- 1Division of Bioinformatics, ICAR-Indian Agricultural Statistics Research Institute, New Delhi, India
- 2Division of Agricultural Economics, ICAR-Indian Agricultural Statistics Research Institute, New Delhi, India
- 3Division of Environment Science, ICAR-Indian Agricultural Statistics Research Institute, New Delhi, India
- 4Rural Economy, Environment and Society, Scotland’s Rural College (SRUC), Edinburgh, United Kingdom
Introduction: There is a global priority to ensure food security, environmental conservation and sustainability. The adoption of inputs such as organic manures, biofertilisers, and biopesticides has been widely promoted as a way to enhance agricultural sustainability, yet their adoption rates remain notably low. The study examines the determinants and impact of the adoption of multiple sustainable inputs by paddy farmers in India. The study also examines the drivers behind the intensity of their adoption and their technical efficiency.
Methods: The study utilises the survey dataset on paddy farmers from “Situation Assessment of Agricultural Households and Land and Livestock Holdings of Households in Rural India” of the 77th National Sample Survey (NSSO) round. The reference period for data collection was the agricultural year from July 2018 to June 2019. The analytical methods employed include the Multivariate Probit and Ordered Probit models, Seemingly Unrelated Regression, Regression Adjustment model, and Stochastic Frontier method.
Results: The empirical findings reveal that the adoption and intensity of sustainable inputs depend significantly on factors such as the household head’s age and education, household size, and access to extension services. Adopting sustainable inputs has significant benefits, such as increased harvest value. However, the currently available technical advisory services are limited in effectively promoting the expenditure on sustainable inputs. Technical efficiency analysis underscores the potential for improvement and the importance of utilising optimal input levels. The research findings provide strong evidence in favour of advocating for the use of sustainable inputs in paddy farming.
1 Introduction
Agrochemicals are essential inputs in Indian agriculture, and the country recognises their contribution to ensuring the food security of its large population (Kishore et al., 2013; Rajna et al., 2023). Fertilisers play a vital role in providing essential nutrients to the soil, improving its fertility, and ultimately boosting crop productivity. They mitigate soil deficiencies and ensure that crops access the necessary nutrients for robust growth (Fageria, 2007). Similarly, pesticides play a crucial role in safeguarding crops against insect pests, thereby preventing substantial yield losses. Through effective pest management, pesticides help sustain high agricultural productivity and meet the escalating food demands of the expanding population (Anani and Adetunji, 2021). The widespread incorporation of these chemical inputs has notably bolstered agricultural productivity in India and has played a crucial role in enhancing the nation’s food security and fostering its economic development (Jafar et al., 2024). Nevertheless, it is vital to prudently regulate the utilisation of these inputs to guarantee their long-term sustainability and reduce adverse environmental effects (Upadhayay et al., 2023).
Historically, India’s agricultural policies, particularly during the Green Revolution, prioritised maximising yields to ensure food security (Goyal et al., 2024). This approach, while successful in boosting production, led to the heavy use of chemical fertilisers and pesticides, resulting in widespread environmental degradation (Paul et al., 2023). Over time, the negative consequences of this productivist paradigm became increasingly apparent, with issues like soil degradation, water pollution, and biodiversity loss coming to the forefront (Maddela et al., 2022). This realisation has spurred a significant policy shift in recent decades, with a growing emphasis on integrating agricultural and environmental policies to promote sustainable agricultural practices (Velayudhan et al., 2021). The majority of policies implemented in the line of the green revolution, which was heavily skewed towards achieving higher production through enhanced use of chemicals, are now being re-assessed (Pingali, 2012; Pathak, 2023). Contemporary policies place equal emphasis on ensuring environmental sustainability and responsibly balancing nutrient application as they do on maximising food production (Velayudhan et al., 2024). Policies like the National Program for Organic Production aim to promote sustainable agricultural practices through the advancement of organic farming. This program provides certification and financial support to encourage farmers to embrace organic methods.
Moreover, the government has introduced incentives to promote the use of eco-friendly biofertilisers and biopesticides as sustainable alternatives to chemical inputs. Subsidies are also available to incentivise the widespread adoption of bio-based inputs in agriculture (Velayudhan et al., 2021). Furthermore, programs to educate and train farmers on sustainable agricultural practices, including balanced nutrient application and integrated pest management, are also in place. Regulations to ensure responsible and sustainable use of agrochemicals, promoting environmental sustainability while maintaining food security, are also expected to benefit in future (Praveen, 2017; Praveen and Alka, 2019). For instance, the National Mission for Sustainable Agriculture (NMSA), launched in 2014–15, aims to enhance resource use efficiency, conserve natural resources, and mitigate climate change impacts. Since 2023, the government of India has also provided market development assistance of Indian Rupees 1,500 per metric tonne of organic manures, under the scheme Galvanizing Organic Bio-Agro Resources Dhan (GOBARdhan). The PM Programme for Restoration, Awareness Generation, Nourishment, and Amelioration of Mother Earth (PM-PRANAM) is another innovative program launched by the Government of India in 2023. It aims to incentivise states that actively contribute to the balanced use of chemical fertilisers in agriculture. The plan involves reducing the usage of chemical fertilisers, and the subsidy saved will be granted to the respective states to promote sustainable inputs. This unique approach aims to encourage regions to take concrete steps towards sustainable agriculture and environmental preservation. Other policies like Nutrient Based Subsidy, One Nation One Fertiliser, PoS-enabled fertiliser sales as part of Direct Benefit Transfer for fertiliser subsidy distribution, etc., are all intended to reduce the excessive use of chemical fertilisers. Similarly, policies promoting organic farming, such as the Paramparagat Krishi Vikas Yojana, reflect a clear shift towards environmentally sustainable agriculture in India (Fertiliser Association of India, 2023).
Indian agriculture is currently at a crucial crossroads, demanding strategic planning to simultaneously uphold food security and ensure environmental sustainability for the future (Praveen and Aditya, 2022). In response to these sustainability concerns, policymakers and development agencies have called for initiatives focused on creating, distributing, and adopting sustainable agricultural inputs (Sharma et al., 2021). Although the adoption of Sustainable Agricultural Practices has been extensively associated with enhanced productivity, welfare, and food security globally (Bezu et al., 2014), adoption rates remain generally low in several countries (Kagoya et al., 2018). A promising development in the Indian agricultural sector is the growing consensus among key stakeholders, including the government, industry, and scientific community, regarding the significant benefits of utilising a diverse range of fertilisers-including inorganic, organic, and biofertilisers—for optimising agricultural productivity (Velayudhan et al., 2023). However, there are numerous challenges to deal with.
Technology adoption is a complex process influenced by multiple factors (Passarelli et al., 2023). It is determined by the adopter’s attitude, including their beliefs and motivation to adopt. If a farmer sees value in adopting technology, there is a higher chance of adoption. However, there are complex dimensions to adoption, including the new paradigm for environmentally friendly and socially acceptable agricultural practices (Akanmu et al., 2023). In the realm of agricultural production, there exists a risks, encompassing both idiosyncratic factors unique to individual farms and covariate risks affecting multiple farms simultaneously. In response to these challenges, farm households find themselves compelled to incorporate a diverse array of sustainable inputs in their operations. Examples of such sustainable inputs include biofertilisers, biopesticides, and organic manures. The decision-making process involved in the adoption of these sustainable inputs is intricately linked to the specific characteristics of each farm household, taking into consideration the various risk profiles associated with their multiple cropping systems (Pretty and Bharucha, 2014). The adoption of one sustainable input may rely on the utilisation of another. Consequently, it is essential to consider the interdependence of sustainable inputs in multiple adoptions to ensure accurate assessment and avoid underestimation or overestimation of factors influencing their adoption (Wu and Babcock, 1998). The significance of understanding farmers’ adoption behavior has been emphasised in the literature, particularly in shaping policies to encourage their adoption patterns effectively (Asprooth et al., 2023; Rizzo et al., 2024). These studies have delved into a wide range of socio-economic, institutional, environmental, and climatic factors that influence the adoption of sustainable inputs in diverse contexts (Nguyen et al., 2023). One key finding from these studies is the existence of diversity in adoption factors, suggesting that the determinants of adoption differ based on household attributes, the specific technology, and geographical locations (Oyetunde-Usman et al., 2021).
Advancements in technology play a vital role in shaping the expansion of economic output within the agricultural sector (Zhao et al., 2022). The integration of technology has proven to enhance productivity and efficiency in agricultural practices. Specifically, the concept of technical efficiency is of paramount importance to better agricultural performance. Technical efficiency guarantees that a maximum yield is achieved from a given level of input use, thereby optimising agricultural outputs. This efficiency is pivotal in driving agricultural productivity and ensuring sustainable production (Sampaio Morais et al., 2021). In response, globally, there is a strong emphasis on improving the efficiency of agricultural output. This entails the effective conversion of inputs into outputs while minimising wastage. By doing so, the aim is to increase overall productivity and reduce resource consumption. A critical initial step in realising this objective is to assess the current level of technical efficiency quantitatively. This assessment allows for the identification of production losses attributed to inefficiencies in the production process, thus providing valuable insights for improvement (Wang et al., 2012). Furthermore, when using sustainable inputs in combination with their chemical counterparts, testing technical efficiency becomes even more crucial. Such assessments offer key indications of the potential for productivity enhancement and provide insights into cost-effective means of achieving it. Therefore, scrutinising technical efficiency is essential for guiding sustainable agricultural practices and ensuring optimal resource utilisation.
Against this backdrop, we utilize the survey dataset obtained from the “Situation Assessment of Agricultural Households and Land and Livestock Holdings of Households in Rural India” of the 77th National Sample Survey (NSSO) round to examine the determinants influencing the uptake of various sustainable inputs in agricultural practices. Alongside this, we investigate the driving forces behind the intensity of their adoption and the technical efficiency in paddy cultivation. To achieve this, we employ a range of analytical methods, including Multivariate Probit and Ordered Probit models, Seemingly Unrelated Regression, Regression adjustment model, and the Stochastic Frontier Technical Efficiency Model.
Our research makes a significant contribution to the existing literature in several key ways. Firstly, it seeks to explicitly evaluate the factors that influence the adoption of multiple sustainable agricultural inputs in India, taking into account the interactions among diverse socio-economic and farm factors. Secondly, our study utilises extensive and nationally representative household data to identify the wide range of sustainable input choices adopted by households, recognising the interconnected nature of various sustainable inputs. This provides valuable insights essential for developing effective policies and strategies to promote agricultural innovations. We also recognise that not all farm households can adopt all available sustainable inputs due to variations in farming and livelihood contexts, and our study acknowledges that farm households combine sustainable inputs in varying numbers. Importantly, we study the intensity of the use of sustainable inputs (expenditure on sustainable inputs), which is poorly covered in adoption literature. Further, the relationship between factors and technical efficiency is another aspect of this study that fills the gap in the literature. Specifically, our research aims to answer the following questions:
(1) What factors influence the adoption of multiple sustainable inputs in paddy cultivation at the farm household level?
(2) What are the economic implications of using sustainable inputs in rice farming?
(3) What is the current level of technical efficiency in paddy cultivation?
2 Literature review
The adoption of sustainable input usage in agriculture is crucial for ensuring long-term food security, environmental sustainability, and the well-being of farmers (Kassie et al., 2013). Numerous research studies have delved into the adoption and impact of sustainable input usage, with a specific focus on organic fertilisers (Sulaiman and Misnan, 2022). These studies have consistently highlighted the positive outcomes associated with integrating sustainable inputs in agriculture, including reduced reliance on chemical inputs, enhanced environmental and soil health, promotion of biodiversity, and improved farmer livelihoods (Dittmer et al., 2023). However, the adoption of sustainable input usage in agriculture is influenced by various factors. The economic viability and profitability of sustainable inputs significantly impact adoption. Farmers are more likely to adopt sustainable practices if they perceive them to be economically advantageous and if they can provide immediate benefits to address their urgent livelihood needs (Piñeiro et al., 2020). Moreover, availability and accessibility can significantly influence the adoption.
Access to education can equip farm families with knowledge about modern farming methods and provide them with the ability to adapt quickly. However, research on joint adoption of these methods has shown differing effects on decision-making, indicating different perspectives (Ndiritu et al., 2014). Sustainable practices are seen as requiring a lot of work in adoption studies. The size of the family affects the availability of labour and, therefore, influences the decisions on adoption (Voss et al., 2024). Some studies suggest that larger farm families are more likely to invest in labour-intensive sustainable practices (Rust et al., 2020). The wealth of a family influences its ability to buy modern varieties and hire labour for farming activities. The significance of farming innovations in affecting livelihoods has been shown by using wealth as an indicator. However, variation in wealth may lead to unequal adoption of Sustainable Agricultural Practices (Onumah et al., 2023). Evidence also points towards the significant influence of land ownership on decisions related to agricultural innovations, as the lack of land can deter farmers from investing due to the risk of eviction.
Farm households’ adoption decisions may conflict with or complement each other, depending on their perceived benefits. Research has shown diverse patterns in joint adoption decisions, with factors such as innovative agricultural techniques and geographic location playing a significant role (Zeweld et al., 2020). Gender also plays a crucial role in the adoption of Sustainable Agricultural Practices within conservation packages (Rola-Rubzen et al., 2020). Variations have been observed in gender-specific adoption decisions within different categories of practices. For example, female plot managers were found to be less likely to adopt specific yield-enhancing and soil-restoring strategies compared to male counterparts. However, no differences were observed in adopting yield-protecting strategies such as manure and compost. Additionally, differences were noted when considering specific groups, such as female plot managers who are household heads versus wives in male-headed households. Similarly, better technology adoption rates were demonstrated among female farmers living in male-headed households compared to those living in female-headed households (Voss et al., 2024).
One critical factor influencing the adoption of sustainable practices is the availability of information and knowledge. Extension agents play a vital role in disseminating information and providing training to farmers on the benefits and implementation of sustainable input use (Oyetunde-Usman et al., 2021). In addition, extension institutions are vital in promoting the adoption of modern farming practices and conservation techniques, although their impact can vary (Jena, 2022). While extension services aim to bridge the information gap, several challenges may hinder their effectiveness, such as farmers’ limited access to complementary inputs, information failures, and the need for tailored learning processes to suit diverse farmer contexts (Abdulai, 2022). Farmers rely on a combination of formal and informal channels to gather knowledge and make informed decisions about their farming practices (Gaddam et al., 2022). Traditional information sources, such as fellow farmers, family members, and local knowledge networks, continue to hold considerable sway. These informal channels often provide context-specific advice and practical insights based on shared experiences (Naveed and Hassan, 2021). However, their effectiveness can be limited by the potential for biases and the slow dissemination of new information (Zhang et al., 2020). Recognising the limitations of traditional channels, government extension services and agricultural universities play a crucial role in disseminating scientifically validated information on sustainable inputs (Lamm et al., 2023). These formal channels often conduct training programs, field demonstrations, and farmer-scientist interactions to bridge the knowledge gap (van den Berg et al., 2020). Finally, the increasing penetration of information and communication technologies (ICTs) in rural India presents new opportunities for disseminating information on sustainable agriculture. Furthermore, the influence of private sector actors, such as input suppliers and agribusiness companies, on information dissemination is growing. While they can play a positive role in promoting sustainable inputs, it’s crucial to ensure transparency and avoid potential conflicts of interest that could prioritise profit over sustainability goals (Stuart, 2021).
Both formal institutions and informal farmer organisations play crucial yet distinct roles in promoting the adoption of sustainable agricultural inputs in India. Government support and incentives, such as subsidies or grants, can contribute to the adoption of sustainable input use by alleviating the financial burden on farmers (Zheng et al., 2021). Formal institutions, including government agencies, research institutions, and agricultural universities, primarily function as sources of knowledge and technical expertise (Prusty et al., 2022). They develop and disseminate scientifically validated information on sustainable practices, conduct training programs, and often provide access to improved inputs (Nagar et al., 2021). On the other hand, informal farmer organisations, such as self-help groups, farmer collectives, and local cooperatives, play a critical role in fostering trust, facilitating social learning, and enabling collective action (Ullaguari et al., 2023). These groups provide a platform for farmers to share experiences, learn from each other’s successes and failures, and reduce the perceived risks associated with adopting new practices. The social capital embedded within these networks can be particularly valuable in promoting behavioral change and encouraging the adoption of sustainable inputs (Isensee et al., 2021). Furthermore, the strength of local institutions and their ability to provide farmers with access to inputs, market outlets, and information can facilitate the adoption of sustainable input use in agriculture (Velayudhan et al., 2021).
Production shocks are also linked to the uptake of agricultural innovations, with different types of shocks interacting differently with specific farming practices (Sargani et al., 2023). Additionally, climatic factors such as temperature and rainfall can influence the adoption of sustainable agricultural practices (Mgomezulu et al., 2023). In short, the adoption of sustainable input use in agriculture not only contributes to long-term environmental sustainability and food security but also has positive economic implications for farmers, including cost savings, market access, and income generation. As more farmers embrace sustainable input use, the agricultural sector stands to benefit from improved productivity, profitability, and resilience. Finally, a multi-pronged approach that leverages the strengths of both formal and informal information sources, while taking advantage of the potential of ICTs, is essential for promoting the widespread adoption of sustainable agricultural inputs in India.
Reaching higher levels of adoption and intensity in the use of sustainable inputs not only benefits the farmers but also helps the nation achieve the targets of sustainability, including the Sustainable Development Goals (SDGs) (Pathak, 2023). For instance, by enhancing soil health and resource use efficiency, these inputs contribute to increased agricultural productivity and resilience, ultimately supporting SDG 2: Zero Hunger by improving food security and nutrition. Furthermore, reducing reliance on chemical fertilisers and pesticides minimises water contamination risks, aligning with SDG 6: Clean Water and Sanitation by protecting water resources and promoting sustainable water management. Sustainable agricultural practices also promote resource efficiency, waste reduction, and the adoption of environmentally friendly inputs, contributing to SDG 12: Responsible Consumption and Production by transitioning to a more sustainable and circular economy. Additionally, these practices often have a lower carbon footprint compared to conventional alternatives, supporting SDG 13: Climate Action through climate change mitigation and adaptation efforts (Hellin and Fisher, 2019). By minimising soil degradation, reducing pollution, and conserving biodiversity, sustainable agriculture also helps protect and restore terrestrial ecosystems, aligning with SDG 15: Life on Land (Kamau et al., 2022). Furthermore, the adoption of sustainable inputs can have positive ripple effects on other SDGs, such as promoting decent work and economic growth by creating new opportunities in sustainable agriculture and enhancing farmer livelihoods (Piñeiro et al., 2020). Ultimately, embracing sustainable inputs in agriculture is not just an environmental imperative but a crucial step towards a more just and sustainable future for all.
3 Methodology
3.1 Data
The study utilises the survey dataset from “Situation Assessment of Agricultural Households and Land and Livestock Holdings of Households in Rural India” of the 77th National Sample Survey (NSSO) round. This comprehensive country-wide dataset covers information from 5,940 villages covering 45,714 agricultural households. The reference period for data collection was the agricultural year from July 2018 to June 2019. After providing some general insights on the sustainable inputs in all the crops of the country, we specifically perform analysis using data from farmers cultivating paddy in the Kharif (monsoon) season. Hence, the data used for all the analyses on sustainable input use in paddy comprises information from 14,313 farmers.
3.2 Analytical techniques
3.2.1 Adoption and impact of multiple sustainable inputs
3.2.1.1 Multivariate probit model
Farmers in our sample have reported using three different types of sustainable inputs to meet the nutritional needs of their crops. The decision-making process regarding these inputs can be analysed using either multinomial or multivariate analysis. While multinomial models assume independence of irrelevant alternatives, it is important to note that the choices among these inputs are not mutually exclusive due to simultaneous adoption by farmers. Therefore, random error components may be correlated. Using a multivariate model would account for potential contemporaneous correlations arising from the use of all three sustainable inputs. This approach allows for increased efficiency in estimation when facing simultaneous adoption decisions. Unlike other binary models, the multivariate model takes into consideration unobservable factors that may affect farmers’ adoption decisions by allowing for correlation across the error terms of latent equations.
In examining the factors influencing the adoption of multiple sustainable inputs, we operate under the assumption of the interdependence of different sustainable inputs, indicating that the decision to adopt these is inherently multivariate. Drawing from the approach employed by Teklewold et al. (2013), we utilised a Multivariate Probit Model (MVP) to assess the factors influencing the adoption of multiple sustainable inputs at the farm household level. The null hypothesis posits that there is no significant disparity in the characteristics of farmers and their adoption of sustainable inputs, suggesting that regardless of age, farm size, or education level, farmers will adopt a given type of sustainable inputs.
The empirical model is as follows:
Where,
i = farmer id,
= 1, if farmer adopts biofertilisers (0 otherwise),
= 1, if farmer adopts biopesticides (0 otherwise),
= 1, if farmer adopts manures (0 otherwise),
= Vector of determinants of adoption,
= Vector of unknown parameters (j = 1,2,3), and
= Error term.
The hypothesis can be examined by conducting three distinct independent binary probit models, assuming that the error terms are mutually exclusive. However, since the decision to utilise different sources may be correlated, the error term components could exhibit stochastic dependence. In such cases, a multivariate probit model of the following type is employed to test the hypothesis.
Where,
(j = 1,2,3) indicate three different choices of sustainable inputs for the ith farmer (i = 1, 2,…,14,313),
is a 1 × k vector of observed factors that determine the adoption,
is k × 1 vector of unknown parameters, and.
is the unobserved error term.
Assuming that the errors (across j = 1, …, m alternatives) follow a multivariate normal distribution with a mean vector of zero, the unknown parameters in the above equation are estimated using simulated maximum likelihood. The technique applies the Geweke-Hajivassiliour-Keane smooth recursive conditioning simulator procedure to assess the multivariate normal distribution. We conduct the model estimation using STATA software version 15.
3.2.1.2 Ordered probit model
The MVP model suggests that before adopting sustainable inputs, farm households consider the overall benefit of adoption versus non-adoption. If they perceive that the benefits of adoption outweigh non-adoption, they will choose to adopt new sustainable inputs. Farm households are more likely to adopt additional sustainable inputs if they have had positive experiences with previous adoptions. Although the MVP model has limitations in gauging adoption intensity, our focus was on assessing the level of adoption by looking at the number of sustainable inputs taken up at the household level. While a Poisson count distribution model is commonly used for this purpose, it does not align with our assumption about interdependence among sustainable inputs and is, therefore, not suitable for our context. Due to limitations in data on relevant variables, we treated our dependent variable as an ordinal one indicating ordered outcomes—such as households adopting one or two or more sustainable inputs—and used an ordered probit model instead.
We sequentially model our ordered outcomes as a latent variable y* similar to the approach by Oyetunde-Usman et al. (2021). This represents an unobserved measure of households’ adoption of sustainable inputs in numbers, and is defined as follows:
In the case of the jth farm household, when normalisation dictates that the predictors x do not contain an intercept, there is minimal adoption of sustainable inputs for low y*. However, as y* surpasses 1, the number of sustainable inputs adopted increases, and for values greater than 2, adoption continues to increase further. Given m categories in accordance with a standard ordered probability model, the likelihood of observing outcome i aligns with the following:
where is assumed to follow a standard normal cumulative distribution function. The coefficients β1….βk are collectively estimated with the cutpoints α1, α2…, αk-1; where k represents the number of potential outcomes.
3.2.1.3 Seemingly unrelated regression model
Further, we employ the Seemingly Unrelated Regression (SUR) model to analyse the determinants of farmers’ expenditure on sustainable and chemical inputs. The SUR model is particularly useful due to the likely correlation between the error terms of the equations modeling expenditures on these inputs. By allowing for such correlations, this model provides more efficient estimators compared to estimating each equation separately. The model is chosen because of the potential interdependence between expenditures on sustainable and chemical inputs; a farmer’s decision to invest in sustainable practices might be influenced by their current use of chemical inputs and vice versa. Ignoring this interdependence could lead to biased estimates and misleading conclusions. Therefore, the SUR approach provides a comprehensive understanding of the factors influencing these expenditures. The parameters of the SUR model are estimated using Generalized Least Squares, which accounts for the correlation between error terms across equations, improving the efficiency of parameter estimates. The model can be specified as follows:
Where.
i = farmer id,
= expenditure on chemical inputs,
= expenditure on sustainable inputs.
= Vector of determinants of adoption,
= Vector of unknown parameters to be estimated (j = 1,2), and
= Error term.
3.2.1.4 Regression adjustment model
To evaluate the effect of using sustainable inputs on harvest value, we used a regression adjustment model. This model involves fitting separate regressions for the treated and control units and estimating the partial regression coefficients for all control variables included in the model (dependent variable – outcome variable such as income). Next, the model calculates the Potential Mean Outcomes (PMO), which represents the average value of the outcome if all the units in the sample are either in the treated or control group. The model first calculates the expected value of the dependent variable for the entire sample based on the coefficients of regression estimated on treated units. The mean of these expected values is termed as the PMO of the treated group. Similarly, the expected value of the dependent variable for the entire sample based on the coefficients of regression estimated on control units is used to estimate the PMO for control units. The difference between the PMO of the treated and control groups is considered as the estimate of the impact.
3.2.2 Estimation of technical efficiency
The concept of technical efficiency, initially introduced by Michael Farrell in the mid-19th century, pertains to an individual’s proficiency in functioning at the frontier isoquant (Farrell, 1957). This fundamental concept holds significant relevance in the spheres of economics and production theory as it governs the evaluation of resource deployment to optimise output. At its core, technical efficiency signifies the capacity of an economic entity, whether it be a farm, an industry, or a firm, to attain the utmost level of output conceivable while operating within a predetermined set of inputs and the prevailing technological capacities. Technical efficiency inherently involves the ability to generate the greatest possible output from a given set of inputs through the optimal utilisation of technology and resources. This notion indicates the extent to which a production process operates at the edge of its potential production range, devoid of inefficiency or waste.
Economists typically differentiate technical efficiency from allocative efficiency, the former pertaining to the productive aspect of resource utilisation and the latter concerning the optimal distribution of resources among alternative uses. While technical efficiency refers to the attainment of maximal output with current technology, allocative efficiency denotes the alignment of the marginal value product with the marginal factor cost. Organisations strive to optimise output following neoclassical principles by reducing input costs and seeking a technically effective allocation, emphasising the production of a greater quantity of output for the same quantity of inputs (Koopmans, 1951).
The foundation of technical efficiency lies in neoclassical economics and production theory, emphasising organisational endeavors to optimise financial gains through cost reduction and output enhancement within the constraints imposed by existing technology. The application of this conceptual framework has led to the development of numerous theoretical models elucidating the factors that influence technical efficiency, encompassing institutional factors, environmental circumstances, and firm-specific variables. Quantification of technical efficiency involves the assessment of the disparity between actual and potential output relative to production inputs. Various methodologies are available for estimating technical efficiency, each offering distinct perspectives and practical applications. Widely employed approaches include Data Envelopment Analysis (DEA) and Stochastic Frontier Analysis (SFA), both of which hold distinct advantages and applications within the realm of technical efficiency estimation.
3.2.2.1 Stochastic frontier method for technical efficiency
Aigner et al. (1977) and Meeusen and van den Broeck (1977) introduced methods for evaluating the stochastic frontier production function. Two separate investigations found that random factors play a crucial role in determining output variability. In general, the SFM method is described as follows:
Let us assume that a farm has a production function denoted by f = (X, β). The farm, denoted as ith, would generate a yield, Yi, as a function of the variables Xi and β, i.e., Yi = f (Xi, β), assuming no errors or inefficiencies. The stochastic production frontier model suggests that each farm may produce less than its maximum capacity due to varying levels of inefficiency.
More precisely,
Where,
Yi = output.
Xi = input vector of farm i.
βi = vector of the estimated parameters.
ξi = the degree of efficiency of farm i.
In this study, f = (X, β) is assumed as a Cobb–Douglas production function for assessing the concept of returns to scale. Furthermore, it is postulated that the output is susceptible to random error denoted as vi. This implies that
where the variable vi is assumed to be independently and identically N (0; σ2v). The expression for the natural logarithm of the production function is given as
Let us suppose that there are k inputs and that the production function is linear when taking logarithms. We define the technical inefficiency effect ui as the negative natural logarithm of ξi which is considered to be independently exponentially distributed σ2u. With this assumption, the production frontier function can be expressed as
The technical inefficiency effect can be stated as:
The variable ωij represents the stochastic noise, while refers to the exogenous factors that influence rice production. The parameter estimates α indicate the relationship between these exogenous factors and the technical efficiency of rice production. A negative value of αj suggests a positive relationship between the exogenous factors and the technical efficiency, and vice versa. Technical efficiency (TE) in an output-oriented ith farm is quantified as ξi = exp.(−ui), where it represents the ratio between the observed output and the frontier output. The value of ξ must be within the range of (0,1). If ξi equals 1, the farm is deemed to be operating at the optimal output level using the technology represented by the production frontier.
4 Results and discussion
4.1 Comparison of farmers’ expenditure on chemical inputs and sustainable inputs
The data presented in Figure 1 provides a visual representation of the average expenditure by Indian farmers on both chemical and sustainable inputs. It is apparent from Figure 1 that farmers allocate a significantly higher amount of their budget to chemical inputs compared to sustainable alternatives. Specifically, farmers spend approximately 7 rupees on chemical inputs for every rupee spent on sustainable inputs. This expenditure ratio is particularly pronounced in the case of gram (7.9:1), followed by cotton (6.2:1), maize (6.1:1), and paddy (5.7,1). Moreover, when analysing the regional disparities in spending on sustainable inputs (Figure 2), it becomes evident that different regions exhibit varying patterns of expenditure. Additionally, a comparison of Figures 2, 3 suggests that southern states tend to allocate a greater portion of their resources to sustainable inputs compared to their counterparts in the northern states. In relation to specific sustainable inputs under consideration, it is noteworthy that farmers allocate the highest portion of their budget to manures (Rs 639/ha), followed by biofertilisers (Rs 347/ha) and biopesticides (Rs 217/ha). On the contrary, their spending on chemical fertilisers and chemical pesticides amounts to Rs 5,431/ha and Rs 1,368/ha, respectively (Figure 4).
4.2 Analysis of paddy farmers’ adoption and expenditure on sustainable inputs and chemical inputs
4.2.1 Description and summary statistics of variables
Table 1 provides a summary of the variables used in this study, including their descriptions and statistics. Age is a crucial factor in adoption studies as it reflects the level of farming experience. Our findings show that the average age of the household head is around 50 years, indicating that, on average, farm households are still actively engaged in farming. Only a small percentage (9%) of households are led by females, which may indicate fewer female roles in the farming population. This observation, however, does not take into account the influence of spouses in male-headed households, who may act as plot managers and play a role in adoption decisions. On the other hand, the average household size is approximately 5, indicating a moderate family setting. Large family sizes were previously common in agricultural settings in developing countries, as they allowed for the potential utilisation of family labor. However, in recent years, farm sizes have declined due to various factors. Additionally, household size is a factor to be considered in adoption decisions, depending on the type of sustainable inputs.
Further analysis of household statistics reveals that the education level of the household heads is relatively low, with an average of 3.3 years of education. This suggests that while the majority of household heads are educated, their education level is low, which could potentially affect their understanding of farm practices and their uptake of technological information. Additionally, 95 percent of the farmers operate their land individually, which could have a positive or negative effect on the adoption of sustainable inputs. Furthermore, 76 percent of the farmers own the entire land they operate. Significant differences were observed in the means of several variables between the treated (farmers adopting sustainable inputs) and control group (farmers not adopting sustainable inputs) (see Table 2).
4.2.2 Multivariate probit estimates of the determinants of adoption of multiple sustainable inputs
In order to investigate the main research question, which involves examining the factors that affect the adoption of various sustainable inputs at the farm household level, we employed the Multivariate probit model. The results of this model are presented in Table 3. The analysis indicates that the log-likelihood ratio (LR) of the model is −7093.24, and the Wald chi-square (57) value is 271.5, both of which are significant at a level of p < 0.01. This suggests that the model fits the data well. The significance of the LR test further implies that the decisions to adopt multiple sustainable inputs are interdependent. The adoption of biofertilisers was found to be significantly and positively affected by factors such as age, farm size, and membership in farm organisations while being negatively influenced by social groups. This indicates that farmers from disadvantaged societal segments have limited access to such inputs. Moreover, education was found to have a positive influence on the adoption of biofertilisers and biopesticides, but no influence on manure adoption. Access to technical advisory, on the other hand, was observed to influence biopesticide and manure adoption but had no significant effect on biofertiliser adoption.
4.2.3 Ordered probit estimates of the determinants of the number of sustainable inputs adopted
The results presented in Table 4 provide a comprehensive overview of the findings obtained from the ordered probit model, which aimed to explore the various factors influencing the adoption of sustainable inputs by agricultural households. Upon analysis, the chi-squared statistics derived from the ordered probit model were found to be highly statistically significant (chi square (19) = 111.96, Prob > chi-square = 0.000), indicating a strong fit of the model to the data. This suggests that the model effectively captures the complexity of the factors influencing the adoption of sustainable inputs. Furthermore, the results indicated that the number of sustainable inputs adopted exhibited a positive association with the age of the household head, implying that as the age of the household head increases, the propensity to adopt sustainable inputs also increases. Conversely, there was an inverse relationship observed between the number of sustainable inputs adopted and the household size, signaling that larger households tend to adopt fewer sustainable inputs. Additionally, the study revealed that households from the disadvantaged segments of society tended to adopt a lower number of sustainable inputs, highlighting a potential disparity in the adoption of sustainable agricultural practices. On the other hand, farmers affiliated with farm organisations displayed a higher propensity to adopt a greater number of sustainable inputs, reflecting the positive influence of organisational membership on sustainable input adoption. Furthermore, the awareness of minimum support price (MSP) and accessibility to technical advisory services emerged as significant factors contributing to the increased adoption of sustainable inputs, underscoring the importance of information dissemination and technical support in promoting sustainable agricultural practices.
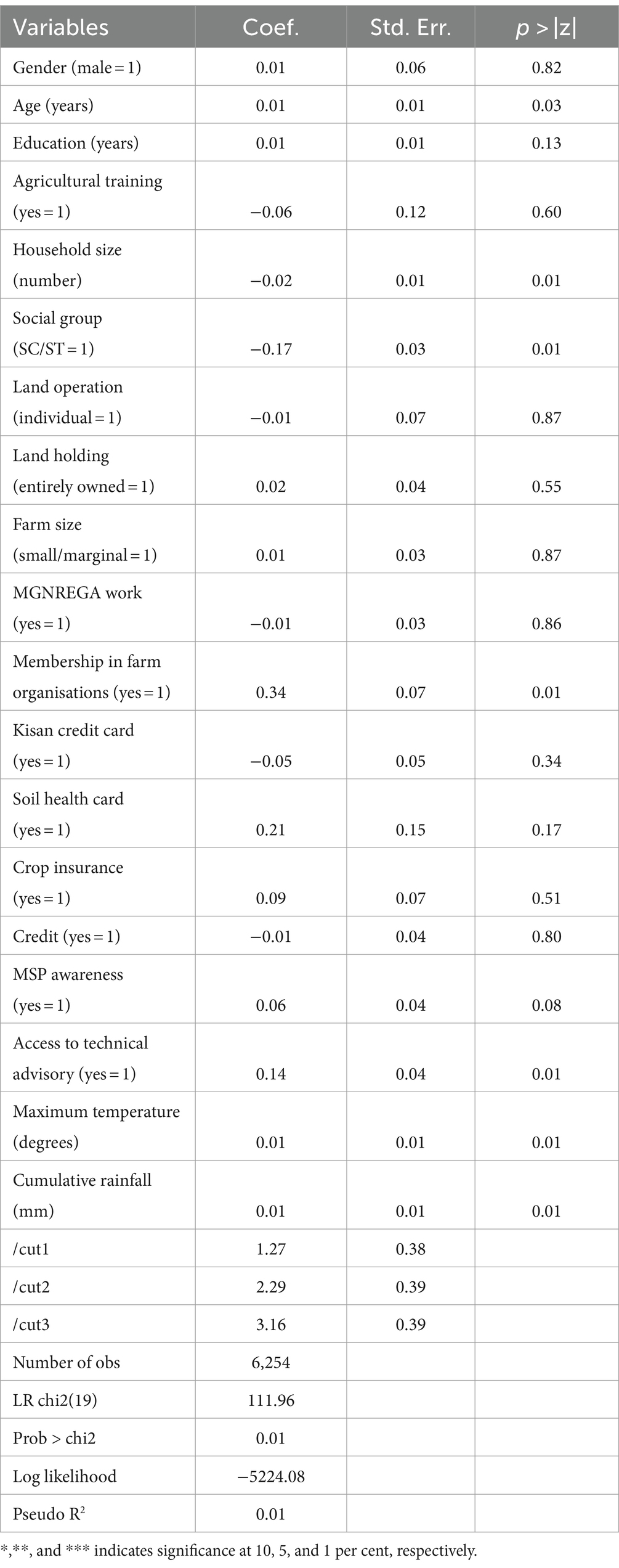
Table 4. Ordered probit estimates of the factors influencing the number of sustainable inputs adopted.
4.2.4 Determinants of expenditure on chemical and sustainable inputs
Further, we estimated the determinants of chemical and sustainable inputs in paddy cultivation through the use of a seemingly unrelated regression model (see Table 5). From the analysis, it was found that age has a positive influence on the expenditure for both chemical and sustainable inputs. Additionally, agricultural training was found to have a positive impact on the expenditure for chemical inputs but no noticeable influence on sustainable inputs expenditure. It was also observed that farmers who are not from disadvantaged backgrounds and larger farmers tend to spend more on both types of inputs. Furthermore, membership in a farm organisation was identified as a crucial determinant of expenditure on sustainable inputs. An important finding was that, despite technical advisories promoting spending on chemical inputs, there was no discernible influence on the expenditure for sustainable inputs.
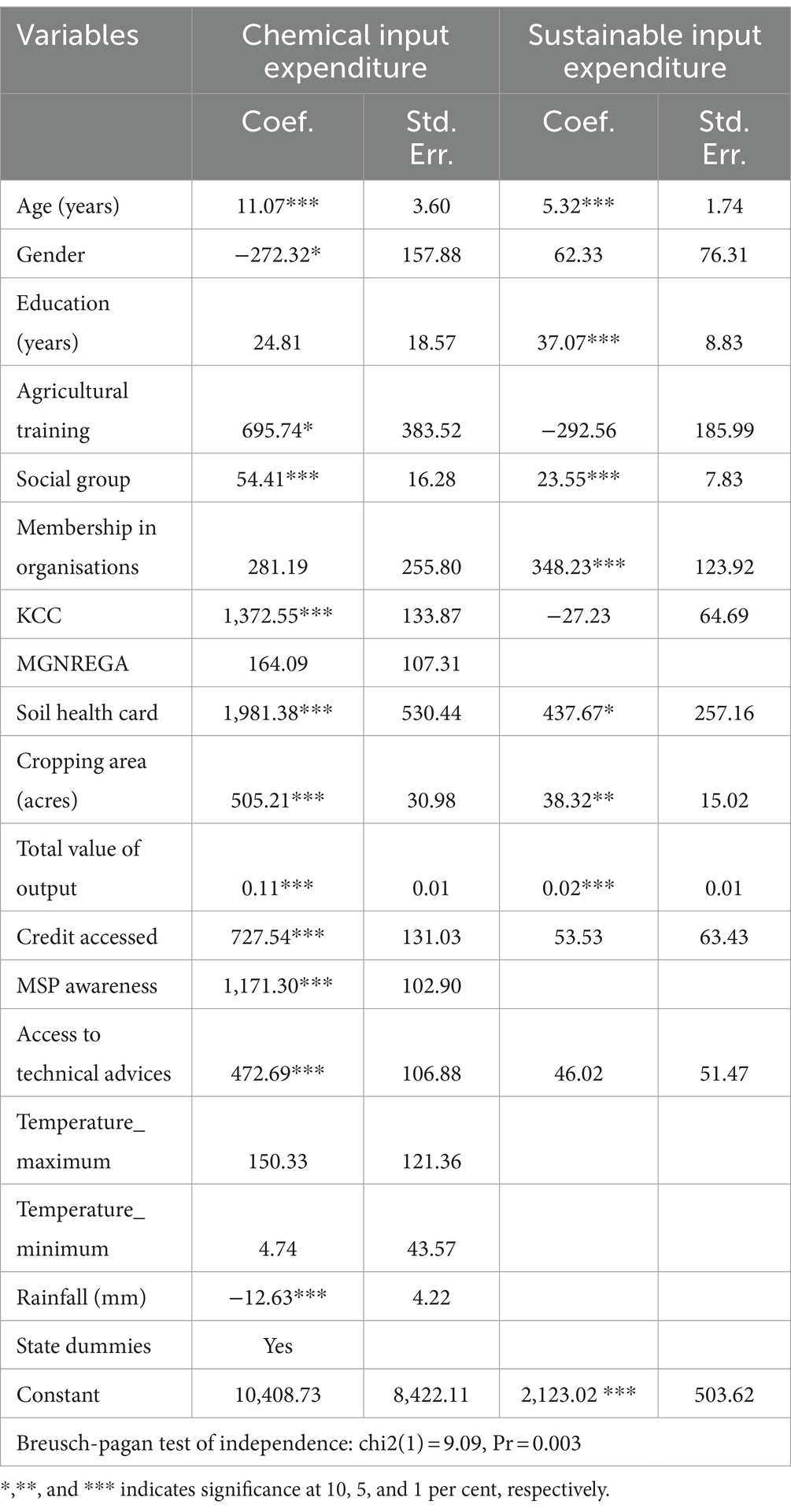
Table 5. Estimates of determinants of expenditure on chemical and sustainable inputs using seemingly unrelated regression.
4.3 Impact using regression adjustment
Table 6 presents the results of the regression adjustment, focusing on the impact of sustainable inputs on harvest value. The findings indicate a statistically significant and positive influence of the adoption of sustainable inputs on harvest value, with an average increase of Rs 3,415 per hectare. This finding holds substantial policy implications, particularly since the government is actively seeking to encourage the adoption of sustainable inputs. The results underscore the necessity for robust government action, as they align with the goals of a sustainable future.
4.4 Technical efficiency
The data in Table 7 illustrates the distribution of paddy farms based on their expenditure levels for chemical and sustainable fertilisers (biofertilisers and manures) per hectare. About 68.37% of paddy farms spend less than 5,000 Rs./ha on chemical fertilisers, while 60.73% spend less than 2000 Rs./ha on sustainable fertilisers. On the other hand, only 22.68% of paddy farms allocate a budget ranging from 5,000 to 10,000 Rs./ha for chemical fertilisers. Additionally, 30.76% of these farms spend between 2000 and 5,000 Rs./ha on sustainable fertilisers. Furthermore, just 8.95% of paddy fields spend over 10,000 Rs./ha on chemical fertilisers, while 8.50% invest more than 5,000 Rs./ha on sustainable fertilisers.
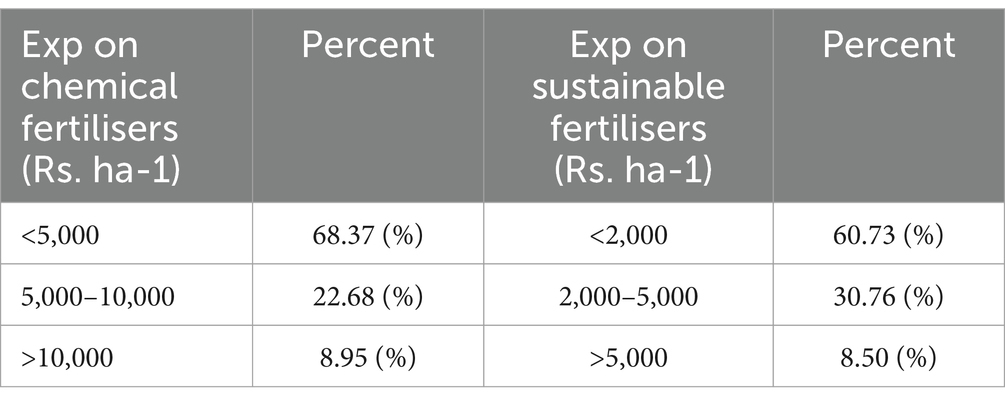
Table 7. Expenditure on chemical fertilisers and sustainable fertilisers (manure and biofertiliser) per hectare by farmers.
The stochastic production frontier model examines nine input variables, including bio-fertiliser, manures, chemical fertiliser, human labor, seed, irrigation, animal labor, maintenance of machinery, and hiring of machinery. The output in this model is based on the income in rupees, as shown in Table 8. In the Stochastic Frontier model, the total income is the dependent variable, while the costs (spending) of seeds, chemical fertilisers, bio-fertilisers, manures, equipment maintenance, human and animal labor, machinery maintenance, and machinery hiring are used as covariates. All variables are in natural logarithm.
The Stochastic Frontier estimates of the coefficients are presented in Table 9. The coefficients of bio-fertilisers, chemical fertilisers, seeds, human labor, and animal labor are statistically significant and have positive signs. Conversely, irrigation and machinery hiring costs have negative coefficients. An increase in bio-fertiliser expenditure by 1% leads to a 0.2% increase in paddy yield. Similarly, a 1% increase in chemical fertiliser expenditure results in a 0.09% increase in yield. Increasing investment in seeds, human labor, and animal labor by 1% leads to yield increases of 0.03, 0.12, and 0.01%, respectively. However, an increase in irrigation expenditure is associated with a 0.02% decline in production output, indicating decreased efficiency. This suggests that excessive irrigation may lead to reduced yields and productivity. Moreover, increasing machinery expenditure by 1% correlates with a reduction in paddy production output by approximately 0.001%. Both variance parameters, σ and λ, are statistically significant. The primary aim of running the stochastic frontier model was to estimate the technical efficiency level of each observational unit in the sample. The findings can be compared across specified groups of interest while excluding the influence of additional variables representing the social attributes of the cross-sectional units in the sample (see Table 10).
The Stochastic production function is used to evaluate the technical efficiency of sample farms, which are then categorised into four distinct groups based on their efficiency levels. From Table 11, it is evident that the average technical efficiency of the selected paddy farms is 87.42%, with the lowest efficiency at 48.52% and the highest at 94.28%. The data in the table indicate that 147 farms operate at a level below 50%, while 15.64% demonstrate an efficiency ranging from 50 to 80%. A majority (67.65%) of the 9,651 households are operating at an efficiency level of 80 to 85 percent, and 15.68% are operating at an even higher efficiency level above 90%. Figure 5 depicts the histogram of technical efficiency scores.
Table 10 provides a comparison between the groups using chemical fertilisers and sustainable fertilisers with different costs incurred. It displays the means of technical efficiency scores for the groups using these fertilisers. The asterisks in the table indicate the significance level of the t-test, showing whether the technical efficiency mean score of the sustainable fertilisers was significantly higher than that of the chemical fertilisers. The findings show that the groups utilising sustainable fertilisers exhibited greater efficiency scores, with the exception of the group using >10,000 (Rs/ha). Those utilising sustainable fertilisers with even lower expenditure levels demonstrated higher mean efficiency scores compared to users of chemical fertilisers.
The data suggests that moderate spending on fertilisers tends to yield better results, possibly because of the use of optimal input levels. It is crucial to apply fertilisers at an ideal amount to preserve the well-being of paddy fields and promote sustainable agriculture methods. Excessive fertiliser can have detrimental effects on plants, soil, water quality, expenses, as well as the environment and human well-being. Hence, meticulous oversight of fertiliser utilisation is vital for the viability of agriculture and the preservation of the ecosystem.
4.4.1 Exogenous variable descriptions in the technical inefficiency effects model and its descriptive statistics
The sample farms show some level of technical inefficiency. Enhancing the level of efficiency can lead to increased production. Farmers who effectively manage production inputs that significantly impact production efficiency can improve the efficiency of their farms. Therefore, it is crucial to identify the important production inputs that either positively or negatively affect output. The level of technical inefficiency is explained by the statistical description of exogenous variables related to farmer and farm characteristics in the inefficiency effects model, as shown in Table 12.
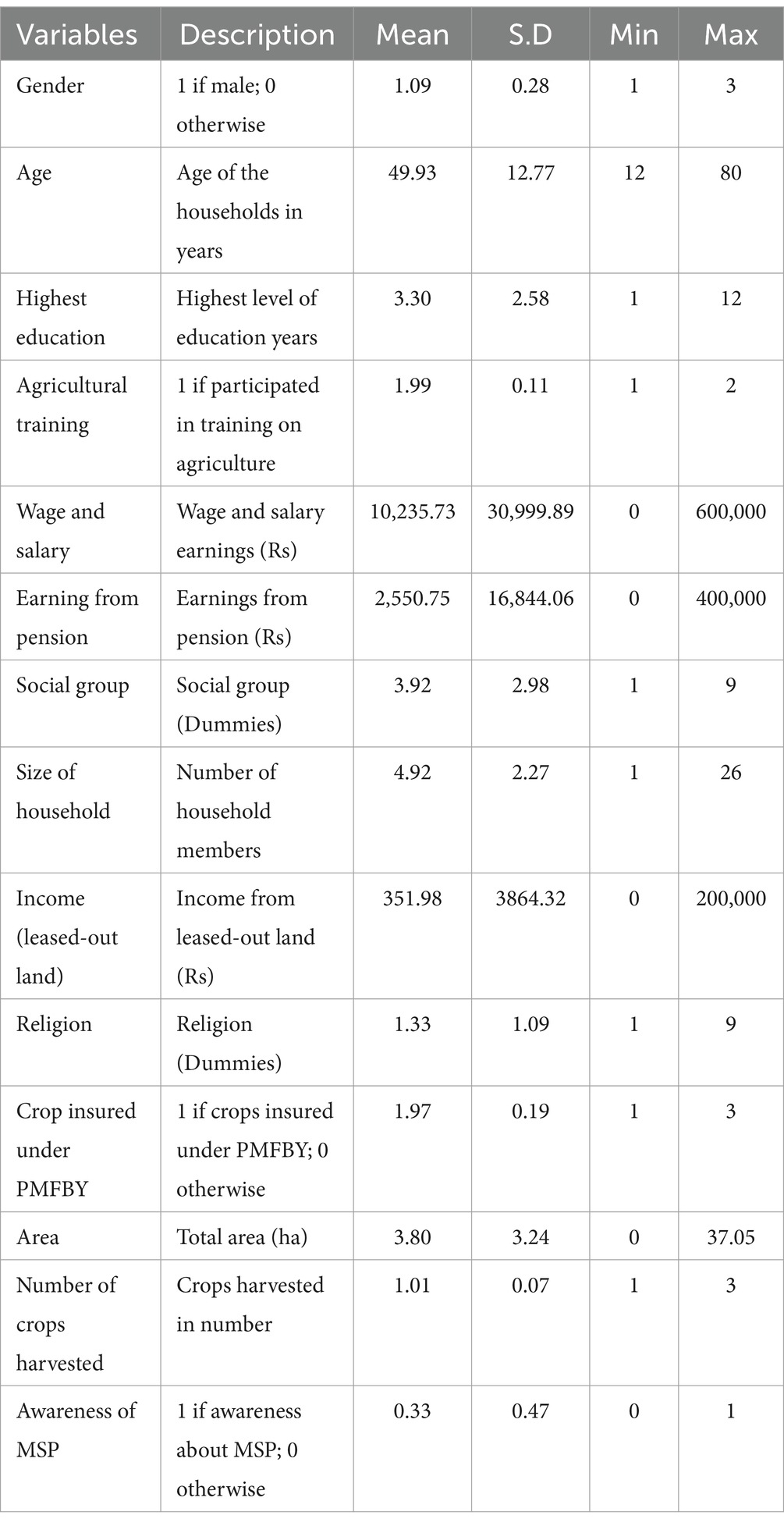
Table 12. Descriptive statistics and exogenous variable descriptions in the technical inefficiency effects model.
Considering the attributes of farmers, it is expected that the level of education will have a negative impact on the technical inefficiency of paddy farming. This suggests that farmers with higher levels of education exhibit lower levels of technical inefficiency due to their enhanced knowledge and ability to utilise information and modern technology. The average educational attainment of farmers in the delta is approximately 6 years of formal education. In terms of experience, farmers with more years of experience are likely to be more skilled, leading to reduced inefficiency. Additionally, farmers who have a larger land area are expected to have superior skills in managing input resources, resulting in a more efficient yield.
4.4.2 Determinants of technical inefficiency
Table 13 presents the estimated relationships between technical inefficiency and various socio-economic and demographic factors of the respondents. This analysis also considers the influence of additional variables on efficiency levels. When the coefficient of the evaluation is negative, it indicates that the relevant variable has a positive effect on technical efficiency; conversely, when it’s positive, it suggests that the variable has a negative effect on technical efficiency.
According to Table 13, higher education has a significant negative impact on technical inefficiency in paddy production. This suggests that as farmers attain higher levels of education, there is a small reduction in technical inefficiency. More specifically, each additional unit of education is associated with a reduction of approximately 0.034% in technical inefficiency. Higher education generally provides individuals with enhanced decision-making and problem-solving abilities. Farmers with a higher level of education are likely to possess superior skills in strategising and overseeing their agricultural activities, resulting in a decrease in inefficiencies. They are also more likely to acquire and understand information regarding optimal farming methods, emerging technologies, and market conditions, which can help them enhance their operational efficiency. Pursuing higher education can also enhance individuals’ inclination and capacity to adopt modern farming techniques and technologies, thereby reducing inefficiencies. Individuals with a higher level of education are more likely to have enhanced networking opportunities and access to support systems such as agricultural extension services and cooperatives, which can further assist them in operating with greater efficiency.
The impact of agricultural training on technical inefficiency is significant. Specifically, for every 1% increase in agricultural training, there is a decrease of approximately 0.001% in technical inefficiency. Agricultural training provides farmers with knowledge of advanced farming techniques, leading to waste reduction, optimal resource utilisation, increased productivity, and improved efficiency. These programs educate farmers on efficient resource management techniques, including optimal use of water, fertilisers, and pesticides, resulting in decreased input expenses and improved efficiency. Farmers gain knowledge about optimal techniques in agriculture, such as crop rotation, soil health management, and integrated pest management, which enhance farming efficiency. Training also improves farmers’ problem-solving abilities, enabling them to effectively tackle issues such as pest infestations, plant diseases, and soil fertility, resulting in reduced inefficiency. Furthermore, agricultural training often includes instruction on risk management techniques, helping farmers reduce the impact of uncertainties such as weather conditions and market fluctuations, leading to more consistent and productive agricultural practices.
Household size also has a significant impact on technical inefficiency. A larger household size is associated with a small rise in technical inefficiency. Specifically, a 1% increase in household size is linked to a roughly 0.045% rise in technical inefficiency. Larger household sizes can strain resources, resulting in less-than-optimal utilisation of agricultural inputs and heightened inefficiency. Additional individuals in a household may lead to increased resource allocation away from productive investments to provide sustenance for more people. Household members involved in non-agricultural tasks or other responsibilities may reduce attention and effort dedicated to farming activities, leading to increased inefficiency and higher overall expenses. This economic burden can reduce the available capital for investment in efficient farming practices and technologies. Larger households may also experience problems related to overcrowding and resource dilution, resulting in decreased overall farm efficiency. Moreover, households with a greater number of people may have a higher dependency ratio, leading to increased pressure on working members and decreased overall productivity.
Membership in a specific social group has a significant negative impact on technical inefficiency. It suggests that belonging to this social group is linked to a reduction of about 0.210% in technical inefficiency. Certain demographic cohorts may have better access to extensive social networks that offer help, knowledge, and resources, leading to more efficient agricultural practices. Belonging to supportive social groups can provide individuals with access to communal resources, collaborative efforts, and shared expertise, thereby reducing inefficiencies. Some social groups may have cultural practices and traditions that prioritise the efficient use of resources and the implementation of sustainable agricultural methods. Social collectives can improve the accessibility of educational and agricultural training programs, thus promoting the adoption of advanced farming methods. Groups often engage in collaborative practices such as cooperative farming, joint purchase of inputs in large quantities, and collective marketing of their produce. These practices can lead to increased efficiency.
The land area has a significant positive impact on technical inefficiency. Increasing the land area is associated with a rise in technical inefficiency of approximately 0.074%. Managing larger land areas can pose significant challenges. Coordinating activities over a larger geographical area can result in inefficiencies, especially if resources and labor are not appropriately managed. Efficiently distributing resources (such as labor, water, and fertilisers) becomes more challenging when dealing with a larger area. Smaller farms could benefit from implementing more meticulous and concentrated management practices. Larger agricultural operations often require a higher number of workers, and overseeing a larger labor force can be complex. Inefficiencies can occur due to difficulties in overseeing and effectively utilising all employees. As the farm expands in size, the operations involved, such as crop rotation, pest management, and harvesting, become more complex, which can lead to inefficiencies if not managed effectively. Although larger farms can take advantage of economies of scale, they may also face diseconomies of scale if their expansion leads to inefficiencies caused by the aforementioned factors.
The number of crops harvested has a significant positive impact on technical inefficiency. Specifically, the data shows that for each additional crop harvested, there is an approximate 1.351% increase in technical inefficiency. Managing multiple crops simultaneously adds complexity to farm management. Various crops have different requirements in terms of timing, labor, and inputs, which can result in inefficiencies if not effectively managed. Efficiently distributing resources such as labor, water, and fertilisers among multiple crops can pose a challenge. The complexity of the situation can result in less-than-optimal utilisation of resources, consequently leading to inefficiency. Every crop requires distinct farming methods, pest control approaches, and harvesting procedures. Simultaneously managing these diverse requirements can lead to inefficiencies. Cultivating multiple crops can increase vulnerability to pests and diseases, as different crops may attract distinct pests or have different susceptibilities. Effectively managing these risks is a complex task that can result in inefficiencies. Agricultural practitioners require a comprehensive range of knowledge to efficiently oversee various types of crops. Inadequate proficiency in a specific crop can result in ineffective methods and decreased overall efficiency.
Awareness of the Minimum Support Price (MSP) is linked to a reduction in technical inefficiency. More precisely, having knowledge of the MSP is linked to a reduction of approximately 0.299% in technical inefficiency. Farmers who are aware of the Minimum Support Price (MSP) are able to secure a fixed price for their agricultural products, mitigating the uncertainty linked to volatile market prices. This guarantee can result in improved strategic planning and allocation of resources, consequently minimising inefficiencies. The existence of a guaranteed minimum price for their crops may incentivise farmers to allocate more resources towards high-quality inputs and farming techniques, thereby improving productivity and minimising inefficiencies. Increased awareness of MSP is often associated with improved access to market information and support services. Farmers are more likely to be well-informed about market conditions, which in turn enables them to make more efficient decisions. The MSP serves as a safeguard that mitigates the financial hazards linked to agriculture. Minimising the potential for harm can result in farming operations that are more consistent and productive. Moreover, farmers tend to be more knowledgeable about other government assistance programs and subsidies, which can further optimise their productivity by offering supplementary resources and support.
5 Conclusion
The literature widely recognises the significant roles of sustainable inputs in improving productivity, ensuring food security, and reducing poverty. Despite these acknowledged benefits and efforts to promote awareness and adoption of these innovations in developing countries, farmers’ adoption rates are often perceived as generally low. In this study, we used cross-sectional data from the 77th round of the National Sample Survey to investigate the factors influencing the adoption of multiple sustainable inputs in India. The reference period for the data is 2018–19, which is a limitation of this study. Yet, this data permitted us to explore the factors influencing the adoption of sustainable inputs and their intensity (expenditure) at the farm household level. This sequence is not generally covered in adoption literature and, hence, is the strength of this study. The sustainable inputs we considered include biofertilisers, biopesticides, and organic manure.
Our study results reveal that several factors, including the age of the household head, gender, education, household size, and access to technical advisory services, influence the adoption of various sustainable inputs and the intensity of their use. Specifically, the probability of adopting these inputs tends to increase with age and farm size. Our findings underscore the interdependence of agricultural technologies and innovations, highlighting the need to consider this nature when devising strategies for the development and dissemination of sustainable inputs in India and other similar developing countries with a focus on agriculture. Given the diverse factors influencing various combinations of sustainable inputs, policymakers must consider farm managerial, socio-economic, and plot-specific factors when designing incentives for farmers to adopt multiple sustainable inputs. Further, the adoption of sustainable inputs significantly increases the harvest value of the crops and, hence, the income of farmers. The analysis employing a stochastic frontier production function in the context of paddy farmers’ efficiency yielded several significant findings. Notably, sustainable fertilisers, chemical fertilisers, seeds, human and animal labour demonstrated positive coefficients, while irrigation and machinery hiring costs exhibited adverse effects. Beyond these findings, the study indicates that governments should focus on investing in education and training initiatives for farmers and supporting agricultural cooperatives to enhance access to resources. In addition, the creation of knowledge systems would help to build further information and training channels capable of activating new learning processes for these inputs.
Our findings have several important implications for policy development. The need to factor in interdependence when devising effective strategies for the advancement and dissemination of sustainable inputs in paddy cultivation in India is reiterated. Given the diverse factors influencing varying combinations of sustainable inputs, policymakers should consider numerous farm management, socio-economic, and plot-specific factors when creating incentives for farmers to adopt multiple sustainable inputs. Though the adoption of sustainable inputs has a significant positive impact on increasing the harvest value for farmers, it is advisable to use them in conjunction with chemical inputs to reach higher levels of technical efficiency. National policies such as PM-PRANAM and Paramparagat Krishi Vikas Yojana can benefit from the findings of this study to improve their effectiveness at the grassroots level. Additionally, these findings emphasize the importance of sustainable inputs for India to achieve SDGs 2, 12, and 13. Although the findings from this research help, future research endeavours in this domain should build upon these findings to develop targeted interventions and policies that improve technical efficiency, productivity, and sustainability in the agricultural sector. Future studies should also consider environmental effects and risks faced by farmers, which were not covered in this study.
Data availability statement
Publicly available datasets were analyzed in this study. This data can be found at: https://microdata.gov.in/nada43/index.php/catalog/157.
Ethics statement
The studies involving humans utilizes the survey dataset from “Situation Assessment of Agricultural Households and Land and Livestock Holdings of Households in Rural India” of the 77th National Sample Survey (NSSO) round. The studies were conducted in accordance with the local legislation and institutional requirements. The participants provided informed consent to participate in this study.
Author contributions
GJ: Conceptualization, Supervision, Writing – original draft, Writing – review & editing. PV: Formal analysis, Methodology, Software, Writing – original draft, Writing – review & editing. AB: Conceptualization, Supervision, Writing – review & editing. CL: Formal analysis, Software, Writing – original draft. DK: Data curation, Formal analysis, Software, Writing – review & editing. TB: Methodology, Writing – review & editing. VE: Conceptualization, Supervision, Writing – review & editing.
Funding
The author(s) declare financial support was received for the research, authorship, and/or publication of this article. The authors report funding support from UKRI GCRF South Asian Nitrogen Hub (SANH). The project team includes partners from across South Asia and the UK. Neither UKRI nor any of the partner institutions are responsible for the views advanced here.
Acknowledgments
The authors acknowledge the contributions of Pradeep Panghal for his contribution to primary data collection.
Conflict of interest
The authors declare that the research was conducted in the absence of any commercial or financial relationships that could be construed as a potential conflict of interest.
Publisher’s note
All claims expressed in this article are solely those of the authors and do not necessarily represent those of their affiliated organizations, or those of the publisher, the editors and the reviewers. Any product that may be evaluated in this article, or claim that may be made by its manufacturer, is not guaranteed or endorsed by the publisher.
References
Abdulai, A. R. (2022). A new green revolution (GR) or neoliberal entrenchment in Agri-food systems? Exploring narratives around digital agriculture (DA), food systems, and development in sub-Sahara Africa. J. Dev. Stud. 58, 1588–1604. doi: 10.1080/00220388.2022.2032673
Aigner, D., Lovell, C. A. K., and Schmidt, P. (1977). Formulation and estimation of stochastic frontier production function models. J. Econom 6, 21–37. doi: 10.1016/0304-4076(77)90052-5
Akanmu, A. O., Akol, A. M., Ndolo, D. O., Kutu, F. R., and Babalola, O. O. (2023). Agroecological techniques: adoption of safe and sustainable agricultural practices among the smallholder farmers in Africa. Front. Sustain. Food Syst. 7:1143061. doi: 10.3389/fsufs.2023.1143061
Anani, O. A., and Adetunji, C. O. (2021). Role of pesticide applications in sustainable agriculture. Appl. Soil Chem., 235–256. doi: 10.1002/9781119711520.CH13
Asprooth, L., Norton, M., and Galt, R. (2023). The adoption of conservation practices in the Corn Belt: the role of one formal farmer network, practical farmers of Iowa. Agric. Human Values 40, 1559–1580. doi: 10.1007/s10460-023-10451-5
Bezu, S., Kassie, G. T., Shiferaw, B., and Ricker-Gilbert, J. (2014). Impact of improved maize adoption on welfare of farm households in Malawi: a panel data analysis. World Dev. 59, 120–131. doi: 10.1016/J.WORLDDEV.2014.01.023
Dittmer, K. M., Rose, S., Snapp, S. S., Kebede, Y., Brickman, S., Shelton, S., et al. (2023). Agroecology can promote climate change adaptation outcomes without compromising yield in smallholder systems. Environ. Manag. 72, 333–342. doi: 10.1007/s00267-023-01816-x
Fageria, N. K. (2007). Soil fertility and plant nutrition research under field conditions: basic principles and methodology. J. Plant Nutr. 30, 203–223. doi: 10.1080/01904160601117887
Farrell, M. J. (1957). The measurement of productive efficiency. J. R. Stat. Soc. Ser. A 120, 253–281. doi: 10.2307/2343100
Fertiliser Association of India (2023). Fertiliser statistics 2022–23. 2023rd Edn. New Delhi: Fertiliser Association of India.
Gaddam, A., Malla, S., Narayana, D., and Kumar Shukla, M. (2022). Creating an optimal portfolio of crops using Price forecasting to increase ROI for Indian farmers co-authors. Available at: https://doi.org/10.48550/arXiv.2211 (Accessed July 23, 2024).
Goyal, S. S., Dave, R., Kumar, R., and Bhatia, U. (2024). Indian interstate trade exacerbates nutrient pollution in food production hubs. Commun. Earth Environ. 5:9. doi: 10.1038/s43247-023-01178-6
Hellin, J., and Fisher, E. (2019). The Achilles heel of climate-smart agriculture. Nat. Clim. Chang. 9, 493–494. doi: 10.1038/s41558-019-0515-8
Isensee, C., Griese, K.-M., and Teuteberg, F. (2021). Sustainable artificial intelligence: A corporate culture perspective. Sustain. Manag. Forum 29, 217–230. doi: 10.1007/s00550-021-00524-6
Jafar, A., Bibi, N., Naqvi, R. A., Sadeghi-Niaraki, A., and Jeong, D. (2024). Revolutionizing agriculture with artificial intelligence: plant disease detection methods, applications, and their limitations. Front. Plant Sci. 15:1356260. doi: 10.3389/fpls.2024.1356260
Jena, P. R. (2022). Extension services as key determining factor for adoption of minimum tillage practice in Kenya: a plot level analysis. J. Public Aff. 22:e2724. doi: 10.1002/PA.2724
Kagoya, S., Paudel, K. P., and Daniel, N. L. (2018). Awareness and adoption of soil and water conservation Technologies in a Developing Country: a case of Nabajuzi watershed in Central Uganda. Environ. Manag. 61, 188–196. doi: 10.1007/s00267-017-0967-4
Kamau, J. W., Schader, C., Biber-Freudenberger, L., Stellmacher, T., Amudavi, D. M., Landert, J., et al. (2022). A holistic sustainability assessment of organic (certified and non-certified) and non-organic smallholder farms in Kenya. Environ. Dev. Sustain. 24, 6984–7021. doi: 10.1007/s10668-021-01736-y
Kassie, M., Jaleta, M., Shiferaw, B., Mmbando, F., and Mekuria, M. (2013). Adoption of interrelated sustainable agricultural practices in smallholder systems: evidence from rural Tanzania. Technol. Forecast Soc. Change 80, 525–540. doi: 10.1016/j.techfore.2012.08.007
Kishore, A., Praveen, K. V., and Roy, D. (2013). Direct cash transfer system for fertilisers: why it might be hard to implement. Econ. Polit. Wkly. 48, 54–63. Available at: https://www.epw.in/journal/2013/52/review-rural-affairs-review-issues/direct-cash-transfer-system-fertilisers.html
Lamm, A. J., Lamm, K. W., Trojan, S., Sanders, C. E., and Byrd, A. R. (2023). A needs assessment to inform research and outreach efforts for sustainable agricultural practices and food production in the Western United States. Food Secur. 12:630. doi: 10.3390/foods12081630
Maddela, N. R., Ramakrishnan, B., Kakarla, D., Venkateswarlu, K., and Megharaj, M. (2022). Major contaminants of emerging concern in soils: a perspective on potential health risks. RSC Adv. 12, 12396–12415. doi: 10.1039/d1ra09072k
Meeusen, W., and van den Broeck, J. (1977). Technical efficiency and dimension of the firm: some results on the use of frontier production functions. Empir. Econ. 2, 109–122. doi: 10.1007/BF01767476
Mgomezulu, W. R., Machira, K., Edriss, A. K., and Pangapanga-Phiri, I. (2023). Modelling farmers’ adoption decisions of sustainable agricultural practices under varying agro-ecological conditions: a new perspective. Innov. Green Dev. 2:100036. doi: 10.1016/j.igd.2023.100036
Nagar, A., Nauriyal, D. K., and Singh, S. (2021). Determinants of farmers’ access to extension services and adoption of technical inputs: evidence from India. Univers. J. Agric. Res. 9:404. doi: 10.13189/UJAR.2021.090404
Naveed, M. A., and Hassan, A. (2021). Sustaining agriculture with information: an assessment of rural citrus farmers’ information behaviour. Inf. Dev. 37, 496–510. doi: 10.1177/0266666920932994
Ndiritu, S. W., Kassie, M., and Shiferaw, B. (2014). Are there systematic gender differences in the adoption of sustainable agricultural intensification practices? Evidence from Kenya. Food Policy 49, 117–127. doi: 10.1016/J.FOODPOL.2014.06.010
Nguyen, L. L. H., Khuu, D. T., Halibas, A., and Nguyen, T. Q. (2023). Factors that influence the intention of smallholder Rice farmers to adopt cleaner production practices: an empirical study of precision agriculture adoption. Eval. Rev. 48, 692–735. doi: 10.1177/0193841X231200775
Onumah, J. A., Osei, R. D., Martey, E., and Asante, F. A. (2023). Welfare dynamics of innovations among agricultural households in Ghana: implication for poverty reduction. Heliyon 9:e18066. doi: 10.1016/j.heliyon.2023.e18066
Oyetunde-Usman, Z., Olagunju, K. O., and Ogunpaimo, O. R. (2021). Determinants of adoption of multiple sustainable agricultural practices among smallholder farmers in Nigeria. Int. Soil Water Conserv. Res. 9, 241–248. doi: 10.1016/J.ISWCR.2020.10.007
Passarelli, M., Bongiorno, G., Cucino, V., and Cariola, A. (2023). Adopting new technologies during the crisis: an empirical analysis of agricultural sector. Technol. Forecast. Soc. Change 186:122106. doi: 10.1016/j.techfore.2022.122106
Pathak, H. (2023). Transforming the Agri-food sector in India for achieving the sustainable development goals. Anthr. Sci. 2, 1–4. doi: 10.1007/s44177-023-00044-6
Paul, B., Murari, K. K., Patnaik, U., Bahinipati, C. S., and Sasidharan, S. (2023). Sustainability transition for Indian agriculture. Sci. Rep. 13:7290. doi: 10.1038/s41598-023-34092-0
Piñeiro, V., Arias, J., Dürr, J., Elverdin, P., Ibáñez, A. M., Kinengyere, A., et al. (2020). A scoping review on incentives for adoption of sustainable agricultural practices and their outcomes. Nat. Sustain. 3, 809–820. doi: 10.1038/s41893-020-00617-y
Pingali, P. L. (2012). Green revolution: impacts, limits, and the path ahead. Proc. Natl. Acad. Sci. 109, 12302–12308. doi: 10.1073/PNAS.0912953109
Praveen, K. (2017). Indian fertilizer policies: revisiting the odyssey and lessons from abroad. Curr. Sci. 113, 1246–1254. doi: 10.18520/cs/v113/i07/1246-1254
Praveen, K. V., and Aditya, K. S. (2022). Fertilizer Consumption in Wheat Production Systems: Trends and Policies. In: New Horizons in Wheat and Barley Research. Eds. P. L. Kashyap, et al. Springer, Singapore.
Praveen, K., and Alka, S. (2019). Realizing the potential of a low-cost technology to enhance crop yields: evidence from a meta-analysis of biofertilizers in India. Agric. Econ. Res. Rev. 32, 77–91. doi: 10.5958/0974-0279.2019.00018.1
Pretty, J., and Bharucha, Z. P. (2014). Sustainable intensification in agricultural systems. Ann. Bot. 114, 1571–1596. doi: 10.1093/AOB/MCU205
Prusty, A. K., Natesan, R., Panwar, A. S., Jat, M. L., Tetarwal, J. P., López-Ridaura, S., et al. (2022). Redesigning of farming systems using a multi-criterion assessment tool for sustainable intensification and nutritional security in northwestern India. Sustainability (Switzerland) 14:3892. doi: 10.3390/su14073892
Rajna, S., Praveen, K. V., and Nebapure, S. M. (2023). The global trend in pesticide research: a bibliometric analysis. Pestic. Res. J. 35, 120–129. doi: 10.5958/2249-524X.2023.00021.3
Rizzo, G., Migliore, G., Schifani, G., and Vecchio, R. (2024). Key factors influencing farmers’ adoption of sustainable innovations: a systematic literature review and research agenda. Org. Agric. 14, 57–84. doi: 10.1007/s13165-023-00440-7
Rola-Rubzen, M. F., Paris, T., Hawkins, J., and Sapkota, B. (2020). Improving gender participation in agricultural technology adoption in Asia: from rhetoric to practical action. Appl. Econ. Perspect. Policy 42, 113–125. doi: 10.1002/AEPP.13011
Rust, N. A., Ptak, E. N., Graversgaard, M., Iversen, S., Reed, M. S., de Vries, J. R., et al. (2020). Social capital factors affecting uptake of sustainable soil management practices: a literature review. Emerald Open Res. 1:2. doi: 10.1108/EOR-10-2023-0002
Sampaio Morais, G. A., Silva, F. F., de Freitas, C. O., and Braga, M. J. (2021). Irrigation, technical efficiency, and farm size: the case of Brazil. Sustain. For. 13:1132. doi: 10.3390/SU13031132
Sargani, G. R., Shen, Y., Liu, Y., Joyo, M. A., Liu, J., Jiang, Y., et al. (2023). Farm risks, livelihood asset allocation, and adaptation practices in response to climate change: a cross-country analysis. Front. Environ. Sci. 10:1066937. doi: 10.3389/fenvs.2022.1066937
Sharma, S. B., Thivakaran, G. A., and Thakkar, M. G. (2021). The game changing role of traditional ecological knowledge based Agri amendment systems in nutrient dynamics in the stress prone semi arid tropics. Sci. Rep. 11, 1–10. doi: 10.1038/s41598-021-88801-8
Stuart, D. (2021). Input industry influence on farmer decision-making: An example of negative impacts to the environment and farmers. In: Handbook on the human impact of agriculture. Ed. H. S. JamesJr. (pp. 51–64). Edward Elgar Publishing.
Sulaiman, N. K., and Misnan, S. H. (2022). Environmental sustainability through agriculture: perspectives of extension agents on adoption of sustainable practices. IOP Conf. Ser. Earth Environ. Sci. 1082:12024. doi: 10.1088/1755-1315/1082/1/012024
Teklewold, H., Kassie, M., and Shiferaw, B. (2013). Adoption of multiple sustainable agricultural practices in rural Ethiopia. J. Agric. Econ. 64, 597–623. doi: 10.1111/1477-9552.12011
Ullaguari, J. X., Landaverde, R., Sanders, A., and Zickafoose, A. (2023). Farmer-producer organizations during the COVID-19 pandemic: examining the role of organization-producer relationships on coping, resiliency, and effectiveness. Adv. Agric. Dev. 4, 131–145. doi: 10.37433/aad.v4i3.331
Upadhayay, V. K., Chitara, M. K., Mishra, D., Jha, M. N., Jaiswal, A., Kumari, G., et al. (2023). Synergistic impact of nanomaterials and plant probiotics in agriculture: a tale of two-way strategy for long-term sustainability. Front. Microbiol. 14:1133968. doi: 10.3389/fmicb.2023.1133968
van den Berg, H., Phillips, S., Dicke, M., and Fredrix, M. (2020). Impacts of farmer field schools in the human, social, natural and financial domain: a qualitative review. Food Secur. 12, 1443–1459. doi: 10.1007/s12571-020-01046-7
Velayudhan, P. K., Singh, A., and Ks, A. (2023). “Paddy farmers’ preference for biofertilizers: insights from the indo-Gangetic Plains of India” in 2023 Annual Meeting, July 23-25, Washington DC: Agricultural and Applied Economics Association. Available at: http://ageconsearch.umn.edu
Velayudhan, P. K., Singh, A., Jha, G. K., Kumar, P., Thanaraj, K. I., and Srinivasa, A. K. (2021). What drives the use of organic fertilizers? Evidence from Rice farmers in indo-Gangetic Plains, India. Sustain. For. 13:9546. doi: 10.3390/SU13179546
Velayudhan, P. K., Sivalingam, N., Jha, G. K., Singh, A., and Pathak, H. (2024). Nitrogen budget of Indian agriculture: trends, determinants and challenges. Environ. Dev. Sustain. 26, 10225–10242. doi: 10.1007/S10668-023-03142-Y/METRICS
Voss, R. C., Gitonga, Z. M., Donovan, J., Garcia-Medina, M., and Muindi, P. (2024). Can I speak to the manager? The gender dynamics of decision-making in Kenyan maize plots. Agric. Human Values 41, 205–224. doi: 10.1007/s10460-023-10484-w
Wang, X., Hockmann, H., and Bai, J. (2012). Technical efficiency and producers’ individual technology: accounting for within and between regional farm heterogeneity. Canadian J. Agric. Econ. 60, 561–576. doi: 10.1111/J.1744-7976.2012.01264.X
Wu, J., and Babcock, B. A. (1998). The choice of tillage, rotation, and soil testing practices: economic and environmental implications. Am. J. Agric. Econ. 80, 494–511. doi: 10.2307/1244552
Zeweld, W., Van Huylenbroeck, G., Tesfay, G., Azadi, H., and Speelman, S. (2020). Sustainable agricultural practices, environmental risk mitigation and livelihood improvements: empirical evidence from northern Ethiopia. Land Use Policy 95:103799. doi: 10.1016/J.LANDUSEPOL.2019.01.002
Zhang, A. J., Matous, P., and Tan, D. K. Y. (2020). Forget opinion leaders: the role of social network brokers in the adoption of innovative farming practices in North-Western Cambodia. Int. J. Agric. Sustain. 18, 266–284. doi: 10.1080/14735903.2020.1769808
Zhao, Z., Peng, P., Zhang, F., Wang, J., and Li, H. (2022). The impact of the urbanization process on agricultural technical efficiency in Northeast China. Sustain. For. 14:12144. doi: 10.3390/SU141912144
Keywords: sustainable inputs, manure, biofertilizersbiofertilisers, biopesticides, technical efficiency, multivariate probit, ordered probit, regression adjustment
Citation: Jha GK, Velayudhan PK, Bhatia A, Laishram C, Kumar D, Begho T and Eory V (2024) Transitioning towards sustainable agriculture: analysing the factors and impact of adopting multiple sustainable inputs by paddy farmers in India. Front. Sustain. Food Syst. 8:1447936. doi: 10.3389/fsufs.2024.1447936
Edited by:
Yari Vecchio, University of Bologna, ItalyReviewed by:
Margherita Masi, University of Bologna, ItalySiphe Zantsi, Agricultural Research Council of South Africa (ARC-SA), South Africa
Copyright © 2024 Jha, Velayudhan, Bhatia, Laishram, Kumar, Begho and Eory. This is an open-access article distributed under the terms of the Creative Commons Attribution License (CC BY). The use, distribution or reproduction in other forums is permitted, provided the original author(s) and the copyright owner(s) are credited and that the original publication in this journal is cited, in accordance with accepted academic practice. No use, distribution or reproduction is permitted which does not comply with these terms.
*Correspondence: Toritseju Begho, VG9yaXRzZWp1LkJlZ2hvQHNydWMuYWMudWs=; Girish Kumar Jha, Z2lyaXNoLnN0YXRAZ21haWwuY29t; Praveen Koovalamkadu Velayudhan, dmVlbmt2QGdtYWlsLmNvbQ==
†These authors have contributed equally to this work and share first authorship