesuf, G., Brown, K. A., and Walford, N. (2019). Assessing regional-scale variability in deforestation and forest degradation rates in a tropical biodiversity hotspot. Remote Sens. Ecol. Conserv. 5, 346–359. doi: 10.1002/rse2.110
Zabel, F., Delzeit, R., Schneider, J. M., Seppelt, R., Mauser, W., and Václavík, T. (2019). Global impacts of future cropland expansion and intensification on agricultural markets and biodiversity. Nat. Commun. 10:2844. doi: 10.1038/s41467-019-10775-z
Xi represents the number of ha of land allocated to each crop 1 on a given farming system, where i = 1, 2, … 7.
X1 = Maize grown in a monoculture farming system.
X2 = Soybeans grown in a monoculture farming system.
X3 = Yam grown in a monoculture farming system.
X4 = Maize grown in the intercropping farming system.
X5 = Soybeans grown in the intercropping farming system.
X6 = Maize grown in a crop rotation farming system.
X7 = Soybeans grown in crop rotation farming system.
The symbols representing purchase activities are defined as follows:
X8 = NPK fertilizer purchased (kg/year).
X9 = Urea fertilizer purchased (kg/year).
X10 = KCL fertilizer purchased (Kg/year).
X11 = Francs des Colonies Francaise d’Afrique (FCFA) borrowed as working capital per year.
The symbols representing crop sale activity are defined as follows:
X12 = quantity of maize sold by the household (kg/year).
X13 = quantity of soilbean sold by the household (kg/year).
X14 = quantity of yam sold by the household (kg/year).
The symbols representing land clearing activities are defined as follows.
X15 = yam land cleared per year (YL).
X16 = maize and soybeans land cleared per year (MSL).
Thus, the total cleared land per year (TCL) in any given year is represented by:
Keywords: forest landscape restoration, deforestation, farming systems, population growth, economic constraints
Citation: Moluh Njoya H, Hounkpati K, Adjonou K, Kokou K, Sieber S and Löhr K (2024) Socioeconomic analysis of deforestation and economically sustainable farming systems to foster forest landscape restoration in central Togo. Front. Sustain. Food Syst. 8:1466008. doi: 10.3389/fsufs.2024.1466008
Received: 31 July 2024; Accepted: 07 November 2024;
Published: 04 December 2024.
Edited by:
Ademola Braimoh, World Bank Group, United StatesReviewed by:
Arlene López-Sampson, Centro Agronomico Tropical De Investigacion Y Ensenanza Catie, Costa RicaCopyright © 2024 Moluh Njoya, Hounkpati, Adjonou, Kokou, Sieber and Löhr. This is an open-access article distributed under the terms of the Creative Commons Attribution License (CC BY). The use, distribution or reproduction in other forums is permitted, provided the original author(s) and the copyright owner(s) are credited and that the original publication in this journal is cited, in accordance with accepted academic practice. No use, distribution or reproduction is permitted which does not comply with these terms.
*Correspondence: Hamza Moluh Njoya, SGFtemEuTW9sdWgtTmpveWFAemFsZi5kZQ==; Katharina Löhr, S2F0aGFyaW5hLkxvZWhyQHphbGYuZGU=
†ORCID: Hamza Moluh Njoya, orcid.org/0000-0002-5067-2871
Kossi Hounkpati, orcid.org/0000-0003-0212-7331
Kossi Adjonou, orcid.org/0000-0001-8491-8107
Kouami Kokou, orcid.org/0000-0002-2400-0852
Stefan Sieber, orcid.org/0000-0002-4849-7277
Katharina Löhr, orcid.org/0000-0003-2691-9712
Disclaimer: All claims expressed in this article are solely those of the authors and do not necessarily represent those of their affiliated organizations, or those of the publisher, the editors and the reviewers. Any product that may be evaluated in this article or claim that may be made by its manufacturer is not guaranteed or endorsed by the publisher.
Research integrity at Frontiers
Learn more about the work of our research integrity team to safeguard the quality of each article we publish.