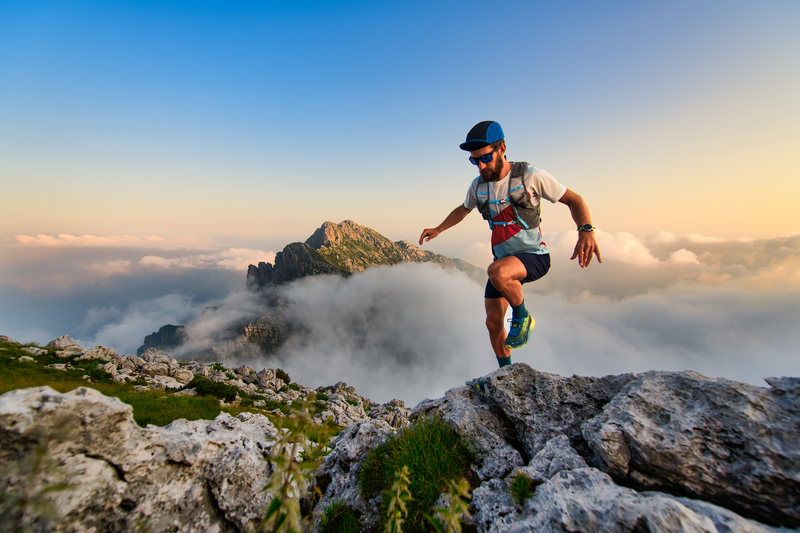
95% of researchers rate our articles as excellent or good
Learn more about the work of our research integrity team to safeguard the quality of each article we publish.
Find out more
SYSTEMATIC REVIEW article
Front. Sustain. Food Syst. , 24 October 2024
Sec. Land, Livelihoods and Food Security
Volume 8 - 2024 | https://doi.org/10.3389/fsufs.2024.1447186
Even if smallholder farmers have a large share in the Ethiopian economy, they operate below their potential. Land use is fundamental in determining the efficiency of farmers. However, the effect of land size on efficiency is still a controversial issue in the country. This review was designed to determine the effect of land size on farmers’ efficiency. The random-effects model showed that the farmers with smaller land sizes (≤0.5 ha) were more efficient by 21% than the farmers with larger land sizes. Moreover, the results of meta-regression analysis showed that resource endowment, labor quality, information contact, and total income were the important moderators of the presence of heterogeneity in the effect size among the studies. It was deduced that farmers are efficient with smaller land sizes since they have resources to manage their farms appropriately. Therefore, improving farmers’ production skills, providing input subsidies, and upgrading development agents’ skills are crucial to boost farmers’ efficiency in the country.
Although Ethiopia is diversifying its economic base, agriculture still accounts for more than three-quarters of employment, 36 percent of production, and more than one-third of total exports. The significant role of agriculture in the economy underscores its importance as a key factor in economic growth and poverty reduction (Birhanu et al., 2020; Diriba, 2020; Essa et al., 2012; Fisseha et al., 2022; Sime et al., 2022). Agricultural productivity has increased rapidly over the past decade, largely due to the intensification of modern seed usage, increased use of fertilizers, and improved farming techniques (FAO, 2023).
Agriculture, being one of the most water-intensive human activities, faces major challenges such as water resource shortages, unbalanced freshwater distribution, and discrepancies between supply and demand (Ingrao et al., 2023). With the rapid expansion of population and increase in food consumption, the shortage of agricultural resources, including farmland, water, and labor, has become increasingly severe (Miao et al., 2023).
In Ethiopia, productivity improvements over the past few years have been limited to key crops, with inadequate investments and insufficient advancements across various subsectors. In addition, yield growth remains inadequate to meet both domestic food security needs and industrial demands, while underdeveloped markets continue to hinder farmers from realizing returns on their input investments (FAO, 2023). Moreover, the agricultural sector faces significant constraints related to land use and administration systems, limited access to high-quality inputs and financing, inefficient market systems, and inadequate research and extension services (Gidey et al., 2021; Mengistu et al., 2024; Milkessa et al., 2019; Musa et al., 2015; Regasa et al., 2019).
To improve the efficient use of agricultural resources, Ethiopia has formulated relevant policies such as digitizing agriculture (PDC, 2020). Furthermore, to advance sectoral reforms, the specific problems that are prioritized under each of the main constraints need to be addressed. It is crucial to increase market-oriented agricultural production and productivity, agricultural value addition, and access to domestic and international markets (Ali et al., 2022; Beyan et al., 2013; Birara et al., 2023). These steps are essential for agriculture to effectively contribute to the structural transformation of the economy. In line with the homegrown economic reform agenda, the use of new and emerging technologies to modernize Ethiopia’s agricultural sector is paramount for optimal utilization and progress (Gidey et al., 2021; Tigabu et al., 2022; Tolesa, 2022; Tsegaye et al., 2022; Wudineh and Endrias, 2016; Zewdie et al., 2021; Gadisa and Addisu, 2022; Gavaghan et al., 2000).
The question of how to produce more food with limited agricultural resources and how to develop sustainable agriculture is currently one of the most important global issues (Eskeziaw et al., 2021; Getachew et al., 2018; Gidey et al., 2023; Hayatu, 2020; Hika and Afsaw, 2019; Hosaena and Holden, 2014; Kebebew et al., 2021; Kifle et al., 2020).
Land, an essential but scarce resource, has the potential to determine agricultural productivity and efficiency. Since this meta-analysis focuses on smallholders, it is better to address the question of how different levels of land size affect the relationship with efficiency change (Beneberu et al., 2018). Based on the findings of various researchers, not all smallholder farmers have a similar level of efficiency due to different systematic and idiosyncratic factors.
Different studies have reported controversial findings on the relationship between farm size and efficiency from a technical point of view. One finding indicated that there was a negative relationship between the two variables. This was because small farms mostly rely on family labor and hence have advantages in labor supervision (Milkessa et al., 2019; Adugna et al., 2023; Adugna et al., 2017; Agerie et al., 2019; Tolesa et al., 2019, Tekleyohannes et al., 2024; Kusse et al., 2021; Alemayehu, 2021; Eskeziaw et al., 2021). Another finding indicated that there was a positive relationship between the two, and this was due to smallholders’ utilization of low-quality inputs. Larger farms can gain large returns at a low cost (Tadesse et al., 2017; Anbes, 2020; Anteneh and Asrat, 2020; Belete, 2020; Beshir, 2017; Teka et al., 2021; Dagmawi, 2021; Hagos, 2021). Furthermore, another finding indicated a U-shaped relationship between farm size and efficiency (Dias and Gustavo, 2020). This pattern means that at first, efficiency decreases as land size increases, then remains constant at a minimum, and finally increases with the size of land. Therefore, based on these facts, the researcher can propose a concrete solution through in-depth analysis.
This review was designed to bridge the conceptual and methodological gaps that exist in the issue under consideration. Regarding the conceptual gap, there are inconsistent findings on the effects of land size on efficiency. Some findings are positive, whereas others have reported a negative effect. Therefore, this review was designed to clarify or resolve these inconsistencies. Moreover, the final gap reviewed was the methodological gap. Previous studies were more oriented toward a narrative type of review, while this study focused on systematic analysis, which is concrete and uses statistical methods. Based on these gaps, this review aimed to unveil the effect of land size on the efficiency (from a technical point of view) of farmers in Ethiopia.
This review is divided into four sections: introduction, methodology, results and discussion, and conclusion and recommendations. A detailed report of the findings is provided in each section of the manuscript.
Only studies conducted in Ethiopia were considered in this review. Ethiopia is partitioned into 12 regions. As the country is mainly based on agriculture, it is possible to obtain a large number of articles on the issue under consideration. In connection with targeted studies, this review paper focuses on agricultural-related articles in general and on crop production-related articles in particular.
Preferred Reporting Items for Systematic reviews and Meta-Analyses (PRISMA) were used to select articles for the review. The PRISMA checklist includes a four-phase flow diagram that incorporates the stages of article identification, screening, eligibility, and inclusion. A literature search was conducted from 1 March 2024 to 25 May 2024.
Articles were browsed using the following keywords: efficiency “OR” technical efficiency “AND” crop production “OR” agricultural production “AND” farmers “AND” Ethiopia. Databases such as Google Scholar, ScienceDirect, Taylor & Francis, and AGRIS were used in this study.
The final relevant articles required for the meta-analysis were identified following the application of inclusion and exclusion criteria, as shown in Table 1.
STATA version 14 software was used for the analysis. Models were selected based on heterogeneity among the studies. A random-effects model was used for high heterogeneity, while a fixed-effects model was used for low heterogeneity. In this review, the random-effects model was applied because of the occurrence of high heterogeneity among studies. The standard mean difference (SMD) was used as the effect size measurement.
A random-effects meta-analysis model assumes that the observed estimates of the treatment effect can vary across studies because of real differences in the treatment effect in each study, as well as sampling variability (chance). Thus, even if all studies had an infinitely large sample size, the observed study effects would still vary because of real differences in treatment effects. Such heterogeneity in treatment effects is caused by differences in study populations (such as the number of inputs used, income, labor quality, and contact with information), interventions received (differences in land size holdings), study design, and measurement of the outcome.
The random-effects model can be written as shown on Equation (1):
where,
yi is the effect size measured in standard mean difference.
b0 is the constant term.
si is a study-specific deviation from the overall mean for the 𝑖th study; it is normally distributed with the between-study variance, often referred to as 𝜏2, and the others are the same as above. Unlike the common-effect model, a random-effects model assumes that different studies have different means. Note that the weights for this model are 1/(τ2 + vi) 1/(𝜏2+𝑣𝑖).
ei is the error term.
Heterogeneity was assessed using Cochran’s Q and tau-squared tests adjusted for degrees of freedom. The significance of Cochran’s q-value indicated the presence of heterogeneity among studies. The classical measure of heterogeneity is Cochran’s Q, which is calculated as the weighted sum of the squared differences between individual study effects and the pooled effect across studies, with the weights being those used in the pooling method. Q is distributed as a chi-squared statistic with k (the number of studies) minus 1 degree of freedom.
Q has too much power as a test of heterogeneity if the number of studies is large (Higgins et al., 2003). Q is referred to as a test of homogeneity in Stata and is computed as shown on Equation (2).
where, Wi is the study weight (1/Vi), Yi is the study effect size, M is the summary effect, and k is the number of studies.
The other measure of heterogeneity used in this study was tau-squared as shown on Equations (3, 4). Tau-squared is defined as the variance of the true effect sizes. The tau-squared value was estimated by calculating T2. To do this, we begin with (Q – df) and divide this quantity by C.
where,
The tau-squared value can never be less than zero because the actual variance of the true effects cannot be less than 0. Moreover, as the tau-squared value approaches zero, it confirms the absence of heterogeneity among the studies.
If heterogeneity is detected, two solutions are recommended: subgroup analysis and meta-regression analysis. The use of either method is determined by the type of the moderator. To solve for heterogeneity, a moderator(s) should be selected. These moderators are responsible for the presence of heterogeneity. Based on the review of the articles, common factors were selected.
In this review, there was high heterogeneity among the studies, following which meta-regression was chosen because there was more than one continuous moderator. The meta-regression is estimated as shown on Equation (5):
where,
is the observed effect size of the studies;
θ is the constant term.
is the coefficient for the moderators.
is the sampling variance of the observed outcomes or effect size estimates (i.e., standardized mean differences).
indicates that even the true effect size of the study was only sampled from an overarching distribution of effect sizes. This means that between-study heterogeneity existed in our data, which was captured by the heterogeneity variance 𝜏2.
A post-estimation test was performed to ensure the validity of the included studies. Two diagnostic tests were performed. The first was a statistical test using Begg’s test. If the test showed insignificance, it confirmed that there was no small study effect, and appropriate studies were incorporated into the analysis. The other post-estimation test used a funnel plot, which is a graphical representation of the symmetrical distribution of the studies on the mean effect. If the distribution is symmetric, it indicates that appropriate studies were included in the meta-analysis.
The important variables used for conducting the meta-analysis are described in the tables. Table 2 shows the outcome variable, technical efficiency, and the intervention variable, land size. Technical efficiency is a continuous variable measured using a score ranging from zero to one. In this review, efficiency studies that used only the stochastic frontier approach rather than data envelopment analysis were selected to maintain methodological similarity. The intervention variable is a dummy variable and is measured as zero if the land size is less than or equal to 0.5 ha and as one if it is more than 0.5 ha. A land size of less than 0.5 ha was considered small, while a land size larger than 0.5 was considered a larger landholding, according to Genet (2012). This classification is used in the context of discussing land scarcity and farmers’ land acquisition in Ethiopia and the member countries of Intergovernmental Authority on Development (IGAD).
Table 3 lists the variables used in the meta-regression analysis. The dependent variable is the effect size, which is a continuous variable measured by the standard mean difference. The moderators or predictors that have a hypothesized effect on the dependent variable are factor endowment, labor quality, information contact, and total income. In this review, the variables that commonly affected the dependent variable in all identified studies were considered as the predictors.
Factor endowment refers to the continuous variables measured by the number of factors or inputs (such as urea, DAP, seed, labor, land, herbicide, and pesticide) used by farmers in producing crops. This was hypothesized to have a positive effect on the dependent variable.
Labor quality is a continuous variable measured in quintals per hour or man-days. This indicates the amount of crop production produced by a household per hour. Skillful farmers have the potential to accomplish multiple farm activities within a short period. Regardless of the type of crop, this is an indicator of performance in the field, meaning that the larger the index, the more qualified the farmer is. This can make them effective and efficient. This is expected to have a positive effect on the dependent variable. Information contact is a continuous variable measured by the frequency of contact made by farmers per annum with extension professionals. This was hypothesized to have a positive effect on the dependent variable.
Finally, total income is a continuous variable measured in birr. This signifies the total amount of birr acquired by farmers through on-farm and off-farm activities. The income earned from working on the farm, along with other alternative income sources, is essential for gaining access to improved production factors. This is expected to have a positive effect on the dependent variable.
Approximately 45 articles were identified based on the Preferred Reporting Items for Systematic reviews and Meta-Analyses article selection criteria. In the initial step, 255 articles were identified through database searching and referencing. Databases such as Google Scholar, ScienceDirect, Taylor & Francis, and AGRIS were used to obtain the relevant types and numbers of articles. A total of 50 articles were excluded due to duplication, leaving 205 articles. Among these articles, approximately 100 were excluded because they were conducted outside Ethiopia, i.e., in other countries.
Moreover, 60 additional articles were excluded because they were book chapters, abstract-only papers, review articles, or non-study articles. Finally, 45 articles were identified and used in the meta-analysis. The overall article identification process is illustrated using the PRISMA flow diagram in Figure 1.
When we analyzed the studies based on area coverage, we found that various technical efficiency-focused studies were conducted in different parts of Ethiopia. The distribution of these studies across regions has implications for the future agricultural policy agenda of the country. The names of the study areas and the regions in which the studies were conducted are presented in the table.
Based on the table results, approximately 17 (37.79%) studies were conducted in the Oromia region, 13 (28.89%) in the Amhara region, two (4.44%) in the Southern region, 2 (4.44%) in the Tigray region, 4 (8.89%) in the Afar region, 2 (4.44%) in the Benishangul-Gumuz region, 2 (4.44%) in the Central Ethiopia region, and 3 (6.67%) in the Southwest region.
Regions with a high number of studies, such as the Oromia and Amhara regions, indicate that a larger number of studies were undertaken in these regions, suggesting that these regions are more closely aligned with agricultural science and technologies than the other regions. Within these regions, the availability of infrastructure, educated manpower, and the focus and commitment to change the sectors are high, which have made them leaders in the production of crops in the country.
Furthermore, fewer studies were conducted in the other cited regions in the table, indicating that these regions have a low focus on agricultural research and technology transfer issues. Therefore, they have low potential for crop production. These regions tend to focus more on the production of oil crops, cotton, vegetables, and livestock because of their comparative advantage.
Table 4 shows the distribution of the studies conducted in different parts of Ethiopia. In the Oromia region, technical efficiency-focused studies were conducted in 13 different areas, representing the largest number compared to the other regions. In the Amhara region, the studies were conducted in 11 study areas, making it the second largest after Oromia. In the Southwest region, technical efficiency-focused studies were conducted in only three areas, making it the third largest after the Oromia and Amhara regions. In these regions, the production potential of crops is better than in the rest of Ethiopia. However, efforts should be made to cover other untapped areas and narrow the existing production gaps in the regions.
Furthermore, in the Southern region, Afar region, Benishangul-Gumuz region, and Central Ethiopian region, technical efficiency-related studies were conducted in only two areas. This finding implies that these regions lack sufficient scientific information. In these regions, farmers conduct their production practices based on indigenous knowledge, which has been developed over time and has not been able to help them boost their crop production potential. This, in turn, adversely affects farmers’ production potential.
Based on the findings shown in Figure 2, the number of studies conducted by crop type is expressed as follows: barley (3), lentil (1), maize (8), malt barley (1), sorghum (3), teff (3), wheat (6), cassava (1), multiple crops (3), crop and livestock (2), faba bean (1), onion (2), potato (2), rice (2), sesame (1), soya bean (2), sweet potato (1), and tomato (3). The numbers in the parentheses indicate the number of studies associated with each crop type. Although there were many studies, they were selected based on the objective of this review.
Moreover, the cross-tabulation shown in Table 5 illustrates the selected studies targeting crops across regions. The results showed that a greater number of studies (about 11 studies) were conducted on maize products. Among these studies, eight were conducted in the Oromia region, two studies were conducted in the Afar region, and one study was conducted in the Amhara region. This implies that the regions, in general, and the Oromia region, in particular, have potential for maize production. The next most researched crop (approximately six studies) was wheat. The number of studies across the regions was distributed in the Oromia region (two studies), Amhara region (one study), Central Ethiopia region (one study), and Southwest region (one study).
The other more researched crops were barley (three studies), sorghum (three studies), teff (three studies), multiple crops (three studies), and tomato (three studies). These studies were distributed across different regions, such as the Amhara, Oromia, and Tigray regions. Other crops, such as cassava, sesame, soybean, and sweet potato, were studied in the south, Amhara, Benishangul, and southern regions.
Tables 6, 7 present the summary statistics of the data used in the meta-analysis. Table 6 compares the mean technical efficiency of two groups of smallholder farmers. According to the results, 75% of the farmers had a land size of less than or equal to 0.5 ha, with a standard deviation of 0.153. Moreover, the farmers with an average farm size of more than 0.5 ha had a mean technical efficiency of 69.6%, with a standard deviation of 0.144. The mean sample size used by the identified studies for the smaller land size holders (≤0.5 ha) was 152, with a standard deviation of 115.49, whereas the sample size was 142, with a standard deviation of 115.89, for the larger land size holders (>0.5 ha).
Table 7 shows the explanatory variables or moderators used in the meta-regression estimation. The summary statistics showed that, on average, the farmers used approximately five factors in crop production. These inputs were either predominantly local or unimproved. The use of local inputs and the difficulties farmers face in mixing production factors result in inefficiency.
Labor quality is another variable hypothesized to have an effect on the efficiency of farmers. The farmers had a mean labor quality of 1.531 qt/man-day, which implied that they had low production skills, meaning they needed many hours to produce more crops. This was due to the fact that in Ethiopia, farmers employed on the farm have low literacy levels, are far from the extension service, and face serious hindrances related to the unavailability and unaffordability of inputs, which limit their production potential. Similar finding was found by Tadesse et al. (2018), Tamirat et al. (2022), Teka et al. (2021), Tigabu et al. (2022), Tolesa (2022), Tsegaye et al. (2022), and Alula et al. (2021).
Regarding total income, the results indicated that the farmers had a total income of 215.029 birr per annum. Moreover, information contact plays a significant role in updating information about the market and production. Farmers can obtain information from development agents, fellow farmers, and the mass media. The results showed that the farmers had a mean number of information contacts of 22 per annum, with a standard deviation of 12. The high standard deviation recorded for this variable indicates that there is a disparity in information access among farmers, which is due to infrastructural problems, information literacy problems, carelessness, and awareness problems regarding the importance of scientific information.
A meta-analysis was conducted to determine the effect of land size on the technical efficiency of farmers. According to the forest plot in Figure 3, the farmers were technically more efficient under smaller land sizes (≤0.5 ha) compared to large land sizes (>0.5 ha). The farmers with smaller land sizes could improve technical efficiency by 21% compared to larger land size holders. The justification for this discrepancy is that smaller landholders rely on farming as their only means of livelihood, which leads them to take better care of their farms. These farmers have limited resource endowments, and farm activities are carried out by family members. Therefore, with a smaller land size, farmers can allocate sufficient amounts of seed, labor, and fertilizer per hectare and can also manage overall farm operations well.
However, the reasons why farmers are less efficient with larger land sizes can be understood in two aspects. First, smallholder farmers with larger land sizes struggle to allocate the recommended amount of factor inputs per hectare of land. This is due to poor knowledge about the issue, financial constraints, and poor follow-up on their farm because of labor shortage. The other reason is that even if farmers with larger land sizes have good resource endowments, most of them are engaged in other non-farm activities. Therefore, they do not take care of their farms as they spend much of their time doing other income-generating activities.
Output heterogeneity was detected from the results of the cumulative meta-analysis. Heterogeneity was detected using a chi-squared test (p < 0.01). Another option for indicating heterogeneity was the use of Q statistics. The test results presented in Table 8 showed that the data were heterogeneous, with a q-value of 817.167 and significance at the 1% probability level.
Heterogeneity suggests that the effect is not the same across studies or there is a variation in outcomes between studies. The heterogeneity was due to the variations in the study design, the variability in the participants, and the interventions used across the studies. Such methodological diversity indicates that the studies may have been affected by varying levels of bias. This means that factors such as the type of sample, sampling techniques, differences in the processing of interventions, and outcome variables were the causes of the heterogeneity observed.
Following the identification of heterogeneity, a meta-analysis was performed based on study-level characteristics. The variability among the studies in this systematic review, also known as heterogeneity, was reduced using four explanatory variables, commonly known as moderators in meta-analysis.
The indication of a reduction in heterogeneity was a decrease in the tau-squared value from 0.272 to 0.1347. Therefore, it can be confirmed that the variation among the studies was explained using moderators such as resource endowment, labor quality, information contact, and total income, as shown in Table 9.
The meta-regression results, as presented in Table 9, showed that resource endowment, labor quality, information contact, and total income have a significant effect on the dependent variable. The interpretation for each moderator is as follows:
• Resource endowment: This has a positive effect on the dependent variable at a probability level of <5%. A unit increase in production input increases the effect size by 11.3%, ceteris paribus. The justification for this is that the use of a greater number of production factors at the recommended rate is essential to boost efficiency because efficiency results from an optimal mix of production factors.
• Labor quality: This has a positive effect on the dependent variable at a probability level of less than 10%. A unit improvement in the labor quality of farmers increases the effect size by 7.3%, ceteris paribus. The reason for this is that qualified labor is skillful in managing every activity on the farm and can operate with a minimum cost of production.
• Information contact: This has a positive effect on the dependent variable at a probability level of less than 10%. A unit increase in extension contact increases the technical effect size by 0.9%, ceteris paribus. It is essential to reduce problems related to mixing inputs and improve the access and utilization of production factors appropriately.
• Total income: This has a positive effect on the dependent variable at a probability level of less than 5%. A unit increase in income increases the effect size by 0.1%, ceteris paribus. The reason for this is that the income generated from on-farm and farm activities is crucial for purchasing or hiring production factors based on the requirements of the available land size.
Therefore, addressing and solving the aforementioned factors is key to improving farmers’ efficiency individually and also has the potential to reduce efficiency differences among farmers.
A publication bias test was performed to determine whether relevant articles were included in the analysis. Moreover, the publication bias test is an indicator of the presence of a small study effect. The results of the publication bias test were presented using a statistical test called Begg’s test and a graph called a funnel plot.
The first step for testing publication bias was to conduct a statistical test using Begg’s test. As shown in Table 10, the test results for publication bias showed insignificant values, which confirmed that there was no publication bias. The insignificance of the test result was denoted by the probability value for publication bias, which was equal to 0.382 and was not significant. This finding implied that relevant studies were included in the meta-analysis.
The funnel plot shown in Figure 4 also confirmed the absence of publication bias. The funnel plot was symmetric with respect to the effect size measured by the standard mean difference (SMD). However, it is important to note that the funnel plot only shows the distribution of the studies in relation to the mean. Therefore, a comparable number of studies were included in the analysis. The presence of studies outside the 95% confidence limit was an indicator of heterogeneity among the studies.
In Ethiopia, the majority of farmers are smallholders, characterized by limited resource endowment, farm activities operated by family members, poor financial capacity, weak production skills, and production levels below potential. In the country, farmers have different efficiencies across different land sizes. Farmers are more efficient when operating on smaller land sizes as compared to larger land sizes. As smallholders, farmers cannot afford the purchase of the inputs required for their farms. Farming on smaller land sizes allows them to easily manage their farms and produce at a relatively lower cost.
Therefore,
• Improving farmers’ production skills, providing input subsidies, and upgrading development agents’ skills are crucial for boosting farmers’ efficiency.
• Strengthening public–private partnerships is essential because it can support farmers by improving the accessibility and availability of production input and knowledge.
• Designing policies to support the digitalization of the sector is crucial. Precision agricultural applications are important because they can support site-specific crop production management. Therefore, they can help lower the cost of production and optimize efficiency.
The raw data supporting the conclusions of this article will be made available by the authors, without undue reservation.
YG: Conceptualization, Data curation, Formal analysis, Funding acquisition, Investigation, Methodology, Project administration, Resources, Software, Supervision, Validation, Visualization, Writing – original draft, Writing – review & editing. AA: Resources, Validation, Visualization, Writing – review & editing.
The author(s) declare that no financial support was received for the research, authorship, and/or publication of this article.
The authors declare that the research was conducted in the absence of any commercial or financial relationships that could be construed as a potential conflict of interest.
All claims expressed in this article are solely those of the authors and do not necessarily represent those of their affiliated organizations, or those of the publisher, the editors and the reviewers. Any product that may be evaluated in this article, or claim that may be made by its manufacturer, is not guaranteed or endorsed by the publisher.
Adugna, A., Adeba, G., and Yadeta, B. (2023). Economic efficiency of smallholder farmers in wheat Producation: in Adiyo District, Kafa zone, southern nations nationalities of People’s region, Ethiopia. Acta Sci. Agricult. 7, 31–46.
Adugna, J., Shiferaw, F., Adane, T., Victor, M., and Thomas, A. (2017). Assessing the efficiency of sweet potato producers in the southern region of Ethiopia. Exp. Agric. 54, 1–16. doi: 10.1017/S0014479717000199
Agerie, W., Tigabu, K., and Abebe, K. (2019). Analysis of technical efficiency of potato (Solanum Tuberosum L.) production in Chilga District, Amhara National Regional State, Ethiopia. J. Econ. Struct. 8, 1–18. doi: 10.1186/s40008-019-0166-y
Alemayehu, B. (2021). Economic efficiency of wheat production: the case of Angecha District, southern Ethiopia. Glob. J. Agricult. Econ. Extension Rural Dev. 9, 1–7.
Ali, O., Amin, M., Michael, B., and Ataklty, H. (2022). Technical efficiency and firm heterogeneity in stochastic frontier models: application to smallholder maize farms in Ethiopia. J. Prod. Anal. 57, 213–241. doi: 10.1007/s11123-022-00627-2
Alula, T., Bekele, M., Abrham, B., Ermias, A., John, W., Philip, M., et al. (2021). Cassava production efficiency in southern Ethiopia: the parametric model analysis. Front. Sustain. Food Syst 2021, 1–12. doi: 10.3389/fsufs.2021.758951
Anbes, T. (2020). Technical efficiency of smallholder agriculture in developing countries: the case of Ethiopia. Economies 8, 1–27. doi: 10.3390/economies8020034
Anteneh, A., and Asrat, D. (2020). Wheat production and marketing in Ethiopia: review study. Cogent Food Agricult. 6:1778893. doi: 10.1080/23311932.2020.1778893
Belete, A. (2020). Analysis of technical efficiency in maize production in Guji zone: stochastic frontier model. Agricult. Food Secur. 9, 1–15. doi: 10.1186/s40066-020-00270-w
Beneberu, T., Belaineh, L., Jema, M., and Girma, K. (2018). Farm level efficiency of crop production in the central highlands of Ethiopia. Am. J. Rural Dev. 6, 49–58. doi: 10.12691/ajrd-6-2-4
Beshir, H. (2017). Technical efficiency measurement and their differential in wheat production: the case of smallholder farmers in south Wollo. Int. J. Econ. Bus. Finan. 4, 1–16.
Beyan, A., Jema, H., and Endrias, G. (2013). Analysis of farm Households' technical efficiency in production of smallholder farmers: the case of Girawa District, Ethiopia. J. Agricult. Environ. Sci. 13, 1615–1621. doi: 10.5829/idosi.aejaes.2013.13.12.12310
Birara, E., Mezgebu, A., Adugnaw, A., and Habtamu, M. (2023). Sources of wheat production technical inefficiency among smallholder farmers in northwestern Ethiopia: Beta regression approach. Cogent Econ. Finan. 11, 1–18. doi: 10.1080/23322039.2023.2208895
Birhanu, A., Jema, H., Mohammed, A., and Gebreegziabehr, F. (2020). Technical, allocative and economic efficiency of soya bean production: the case of smallholder farmers in Pawe District, Ethiopia. Ethiopian J. Econ. 29, 43–70.
Dagmawi, A. (2021). Analysis of technical efficiency of smallholder tomato producers in Asaita district, Afar National Regional State, Ethiopia. PLoS One 16:e0257366. doi: 10.1371/journal.pone.0257366
Dias, M., and Gustavo, J. (2020). Farm size and land use efficiency in the Brazilian Amazon. Land Use Policy 99, 13–25. doi: 10.1016/j.landusepol.2020.104901
Diriba, G. (2020). Agricultural and rural transformation in Ethiopia: Obstacles, Triggers and Reform Considerations Policy Working Paper.
Eskeziaw, M., Mengestu, K., Jemma, H., and Ketema, B. (2021). Production efficiency of major crops among smallholder farmers in Central Ethiopia. J. Agribus. Rural Dev. 3, 235–245. doi: 10.17306/J.JARD.2021.01391
Essa, C., Franklin, S., and Gideon, O. (2012). Factor productivity in smallholder Pigeonpea production systems: empirical evidence from northern Tanzania. J. Agricult. Econ. Dev. 1, 138–144.
FAO. (2023). The Global Network of Digital Agriculture Innovation Hubs. Available at: https://www.fao.org/in-action/global-network-digital-agriculture-innovation-hubs/countries/ethiopia/en.
Fisseha, Z., Abrham, S., and Dawit, A. (2022). Cereal production practices and technical efficiency among farm households in major “Teff” growing mixed farming areas of Ethiopia: a stochastic Meta-frontier approach. Cogent Econ. Finan. 10, 1–33. doi: 10.1080/23322039.2021.2012986
Gadisa, M., and Addisu, G. (2022). Technical, allocative, and economic efficiency of potato producers in Central Oromia, Ethiopia. Int. J. Bus. Econ. Res. 11, 158–165. doi: 10.11648/j.ijber.20221103.17
Gavaghan, D., Moore, A., and Mcqay, H. (2000). An evaluation of homogeneity tests in meta-analysis in pain using simulations of patient data. Pain 85, 415–424. doi: 10.1016/S0304-3959(99)00302-4
Genet, A. (2012). Rural land policy, rural transformation and recent trends in large-scale rural land acquisitions in Ethiopia. pp. 1–28.
Getachew, W., Lemma, Z., and Bosena, T. (2018). Economic efficiency of smallholder farmers in barley production in Meket District, Ethiopia. J. Dev. Agric. Econ. 10, 328–338. doi: 10.5897/JDAE2018.0960
Gidey, K., Dawit, G., and Kidane, T. (2021). Do smallholder farmers ensure resource use efficiency in developing countries? Technical efficiency of sesame production in Western Tigrai, Ethiopia. Heliyon 7, 1–8. doi: 10.1016/j.heliyon.2021.e07315
Gidey, E., Oagile, D., Reuben, S., Eagilwe, S., Amanuel, Z., Said, M., et al. (2023). Land use and land cover change determinants in Raya Valley, Tigray, northern Ethiopian highlands. Agriculture 13, 1–15. doi: 10.3390/agriculture13020507
Hagos, W. (2021). The determinants of technical efficiency of farmers in teff, maize and sorghum production: empirical evidence from central zone of Tigray region. Ethiop. J. Econ. 22, 1–36.
Hayatu, M. (2020). Efficiency analysis of crop production in Gurage zone: the case of Abeshige Woreda, SNNPR Ethiopia. Int. J. Environ. Agricult. Biotechnol. 5, 1338–1350. doi: 10.22161/ijeab.55.21
Higgins, J., Thompson, S., Deeks, J., and Altman, D. (2003). Measuring inconsistency in meta-analyses. Br. Med. J. 327, 557–560. doi: 10.1136/bmj.327.7414.557
Hika, W., and Afsaw, L. (2019). Analysis of productivity and efficiency of maize production in Gardega-Jarte District of Ethiopia. Int. J. Environ. Agricult. Biotechnol. 15, 180–193. doi: 10.5829/idosi.wjas.2019.180.193
Hosaena, H., and Holden, S. (2014). Efficiency and productivity differential effects of land certification program in Ethiopia: Quasi-experimental evidence from Tigray. ESSP Working Papers 64. International Food Policy Research Institute (IFPRI). 1–24. Available at: https://media.africaportal.org/documents/Agricultural_and_rural_transformation_in_Ethiopia.pdf.
Ingrao, C., Strippoli, R., Lagioia, G., and Huisingh, D. (2023). Water scarcity in agriculture: an overview of causes, impacts and approaches for reducing the risks. Heliyon 9, e18507–e18516. doi: 10.1016/j.heliyon.2023.e18507
Kebebew, H., Wolteji, B., and Milkessa, W. (2021). Economic efficiency of wheat producers: the case of Debra Libanos District, Oromia, Ethiopia. Turk. J. Agricult. Food Sci. Technol. 9, 953–960. doi: 10.24925/turjaf.v9i6.953-960.4244
Kifle, D., Getachew, B., and Galmesa, A. (2020). Economic efficiency of smallholder farmers in tomato production in BakoTibe District, Oromia region, Ethiopia. J. Agricult. Sci. Food Res. 11, 1–8. doi: 10.35248/2593-9173.20.11.273
Kusse, H., Engida, G., and Agegnehu, W. (2021). Does technical efficiency matter for Ethiopia’s Sorghum producer farmers? A study on its implication for productivity improvement. Ethiopian J. Econ. 29, 71–104.
Mengistu, A., Berihun, D., Jourdain, C., and Yismaw, B. (2024). Productivity and efficiency heterogeneity among maize smallholder farmers in Ethiopia. Cogent Food Agricult. 10, 1–12. doi: 10.21203/rs.3.rs-2199516/v1
Miao, B., Liu, Y., Fan, Y., Niu, X., Jiang, X., and Tang, Z. (2023). Optimization of agricultural resource allocation among crops: a portfolio model analysis. Land 12, 1–18. doi: 10.3390/land12101901
Milkessa, A., Endrias, G., and Fikadu, M. (2019). Economic efficiency of smallholder farmers in wheat production: in Abuna Gindeberet District, Oromia National Regional State, Ethiopia. Open Access J. Agricult. Res. 22, 65–75. doi: 10.15414/raae.2019.22.01.65-75
Musa, A., Lemma, S., and Endrias, G. (2015). Measuring technical, economic and allocative efficiency of maize production in subsistence farming: evidence from the central Rift Valley of Ethiopia. Appl. Stud. Agribusiness Commerce 9, 63–73. doi: 10.19041/APSTRACT/2015/3/9
PDC (2020). The Pathway to Prosperity Ten Years Perspective Development Plan (2021–2030). Available at: https://faolex.fao.org/docs/pdf/eth215704.pdf.
Regasa, D., Mesay, Y., and Adam, B. (2019). Analysis of production efficiency, productivity variances and resource allocation among smallholder farmers of soybean producers: evidence from BenishangulGumuz region Ethiopia. Int. J. Res. Stud. Agricult. Sci. 5, 18–36. doi: 10.20431/2454-6224.0507003
Sime, S., Jema, H., Mengestu, K., and Million, S. (2022). Technical, allocative and economic efficiency of malt barley producers in Arsi zone, Ethiopia. Cogent Food Agricult. 8, 1–17. doi: 10.1080/23311932.2022.2115669
Tadesse, J., Leta, T., Techale, B., and Lemi, B. (2018). Genetic variability, heritability and genetic advance of maize (Zea Mays L.) inbred lines for yield and yield related traits in southwestern Ethiopia. J. Plant Breed. Crop Sci. 10, 281–289. doi: 10.5897/JPBCS2018.0742
Tadesse, Z., Solomon, A., and Fentahun, A. (2017). Analysis of the technical efficiency of Rice production in Fogera District of Ethiopia: a stochastic frontier approach. Ethiopian J. Econ. 26, 90–108.
Tamirat, F., Mekonnen, S., Yared, D., and Ali, M. (2022). Technical efficiency of irrigated onion and tomato production in the central Rift Valley of Ethiopia. Ethiop. J. Agric. Sci. 32, 148–162.
Teka, E., Osman, R., and Yadeta, B. (2021). Resource use efficiency of smallholder maize producers’ in Bilo Nopa District, Ilu Ababor zone, Oromia region, Ethiopia: an application of stochastic frontier analysis. Ethiopian J. Appl. Sci. Technol. 13, 94–103. doi: 10.13140/RG.2.2.10607.56489
Tekleyohannes, H., Berhanu, G., and Tewodros, T. (2024). Technical inefficiency of smallholder wheat production system: empirical study from northern Ethiopia. Ethiopian J. Econ. 27, 151–171. doi: 10.22004/ag.econ.343219
Tigabu, D., Abebe, D., and Taye, M. (2022). Analysis of technical efficiency of irrigated tomato production in North Gondar zone of Amhara regional state, Ethiopia. Springer 15, 599–620. doi: 10.21203/rs.3.rs-150323/v1
Tolesa, T. (2022). Determinants of production efficiency of maize-dominated farmers in Western parts of Ethiopia in Gudeya Bila District: evidence under shifting cultivation area. Sci. World J. 2022, 1–6. doi: 10.1155/2022/3355224
Tolesa, T., Temesgen, K., and Zekarias, S. (2019). Economic efficiency of smallholder farmers in maize production in Gudeya Bila District, Oromia National Regional State, Ethiopia: parametric approach. J. Appl. Agricult. Econ. Policy Anal. 2, 1–7. doi: 10.12691/jaaepa-2-1-1
Tsegaye, M., Mebratu, A., Amsalu, M., and Nesre, K. (2022). Economic efficiency of smallholder farmers in Rice production: the case of Guraferda Woreda, southern nations nationalities People's region, Ethiopia. Int. J. Agricult. Innov. Res. 8, 151–167.
Wudineh, G., and Endrias, G. (2016). Technical efficiency of smallholder wheat farmers: the case of Welmera District, Central Oromia, Ethiopia. J. Dev. Agric. Econ. 8, 39–51. doi: 10.5897/JDAE2015.0660
Keywords: effect model, efficiency, land size, smallholders, meta-analysis, crop, Ethiopia
Citation: Girma Asefa Y and Ayalew Muluken A (2024) Land size and efficiency in agriculture: the case of Ethiopian smallholder farmers. A meta-analysis. Front. Sustain. Food Syst. 8:1447186. doi: 10.3389/fsufs.2024.1447186
Received: 11 June 2024; Accepted: 26 September 2024;
Published: 24 October 2024.
Edited by:
Cosmas Parwada, Midlands State University, ZimbabweReviewed by:
Lovemore Musemwa, Bindura University of Science Education, ZimbabweCopyright © 2024 Girma Asefa and Ayalew Muluken. This is an open-access article distributed under the terms of the Creative Commons Attribution License (CC BY). The use, distribution or reproduction in other forums is permitted, provided the original author(s) and the copyright owner(s) are credited and that the original publication in this journal is cited, in accordance with accepted academic practice. No use, distribution or reproduction is permitted which does not comply with these terms.
*Correspondence: Yohannes Girma Asefa, Z2lybWF5b2hhbm5lczg2QGdtYWlsLmNvbQ==
Disclaimer: All claims expressed in this article are solely those of the authors and do not necessarily represent those of their affiliated organizations, or those of the publisher, the editors and the reviewers. Any product that may be evaluated in this article or claim that may be made by its manufacturer is not guaranteed or endorsed by the publisher.
Research integrity at Frontiers
Learn more about the work of our research integrity team to safeguard the quality of each article we publish.