- 1School of Economics and Administration, Xi'an University of Technology, Xi'An, China
- 2School of Microelectronics, Xidian University, Xi'An, China
Climate change is significantly and enduringly impacting global agricultural supply chains (ASCs), underscoring the urgent need to enhance their climate resilience. This study aims to identify key strategies for building agricultural supply chain resilience (ASCRes) in the context of climate change and their interrelationships. Through a comprehensive literature review and expert consultations, a framework of 12 strategies was developed. Using survey data from 312 Chinese ASC companies, BP-DEMATEL-ISM and PLS-SEM methods were employed to conduct causal analysis and factor level evaluation. The results indicate that “diversification of agricultural products and supply chain (SC) networks,” “Agriculture 4.0 and digital transformation,” and “taking proactive climate action” are critical strategies to enhance ASCRes in the context of climate change. These findings can provide theoretical basis for farmers, ASC companies, and governments to cope with the impact of climate change, so as to build more robust ASC systems.
1 Introduction
ASCs are a key component of global food security and economic stability, as well as a crucial link in promoting rural economic development and increasing farmers' incomes. Their efficient operation depends on close collaboration and coordination among multiple nodes (Tendall et al., 2015; Stone and Rahimifard, 2018; Zhao et al., 2020). With the acceleration of globalization and the increasing complexity of environmental conditions, traditional approaches to ASC management are facing significant challenges and are in urgent need of innovation and transformation. In particular, climate change has become one of the major factors affecting the stability and efficiency of ASCs, compelling practitioners to reassess and adapt their management strategies and practices (Anderson et al., 2020; Godde et al., 2021). Uncertainties associated with climate change and extreme weather events, such as droughts, floods, high temperatures, and storms, not only directly affect agricultural production, but also have potential ripple effects on various nodes of the supply chain (SC) (Alam et al., 2024; Marcucci et al., 2024). To address these challenges, enhancing the climate resilience of ASCs has become a crucial research topic, i.e., how to maintain the stability and efficient functioning of SCs in the face of climate shocks (Sá et al., 2019; Davis et al., 2021).
For ASCs, considering the interactions between humans, technological systems, and the natural environment is paramount in building supply chain resilience (SCRes), due to the fact that the upstream cultivation activities often play a pivotal role in ensuring SC continuity (Aboah et al., 2019). Unlike other industries, ASCs are exposed to distinct challenges, including seasonal production, biodiversity considerations and impact of natural resource scarcity. These factors render existing SCRes research frameworks less directly applicable to the agricultural sector. Due to this, research addressing ASCRes remains in its nascent stages. Over the past few years, the majority of studies have concentrated on enhancing ASCRes in the context of the COVID-19 pandemic (Coopmans et al., 2021; Perrin and Martin, 2021; Blessley and Mudambi, 2022; Kumar and Kumar, 2022; Mishra et al., 2022), to help ASCs recover from barriers as quickly as possible. Relatively scant research has urged ASC practitioners to pivot toward addressing the adverse impacts of climate change (Davis et al., 2021) and fostering ASCRes as a strategy to mitigate climate risks, all while striving to achieve sustainability objectives (Macfadyen et al., 2015; Buitenhuis et al., 2022; Ribašauskiene et al., 2024).
Meanwhile, research focus has gradually shifted from conceptualizing resilience to analyzing its intrinsic factors. It's widely acknowledged that resilience comprises various “strategies,” akin to response cards addressing diverse management gaps (Christopher and Peck, 2004; Kamalahmadi and Parast, 2016; Ali et al., 2017; Hosseini et al., 2019). In the field of ASC research, ASCRes is clearly defined and widely accepted as per the studies by Tendall et al. (2015) and Stone and Rahimifard (2018). Aboah et al. (2019) argued, from a perspective that focuses on human-nature interactions, that flexibility, collaboration, adaptability, and resourcefulness are key strategies for assessing resilience in ASCs at the level of individual chain participants. Buitenhuis et al. (2022) provided a climate change resilience framework for the agricultural sector, proposing key strategies such as climate risk management, adaptive practices, diversification, and natural resource management. However, research on designing ASCRes strategies remain relatively limited, particularly in the context of climate change.
In this study, we creatively propose the BP-DEMATEL-ISM (Back Propagation neural networks-Decision-Making Trial and Evaluation Laboratory-Interpretative Structural Modeling) method, which introduces BP neural networks to enhance the theoretical framework for developing ASCRes in the face of climate change. Specifically, the BP-DEMATEL-ISM method quantifies the relationships between strategies by integrating BP neural networks, thus overcoming the limitations of the original DEMATEL method that relies on human subjectivity and capability. This approach accurately represents the complex relationships between strategies, improving the accuracy and reliability of the analysis. Following the BP-DEMATEL method, we used ISM to further analyze the results and establish a strategy hierarchy, leading to valuable measures and suggestions for strengthening ASCRes. The study concluded that diversification of agricultural products and SC networks is a crucial strategy for developing climate resilience in ASCs, followed by Agriculture 4.0 and digital transformation, and taking proactive climate action. In addition, we also tested the influence degree of each strategy on SCRes performance through PLS-SEM analysis, and compared and mutually verified with the results of BP-DEMATEL-ISM. The results showed that the two presented a high degree of consistency. This proves the effectiveness of the BP-DEMATEL-ISM method and shows the huge application potential of the method.
The rest of the paper is organized as follows: Section 2 reviews the previous literature and summarizes the definition and types of strategies for building ASCRes. Section 3 analyzes and defines the key strategies for enhancing ASCRes under climate change. Section 4 demonstrates the methodology framework adopted in this study, detailing the key steps of BP-DEMATEL, ISM, and questionnaire data collection. Section 5 processes and analyzes the results. Section 6 discusses the results, presenting theoretical contributions and Managerial implications. Finally, Section 7 summarizes the conclusions, highlights the limitations, and suggests directions for future research.
2 Literature review
2.1 Dynamic capability theory
Dynamic Capability Theory (DCT) originated from the Resource-Based View (RBV), which posits that a company's resources and capabilities should be valuable, rare, inimitable, non-substitutable, and conducive to achieving sustained competitive advantage (Wernerfelt, 1984; Barney, 1991). However, as RBV adopts a static perspective and primarily focuses on analyzing companies' internal resources and capabilities, it ignores the influence of resource changes, capability evolution, and external environmental fluctuations on companies' competition (Eisenhardt and Martin, 2000; Wang and Ahmed, 2007). To better elucidate companies' competitive advantage and sustained competitiveness in dynamic environments, Teece et al. (1997) proposed DCT, defining dynamic capabilities as “the company's ability to integrate, build, and reconfigure internal and external competences to address rapidly changing environments.” DCT underscores how companies can shape and adapt to the external landscape through continuous learning, innovation, and transformation to attain competitive advantage. How companies maintain and enhance competitive advantage, and thus capture value, is a key concern for both practitioners and academics, leading to an increasingly widespread interest in DCT (Ambrosini and Bowman, 2009). Numerous studies have explored the concept of SCRes as a dynamic capability (Chowdhury and Quaddus, 2017; Yu et al., 2019; Zhao et al., 2023). SCRes equips SC with the capacity to swiftly adapt and adjust, learn and innovate, integrate and synergize, and continuously improve. This enables SC to efficiently absorb, respond, and recover from disruptions, and consistently improve competitiveness and adaptability, thus maintaining robustness and competitive advantage in a changing market (Kamalahmadi and Parast, 2016; Ali et al., 2017; Yu et al., 2019; Zhao et al., 2023).
2.2 Definition of ASCRes
Two prominent perspectives for comprehending “resilience” exist, namely engineering resilience and ecological resilience (Novak et al., 2021; Wieland and Durach, 2021). Engineering resilience refers to a system's capability to revert to equilibrium or a stable state following a disturbance, emphasizing the restoration of the initial equilibrium promptly. Ecological resilience refers to the magnitude of the disturbance that can be absorbed by a system before it changes its structure, with a focus on determining the optimal equilibrium, which may be a return to the original equilibrium, or a new equilibrium (Fiksel, 2003; Folke, 2006). Since both engineering and ecological resilience definitions operate within the framework of closed systems, overlooking external shocks such as social, environmental, and economic disruptions. Consequently, a third definition, adaptive resilience, has been proposed. Adaptive resilience diverges from equilibrium-focused approaches, emphasizing instead adaptive responses to the variable external environment (Kamalahmadi and Parast, 2016). Tendall et al. (2015) asserted that unlike traditional resilience strategies which prioritize enhancing organizational competitiveness, improving resilience in ASCs prioritizes withstanding disruptions to ensure the safe and appropriate provision of food to end consumers. This implies that the prevailing, widely accepted definitions, elements, and strategies of resilience must undergo adaptation when applied to ASCs. Based on this, Stone and Rahimifard (2018) defined resilience as “the collective ability of Agri-food SC stakeholders to ensure acceptable, sufficient and stable food supplies, at the required times and locations, via accurate anticipation of disruptions and the use of strategies which delay impact, aid rapid recovery and allow cumulative learning postdisruption.” This definition has been widely accepted and adopted recently in research related to ASCRes.
2.3 Strategies of ASCRes
Existing studies have used various terms in describing SCRes components, such as enhancers (Shekarian and Mellat Parast, 2021), capabilities (Ali et al., 2017; Han et al., 2020), elements (Christopher and Peck, 2004; Stone and Rahimifard, 2018), metrics (Shashi et al., 2019; Behzadi et al., 2020), strategies (Tukamuhabwa et al., 2015), drivers (Hosseini et al., 2019), and others. In this study, the term “strategies” is used.
The necessity to define the connotation of SCRes and to develop measurement methods has rendered the investigation of its constituent strategies one of the broadest and deepest subjects in the field of SCRes. Among numerous strategies, flexibility, redundancy, agility, collaboration, and visibility have been viewed as five of the most important (Balezentis et al., 2023). Nonetheless, it's imperative to note that these strategies are not fixed across various types of SCs and industries. Stone and Rahimifard (2018) emphasized that ASCRes should be more related to the interactions and relationships among organizations, communities, and the natural environment, in the meanwhile considering the adaptive capacity of ASCs. Common resilience strategies may not necessarily apply to ASCs, particularly in special shock situations such as COVID-19 pandemic or climate change. Table 1 summarizes representative ASCRes studies in recent years, which can help understand the specificity of resilience strategies in ASC.
3 Key strategies for building ASCRes
In order to identify the key strategies of building ASCRes under climate change, this study conducted a comprehensive literature review using Google Scholar and Web of Science. Given the limited literature specifically discussing ASCRes in the context of climate change, the study sought insights from endeavors to develop resilience in alternative shock contexts (e.g., COVID-19 pandemic, geopolitical conflicts) and diverse industries (e.g., manufacturing, energy, chemical industries). The search keywords used are: “Supply chain AND Resilien*; Climate change AND Supply chain AND resilien*; agri* supply chain AND resilien*; agri* supply chain AND resilien* AND Climate change.” The strategy types were refined and finalized after discussions with experts involved in agricultural supply chain management (SCM). After screening, 12 key strategies for ASCRes under 3 dimensions were identified from the relevant literature (Figure 1).
According to Figure 1, this paper constructs a novel framework of ASCRes consisting of three perspectives: General strategies, strategies for agriculture, and strategies for climate change response. Firstly, the analysis commences from the overarching SC dimension, which transcends industry-specific boundaries, to identify common resilience strategies. Secondly, focusing on common resilience strategies in agricultural sector, taking into account that the industry's distinct requirements and strategies in confronting disruptions. Finally, attention shifts to ASCRes under climate change. Here, specialized resilience strategies are sought to address climate-related shocks, with a focus on overcoming barriers to climate uncertainty. This gradually deepening analytical approach enables a thorough exploration and optimization tailored to industry and shock conditions, enhancing the framework's reliability.
3.1 General strategies
3.1.1 Information sharing and resource integration (G1)
Effective information sharing across various segments of the SC enables a swift understanding of market demand fluctuations, supplier status, and logistics dynamics. This capability allows for timely adjustments in production plans and strategies, thereby mitigating delays and losses caused by information asymmetry (Scholten and Schilder, 2015). Jointly planning and resource management among all SC parties can prevent resource wastage and overstocking, enhancing resource utilization and operational efficiency (Juan et al., 2022). In the face of disruptions, collaborative SC partners can share risks and mitigate the impact of unexpected events on individual nodes (Duong and Chong, 2020). Additionally, information sharing and resource integration can also foster innovation and improvement. Collaboration and joint learning enhance innovation awareness and experience sharing, driving ongoing optimization and improvement of the SC. This, in turn, boosts overall competitiveness and adaptability (Al-Omoush et al., 2023).
3.1.2 Rapid recognition and timely response (G2)
Regular environmental monitoring enhances the ability to detect early warning signals, thereby improving risk recognition efficiency. Swift risk diagnosis and the implementation of preselected contingency plans significantly mitigate the impact of disruptions (Bode and Macdonald, 2017). Timely responses to disruptions require adaptability, flexibility, agility, and resilience, managers fine-tune these dimensions by optimizing the alignment between products, strategies, and expectations to achieve the appropriate level of responsiveness (Gligor et al., 2019; Richey et al., 2022). Rapid perception and timely responses ensure SC continuity and on-time product delivery, crucial for ensuring robustness, operational efficiency, and sustained competitiveness.
3.1.3 Dynamic adjustments for continuous operation (G3)
SCs need to possess the capability to swiftly modify production processes, adjust supplier relationships, promptly fulfill customer orders, and flexibly cater to varying market demands and evolving conditions (Christopher and Peck, 2004; Huo et al., 2018). This facilitates rapid adaptation to market fluctuations, thereby mitigating the risks of inventory excess or stock-outs (Bai et al., 2020). Through dynamic adjustments to production and supply plans, SCs can effectively respond to unforeseen events or disruptions, thereby ensuring robust operational continuity (Shekarian et al., 2020; Kamalahmadi et al., 2021).
3.1.4 Backup resources and contingency plans (G4)
Backup resources and contingency plans play a crucial role in building SCRes, ensuring business continuity in the face of challenges or unforeseen circumstances. For instance, in cases of critical supplier issues or natural disasters affecting logistics routes, the swift mobilization of backup resources and corridors prevents production disruptions or SC breakdowns. Simultaneously, backup resources and contingency plans can help reduce supply and environmental risks linked to single points of failure, enhancing overall stability and reliability (Kamalahmadi and Parast, 2017).
3.2 Strategies for agriculture
3.2.1 Transparency and predictive capacity (A1)
Emerging technologies such as AI (artificial intelligence), IoT (Internet of Things), blockchain and big data analysis are propelling traditional ASCs toward a data-driven digital environment, necessitating organizations to attain a high level of visibility (Kamble et al., 2020). ASCs are inherently characterized by seasonal or cyclical fluctuations in supply and demand, along with the storage challenges of perishable products. Data transparency facilitates more accurate demand prediction and market trend analysis, enabling timely adjustments to production plans and inventory management (Kamble et al., 2020). Moreover, ASCs are significantly impacted by natural disasters, climate change, environmental regulations, and other factors, resulting in high levels of uncertainty. Enhanced forecasting capabilities are essential for implementing timely countermeasures to ensure stable operations (Lezoche et al., 2020).
3.2.2 Agriculture 4.0 and digital transformation (A2)
Digital transformation emerges as a transformative factor in agriculture (Kazancoglu et al., 2024). Effective data management is one of the core objectives of Industry 4.0, encompassing the collection, analysis, and integration of information technology, manufacturing, and operational systems to enable more timely, rapid, and flexible data access (Brettel et al., 2014). Thus, the infusion of Industry 4.0 technologies has the potential to revolutionize agricultural systems (Shepherd et al., 2020). The influence of Industry 4.0 on ASCs primarily lies in the digitalization of processes and services through ICT-based methods or tools (Yadav et al., 2022). The embedding of digital technologies substantially decreases uncertainty, and the accurate acquisition and processing of data enhance perception, prediction, decision-making, and adaptive capacities (Lezoche et al., 2020).
3.2.3 Continuous learning and knowledge management (A3)
ASCs not only need to enhance their capacity to cope with external shocks, but also consider the accessibility and stability of food supply. Therefore, in bolstering resilience capacity, the focus should extend beyond response and recovery capabilities to encompass the reinforcement of anticipation and learning from disruptions (Hohenstein et al., 2015; Stone and Rahimifard, 2018). Advances in information technology, effective collaborative cultures, and the presence of core companies, foster the generation, dissemination, and application of knowledge (Umar et al., 2021). As the digitization of ASCs in the context of Industry 4.0 continues to evolve, there arises a need to enhance the process of translating vast amounts of data into knowledge. Understanding how knowledge can be extracted, shared, and utilized assumes increasing significance in this regard (Schniederjans et al., 2020).
3.2.4 Government support (A4)
As a distinct industry, agriculture is more dependent on government support. It is profoundly influenced by natural environment, engendering a high degree of uncertainty and risk. The government plays a pivotal role by providing support in terms of resource allocation, assistance during interruptions, and infrastructure development (Zhong et al., 2024). Serving as the main body for industry regulation and policy formulation, the government can standardize and guide the development of ASCs through policies and regulations. Additionally, governments can bolster the development of ASCs through economic instruments such as financial injections, tax incentives, and subsidized programs. These supports enable ASCs to upgrade technical equipment, improve production processes, and enhance product quality, thereby increasing competitiveness and resilience.
3.3 Strategies for climate change
3.3.1 Climate change awareness (C1)
Before SC managers can undertake climate change adaptation or mitigation efforts, they must gain a comprehensive awareness of the intricate relationship between climate change and business operations (Pesonen and Horn, 2014). This awareness facilitates a focused and thorough assessment of climate change adaptation strategies, leading organizations to take a proactive stance in climate action (Todaro et al., 2020). The efficacy of climate change adaptation significantly depends on community awareness levels and agricultural practitioners' perceptions of climate change impacts (Ado et al., 2019). Investing in climate change awareness represents a valuable endeavor for the agricultural sector, enabling it to better manage climate risks, adapt to climate change, and capitalize on future opportunities (Marshall et al., 2013).
3.3.2 Diversification of agricultural products and SC networks (C2)
In the context of escalating climate change pressures, the expansion of diversified agricultural systems emerges as an effective strategy for enhancing resilience (Lin, 2011). Diversification encompasses both SC structure diversification and agricultural product diversification. Managers should mitigate reliance on single-source supply channels (Park et al., 2013), e.g., choosing a supplier portfolio with minimal climate vulnerability, opting for alternative suppliers with limited climate risk exposure (Pankratz et al., 2024), and prioritizing green suppliers (Wong, 2020). Diversification of agricultural products reduces the risk of a particular crop loss due to climatic hazards or diseases. It contributes to the preservation of soil health and ecological balance, curbing excessive soil nutrient depletion and environmental pressure associated with monoculture practices (Lin, 2011).
3.3.3 Taking proactive climate action (C3)
Proactive climate action plays a pivotal role in enabling ASCs to swiftly address the challenges posed by climate change. Through initiatives like crop enhancement, land management improvements, and optimization of SC networks, they can more flexibly respond to climate-induced risks such as extreme weather events, diseases, and disasters, thereby enhancing food security and reliability (Anderson et al., 2020). Positive climate action can also stimulate innovation. In the face of climate change, agricultural systems need to continually explore novel cultivation methods, technological tools, resource utilization forms, and SC management paradigms. Embracing adaptive strategies can catalyze innovation and propel ASCs toward smarter, more efficient, and sustainable development, exemplified by the adoption of climate-smart agriculture (CSA) (Azadi et al., 2021).
3.3.4 Sustainable development orientation (C4)
Sustainable development orientation refers to the management of SC operations, resources, information, and finances to optimize profitability while minimizing environmental footprints and maximizing social welfare (Hassini et al., 2012). For the economy, sustainability practices hold the potential to curtail production costs by minimizing resource wastage and energy usage. For the environment, sustainability practices facilitate the efficient utilization of resources, encompassing initiatives like recycled agriculture, water-saving irrigation, energy conservation, and emissions reduction (Zeweld et al., 2017). For society, through measures such as promoting rural economic development, improving the living standards of farmers, and fostering the welfare of rural communities (Kamble et al., 2020), sustainability practices enhance the overall stability and resilience of the ASC system.
4 Methodology
To achieve the research objectives, the methodology framework presented in Figure 2 was followed.
4.1 BP-DEMATEL methodology
DEMATEL (Decision-Making Trial and Evaluation Laboratory) is a qualitative and quantitative analysis method used to analyze the degree of causation and influence among elements within a system (Gabus and Fontela, 1972). By examining the logical relationships and direct influence matrix, it calculates the influence and impact of each element on others, thereby determining the cause and centrality of each element. This serves as the foundation for constructing a model that clarifies the causal relationships and status of each element within the system. The traditional DEMATEL method derives the direct influence matrix through expert opinion collection and analysis, which has two main limitations: (1) Expert opinions are often influenced by their research field and experience, introducing a degree of subjectivity (Qin et al., 2020); (2) Experts may struggle to analyze complex nonlinear, multistage, or loop relationships among factors due to their limitations. To address these limitations, we propose integrating BP neural networks. BP (Back Propagation) neural networks are a type of feedforward neural network capable of modeling complex nonlinear relationships through design and nonlinear activation functions, suitable for various complex classification and regression problems. In this study, we trained a BP neural network to function as an expert in DEMATEL. Firstly, the BP neural network is devoid of subjectivity, overcoming the bias inherent in expert opinions. Secondly, the BP neural network's strong fitting and generalization capabilities allow it to capture complex relationships between elements, thereby presenting a more accurate depiction of real-world relationships. In summary, compared to traditional DEMATEL, the BP-DEMATEL method overcomes its deficiencies, providing a more realistic assessment of causal relationships and the positions of elements within the system.
Based on this, this paper proposes the following steps for BP-DEMATEL:
STEP 1: Constructing a matrix of influencing factors x = (xij)m×n (i = 1, 2, …, m; j = 1, 2, …, n) and a matrix of target factors y = (yiq)m×k(q = 1, 2, …, k), where m is the sample size, n is the number of influencing factors, and k is the number of outcome factors. Normalizing x and y yields and ȳ.
STEP 2: Train the BP neural network by using and ȳ as the input and output vectors, respectively. Calculate the weight matrix wn×g between the input and hidden layers and the weight vector wg×k between the hidden and output layers, where g is the number of neurons in the hidden layer. The design of the hidden layer and the number of neurons within it require an optimal balance between computational cost and predictive accuracy.
STEP 3: Calculate the overall weight vector:
Where the mean function is used to take the average of each column of |W|*|w|, when the number of rows of |W|*|w| is greater than one.
STEP 4: Calculate the direct influence matrix A of the influencing factors:
Where aii = 0, aij = ωi/ωj(if ωj = 0, then aij = 0) is the degree of direct influence of factor i on factor j.
STEP 5: Normalizing the direct influence matrix A yields the matrix B:
STEP 6: Calculate the comprehensive influence matrix T:
Where (I−B)−1 is the inverse matrix of I−B and I is the identity matrix.
STEP 7: Define as the sum of the rows of T and R as the sum of the columns of T. Then:
Where Di represents the degree of influence, i.e., indicating how much factor i influences other factors, while Ri represents the degree of being influenced, signifying how much other factors influence factor i. Di+Ri is the centrality degree of factor i, reflecting its relative importance in the system. A higher centrality degree suggests greater importance. Di−Ri is the causality degree of factor i, indicating the extent to which factor i is associated with other factors. A value >0 suggests that factor i has a stronger influence on other factors, classifying i as a cause factor. Conversely, a value < 0 suggests that other factors exert a greater influence on factor i, categorizing i as an effect factor.
STEP 8: Using Di+Ri as the horizontal axis and Di−Ri as the vertical axis, create a causal diagram to identify key factors.
4.2 ISM methodology
ISM (Interpretive Structural Modeling) is a method used to analyze and solve problems in complex systems (Warfield, 1974). ISM first divides the system into various subsystems (factors, elements) and then analyzes the factors and their direct binary relationships. This conceptual model is mapped into a directed graph, and the system's structure is revealed through Boolean logic operations, presented in a hierarchical directed topological graph without losing the system's overall function (Attri et al., 2013). Compared to tables, words, and mathematical formulas, ISM presents conclusions in a hierarchical topology diagram, offering an intuitive display. This allows for a clear understanding of the causal levels and ladder structure of system factors, making ISM a mainstream analysis method. More importantly, since the reachability matrix of ISM and the comprehensive influence matrix of DEMATEL contain almost the same information, both of which are direct relationships between various factors in the system, the reachability matrix of ISM can be obtained directly from the comprehensive influence matrix of DEMATEL through simple conversion, which greatly reduces the threshold of the use of ISM analysis method and improves the analysis efficiency.
Therefore, the combination of ISM analysis method and BP-DEMATEL in the above section can, on the one hand, transform the causal diagram into a hierarchy diagram, providing a more systematic and structured research result; on the other hand, the comprehensive impact matrix of BP-DEMATEL can be used to directly transform the reachable matrix of ISM. It can improve the comprehensiveness of the research while avoiding the sharp increase in time cost.
The steps of ISM are as follows:
STEP 1: Calculate the overall influence matrix H based on the comprehensive influence matrix T and the identity matrix I:
STEP 2: Calculate the mean value of all elements in the comprehensive influence matrix T as the threshold value λ. Convert the overall influence matrix H to a reachability matrix K based on the following rules:
Element kij denotes the reachability from strategy i to strategy j. A value of kij = 1 signifies that strategy i can reach strategy j directly or indirectly, whereas kij = 0 indicates that strategy i cannot reach strategy j either directly or indirectly.
STEP 3: Calculate the Reachability set and the Antecedent set:
Where R(xi) is the set of factors corresponding to the columns of kij = 1 in the rows corresponding to factors xi in the reachability matrix K. Q(xi) is the set of factors corresponding to the rows of kji = 1 in the columns corresponding to factors xi in the reachability matrix K. If R(xi)∩Q(xi) = R(xi) is satisfied, it indicates that all factors in Q(xi) can find antecedents in R(xi). Then factor xi is the highest level element.
STEP 4: Delete the rows and columns corresponding to factor xi from the reachability matrix K and continue with the judgment under the principle of R(xi)∩Q(xi) = R(xi) to extract the highest level factor. The process is repeated until all rows and columns are removed.
STEP 5: Draw the hierarchical model.
4.3 Data collection
This study ensured an accurate reflection of management and operations by surveying a broad sample of middle and senior managers from Chinese ASC companies. Variables were measured using established questionnaires from prior studies, with minor adjustments tailored to this study's specifics (Appendix Table 1). All measurements utilized a 7-point Likert scale, spanning from “1—very non-compliant” to “7—very compliant.” To fulfill the prediction requirements of the BP neural network model, an additional outcome variable, “SCRes,” was introduced to assess subjects' SCRes performance. To enhance the questionnaire's quality, a pre-test questionnaire design preceded the final questionnaire formation. To ensure data sources diversity, various channels, including mail, online, and offline surveys, were utilized. Out of 350 distributed questionnaires, 312 were successfully recovered and utilized for data analysis after screening and cleaning to maintain data quality. Preliminary data analysis was conducted using SmartPLS 4. Table 2 provides demographic information on subjects.
5 Result analysis
5.1 BP-DEMATEL results analysis
In the specific forecasting task, we use data from 12 ASCRes strategies as the input matrix and SCRes performance data as the output matrix. Based on this, we designed a BP neural network model with 12 input neurons and 1 output neuron. Drawing on BP neural network design principles and insights from the references, we optimized the model by adjusting three hyper-parameters: the number of neurons in the hidden layer, the selection of activation functions, and the learning rate. Given the dataset size and the number of input and output units, we designed a single hidden layer with 5 neurons to balance prediction accuracy and computational efficiency. To address the gradient vanishing problem associated with the Sigmoid activation function, we selected the Rectified Linear Unit (ReLU) for its simplicity and efficiency. Since grid search and learning rate decay methods require extensive training iterations and significant time investment, we implemented an automatic learning rate selection mechanism to dynamically adjust the learning rate according to the training stage, thereby enhancing training efficiency and performance. The Mean Squared Error (MSE) function was used as the loss function during training due to its robustness in handling outliers and suitability for regression tasks (Efron, 1986).
The designed BP neural network model is trained and deployed by Matlab. The overall weight vector is calculated using Equation (1): ω = (0.1021, 0.2387, 0.1861, 0.1249, 0.2909, 0.0632, 0.3086, 0.1638, 0.1659, 0.5572, 0.0732, 0.1141) The direct influence matrix A is calculated (Table 3). Following normalization based on Equation (2) and calculation of the comprehensive influence matrix T according to Equation (3) (Table 4).
Subsequently, using Equation (4), (5), and (6), the degree of influence (D), the degree of being influenced (R), the centrality degree (D+R), and the causality degree (D−R) of each strategy were computed (Table 5).
The causal diagram is constructed (Figure 3).
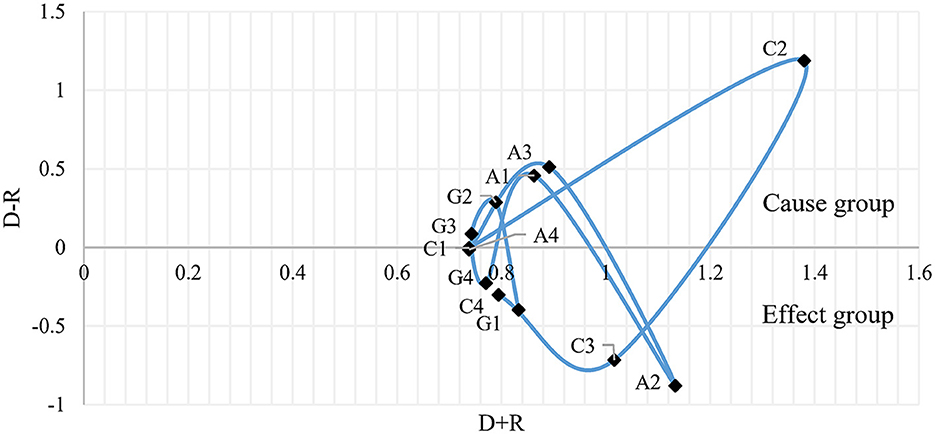
Figure 3. The overall causal diagram. G1-Information sharing and resource integration; G2-Rapid recognition and timely response; G3-Dynamic adjustment for continuous operations; G4-Backup resources and contingency plan; A1-Transparency and predictive capacity; A2-Agricultural 4.0 and digital transformation; A3-Continuous learning and knowledge management; A4-Government support; C1-Climate change awareness; C2-Diversification of agricultural products and SC networks; C3-Taking proactive climate section; C4-Sustainable development orientation.
It is evident that G2, G3, A1, A3, and C2 serve as causal factors, while G1, G4, A2, A4, C1, C3, and C4 are identified as effect factors. Notably, diversification of agricultural products and SC networks (C2) exhibits the highest centrality and causality degree, underscoring its paramount significance in the overall system and its substantial influence over other factors.
The causality diagram depicting the sub-dimensions is illustrated in Figure 4.
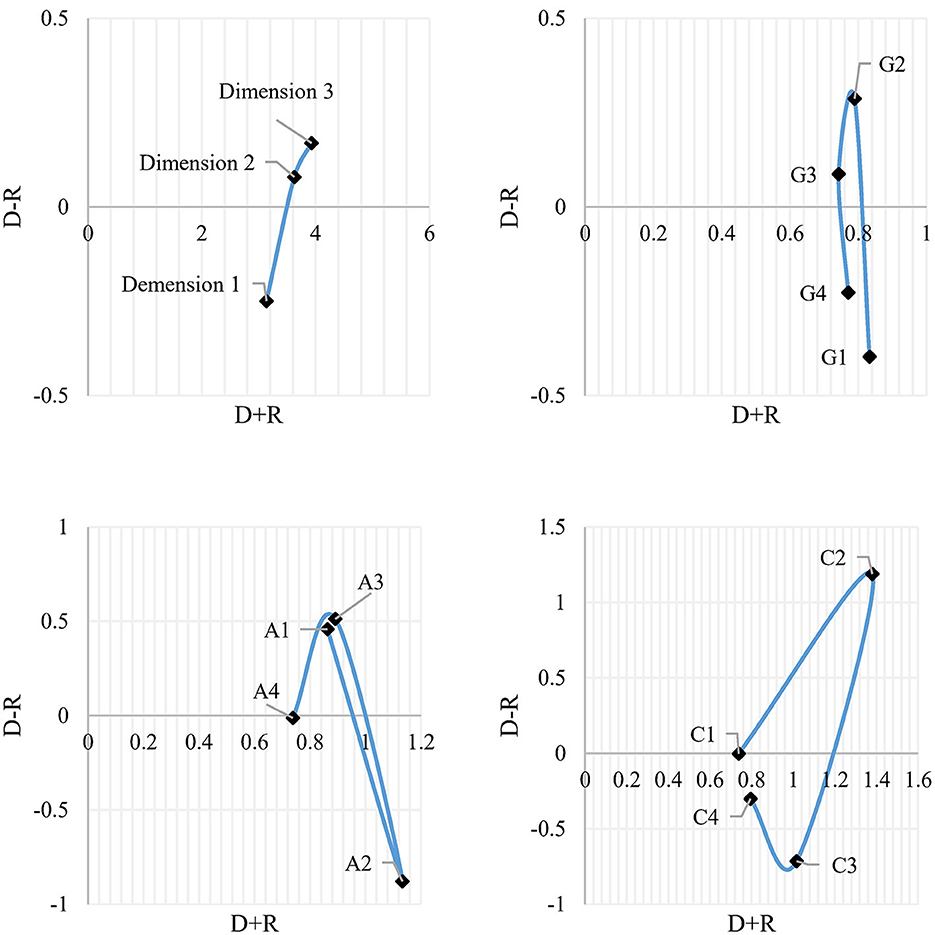
Figure 4. Dimensional causal diagrams. G1-Information sharing and resource integration; G2-Rapid recognition and timely response; G3-Dynamic adjustment for continuous operations; G4-Backup resources and contingency plan; A1-Transparency and predictive capacity; A2-Agricultural 4.0 and digital transformation; A3-Continuous learning and knowledge management; A4-Government support; C1-Climate change awareness; C2-Diversification of agricultural products and SC networks; C3-Taking proactive climate section; C4-Sustainable development orientation.
According to Figure 4A, dimension 3 (strategies for climate change) emerges as the paramount factor in constructing ASCRes. This highlights the fact that climate change is currently a significant concern for ASC practitioners, emphasizing the imperative need to adopt resilience strategies to enhance ASCs against its impacts. The dimension 1 (general strategy) exhibits the lowest centrality degree, aligning with observations made by Tendall et al. (2015) and Stone and Rahimifard (2018), which suggest that ASCs have a distinct mandate to ensure the provision of safe and appropriate food to end consumers during disruptions. Consequently, resilience strategies focus on organizational competitiveness in the broader SC context must be adapted to fit the ASC context.
According to Figure 4B, G1 exhibits the highest centrality degree among the general strategies, underscoring the importance of information sharing and resource integration. With information and resources breaking through the organizational boundaries, the SC gains the ability to rapidly respond to disruptions, dynamically adjust deliveries, and reserve redundant resources. This enables the entire SC to collaboratively address external challenges more effectively. G2 exhibits the highest causality degree, indicating that rapid recognition and timely response to disruptions has the greatest impact on other strategies. Early risk perception allows for a more comprehensive layout of SCRes strategies, facilitating flexible adjustments, coordinated arrangements, and the preparation of adequate resources.
According to Figure 4C, A2 exhibits the highest centrality degree among agricultural strategies, underscoring the importance of Agriculture 4.0 and digital transformation. The integration of Industry 4.0 technologies into ASCs has the potential to fundamentally transform agricultural systems, marking a pivotal development direction for the foreseeable future. A3 has the highest causality degree, indicating that continuous learning and knowledge management have the greatest impact on other strategies. By continuously learning from disruptions and systematically managing the acquired knowledge, organizations can improve their skills, thereby increasing their competitive advantage.
According to Figure 4D, C2 exhibits the highest centrality and causality degree among climate change response strategies. This indicates that diversification of agricultural products and SC networks is both the most crucial and the most impactful strategy. For ASCs, diversifying agricultural products enhances production adaptability and mitigates risks, while diversifying SC networks disperses risks, optimizes resource allocation, and boosts flexibility. Together, these strategies ensure that ASCs remain stable and efficient in the face of climate shocks.
5.2 ISM results analysis
Using the comprehensive influence matrix T, the overall influence matrix H is calculated according to Equation (7). The empirical threshold λ is set as the mean value of the comprehensive influence matrix. The rule transformation based on Equation (8) yields the reachability matrix K (Table 6).
Following Equation (9) and (10), the reachability set R(xi), the antecedent set Q(xi), and their intersection are calculated (Table 7). Each strategy's hierarchy is then determined based on the rule R(xi)∩Q(xi) = R(xi), resulting in a five-hierarchies model (Figure 5).
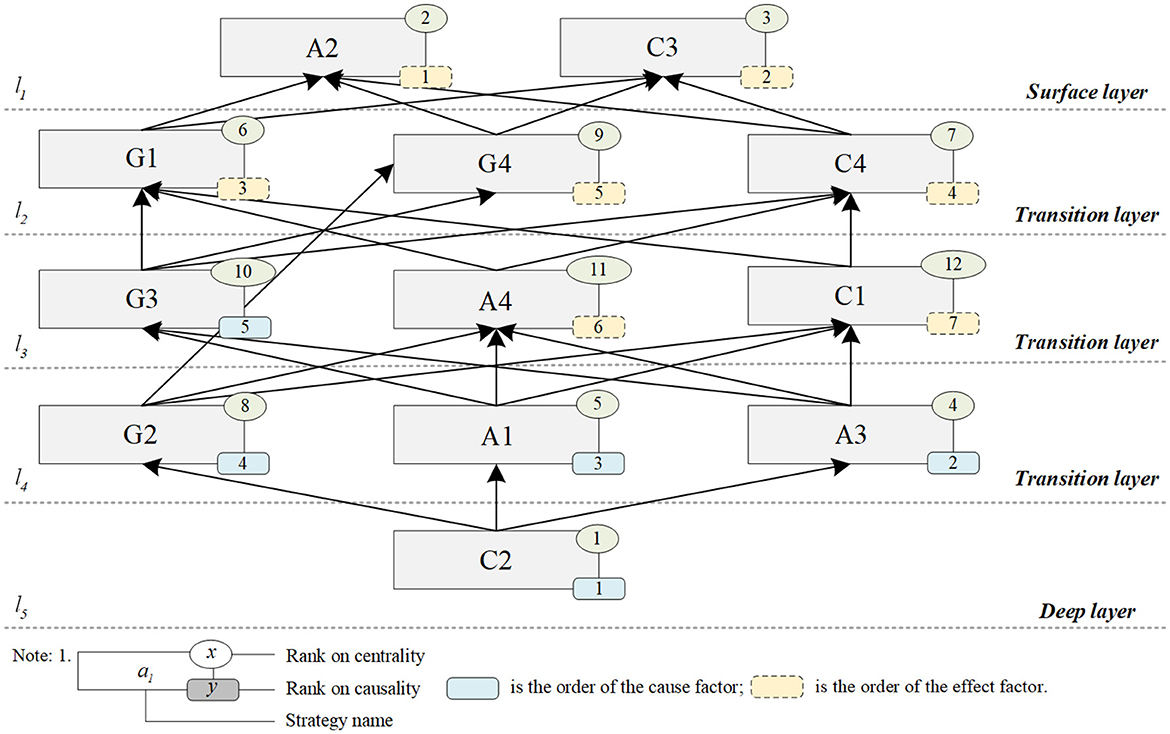
Figure 5. ISM hierarchical model. G1-Information sharing and resource integration; G2-Rapid recognition and timely response; G3-Dynamic adjustment for continuous operations; G4-Backup resources and contingency plan; A1-Transparency and predictive capacity; A2-Agricultural 4.0 and digital transformation; A3-Continuous learning and knowledge management; A4-Government support; C1-Climate change awareness; C2-Diversification of agricultural products and SC networks; C3-Taking proactive climate section; C4-Sustainable development orientation.
In the ISM hierarchical model, surface factors are those directly influenced by other factors and are often the first to be identified or most readily observable in a study. Deep factors typically result from the combination of multiple surface factors or abstract concepts that may not be directly visible but exert indirect influence by affecting surface factors. Transitional factors occupy the middle layer of the ISM hierarchical model, bridging the gap between surface factors and deep factors. They function as transmitters and connectors, facilitating the transmission of effects from bottom to top.
According to Figure 5, A2 and C3 represent surface layer strategies, which encompass specific operations, activities, or resources. Agriculture 4.0 and digital transformation, taking proactive climate action serve as pivotal supporting factors in developing climate resilience in ASCs. These strategies provide support in terms of both technical means and practical actions, respectively: digital transformation introduces cutting-edge technological capabilities and facilitates efficient information management, while proactive climate action fortifies system resilience through tailored agricultural practices. Collectively, these two strategies form a robust groundwork for realizing the ultimate goal. C2 is the only deep strategy that leads and supports the other strategies in the system. Diversification of agricultural products and SC network not only mitigates systemic risks and enhances adaptability and flexibility, but also lays a solid foundation for implementing other strategies, thereby enabling the entire system to achieve its objectives more effectively. The remaining nine strategies are situated in the transition layer, playing the role of transferring and connecting, helping to transfer the impacts of the deep layer strategies to the surface layer strategies.
5.3 PLS-SEM analysis
Based on the DEMATEL-ISM analysis, this paper obtains the direct and indirect influence relationships among variables, along with their relative significance and hierarchy in the system. To further validate the DEMATEL-ISM results and explore the quantitative relationships between variables, this paper incorporates the PLS-SEM (Partial Least Squares Structural Equation Modeling) analysis. PLS-SEM is a structural equation modeling technique that is particularly suitable for causal modeling in cases involving small sample sizes, non-normal data distributions, and complex models. The path relationships between the variables were plotted using SmartPLS 4, where the 12 SCRes strategies are linked to SCRes performance to form a path model.
The results indicate that the R2 of SCRes performance is 0.566, suggesting that the selected SCRes strategies can explain 56.6% of the variability in SCRes performance. Additionally, Q2 is 0.387, indicating that the SCRes strategies can effectively predict changes in SCRes performance. The path estimation results are presented in Table 8.
According to the PLS-SEM results, strategies G1, G2, G4, A1, A3, A4, C2, and C4 significantly contribute to enhancing ASCRes performance, while the remaining strategies are not significant. Notably, C2 emerges with the highest path coefficient of 0.162, indicating that the “diversification of agricultural products and SC network” strategy has the largest effect on ASCRes, which is consistent with the DEMATEL-ISM results. The path coefficient of A3 is 0.124, the second largest, emphasizing the significance of “continuous learning and knowledge management” in improving ASCRes. Consistently, A3 is positioned at the fourth level of the ISM hierarchy diagram, its impact on ASCRes is second only to that of C2. In contrast, the path coefficients of A2 and C3 are not significant and exhibit high P-values. Both strategies show high centrality but low causality degree in the DEMATEL analysis, suggesting that while A2 and C3 hold relative importance in the system, they might play a moderating role through intricate relationships with other factors rather than directly impacting the system goals.
6 Discussion and implication
6.1 Discussion
This paper explores the establishment of ASCRes in the context of climate change, identifying the following results:
First, diversification of agricultural products and SC networks (C2) is the most critical strategy of ASCRes. Embracing diversification in agricultural systems presents a potent avenue for bolstering resilience in the face of escalating climate pressures. In agricultural economics, numerous research underscores the imperative of fostering resilience in agricultural systems through crop diversification (Lin, 2011; Roesch-McNally et al., 2018; Bowles et al., 2020; Stevens and Teal, 2024). Birthal and Hazrana (2019), for example, noted that crop diversification serves as a pivotal ex-ante adaptation strategy against climate shocks, particularly demonstrating enhanced resilience in the face of severe shocks and over the long term. Despite the acknowledged benefits of crop diversification for resilience, its adoption has been sluggish (Lin, 2011). This can be attributed to various factors such as entrenched farming practices and traditional mindsets, inadequate economic incentives to switch crops, limited technological advancements and information dissemination, constraints on land and resources, or insufficient policy support. Moreover, in SCM, diversifying the structure of SCs serves as another avenue to enhance resilience. Climate change prompts the restructuring of global SCs, and complex SCs with numerous nodes and links often exhibit greater resilience to disruptions (Lim-Camacho et al., 2017). Diversifying suppliers, customers, and products can facilitate swift recovery from disruptions for SC companies (Lin et al., 2021; Wang et al., 2023b).
Second, Agriculture 4.0 and digital transformation (A2), and taking proactive climate action (C3) also emerged as pivotal strategies, ranking second in importance. ASCs still face hurdles due to various factors, such as inadequate technology adoption, information asymmetry, resource constraints, and cost pressures. Emerging technologies such as IoT, cloud computing, robotics, and AI have the potential to change agricultural system, leading to the advent of Agriculture 4.0 (Rose and Chilvers, 2018). Agriculture 4.0 is utilizing advanced digital technologies to transform the agricultural sector, making it smarter, more efficient, and environmentally sustainable (Javaid et al., 2022). Data capture, storage, transmission, transformation, and analysis contribute to sensing, monitoring, controlling, describing, predicting, decision-making, and adaptive learning in ASCs, enabling intelligent management and real-time monitoring of all nodes of production, transportation, warehousing, and sales, thus improving resilience and sustainability (Lezoche et al., 2020). On the other hand, existing studies usually treat “climate change awareness/adaptation intention” and “taking climate action” as distinct variables (Masud et al., 2016; Zhang et al., 2020; Todaro et al., 2020), it is clear that mere awareness without corresponding action falls short. Taking proactive climate action is paramount for translating awareness into tangible change. This entails initiatives such as implementing measures to curtail emissions, advocating for sustainable development policies, and fostering the adoption of clean energy. Such actions, undertaken by individuals and organizations, are pivotal in truly adapting to climate change and mitigating its adverse impacts.
6.2 Theoretical contributions
This paper distinguishes itself from studies such as Wang et al. (2023a) and Zhong et al. (2024) which also examine multiple strategies of ASCRes using the DEMATEL-ISM method as the core. In terms of theoretical construction, this paper employs a novel approach to strategy selection, forming a progressive theoretical framework. It starts from the broader SC dimension, then narrows down to the ASC dimension, considering the characteristics of the agricultural industry, and further focuses on the ASC's climate change response dimension. This framework ensures completeness and comprehensiveness by encompassing not only general SCM practices but also those specific to the agricultural industry and climate change context. By progressively narrowing down the scope of strategies to be examined, this approach enables a more precise understanding of the challenges in building ASCRes, facilitating the provision of targeted recommendations and strategies. In terms of experimental methodologies, this paper adopts the BP neural network algorithm to generate the direct influence matrix instead of the expert scoring. The latter relies on the subjective views and professional experiences of interviewees, lacking real data support and potentially leading to detachment from reality and poor objectivity. By using real data from 312 ASC companies and the introduction of the BP neural network algorithm, the objectivity and authenticity of the results can be ensured.
6.3 Managerial implications
The findings of this paper identify three key strategies for building ASCRes in the era of climate change: “diversification of agricultural products and SC networks,” “Agriculture 4.0 and digital transformation,” and “taking proactive climate action.” We believe that these findings can offer valuable insights for the agricultural sector, ASC business practices, and policymakers. Specifically as follows:
First, the findings emphasize the critical role of diversifying agricultural products and SC networks in addressing climate change. The agricultural sector should prioritize diversification to enhance the resilience and adaptability of the overall system. By promoting diversified cultivation patterns and establishing varied SC networks, ASC companies can mitigate the risks associated with single varieties and SC paths, enhancing their resilience to the uncertainties and disruptions caused by climate change. Specific measures include encouraging the cultivation of a wide range of crops, expanding supplier networks, and spreading production across different geographical areas to diversify climate risks. Additionally, policymakers should offer incentives to support companies in implementing diversification strategies. Through policy guidance and technical support, they can help companies achieve more efficient resource utilization and risk management during the diversification process.
Second, the findings highlight the importance of Agriculture 4.0 and digital transformation in enhancing ASCRes. The agriculture sector should actively promote the application of digital technologies, such as AI, IoT and big data analytics, to improve the efficiency and intelligence of agricultural production and SCM. Digital transformation not only improves productivity and quality, but also enhances the ability of ASC companies to respond and adjust quickly in the face of climate change. For example, precision agriculture technology allows companies to optimize water and fertilizer management, reducing resource wastage. SC visualization technology enables real-time monitoring of all SC links, allowing for the rapid identification and response to potential disruption risks. Policymakers should support this transformation by investing in digital infrastructure, providing technical training, and developing data-sharing platforms to facilitate the comprehensive implementation of agricultural digital transformation.
Finally, proactive climate action is crucial for enhancing ASCRes. Governments and the agricultural sector should collaborate to develop and implement effective climate adaptation and mitigation measures. These measures may include promoting water-saving irrigation technologies, improving soil health, and adopting climate-friendly agricultural practices. ASC companies should strengthen partnerships with research institutions and other stakeholders to advance the application of innovative technologies and practices, thereby mitigating the adverse effects of climate change on agricultural production and SCs. For example, adopting sustainable agricultural practices such as conservation tillage, crop rotation and cover crops, improve water utilization, and increase farmland's drought resilience. Policymakers should establish incentive programs, including subsidies, tax incentives, and technical support, to encourage companies to undertake proactive climate actions. These efforts will collectively advance sustainable development and bolster climate resilience in ASCs.
7 Conclusion, limitations, and future research
This paper explores strategies to enhance ASCRes in the context of climate change. An assessment framework, comprising 12 strategies across three dimensions, was developed through literature research and expert consultations. Utilizing survey data from 312 Chinese ASC companies, the BP-DEMATEL-ISM method was employed to investigate the importance and interrelationships of each strategy, while path analysis using PLS-SEM provided mutual validation. The research conclusions are as follows:
(1) Using the BP-DEMATEL model, this study calculated the causality and centrality of each factor, identifying five causal factors and seven effect factors related to ASCRes strategies. Among these, three critical strategies are highlighted: “diversification of agricultural products and SC networks (C2),” “Agriculture 4.0 and digital transformation (A2),” and “taking proactive climate action (C3).”
(2) This study performed a multi-level structural modeling of ASCRes using ISM. The 12 strategies across 3 dimensions were categorized into 5 levels. This analysis reveals that “diversification of agricultural products and SC networks (C2)” occupies a deep layer, while “Agriculture 4.0 and digital transformation (A2)” and “taking proactive climate action (C3) are positioned at the surface layer.
This study has limitations that should be addressed in future research. The 12 resilience strategies identified here are based on previous research and expert judgment, which may introduce time lag and idealization issues. Future studies could benefit from conducting semi-structured or in-depth interviews with agricultural companies to gain additional insights. By using the grounded theory approach to conceptually extract key resilience strategies and then integrating these findings with existing literature, a more comprehensive ASCRes framework of strategies could be developed.
Building on these conclusions, future research could further investigate the key strategies identified. Specifically, examining the impact of diversification strategies on the risk resilience and stability of ASCs, as well as exploring optimal diversification models through modeling approaches, could provide valuable insights. At the same time, the effects and best practices of implementing Agriculture 4.0 technologies—such as the AI, IoT, big data, and blockchain—in ASCs can be explored in depth. The challenges and solutions associated with digital transformation can be analyzed, with a particular focus on how these technologies can assist enterprises in addressing climate change and enhancing their adaptive capacity. Additionally, it is important to evaluate the effectiveness of climate action policies and their implementation, as well as to examine strategies for climate action and their economic, social and environmental benefits. This will provide comprehensive guidance to ASC companies, helping them to better cope with climate change and market challenges in the future, thereby enhancing the resilience and sustainability of their SCs.
Data availability statement
The raw data supporting the conclusions of this article will be made available by the authors, without undue reservation.
Ethics statement
Ethical review and approval was not required for the study on human participants in accordance with the local legislation and institutional requirements. Written informed consent from the [patients/ participants OR patients/participants legal guardian/next of kin] was not required to participate in this study in accordance with the national legislation and the institutional requirements.
Author contributions
MY: Conceptualization, Data curation, Investigation, Methodology, Writing – original draft. HH: Funding acquisition, Supervision, Writing – review & editing. MX: Conceptualization, Supervision, Writing – review & editing. JL: Supervision, Validation, Writing – review & editing.
Funding
The author(s) declare that financial support was received for the research, authorship, and/or publication of this article. This research was sponsored by NSFC (72072144, 71672144, and 71372173), the Key project of soft science research plan of Xi'an Science and Technology Bureau (23RKYJ0001 and 21RKYJ0009), Shaanxi Province Philosophy and Social Science Research Special Project (2023HZ1036, 2022HZ1824, and 2022HZ1581), Shaanxi Innovation Capability Support Program Soft Science Research Program (2024ZC-YBXM-031, 2019KRZ007, 2021KRM183, 2022KRM129, and 2022KRM097), and the Three Qin Talents Special Support Program for Leading Talents in Philosophy, Social Sciences, and Cultural Arts.
Conflict of interest
The authors declare that the research was conducted in the absence of any commercial or financial relationships that could be construed as a potential conflict of interest.
Publisher's note
All claims expressed in this article are solely those of the authors and do not necessarily represent those of their affiliated organizations, or those of the publisher, the editors and the reviewers. Any product that may be evaluated in this article, or claim that may be made by its manufacturer, is not guaranteed or endorsed by the publisher.
Supplementary material
The Supplementary Material for this article can be found online at: https://www.frontiersin.org/articles/10.3389/fsufs.2024.1444910/full#supplementary-material
References
Aboah, J., Wilson, M. M. J., Rich, K. M., and Lyne, M. C. (2019). Operationalising resilience in tropical agricultural value chains. Supply Chain Manag. 24, 271–300. doi: 10.1108/SCM-05-2018-0204
Ado, A. M., Leshan, J., Savadogo, P., Bo, L., and Shah, A. A. (2019). Farmers' awareness and perception of climate change impacts: case study of Aguie district in Niger. Environ. Dev. Sustainability 21, 2963–2977. doi: 10.1007/s10668-018-0173-4
Alam, M. F. B., Tushar, S. R., Ahmed, T., Karmaker, C. L., Bari, A. B. M. M., de Jesus Pacheco, D. A., et al. (2024). Analysis of the enablers to deal with the ripple effect in food grain supply chains under disruption: Implications for food security and sustainability. Int. J. Prod. Econ. 270. doi: 10.1016/j.ijpe.2024.109179
Ali, A., Mahfouz, A., and Arisha, A. (2017). Analysing supply chain resilience: integrating the constructs in a concept mapping framework via a systematic literature review. Supply Chain Manag. 22, 16–39. doi: 10.1108/SCM-06-2016-0197
Al-Omoush, K. S., de Lucas, A., and del Val, M. T. (2023). The role of e-supply chain collaboration in collaborative innovation and value-co creation. J. Bus. Res. 158:113647. doi: 10.1016/j.jbusres.2023.113647
Ambrosini, V., and Bowman, C. (2009). What are dynamic capabilities and are they a useful construct in strategic management? Int. J. Manag. Rev. 11, 29–49. doi: 10.1111/j.1468-2370.2008.00251.x
Anderson, R., Bayer, P. E., and Edwards, D. (2020). Climate change and the need for agricultural adaptation. Curr. Opin. Plant Biol. 56, 197–202. doi: 10.1016/j.pbi.2019.12.006
Attri, R., Dev, N., and Sharma, V. (2013). Interpretive structural modelling (ISM) approach: an overview. Res. J. Management Sci. 2, 3–8.
Azadi, H., Movahhed Moghaddam, S., Burkart, S., Mahmoudi, H., Van Passel, S., Kurban, A., et al. (2021). Rethinking resilient agriculture: from climate-smart agriculture to vulnerable-smart agriculture. J. Cleaner Prod. 319:128602. doi: 10.1016/j.jclepro.2021.128602
Bai, C., Sarkis, J., Yin, F., and Dou, Y. (2020). Sustainable supply chain flexibility and its relationship to circular economy-target performance. Int. J. Prod. Res. 58, 5893–5910. doi: 10.1080/00207543.2019.1661532
Balezentis, T., Zickiene, A., Volkov, A., Streimikiene, D., Morkunas, M., Dabkiene, V., et al. (2023). Measures for the viable agri-food supply chains: a multi-criteria approach. J. Bus. Res. 155:113417. doi: 10.1016/j.jbusres.2022.113417
Barney, J. (1991). Firm resources and sustained competitive advantage. J. Manage. 17, 99–120. doi: 10.1177/014920639101700108
Behzadi, G., O'Sullivan, M. J., and Olsen, T. L. (2020). On metrics for supply chain resilience. Eur. J. Oper. Res. 287, 145–158. doi: 10.1016/j.ejor.2020.04.040
Birthal, P. S., and Hazrana, J. (2019). Crop diversification and resilience of agriculture to climatic shocks: evidence from India. Agric. Syst. 173, 345–354. doi: 10.1016/j.agsy.2019.03.005
Blessley, M., and Mudambi, S. M. (2022). A trade war and a pandemic: disruption and resilience in the food bank supply chain. Ind. Market Manag. 102, 58–73. doi: 10.1016/j.indmarman.2022.01.002
Bode, C., and Macdonald, J. R. (2017). Stages of supply chain disruption response: direct, constraining, and mediating factors for impact mitigation. Decis. Sci. 48, 836–874. doi: 10.1111/deci.12245
Bowles, T. M., Mooshammer, M., Socolar, Y., Calderón, F., Cavigelli, M. A., Culman, S. W., et al. (2020). Long-term evidence shows that crop-rotation diversification increases agricultural resilience to adverse growing conditions in North America. One Earth 2, 284–293. doi: 10.1016/j.oneear.2020.02.007
Brettel, M., Friederichsen, N., Keller, M., and Rosenberg, M. (2014). How virtualization, decentralization and network building change the manufacturing landscape: an Industry 4.0 Perspective. Int. J. Inform. Commun. Eng. 8, 37–44.
Buitenhuis, Y., Candel, J. J. L., Termeer, K. J. A. M., and Feindt, P. H. (2022). Reconstructing the framing of resilience in the European Union's Common Agricultural Policy post-2020 reform. Sociol. Ruralis 62, 564–586. doi: 10.1111/soru.12380
Chowdhury, M. M. H., and Quaddus, M. (2017). Supply chain resilience: conceptualization and scale development using dynamic capability theory. Int. J. Prod. Econ. 188, 185–204. doi: 10.1016/j.ijpe.2017.03.020
Christopher, M., and Peck, H. (2004). Building the resilient supply chain. Int. J. Logist. Manag. 15, 1–14. doi: 10.1108/09574090410700275
Coopmans, I., Bijttebier, J., Marchand, F., Mathijs, E., Messely, L., Rogge, E., et al. (2021). COVID-19 impacts on Flemish food supply chains and lessons for agri-food system resilience. Agric. Syst. 190:103136. doi: 10.1016/j.agsy.2021.103136
Davis, K. F., Downs, S., and Gephart, J. A. (2021). Towards food supply chain resilience to environmental shocks. Nat. Food 2, 54–65. doi: 10.1038/s43016-020-00196-3
Duong, L. N. K., and Chong, J. (2020). Supply chain collaboration in the presence of disruptions: a literature review. Int. J. Prod. Res. 58, 3488–3507. doi: 10.1080/00207543.2020.1712491
Efron, B. (1986). How biased is the apparent error rate of a prediction rule? J. Am. Stat. Assoc. 81, 461–470. doi: 10.1080/01621459.1986.10478291
Eisenhardt, K. M., and Martin, J. A. (2000). Dynamic capabilities: what are they? Strategic Manage. J. 21, 1105–1121.
Fiksel, J. (2003). Designing resilient, sustainable systems. Environ. Sci. Technol. 37, 5330–5339. doi: 10.1021/es0344819
Folke, C. (2006). Resilience: the emergence of a perspective for social–ecological systems analyses. Global Environ. Change 16, 253–267. doi: 10.1016/j.gloenvcha.2006.04.002
Gabus, A., and Fontela, E. J. B. G. R. C. (1972). World Problems, an Invitation to Further Thought Within the Framework of DEMATEL. Geneva: Battelle Geneva Research Center, 12–14.
Gligor, D., Gligor, N., Holcomb, M., and Bozkurt, S. (2019). Distinguishing between the concepts of supply chain agility and resilience. Int. J. Logist. Manag. 30, 467–487. doi: 10.1108/IJLM-10-2017-0259
Godde, C. M., Mason-D'Croz, D., Mayberry, D. E., Thornton, P. K., and Herrero, M. (2021). Impacts of climate change on the livestock food supply chain; a review of the evidence. Global Food Secur. 28, 100488. doi: 10.1016/j.gfs.2020.100488
Han, Y., Chong, W. K., and Li, D. (2020). A systematic literature review of the capabilities and performance metrics of supply chain resilience. Int. J. Prod. Res. 58, 4541–4566. doi: 10.1080/00207543.2020.1785034
Hassini, E., Surti, C., and Searcy, C. (2012). A literature review and a case study of sustainable supply chains with a focus on metrics. Int. J. Prod. Econ. 140, 69–82. doi: 10.1016/j.ijpe.2012.01.042
Hohenstein, N.-O., Feisel, E., Hartmann, E., and Giunipero, L. (2015). Research on the phenomenon of supply chain resilience. Int. J. Phys. Distr. Log. 45(1/2), 90-117. doi: 10.1108/IJPDLM-05-2013-0128
Hosseini, S., Ivanov, D., and Dolgui, A. (2019). Review of quantitative methods for supply chain resilience analysis. Transport Res. E-Log. 125, 285–307. doi: 10.1016/j.tre.2019.03.001
Huo, B., Gu, M., and Wang, Z. (2018). Supply chain flexibility concepts, dimensions and outcomes: an organisational capability perspective. Int. J. Prod. Res. 56, 5883–5903. doi: 10.1080/00207543.2018.1456694
Javaid, M., Haleem, A., Singh, R. P., and Suman, R. (2022). Enhancing smart farming through the applications of Agriculture 4.0 technologies. Int. J. Intell. Networks 3, 150–164. doi: 10.1016/j.ijin.2022.09.004
Juan, S.-J., Li, E. Y., and Hung, W.-H. (2022). An integrated model of supply chain resilience and its impact on supply chain performance under disruption. Int. J. Logist. Manag. 33, 339–364. doi: 10.1108/IJLM-03-2021-0174
Kamalahmadi, M., and Parast, M. M. (2016). A review of the literature on the principles of enterprise and supply chain resilience: major findings and directions for future research. Int. J. Prod. Econ. 171, 116–133. doi: 10.1016/j.ijpe.2015.10.023
Kamalahmadi, M., and Parast, M. M. (2017). An assessment of supply chain disruption mitigation strategies. Int. J. Prod. Econ. 184, 210–230. doi: 10.1016/j.ijpe.2016.12.011
Kamalahmadi, M., Shekarian, M., and Mellat Parast, M. (2021). The impact of flexibility and redundancy on improving supply chain resilience to disruptions. Int. J. Prod. Res. 60, 1992–2020. doi: 10.1080/00207543.2021.1883759
Kamble, S. S., Gunasekaran, A., and Gawankar, S. A. (2020). Achieving sustainable performance in a data-driven agriculture supply chain: a review for research and applications. Int. J. Prod. Econ. 219, 179–194. doi: 10.1016/j.ijpe.2019.05.022
Kazancoglu, Y., Lafci, C., Kumar, A., Luthra, S., Garza-Reyes, J. A., and Berberoglu, Y. (2024). The role of agri-food 4.0 in climate-smart farming for controlling climate change-related risks: A business perspective analysis. Bus. Strateg. Environ. 33, 2788–2802. doi: 10.1002/bse.3629
Kumar, P., and Kumar, R. (2022). Strategic framework for developing resilience in Agri-Food Supply Chains during COVID 19 pandemic. Int. J. Logist. Res. Appl. 25, 1401–1424. doi: 10.1080/13675567.2021.1908524
Lezoche, M., Hernandez, J. E., Alemany Díaz, M. E., Panetto, H., and Kacprzyk, J. (2020). Agri-food 4.0: A survey of the supply chains and technologies for the future agriculture. Comput. Ind. 117. doi: 10.1016/j.compind.2020.103187
Lim-Camacho, L., Plagányi, É. E., Crimp, S., Hodgkinson, J. H., Hobday, A. J., Howden, S. M., et al. (2017). Complex resource supply chains display higher resilience to simulated climate shocks. Global Environ. Change 46, 126–138. doi: 10.1016/j.gloenvcha.2017.08.011
Lin, B. B. (2011). Resilience in agriculture through crop diversification: adaptive management for environmental change. Bioscience 61, 183–193. doi: 10.1525/bio.2011.61.3.4
Lin, Y., Fan, D., Shi, X., and Fu, M. (2021). The effects of supply chain diversification during the COVID-19 crisis: evidence from Chinese manufacturers. Transport Res. E-Log 155:102493. doi: 10.1016/j.tre.2021.102493
Macfadyen, S., Tylianakis, J. M., Letourneau, D. K., Benton, T. G., Tittonell, P., Perring, M. P., et al. (2015). The role of food retailers in improving resilience in global food supply. Global Food Secur. 7, 1–8. doi: 10.1016/j.gfs.2016.01.001
Marcucci, G., Ciarapica, F. E., Mazzuto, G., and Bevilacqua, M. (2024). Analysis of ripple effect and its impact on supply chain resilience: a general framework and a case study on agri-food supply chain during the COVID-19 pandemic. Oper. Manage. Res. 17, 175–200. doi: 10.1007/s12063-023-00415-7
Marshall, N. A., Park, S., Howden, S. M., Dowd, A. B., and Jakku, E. S. (2013). Climate change awareness is associated with enhanced adaptive capacity. Agric. Syst. 117, 30–34. doi: 10.1016/j.agsy.2013.01.003
Masud, M. M., Al-Amin, A. Q., Junsheng, H., Ahmed, F., Yahaya, S. R., Akhtar, R., et al. (2016). Climate change issue and theory of planned behaviour: relationship by empirical evidence. J. Cleaner Prod. 113, 613–623. doi: 10.1016/j.jclepro.2015.11.080
Mishra, R., Singh, R. K., and Subramanian, N. (2022). Impact of disruptions in agri-food supply chain due to COVID-19 pandemic: contextualised resilience framework to achieve operational excellence. Int. J. Logist. Manag. 33, 926–954. doi: 10.1108/IJLM-01-2021-0043
Novak, D. C., Wu, Z., and Dooley, K. J. (2021). Whose resilience matters? Addressing issues of scale in supply chain resilience. J. Bus. Logist. 42, 323–335. doi: 10.1111/jbl.12270
Pankratz, N. M. C., Schiller, C. M., and Mueller, H. (2024). Climate change and adaptation in global supply-chain networks. Rev. Financ. Stud. 37, 1729–1777. doi: 10.1093/rfs/hhad093
Park, Y., Hong, P., and Roh, J. J. (2013). Supply chain lessons from the catastrophic natural disaster in Japan. Bus. Horizons 56, 75–85. doi: 10.1016/j.bushor.2012.09.008
Perrin, A., and Martin, G. (2021). Resilience of French organic dairy cattle farms and supply chains to the Covid-19 pandemic. Agric. Syst. 190, 103082. doi: 10.1016/j.agsy.2021.103082
Pesonen, H.-L., and Horn, S. (2014). Evaluating the climate SWOT as a tool for defining climate strategies for business. J. Cleaner Prod. 64, 562–571. doi: 10.1016/j.jclepro.2013.10.013
Qin, X., Yuan, W., Li, J., and Qi, X. (2020). Identification and analysis of urban Shrinkage risk based on residential property values. Habitat Int. 106, 102265. doi: 10.1016/j.habitatint.2020.102265
Ribašauskiene, E., Volkov, A., Morkunas, M., Žičkiene, A., Dabkiene, V., Štreimikiene, D., et al. (2024). Strategies for increasing agricultural viability, resilience and sustainability amid disruptive events: An expert-based analysis of relevance. J. Bus. Res. 170, 114328. doi: 10.1016/j.jbusres.2023.114328
Richey, R. G., Roath, A. S., Adams, F. G., and Wieland, A. (2022). A responsiveness view of logistics and supply chain management. J. Bus. Logist. 43, 62–91. doi: 10.1111/jbl.12290
Roesch-McNally, G. E., Arbuckle, J. G., and Tyndall, J. C. (2018). Barriers to implementing climate resilient agricultural strategies: The case of crop diversification in the U.S. Corn Belt. Global Environ. Change 48, 206–215. doi: 10.1016/j.gloenvcha.2017.12.002
Rose, D. C., and Chilvers, J. (2018). Agriculture 4.0: broadening responsible innovation in an era of smart farming. Front. Sust. Food Syst. 2:87. doi: 10.3389/fsufs.2018.00087
Sá, M. M., Miguel, P. L. S., Brito, R. P., and Pereira, S. C. F. (2019). Supply chain resilience: the whole is not the sum of the parts. Int. J. Oper. Prod. Manage. 40, 92–115. doi: 10.1108/IJOPM-09-2017-0510
Schniederjans, D. G., Curado, C., and Khalajhedayati, M. (2020). Supply chain digitisation trends: An integration of knowledge management. Int. J. Prod. Econ. 220:12. doi: 10.1016/j.ijpe.2019.07.012
Scholten, K., and Schilder, S. (2015). The role of collaboration in supply chain resilience. Supply Chain Manag. 20, 471–484. doi: 10.1108/SCM-11-2014-0386
Shashi, C. P., Cerchione, R., and Ertz, M. (2019). Managing supply chain resilience to pursue business and environmental strategies. Bus. Strateg. Environ. 29, 1215–1246. doi: 10.1002/bse.2428
Shekarian, M., and Mellat Parast, M. (2021). An integrative approach to supply chain disruption risk and resilience management: a literature review. Int. J. Logist. Res. Appl. 24, 427–455. doi: 10.1080/13675567.2020.1763935
Shekarian, M., Reza Nooraie, S. V., and Parast, M. M. (2020). An examination of the impact of flexibility and agility on mitigating supply chain disruptions. Int. J. Prod. Econ. 220. doi: 10.1016/j.ijpe.2019.07.011
Shepherd, M., Turner, J. A., Small, B., and Wheeler, D. (2020). Priorities for science to overcome hurdles thwarting the full promise of the 'digital agriculture' revolution. J. Sci. Food Agric. 100, 5083–5092. doi: 10.1002/jsfa.9346
Stevens, A. W., and Teal, J. (2024). Diversification and resilience of firms in the agrifood supply chain. Am. J. Agric. Econ. 106, 739–778. doi: 10.1111/ajae.12398
Stone, J., and Rahimifard, S. (2018). Resilience in agri-food supply chains: a critical analysis of the literature and synthesis of a novel framework. Supply Chain Manag. 23, 207–238. doi: 10.1108/SCM-06-2017-0201
Teece, D. J., Pisano, G., and Shuen, A. (1997). Dynamic capabilities and strategic management. Strategic Manage. J. 18, 509–533.
Tendall, D. M., Joerin, J., Kopainsky, B., Edwards, P., Shreck, A., Le, Q. B., et al. (2015). Food system resilience: Defining the concept. Global Food Secur. 6, 17–23. doi: 10.1016/j.gfs.2015.08.001
Todaro, N. M., Testa, F., Daddi, T., and Iraldo, F. (2020). The influence of managers' awareness of climate change, perceived climate risk exposure and risk tolerance on the adoption of corporate responses to climate change. Bus. Strateg. Environ. 30, 1232–1248. doi: 10.1002/bse.2681
Tukamuhabwa, B. R., Stevenson, M., Busby, J., and Zorzini, M. (2015). Supply chain resilience: definition, review and theoretical foundations for further study. Int. J. Prod. Res. 53, 5592–5623. doi: 10.1080/00207543.2015.1037934
Umar, M., Wilson, M., and Heyl, J. (2021). The structure of knowledge management in inter-organisational exchanges for resilient supply chains. J. Knowl. Manag. 25, 826–846. doi: 10.1108/JKM-06-2020-0488
Wang, C. L., and Ahmed, P. K. (2007). Dynamic capabilities: a review and research agenda. Int. J. Manag. Rev. 9, 31–51. doi: 10.1111/j.1468-2370.2007.00201.x
Wang, J., Liu, M., Chen, Y., and Yu, M. (2023a). Influencing factors on green supply chain resilience of agricultural products: an improved gray-DEMATEL-ISM approach. Front. Sustainable Food Syst. 7. doi: 10.3389/fsufs.2023.1166395
Wang, Q., Zhou, H., and Zhao, X. (2023b). The role of supply chain diversification in mitigating the negative effects of supply chain disruptions in COVID-19. Int. J. Oper. Prod. Manage. 44, 99–132. doi: 10.1108/IJOPM-09-2022-0567
Warfield, J. N. (1974). Toward interpretation of complex structural models. IEEE T. Syst. Man. Cy. (5), 405-417. doi: 10.1109/TSMC.1974.4309336
Wernerfelt, B. (1984). A resource-based view of the firm. Strategic Manage. J. 5, 171–180. doi: 10.1002/smj.4250050207
Wieland, A., and Durach, C. F. (2021). Two perspectives on supply chain resilience. J. Bus. Logist. 42, 315–322. doi: 10.1111/jbl.12271
Wong, J.-T. (2020). Dynamic procurement risk management with supplier portfolio selection and order allocation under green market segmentation. J. Cleaner Prod. 253. doi: 10.1016/j.jclepro.2019.119835
Yadav, V. S., Singh, A. R., Raut, R. D., Mangla, S. K., Luthra, S., and Kumar, A. (2022). Exploring the application of Industry 4.0 technologies in the agricultural food supply chain: A systematic literature review. Comput. Ind. Eng. 169. doi: 10.1016/j.cie.2022.108304
Yu, W., Jacobs, M. A., Chavez, R., and Yang, J. (2019). Dynamism, disruption orientation, and resilience in the supply chain and the impacts on financial performance: a dynamic capabilities perspective. Int. J. Prod. Econ. 218, 352–362. doi: 10.1016/j.ijpe.2019.07.013
Zeweld, W., Van Huylenbroeck, G., Tesfay, G., and Speelman, S. (2017). Smallholder farmers' behavioural intentions towards sustainable agricultural practices. J. Environ. Manage. 187, 71–81. doi: 10.1016/j.jenvman.2016.11.014
Zhang, L., Ruiz-Menjivar, J., Luo, B., Liang, Z., and Swisher, M. E. (2020). Predicting climate change mitigation and adaptation behaviors in agricultural production: a comparison of the theory of planned behavior and the Value-Belief-Norm Theory. J. Environ. Psychol. 68. doi: 10.1016/j.jenvp.2020.101408
Zhao, G., Liu, S., Lopez, C., Chen, H., Lu, H., Mangla, S. K., et al. (2020). Risk analysis of the agri-food supply chain: a multi-method approach. Int. J. Prod. Res. 58, 4851–4876. doi: 10.1080/00207543.2020.1725684
Zhao, G., Liu, S., Wang, Y., Lopez, C., Zubairu, N., Chen, X., et al. (2024). Modelling enablers for building agri-food supply chain resilience: insights from a comparative analysis of Argentina and France. Prod. Plan. Control 35, 283–307. doi: 10.1080/09537287.2022.2078246
Zhao, N., Hong, J., and Lau, K. H. (2023). Impact of supply chain digitalization on supply chain resilience and performance: a multi-mediation model. Int. J. Prod. Econ. 259, 108817. doi: 10.1016/j.ijpe.2023.108817
Keywords: climate change, agricultural supply chain, resilience, BP-DEMATEL-ISM, diversification
Citation: Yuan M, Hu H, Xue M and Li J (2024) Framework for resilience strategies in agricultural supply chain: assessment in the era of climate change. Front. Sustain. Food Syst. 8:1444910. doi: 10.3389/fsufs.2024.1444910
Received: 06 June 2024; Accepted: 13 August 2024;
Published: 10 September 2024.
Edited by:
Abiodun Ogundeji, University of the Free State, South AfricaReviewed by:
Alison Gerken, Agricultural Research Service (USDA), United StatesLucia Briamonte, Council for Agricultural Research and Agricultural Economy Analysis | CREA, Italy
Copyright © 2024 Yuan, Hu, Xue and Li. This is an open-access article distributed under the terms of the Creative Commons Attribution License (CC BY). The use, distribution or reproduction in other forums is permitted, provided the original author(s) and the copyright owner(s) are credited and that the original publication in this journal is cited, in accordance with accepted academic practice. No use, distribution or reproduction is permitted which does not comply with these terms.
*Correspondence: Haiqing Hu, aHVoYWlxaW5nQHhhdXQuZWR1LmNu; Meng Xue, eG1AeGF1dC5lZHUuY24=