- 1School of Civil Engineering, Hefei University of Technology, Hefei, China
- 2Key Laboratory of Water Conservancy and Water Resources of Anhui Province, Water Resources Research Institute of Anhui Province and Huaihe River Commission, Ministry of Water Resources, Hefei, China
Introduction: Continuous drought stress aggravates agricultural losses and threats food security. However, the responses of crops to continuous drought stress remain uncertain.
Methods: To make up the limitations of field experiment and achieve the setting of multiple continuous drought stress scenarios, AquaCrop model is calibrated and validated using field experiment data of summer maize in 2017 and 2018 seasons. Then, the whole growth processes under different continuous drought stress scenarios at two growth stages of maize are simulated. The quantitative responses of transpiration (Tr), biomass accumulation, and yield formation to continuous drought stress are analyzed.
Results and discussion: The results show that when the maize encounters serious drought at the seedling stage, the reduction rates of Tr at the jointing stage, the tasseling stage, and the milking stage are 57.45%, 43.61%, and 5.24%, respectively. Drought stress at a growth stage of maize not only have negative impacts on transpiration and biomass accumulation at this stage, but also have after-effects on these elements at the subsequent stages. In addition, continuous serious drought at the seedling and jointing stages reduces yield by 100%, which is higher than the sum of the loss rates at these two stages [>33.30% + 24.16%)], while the loss rate due to continuous light drought is lower than the sum [20.66% < (18.80% + 12.45%)]. The impact of continuous drought stress at two growth stages generally exceeds the sum of the impacts of the two single stages. Nevertheless, drought at the seedling stage promotes the adaptability of maize to drought, alleviating the negative impacts of light drought at the jointing stage, while the adaptability disappears when drought at the jointing stage is serious. Therefore, in the actual production of maize, serious drought at the seedling stage should be avoided to ensure seed survival. Meanwhile, continuous drought at the seedling and jointing stages should be prevented to reduce the severe accumulative effects, which guides drought disaster reduction and sustainable agricultural production.
1 Introduction
In recent years, the frequency, intensity, and scope of drought disasters have increased prominently due to global climate change and human activities (Ge et al., 2021). As a result, drought disasters have become one of the major bottlenecks restricting economic development and social stability in many countries and regions (Ali et al., 2020; Zhang et al., 2020; Zhou et al., 2022). Since the 21st century, global drought disasters have become increasingly severe. Due to the location of the eastern monsoon region, continuous droughts occur more frequently during successive seasons and years in China (Chang et al., 2016; Salgotra and Chauhan, 2023). For example, in 2006, there was a four-season continuous drought in southwest China. In 2022, the Yangtze River Basin had experienced a rare drought in summer, autumn, and winter. As agricultural production is highly dependent on climatic conditions, the negative impacts of drought on agricultural production are the most obvious and direct (Mondol et al., 2021). In addition, continuous drought that spans several growth stages has a more serious negative impact on crop growth and yield formation. It has been estimated that global crop production loss due to drought has reached about $30 billion over the past decade (Gupta et al., 2020). Furthermore, there is an average annual grain loss of 2.31 × 1010 kg due to drought from 2001 to 2021 in China (Cui et al., 2023). Thus, drought disasters have become one of the most significant threats to global food security and regional stable development (Zhang et al., 2004; Hussain et al., 2023), the research on the impacts of continuous drought stress on crops is conducive to agricultural drought risk management.
The impact of drought on crops is a complex process that closely relates to the drought intensity, drought duration, and crop growth stage of drought occurrence (Wang et al., 2023; Li et al., 2024). Moreover, the continuous drought at several stages further complicates the impact (Ors et al., 2021). Currently, numerous studies have been conducted on the impacts of drought on crop physiology and growth indicators. For instance, the influences of different levels and durations of drought on crops (Dao et al., 2023), the impacts of drought on different organs of crops (Shafi et al., 2023), and the influences of drought at different stages (Lamin-Samu et al., 2021). However, most studies concentrate on the effects of drought at a single growth stage of crops, and there are few studies on the lasting impact of drought at a certain stage and the cumulative impact of continuous drought during several stages (Zhang et al., 2015).
Due to the complexity of crop responses under different drought conditions, it is difficult to accurately describe and quantify the effects of drought on the crop growth process. Crop models can mechanistically simulate important physiological and growth processes such as photosynthesis, transpiration, and biomass accumulation of crops under various drought conditions (Tojo Soler et al., 2013; Irmak et al., 2024). The entire process of crop growth under continuous drought at different growth stages can be simulated more conveniently using a crop model after the localization by field experiments. The commonly used crop models include DSSAT (Guo et al., 2016), APSIM (Wang et al., 2022), and AquaCrop (Greaves and Wang, 2016), etc. AquaCrop model is a crop model developed by the Food and Agriculture Organization of the United Nations (FAO), it can reflect the responses mechanism of crops to drought (Li et al., 2022). Driven by water factors, AquaCrop model can effectively separate crop transpiration from soil evaporation and is most suitable for simulating crop in response to drought (Razzaghi et al., 2017). Meanwhile, this model has a user-friendly interface and requires only a few input parameters (Xie et al., 2023). AquaCrop model has been widely used to forecast crop yield and dry matter amount under different water conditions (Ćosić et al., 2017). It has also been employed to simulate soil water content and canopy coverage for determining crop water requirements (Voloudakis et al., 2015), and to evaluate the impact of irrigation scheduling on crop growth (Corbari et al., 2021).
Maize is one of the world’s major crops (Zhu et al., 2021; Ge et al., 2024) and sustainable maize production plays a vital role in meeting the rapidly growing global food demand and ensuring national food security (Peng et al., 2023a). Since 2001, maize has surpassed rice as the world’s second-largest cereal. In 2021, China is the world’s second-largest producer of maize, with about 4.32 × 107 ha of planting area, and the yield reaches 2.72 × 1011 kg, accounting for 22% of total global production. Furthermore, the Huaibei Plain is one of the leading areas for maize cultivation in China (Wei et al., 2019), with one-third of the national planting area. Nevertheless, this region is located in the transitional region of temperate and subtropical climate, with a large interannual variation and an uneven distribution of precipitation within a year, frequent continuous droughts, which seriously limits the maize production.
Continuous drought events severely threaten global food security and regional stable development. As maize is one of the world’s important crops, the study on the quantitative effects of continuous drought stress on its growth process and yield formation, thus revealing the complex response mechanism of crops to continuous drought stress, is the foundation for evaluating grain drought losses and agricultural drought risk. In addition, it is assumed that all summer maize plants grow consistently without randomness such as gene mutations, plant growth is only affected by drought stress, without diseases, pests, and high temperatures, and plants can pollinate and bear fruit normally in this research. The current contributions of this study are as follows: (1) calibrating and verifying the AquaCrop model by using two seasons of field experiments of summer maize at Xinmaqiao Experimental Station in Bengbu City, Huaibei Plain, China; (2) selecting a base year and designing the continuous drought stress scenarios at two growth stages of maize by changing the precipitation in the base year, and using the calibrated AquaCrop model to simulate the maize growth processes under different drought stress scenarios; (3) analysing the quantitative responses of maize to various continuous droughts at two growth stages, including plant transpiration, dry matter accumulation, and yield formation. And revealing the complex phenomena such as the after-effect of drought at a growth stage and the accumulative and adaptive effects of continuous drought stress on maize. This study can provide a scientific decision-making basis for maize production and disaster reduction under the current trend of continuous and serious drought. It is conducive to promoting regional sustainable agricultural development and providing support for ensuring global food security.
2 Materials and methods
In this paper, we conduct a study on the quantitative responses of summer maize to continuous drought stress at two growth stages by combining field experiments and AquaCrop model. Figure 1 illustrates the process. The AquaCrop model is calibrated and verified using the field experiment data of summer maize in 2017 and 2018. Then, various continuous drought scenarios at two growth stage are set up, and the growth process of maize under different scenarios are simulated. Finally, the quantitative responses of summer maize to continuous drought stress at two growth stage are analyzed, including crop transpiration, dry matter accumulation, and yield formation.
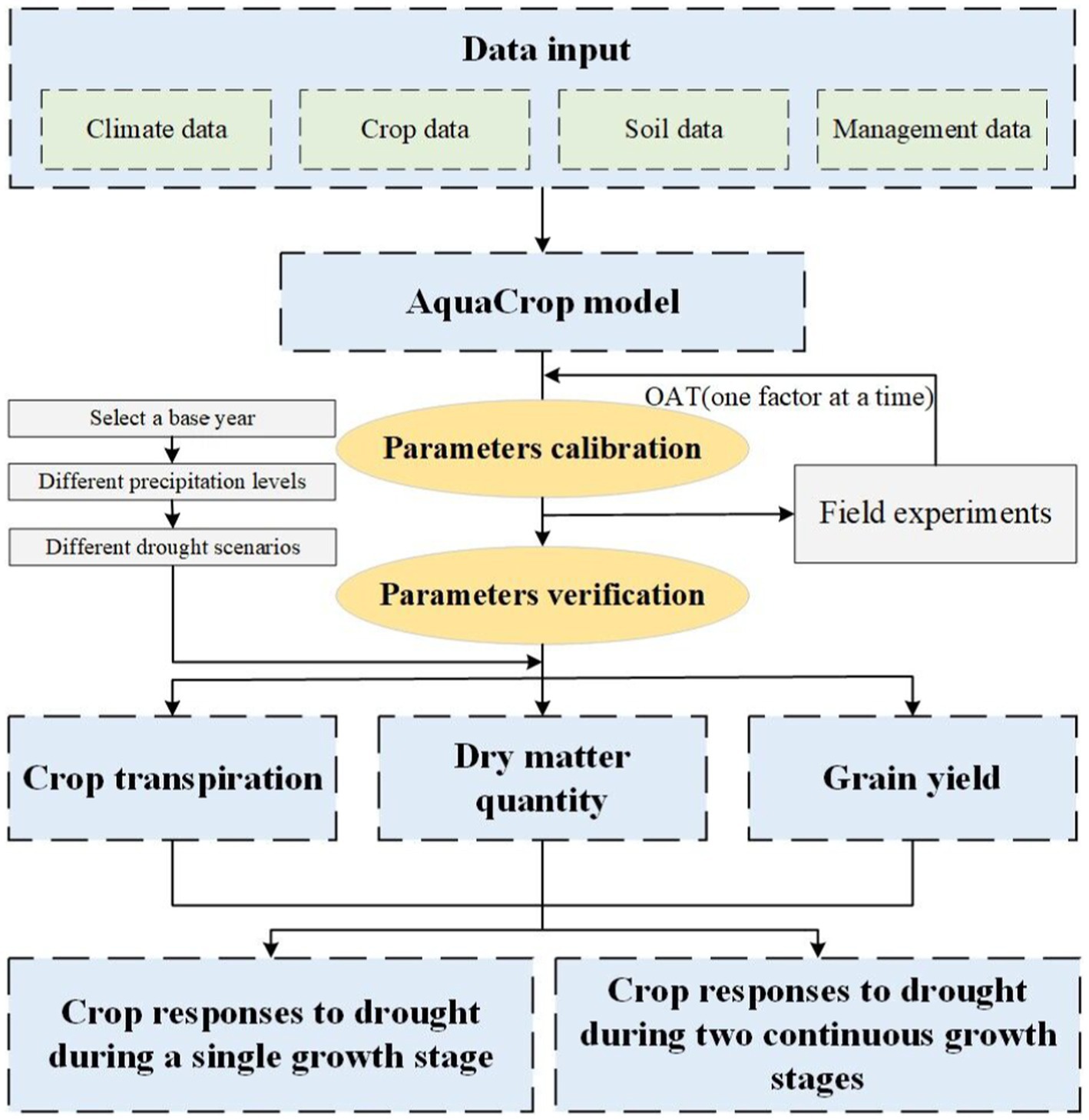
Figure 1. Flowchart of the study on quantitative responses of maize to continuous drought stress at two growth stages.
2.1 Study area
The study is based on two seasons of field experiment data from the Xinmaqiao Agricultural Water Experiment Station. The experiment station is located in the Xinmaqiao Town, Bengbu City, Huaibei Plain (117°22′E, 33°09′N), on the north bank of the middle reaches of the Huai River (Figure 2). The average elevation of the station is 19.7 m, the annual average temperature is 14.9°C, the annual sunshine time is 2,170 h, and the annual average precipitation is 911 mm and the evapotranspiration is 916 mm. The soil in the station is the typical Shajiang black soil of the Huaibei Plain, which is clayey, poorly structured, solid, fissure-developed, with poor soil water retention, and susceptible to drought (Cui et al., 2019). The site has sufficient sunshine to meet the needs of summer maize production. However, because the station is located in the climatic transition zone between the north and south, there are large inter-annual variations in precipitation and uneven distribution within a year. As a result, drought disasters frequently occur and seriously restrict the maize production. Accordingly, the natural geographic conditions and maize production of this station are typical of the Huaibei Plain region. Therefore, the selected area in this study is representative.
2.2 Summer maize field experiments
Summer maize experiments are conducted at the Xinmaqiao Experiment Station. The plants are cultivated in pit plots, which are sown on June 9 and harvested on September 21 in 2017. The size of each plot is 2.00 m (length) × 3.33 m (width) × 2.30 m (depth), and the sowing density is 48,000 plants/hm2. After sowing, 500 g of compound fertilizer (N 15%, P2O5 15%, K2O 15%) and 200 g of urea are applied to promote the growth of seeds. In 2018, a field experiment is conducted. The plants are sown on June 15 and harvested on September 27. Each field plot has an area of 4.00 m (length) × 6.00 m (width), and the sowing density is 65,000 plants/hm2. A total of 700 g compound fertilizer and 300 g urea are applied to each field plot after sowing. In addition, three groups of replications are set up for both seasons.
The summer maize varieties in 2017 and 2018 are “Long Ping 206,” and the experimental designs are both under rain-fed conditions, the soil water content during the experimental period is only supplemented by precipitation (Figure 3). Based on the actual growth processes of maize observed in the experiments, and the whole growth period of maize is divided into four stages: seedling, jointing, tasseling, and milking stages. Furthermore, the soil water content at different depths (10, 20, 30, 40, and 60 cm) at each growth stage is measured, and the plant samples are selected randomly to measure the dry matter amount at the end of each stage and seed weight at harvest.
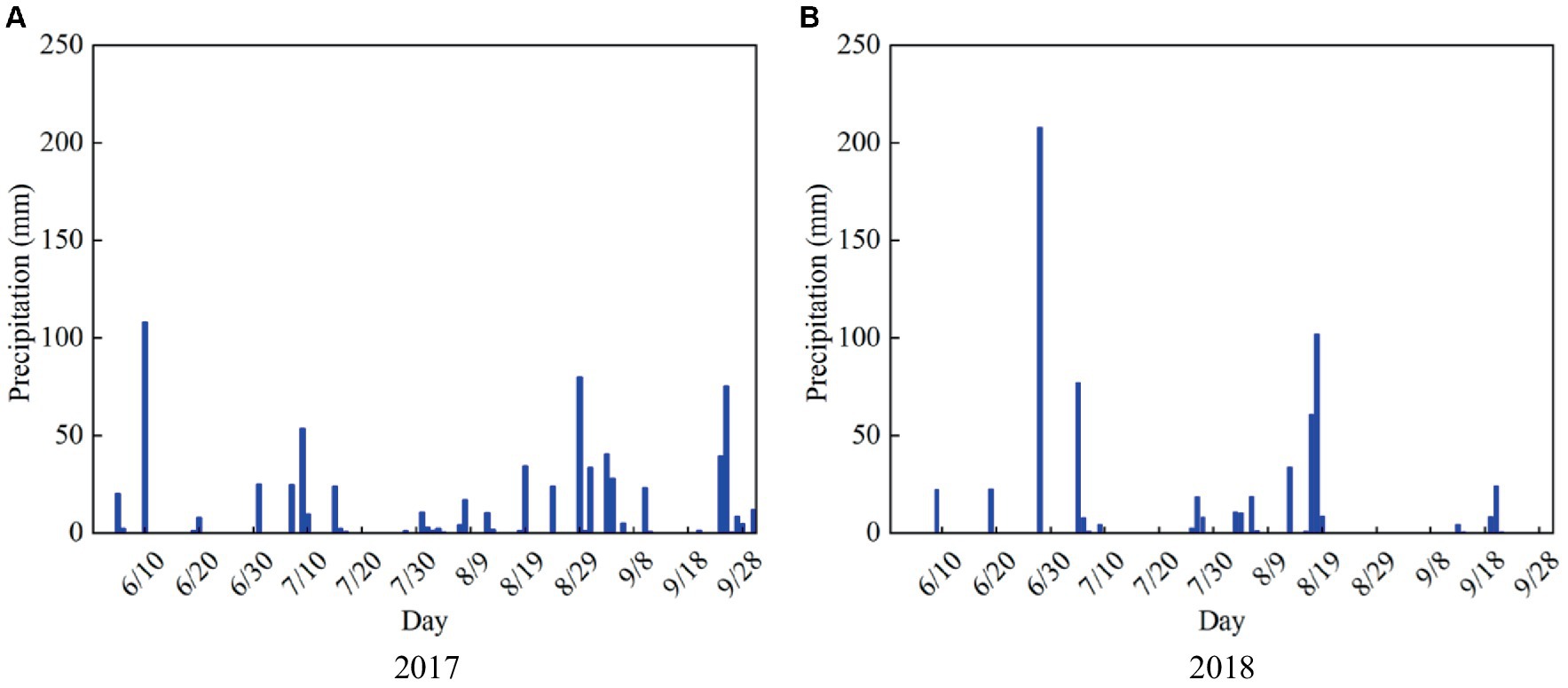
Figure 3. Daily precipitation from June to September in (A) 2017 and (B) 2018 growing seasons of summer maize in the study area.
2.3 AquaCrop model input
AquaCrop model includes a weather module, a crop module, a soil module, and a field management module (Dercas et al., 2022; Alvar-Beltrán et al., 2023). The meteorological data input to the model in this study mainly consisted of daily precipitation, maximum and minimum temperatures, relative humidity, average wind speed, sunshine duration, and CO2 concentration during the whole growth period of summer maize in 2017 and 2018. The meteorological data used are obtained from the automatic weather station located at the Xinmaqiao experiment site. The CO2 concentration is obtained using the Mauna Loa. CO2 data that provided in AquaCrop model. For the crop parameters of the model, we obtain the more sensitive crop genetic parameters first by referring to the default values in the FAO Crop Handbook (Hsiao et al., 2009), and then calibrate them using the field experimental data. The non-sensitive parameters are determined by referring to the FAO Crop Handbook and the relevant studies (Cui et al., 2021; Zhang et al., 2023). The experimental data in 2017 are used to calibrate model parameters, while those in 2018 are used for validation. The soil of the experimental station is typical Shajiang black soil in the Huaibei Plain. The AquaCrop model soil parameters are set with reference to the measured results of the experimental station and existing research of Shajiang black soil (Chen et al., 2020). Due to that two seasons of summer maize experiments are conducted under rain-fed conditions, the field management parameters meet the normal growth requirements of plant.
2.4 AquaCrop model calibration and validation
The calibration and validation of AquaCrop model is an important step in conducting scenario simulations, and the reliability and accuracy of simulated results are dominated by highly sensitive crop genetic parameters. The OAT (one factor at a time) method (Daniel, 2012) is a fast and effective method of parameter sensitivity analysis by changing only one parameter at a time and selecting it based on the correlation between the change of parameter and that of simulation result. In this study, combining the field experiment data of summer maize at Xinmaqiao Experimental Station in 2017 with existing research (Cui et al., 2022), and referring to the FAO Crop Handbook (Hsiao et al., 2009), the OAT method is adopted to calibrate some of the sensitive crop genetic parameters using dry matter amount and grain yield as the objective functions and to derive locally adapted model parameters. Furthermore, the observed results of summer maize field experiment in 2018 are used to verify the calibrated AquaCrop model.
2.5 Data analysis
To evaluate the reliability of the calibrated AquaCrop model, R-Square (R2) Equation 1, absolute relative error (ARE) Equation 2, and relative root mean square error (RRMSE) Equation 3 are used, ensuring the accuracy of model parameters (Lu et al., 2022):
where n is the number of samples of the output variable (dry matter amount during the growth process of maize in this study); Simi is the simulated value of dry matter amount for the ith sample (i = 1, 2, 3, 4, 5) of the AquaCrop model; Obsi is the observed value of the ith sample from the maize field experiments; is the average value of all samples of the output variable. The closer the value of R2 is to 1, the more accurate the simulation results are.
where k is the number of samples of the output variable (dry matter amount and grain yield at harvest of maize in this study); Simi is the simulated value of dry matter amount (or grain yield) at harvest for the ith sample (i = 1, 2 indicates 2017 and 2018 seasons, respectively) of the AquaCrop model; Obsi is the observed value of the ith sample from the maize field experiments. The smaller the value of ARE or RRMSE, the closer the simulated value is to the observed result and the more accurate the calibrated AquaCrop model is.
2.6 Drought stress scenarios setting
To quantify the responses of maize to continuous drought stress at two growth stages, the calibrated and validated AquaCrop model is used to simulate the whole growth processes of maize under various drought stress scenarios.
The continuous drought stress scenarios at two growth stages of maize designed in this study are as follows: Firstly, the years without drought during the whole growth period of summer maize (June–September) from 1960 to 2019 are selected based on the results of drought identification in the study area (Bengbu City) (Wei et al., 2021). Then, combined with the water consumption of summer maize at each growth stage under sufficient water supply condition, and the year in which the precipitation and the water consumption of maize at each stage are close is considered as the base year. In this study, by analyzing and comparing, 2015 year is chosen as the base year, and the drought stress scenarios are designed by changing the amount of precipitation at each stage of maize in this year.
Table 1 shows the continuous drought stress scenarios at two growth stages of summer maize. The scenarios are designed to set three situations of no drought, light drought, and serious drought stress at one growth stage of maize, and additional three situations of no drought, light drought, and serious drought stress at the next stage, for a total of 21 scenarios. Moreover, no drought is defined as 100% of the precipitation in the base year, light and serious drought are defined as 70% and 40% of the precipitation in the base year, respectively. The actual meteorological data (maximum and minimum temperatures, relative humidity, average wind speed, etc.) in the base year and the precipitation data under various scenarios are input into the calibrated and validated AquaCrop model to simulate the whole growth processes of summer maize under different continuous drought conditions at two growth stages.
3 Results and discussion
3.1 Calibration and validation results of AquaCrop model
This study combines the research on AquaCrop model parameters (Li et al., 2022), selects the sensitive crop genetic parameters of summer maize, and uses the OAT method to calibrate (2017) and validate (2018) the parameters (Table 2). The soil water content, dry matter amount, and grain yield of maize are used as the outputs. Furthermore, the accuracy of the AquaCrop model parameters after calibration are evaluated using the ARE and RRMSE.
The comparison between the simulated and observed results of relative soil water content during the growth process of maize in 2017 and 2018 are shown in Figure 4. It can be seen that the simulated and measured values of soil water content at different growth stages of maize in the two seasons are similar, meanwhile, the trends and distributions of soil water content at different depths (10, 20, 30, 40, and 60 cm) are basically consistent.
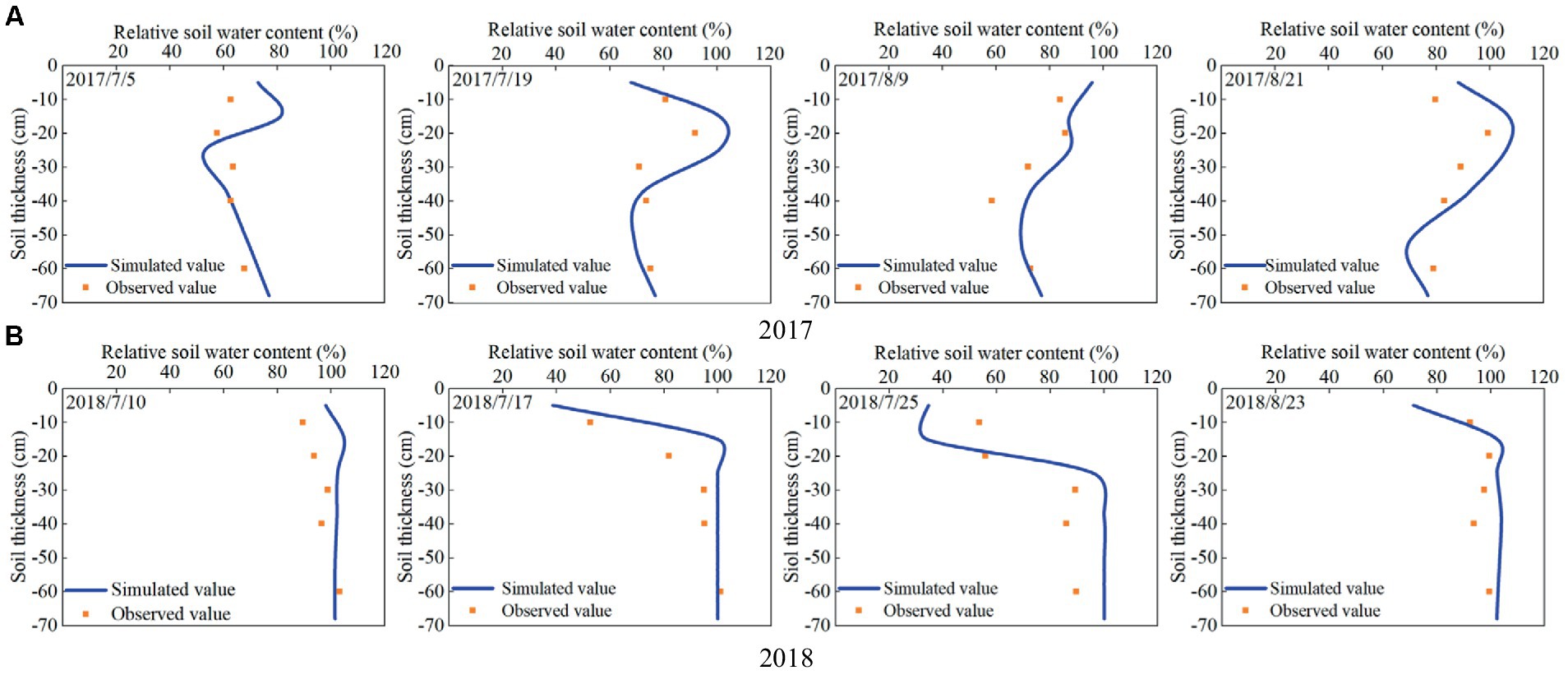
Figure 4. Simulated and observed relative soil water content during the growth process of summer maize in (A) 2017 and (B) 2018.
The simulated and observed results of dry matter amount during the whole growth period of maize in 2017 and 2018 are shown in Figure 5. It indicates that the simulated dry matter amount in the two seasons are close to the observed values, with R2 values of 0.91 and 0.96, respectively. The values of ARE between the simulated and observed dry matter amount at harvest are 2.55 and 7.15%, respectively, and the RRMSE is 5.54% (Table 3). Thus, the calibrated AquaCrop model accurately simulates the growth process of maize, and the model parameters are reliable.
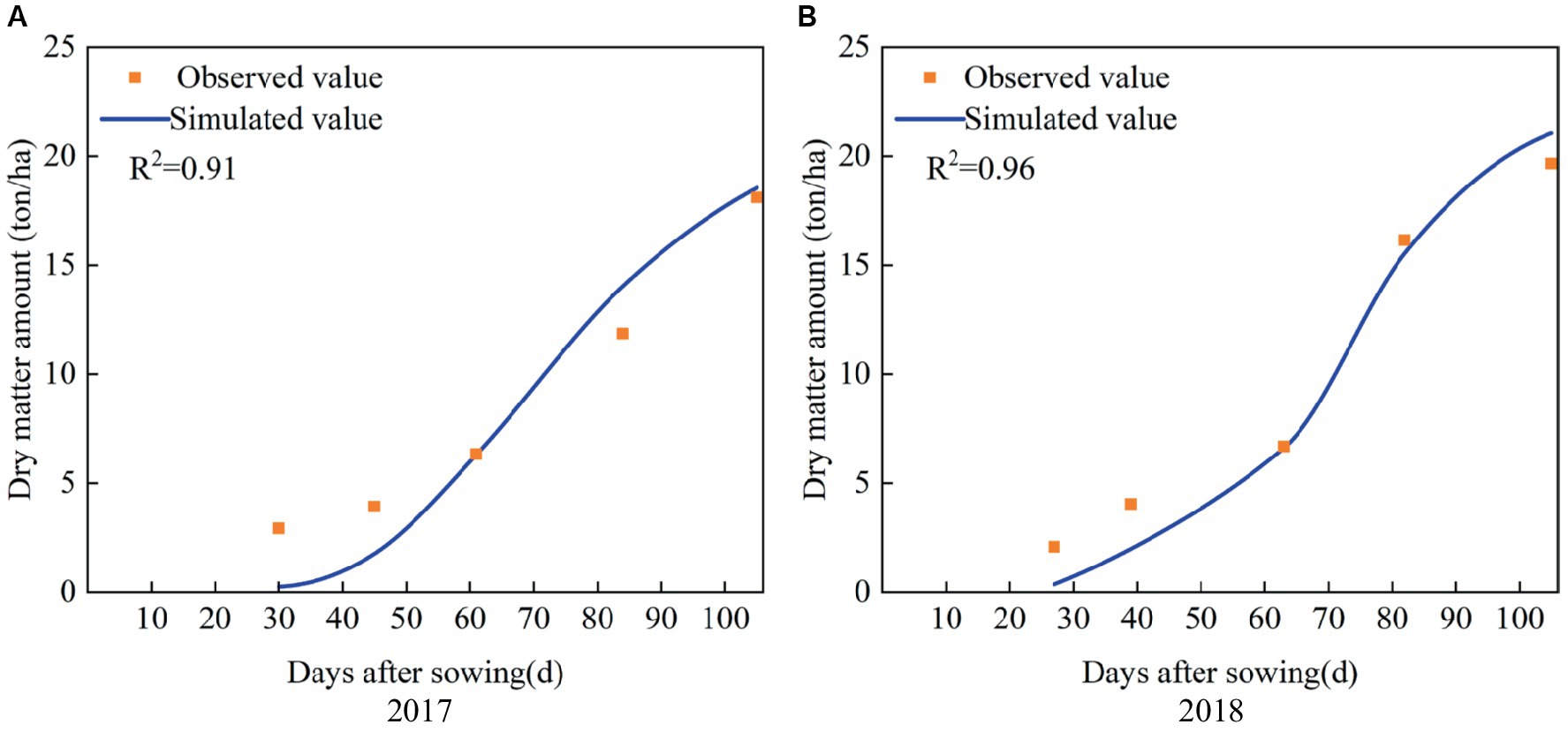
Figure 5. Simulated and observed dry matter amount during the whole growth period of summer maize in (A) 2017 and (B) 2018.

Table 3. The values of ARE and RRMSE between the simulated and observed dry matter amount at harvest and gain yield of summer maize.
The values of ARE between the simulated and observed grain yield in 2017 and 2018 are 0.21 and 1.99%, respectively, and the RRMSE is 1.51% (Table 3). There is a high simulation accuracy using the AquaCrop model.
It can be seen that the calibrated and validated AquaCrop model can accurately simulate the water supply and demand process of summer maize, and the obtained genetic parameters of maize in the Huaibei Plain are reasonable, which ensures the simulation reliability of the growth processes of maize under drought stress scenarios subsequently.
AquaCrop model has a high fit in simulating maize evapotranspiration, biomass, and yield under different water conditions in the Nebraska (Lu et al., 2022), Uganda (Zizinga et al., 2024), North China Plain (Wu et al., 2024), and Huaibei Plain (Cui et al., 2022). However, Zhou et al. (2024) found that the difference in the total dried yield when using the rain-fed treatment was obvious in 2018 for the North China Plain by AquaCrop model. The reason is that the climate in this area in 2018 is an extreme outlier, with little rainfall and frequent strong winds, while the model cannot take these factors into account. In this study, the precipitation is not extreme (Figure 3) and climate is normal in 2018 for the Huaibei Plain, thus the simulated dry matter and grain yield are close to the measured values (Table 3). Accordingly, the extreme or unusual climatic conditions should be considered when adopting AquaCrop model.
3.2 Quantitative response of maize transpiration to drought stress
3.2.1 Response of transpiration to drought stress at a single growth stage
Figure 6 shows the transpiration (Tr) under continuous light and serious drought at two growth stages of maize. (1) Maize suffers light and serious drought at the seedling stage, Tr at this single stage decreases by 40.00 and 78.57%, respectively, compared with that under no drought (Figure 6A); Tr decreases by 20.00 and 44.26% at the jointing stage, respectively (Figure 6B); Tr decreases by 5.45 and 15.67% at the tasseling stage, respectively (Figure 6C); and Tr decreases by 2.00 and 10.44% at the milking stage, respectively (Figure 6C). The proportion of Tr reduction under drought condition is the highest at the seedling stage, followed by the jointing stage, tasseling stage, and milking stage. It indicates that drought at a certain growth stage of maize causes a reduction in plant transpiration at this stage, and the more serious the drought stress, the more obvious the reduction.
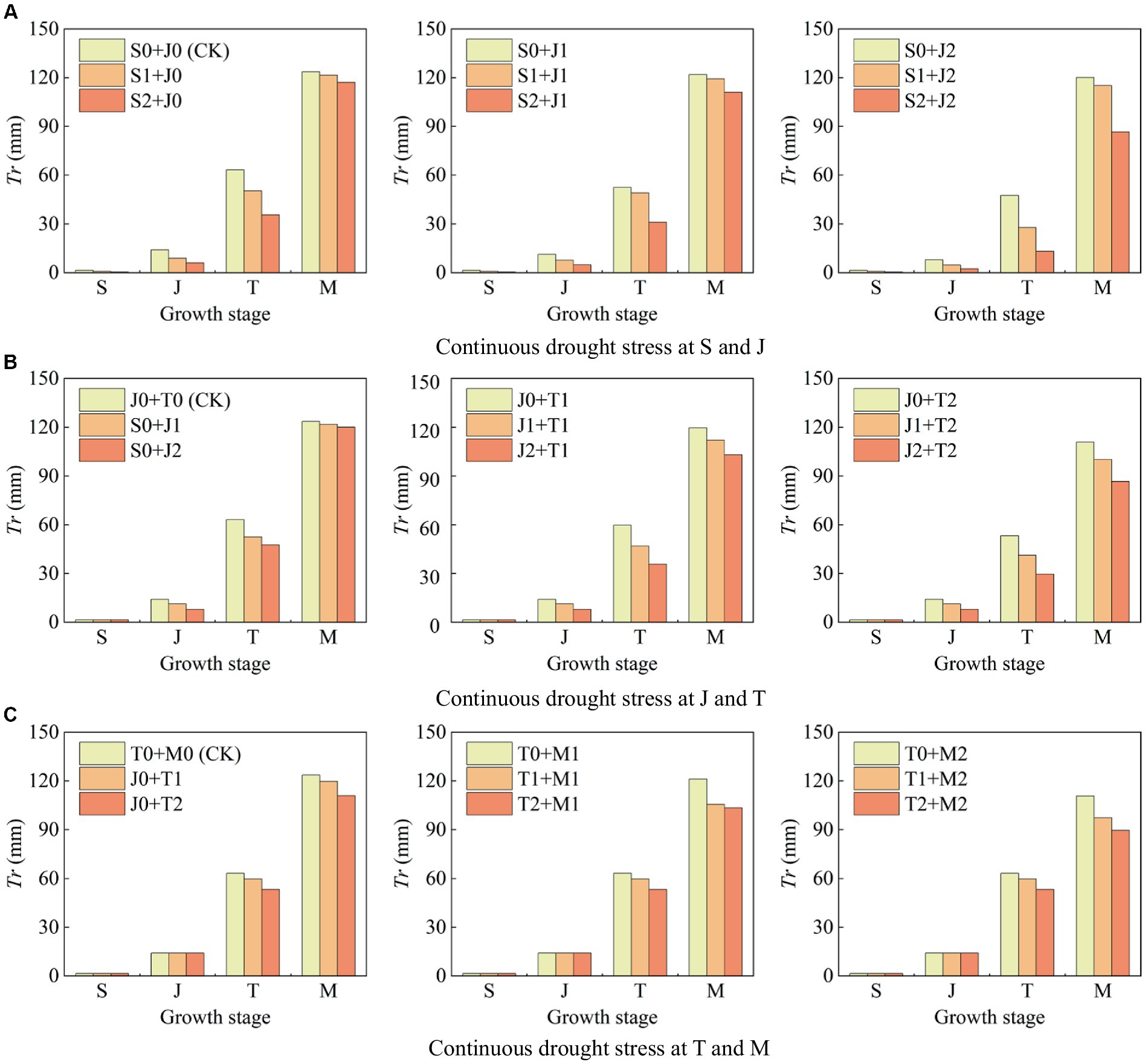
Figure 6. Transpiration (Tr) at each growth stage of summer maize under continuous drought stress at (A) S and J, (B) J and T, and (C) T and M (S, seedling stage; J, jointing stage; T, tasseling stage; M, milking stage; and 0, no drought stress; 1, light drought stress; 2, serious drought stress).
The effect of drought at the seedling stage is the most severe. Tang et al. (2018) showed that maize canopy increased significantly after 40 d of planting, reflecting that the transpiration was vigorous during the seedling and jointing stages, thus the transpiration was markedly affected by drought at these two stages. Yuan et al. (2019) found that drought caused the close of leaf stomata of summer maize, which resulted in the reduction of plant transpiration, and the reduction increased with the intensity of drought. These results are consistent with the findings of this study. (2) Serious drought at the seedling stage causes the reduction of Tr at the jointing stage, the tasseling stage, and the milking stage by 57.45, 43.61, and 5.24%, respectively (Figure 6A); serious drought at the jointing stage reduces Tr at the tasseling stage and the milking stage by 24.68 and 2.84% (Figure 6B); and serious drought at the tasseling stage reduces Tr at the milking stage by 10.29% (Figure 6C). It indicates that when maize is subjected to drought at a growth stage, the drought not only affects plant transpiration at this stage, but also that the drought impact is continuous and causes the reduction of Tr at the subsequent stages, which is the after-effect of drought.
Similarly, Hao et al. (2010) found that drought at the early stage of maize had a lasting impact on transpiration at the later stage. Cui et al. (2020) conducted pot experiments and discovered that when soybean suffered from mild drought at the seedling stage, the evapotranspiration at the branching stage, the flowering-podding stage, and the seed-filling stage reduced by 14.80, 13.23, and 6.12%, respectively, compared with those under full irrigation.
3.2.2 Response of transpiration to continuous drought stress at two growth stages
It can be found that drought at a growth stage of maize has an after-effect on Tr in the following stages (Figure 6). Therefore, the impact of continuous drought stress should be fully reflected by the change in Tr during the whole growth period. Figure 7 shows the reduction rate of Tr under continuous drought stress at two growth stages of maize.
1. The reduction rate of Tr during the whole growth period is 21.37% when the maize is subjected to serious drought at the seedling stage, and that is 12.53% under serious drought at the jointing stage; while the reduction rate of Tr under continuous serious drought at these two stages is 49.40%, which is higher than the sum of the reduction rates caused by serious drought at the two single stages (>21.37% + 12.53%) (Figure 7A). The reduction rate of Tr under continuous serious drought at the jointing stage and the tasseling stages is 37.96% (>12.53% + 11.18%) (Figure 7B). Furthermore, the reduction rate of Tr under continuous serious drought at the tasseling stage and the milking stages is 21.75% (>11.18% + 6.38%) (Figure 7C). It indicates that the adverse effect of continuous drought at two growth stages on Tr basically exceeds the sum of the effects of drought at the two single stages for summer maize. Moreover, continuous serious drought at the seedling and jointing stages has the most obvious impact on Tr.
2. The reduction rate of Tr is 10.23% when the maize is subjected to light drought at the seedling stage, and that is 7.52% under light drought at the jointing stage; while the reduction rate of Tr under continuous light drought at these two stages is 12.56%, which is lower than the sum of the reduction rates caused by light drought at the two single stages (<10.23% + 7.52%). Similarly, for serious drought at the seedling stage, the reduction rate of Tr is 21.37, and for light drought at the jointing stage, the reduction rate of Tr is 7.52%, while for serious drought at the seedling stage and light drought at the jointing stage, the reduction rate of Tr is 27.29% (<21.37% + 7.52%). It shows that the negative effect of continuous drought at the seedling and jointing stages on Tr is less than the sum of the effects of drought at the two single stages. It can be explained that drought at the seedling stage has endowed the maize with an adaptability to drought, which alleviates the negative effect of light drought at the jointing stage on transpiration. However, the adaptability has disappeared when the plant encounters serious drought at the jointing stage. For instance, as shown in Figure 7A, the reduction rate of Tr under continuous drought at the seedling and jointing stages is larger than the sum of the reduction rates of Tr under drought at the two single stages for maize.
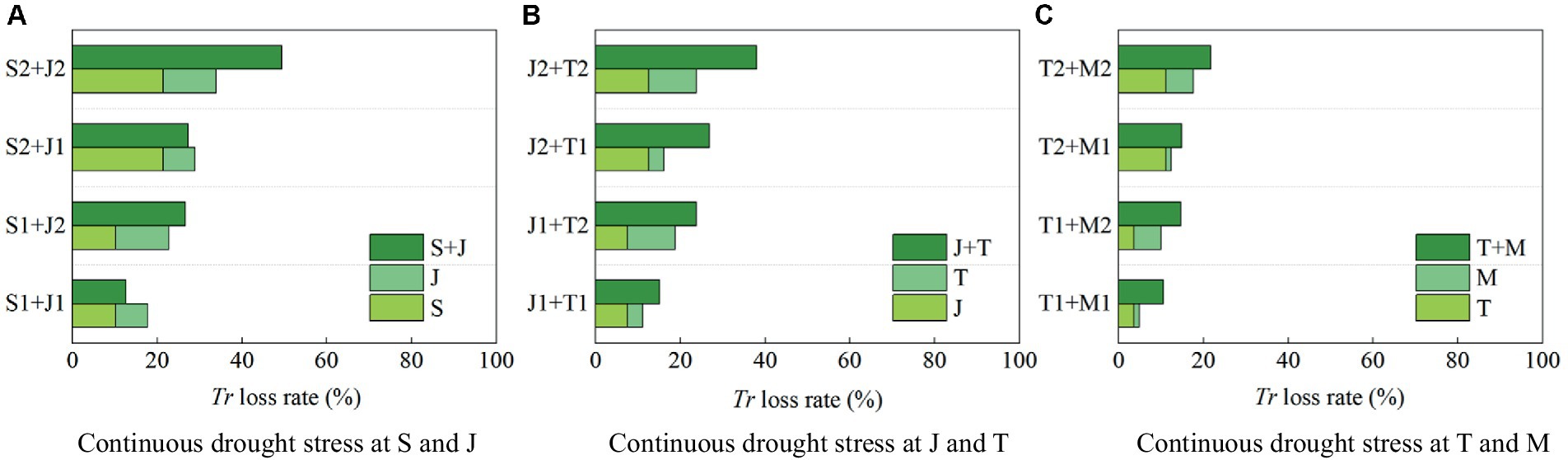
Figure 7. The reduction rates of transpiration (Tr) during the whole growth period of summer maize under continuous drought stress at (A) S and J, (B) J and T, and (C) T and M (S, seedling stage; J, jointing stage; T, tasseling stage; M, milking stage; and 0, no drought stress; 1, light drought stress; 2, serious drought stress).
The reduction in maize transpiration caused by drought is primarily due to decreased stomatal conductance (Kinoshita et al., 2021) and an impaired photosynthetic apparatus (Munnus and Millar, 2023). Additionally, there are a series of adaptive changes in physiology and metabolism after early drought for maize (Ru et al., 2023), and the leaves have the ability to recover rapidly but the ability is limited (Yao et al., 2012). Nevertheless, when the plant is subjected to severe drought at a growth stage or continuous serious drought, it may lead to that some photosynthetic apparatus and functions are difficult to recover and irreversibly damaged. Therefore, in this study, drought at a growth stage of summer maize causes reductions of transpiration at the subsequent stages. Meanwhile, compared with the impact on transpiration caused by drought at a single growth stage, continuous drought has a more serious effect.
3.3 Quantitative response of maize dry matter accumulation to drought stress
3.3.1 Response of dry matter accumulation to drought stress at a single growth stage
Figure 8 shows the dry matter accumulation at each growth stage of maize under different continuous drought stress.
1. The dry matter accumulation of maize under light drought and serious drought at the seedling stage decreases by 40.61 and 58.10%, respectively, compared to that with no drought (Figure 8A). Moreover, the values are 17.94 and 30.05% for the jointing stage (Figure 8B), 9.68 and 18.76% for the tasseling stage (Figure 8C), and 7.33 and 13.02% for the milking stage, respectively (Figure 8C). It can be seen that the reduction proportion of dry matter accumulation caused by drought at the seedling stage is the greatest, followed by the jointing stage, the tasseling stage, and the milking stage. It indicates that drought at a growth stage of maize causes a reduction in dry matter accumulation at this stage, and the more serious the drought, the larger the reduction. Photosynthesis is the source of dry matter for maize (Peng et al., 2023b). Zhang et al. (2018) found that under water deficit condition, the dry matter of maize is decreased due to the inhibition of light capture, which is consistent with the results of this study.
2. Serious drought at the seedling stage reduces the dry matter accumulation at the jointing, the tasseling stage, and the milking stage by 56.76, 49.28, and 23.82%, respectively (Figure 8A); serious drought at the jointing stage reduces the dry matter accumulation at the tasseling stage and the milking stage by 26.69 and 24.00%, respectively (Figure 8B); serious drought at the tasseling stage reduces the dry matter accumulation at the milking stage by 12.82% (Figure 8C). It reflects that drought at a growth stage of summer maize does not only affect the plant growth at this stage, but the negative impact is continuous, resulting in the reductions of biomass at the following stages. The serious drought at the seedling stage has the most severe impact on maize growth at the jointing stage.
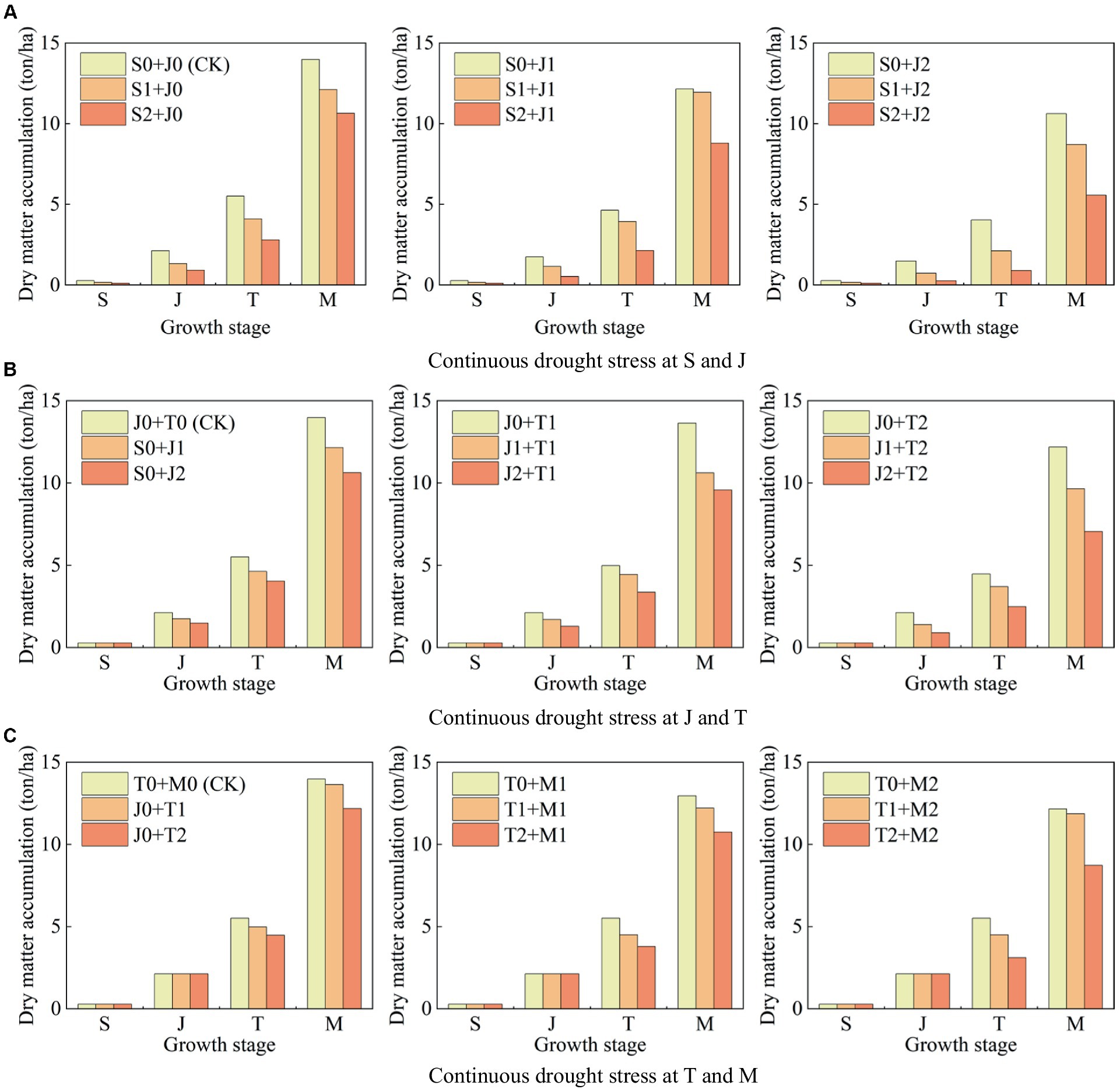
Figure 8. Dry matter accumulation at each growth stage of summer maize under continuous drought stress at (A) S and J, (B) J and T, and (C) T and M (S, seedling stage; J, jointing stage; T, tasseling stage; M, milking stage; and 0, no drought stress; 1, light drought stress; 2, serious drought stress).
Consistent with this study, Hao et al. (2010) found that maize senescence was accelerated by serious drought in the late seedling and early jointing stages. In addition, Cui et al. (2020) obtained that when soybean was under mild drought at the seedling stage, the biomass accumulations at the branching stage and the flowering-podding stage reduced by 24.01 and 11.49%, respectively, compared with those under no drought stress.
3.3.2 Response of dry matter accumulation to continuous drought stress at two growth stages
It can be seen that drought at a growth stage of summer maize has an after-effect on dry matter accumulation at the later stages, so the dry matter amount at harvest can be used to analyze the impact of continuous drought more accurately. Figure 9 shows the loss rates of dry matter amount at harvest under different scenarios of continuous drought stress at two growth stages for summer maize, compared with that under no drought stress.
1. The loss rate of dry matter amount at harvest is 33.85% when the maize encounters serious drought at the seedling stage, and that is 24.97% when the plant is subjected to serious drought at the jointing stage; while the loss rate under continuous serious drought at these two stages is 68.74%, which is higher than the sum of the loss rates caused by serious drought at the two single stages (>33.85% + 24.97%) (Figure 9A). The loss rates of dry matter amount are 24.97 and 12.91% under serious drought at the jointing stage and the tasseling stage, respectively, while the loss rate under continuous serious drought at these two stages is 51.13% (>24.97% + 12.91%) (Figure 9B). Moreover, the loss rates of dry matter amount are 12.91 and 8.32% for serious drought at the tasseling stage and the milking stage, respectively, while the loss rate for continuous serious drought at these two stages is 35.06% (>12.91% + 8.32%) (Figure 9C). It shows that the adverse impact on biomass of continuous drought at two growth stages is basically more serious than the sum of the impacts of drought at the two single stages for maize. Furthermore, the most severe impact is caused by continuous serious drought at the seedling and jointing stages.
2. The loss rates of dry matter amount at harvest are 19.13 and 14.13% under light drought at the seedling stage and the jointing stage, respectively, while the loss rate under continuous light drought at these two stages is 21.46%, which is less than the sum of the loss rates induced by light drought at the two single stages (<19.13% + 14.13%). Additionally, the loss rate under serious drought at the seedling stage is 33.85, and that under light drought at the jointing stage is 14.13%, while the loss rate caused by serious drought at the seedling stage and light drought at the jointing stage is 47.10% (<33.85% + 14.13%). It reflects that a degree of adaptability to drought for maize has been promoted by drought at the seedling stage, alleviating the impact of light drought at the jointing stage. However, the adaptability has disappeared when the drought at the jointing stage becomes serious.
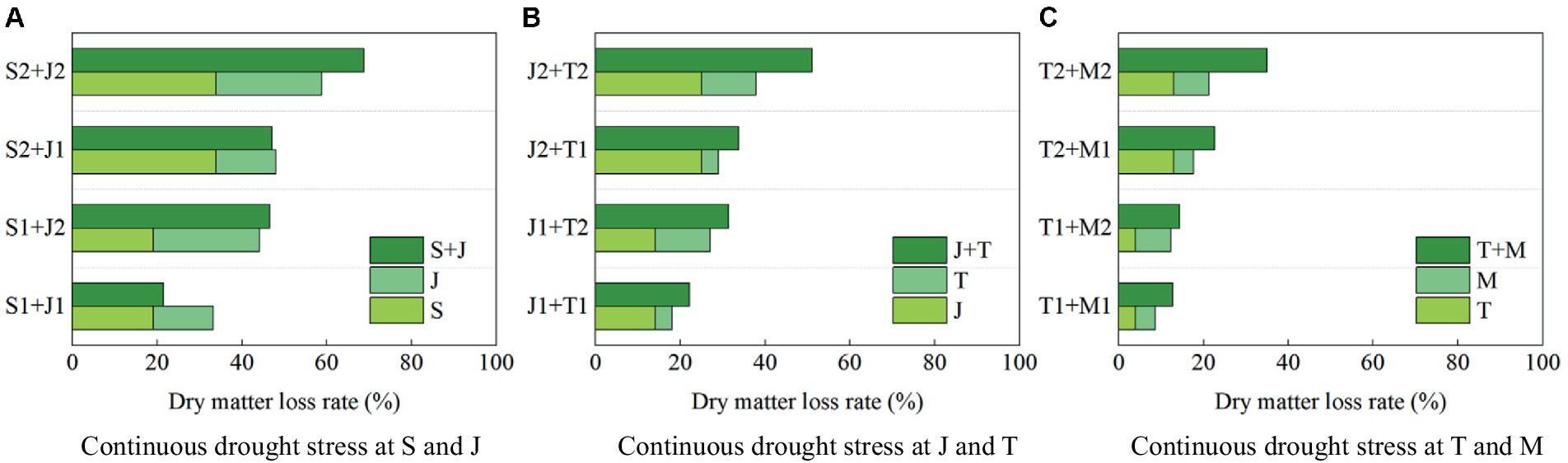
Figure 9. The loss rates of dry matter amount at harvest of summer maize under continuous drought stress at (A) S and J, (B) J and T, and (C) T and M (S, seedling stage; J, jointing stage; T, tasseling stage; M, milking stage; and 0, no drought stress; 1, light drought stress; 2, serious drought stress).
Drought at different growth stages affects crop biomass accumulation differently. Yao et al. (2012) found that dry matter accumulation of maize was adaptive to drought, but it was detrimental to plant growth if the drought was severe. In addition, drought tolerance is limited at the seedling stage of maize, which is due to that during this stage the growth and development of plant has just began, and part physiological functions have not completed. Therefore, serious drought at the seedling stage has the greatest after-effect on plant growth and biomass accumulation of maize.
Drought accelerates the leaf senescence and decreases the photosynthetic capacity, thus impeding dry matter accumulation for summer maize (Hu et al., 2023). The substantial changes in biochemical and physiological processes under drought conditions decelerate or halt the growth and cause biomass suppression (Gupta et al., 2020). These are consistent with the lasting reductions of dry matter amount caused by drought at a growth stage and exceeded reductions caused by continuous drought for maize. Furthermore, among the initial response to drought is stomatal closure, which triggers a series of biochemical and physiological reactions to boost plant defense mechanisms (including antioxidant formation and increased osmolyte accumulation) and maintain the photosynthetic rate (Gusain et al., 2024; Sato et al., 2024). These explain the adaptability of maize plant induced by drought at the seedling stage.
3.4 Quantitative response of maize yield to drought stress
3.4.1 Response of yield to drought stress at a single growth stage
The yield loss rates of summer maize under continuous drought stress at two growth stages are shown in Figure 10. The yield loss rates induced by light and serious drought at the seedling stage are 18.80 and 33.30, respectively, compared with that with no drought (Figure 10A), those for the jointing stage are 12.78 and 24.16%, respectively (Figure 10B), those for the tasseling stage are 3.65 and 12.22%, respectively (Figure 10C), and those for the milking stage are 1.02 and 7.44%, respectively (Figure 10C). The yield loss rates is largest under drought conditions at the seedling stage, followed by the jointing stage, the tasseling stage, and the milking stage. Thus drought at a growth stage of maize causes the yield loss, and the more serious the drought, the more significant the loss.
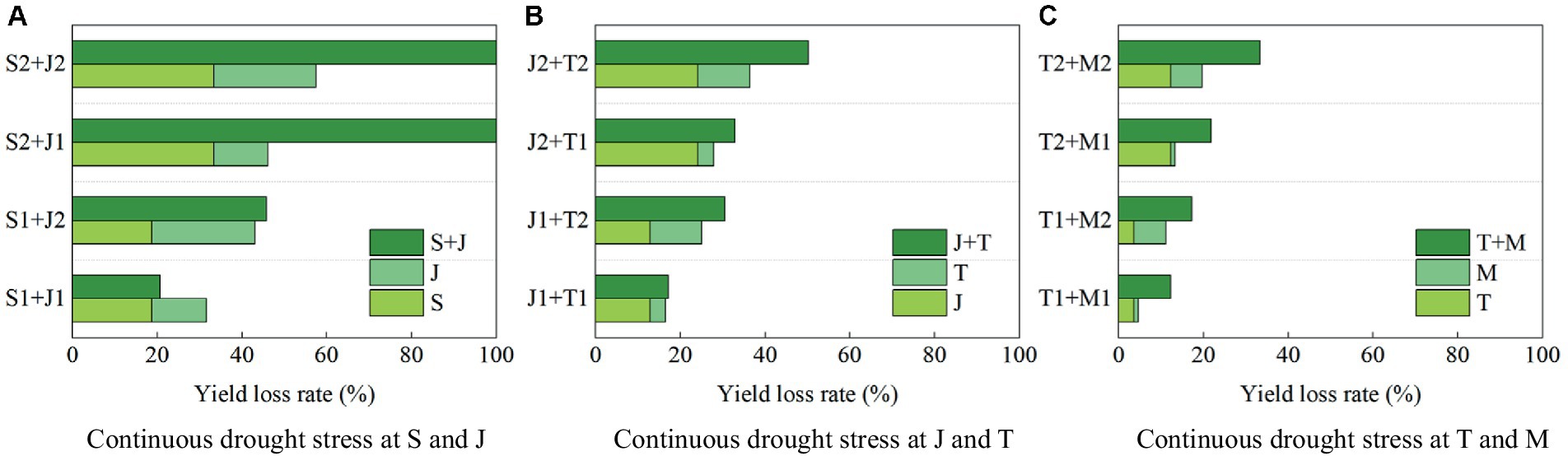
Figure 10. The yield loss rates under continuous drought stress of summer maize at two growth stages at (A) S and J, (B) J and T, and (C) T and M (S, seedling stage; J, jointing stage; T, tasseling stage; M, milking stage; and 0, no drought stress; 1, light drought stress; 2, serious drought stress).
3.4.2 Response of yield to continuous drought stress at two growth stages
When the maize is subjected to serious drought at the seedling stage and the jointing stage, the yield loss rates are 33.30 and 24.16%, respectively, while when the plant encounters continuous serious drought at these two stages, the loss rate is 100%, which is higher than the sum of the loss rates induced by drought at the two single stages (>33.30% + 24.16%) (Figure 10A). When the maize suffers serious drought at the jointing stage and the tasseling stage, the yield loss rates are 24.16 and 12.12%, respectively, while the loss rate under continuous serious drought at these two stages is 50.16% (>24.16% + 12.12%) (Figure 10B). Furthermore, serious drought at the tasseling stage and the milking stage reduce yield by 12.22 and 7.44%, respectively, while continuous serious drought at these two stages causes yield loss by 33.28% (>12.22% + 7.44%) (Figure 10C). It shows that the negative impact on yield formation of continuous drought at two stages for maize is basically more severe than the sum of the impacts induced by drought at the two single stages. In addition, the maximum yield loss rate is caused by continuous serious drought at the seedling and jointing stages, reaching 100%. The loss rate is 50.16% due to continuous serious drought at the jointing and tasseling stages. These can be explained that the growth of maize at the seedling stage is vigorous, and at this period the reproductive organs have not developed and have less resistance to drought stress, so drought at the seedling and jointing stages causes serious yield loss, which is consistent with the study of Zou et al. (2023).
The yield loss rate are 18.80 and 12.78% when the maize is subjected to light drought at the seedling stage and the jointing stage, respectively, while the loss rate caused by continuous drought at these two stage is 20.66% (<18.80% + 12.45%). It reflects that the negative impact of continuous light drought at the seedling and jointing stages on maize yield formation is less than the sum of the impacts of drought induced by the two single stages. The maize plant has been adaptive to drought at the jointing stage after light drought at the seedling stage. In addition, serious drought at the seedling stage reduces yield by 100% regardless of light or serious drought at the jointing stage, indicating that serious drought at the seedling stage causes irreversible impact on the growth of maize. It is also mentioned in the study of Liu (2015), who found that appropriate drought at the seedling stage could promote maize growth, but prolonged or serious drought stress had caused serious yield reduction or even no harvest.
Maize is tall and broad-leaved, extreme drought at planting could cause leaves to curl and stunt growth. Additionally, high temperatures caused by drought could inhibit the induction of flowering in male flowers and cause factors that interfere with pollen development (Kim and Lee, 2023). These are consistent with the high yield losses caused by drought at the seedling stage and the tasseling stage for summer maize in this study. Furthermore, Hou et al. (2024) found that concurrent drought would induce larger yield gaps, which would be, on average, 2–30% higher than single-type drought, which is consistent with the exceeded reduction of maize yield caused by continuous drought.
The after-effect of drought at different growth stages of summer maize on dry matter partitioning (DMP) are various, and that of drought during vegetative period enlarges and drastically induces the reduction of harvest index (HI), which is larger than that of drought during reproductive period, demonstrating obvious alleviation in the previous adverse impacts on DMP and HI (Cai et al., 2023). In addition, the resilience of maize is strong after rewatering under light drought then leaf biomass and root growth could be enhanced, thus improving yield (Jing et al., 2023). These explain the larger grain yield losses caused by drought at the seedling stage and the joint stage (vegetative period) than those caused by drought at the tasseling stage and the milking stage (reproductive period), and also the less yield loss caused by continuous light drought at the seedling and jointing stages in this study.
4 Conclusion
In this study, the quantitative responses of plant transpiration, dry matter accumulation, and yield formation to continuous drought stress at two growth stages of summer maize are analyzed. The main conclusions are as follows:
1. Drought stress at a single growth stage of summer maize has negative impacts on plant transpiration and biomass accumulation at this stage, and the more serious the drought stress, the more significant the impacts. Meanwhile, drought stress at a stage has after-effects on maize transpiration and biomass at the subsequent stages.
2. The impact of continuous drought stress at two growth stages of maize generally exceeds the sum of the impacts of drought at the two single stages, especially continuous serious drought at the seedling and jointing stages, which causes the most severe accumulative effects on plant transpiration, biomass accumulation, and yield formation.
3. Drought at the seedling stage promotes the adaptability of maize, alleviating the negative impacts of light drought at the jointing stage, while the adaptability disappears when drought at the jointing stage becomes serious.
Therefore, in the actual production of summer maize, serious drought at the seedling stage should be avoided to ensure seed survival, and meanwhile, continuous drought stress, especially that at the seedling and jointing stages should be prevented to reduce the accumulative effect and unrecoverable impact. It is conducive to conduct drought disaster prevention planning and irrigation allocation, ensuring normal growth and reduce drought losses of maize. Additionally, climate change impacts (e.g., CO2 concentration and climate pattern) could be considered in crop growth simulation, field experiments of continuous drought would be carried out if the natural climate meets the conditions, and a more detailed growth stage division of maize in setting continuous drought stress scenarios in future work.
Data availability statement
The original contributions presented in the study are included in the article/supplementary material, further inquiries can be directed to the corresponding author.
Author contributions
YC: Formal analysis, Funding acquisition, Methodology, Writing – original draft. HT: Formal analysis, Investigation, Visualization, Writing – original draft. YZ: Funding acquisition, Methodology, Supervision, Writing – review & editing. JJ: Conceptualization, Funding acquisition, Supervision, Writing – review & editing. SJ: Investigation, Supervision, Writing – review & editing.
Funding
The author(s) declare that financial support was received for the research, authorship, and/or publication of this article. This study was supported by the National Natural Science Foundation of China (Grant nos. 52109009, U2240223, 42271084, and 52379006), the Natural Science Foundation of Anhui Province, China (Grant no. 2308085US06), and the Fundamental Research Funds for the Central Universities (Grant no. JZ2024HGTB0208).
Acknowledgments
The authors sincerely appreciate the reviewers and editors comments to improve the manuscript.
Conflict of interest
The authors declare that the research was conducted in the absence of any commercial or financial relationships that could be construed as a potential conflict of interest.
Publisher’s note
All claims expressed in this article are solely those of the authors and do not necessarily represent those of their affiliated organizations, or those of the publisher, the editors and the reviewers. Any product that may be evaluated in this article, or claim that may be made by its manufacturer, is not guaranteed or endorsed by the publisher.
References
Ali, S. A., Khatun, R., Ahmad, A., and Ahmad, S. N. (2020). Assessment of cyclone vulnerability, hazard evaluation and mitigation capacity for analyzing cyclone risk using GIS technique: a study on Sundarban biosphere reserve, India. Earth Syst. Environ. 4, 71–92. doi: 10.1007/s41748-019-00140-x
Alvar-Beltrán, J., Saturnin, C., Grégoire, B., Camacho, J. L., Dao, A., Migraine, J. B., et al. (2023). Using AquaCrop as a decision-support tool for improved irrigation management in the Sahel region. Agric. Water Manag. 287:108430. doi: 10.1016/j.agwat.2023.108430
Cai, F., Mi, N., Ming, H., Zhang, Y., Zhang, H., Zhang, S., et al. (2023). Responses of dry matter accumulation and partitioning to drought and subsequent rewatering at different growth stages of maize in Northeast China. Front. Plant Sci. 14:1110727. doi: 10.3389/fpls.2023.1110727
Chang, J., Li, Y., Wang, Y., and Yuan, M. (2016). Copula-based drought risk assessment combined with an integrated index in the Wei River basin, China. J. Hydrol. 540, 824–834. doi: 10.1016/j.jhydrol.2016.06.064
Chen, L., Li, F., Li, W., Ning, Q., Li, J., Zhang, J., et al. (2020). Organic amendment mitigates the negative impacts of mineral fertilization on bacterial communities in Shajiang black soil. Appl. Soil Ecol. 150:103457. doi: 10.1016/j.apsoil.2019.103457
Corbari, C., Charfi, I. B., and Mancin, M. (2021). Optimizing irrigation water use efficiency for tomato and maize fields across Italy combining remote sensing data and the AquaCrop model. Hydrology. 8:39. doi: 10.3390/hydrology8010039
Ćosić, M., Stričević, R., Djurović, N., Moravčević, D., Pavlović, M., and Todorović, M. (2017). Predicting biomass and yield of sweet pepper grown with and without plastic film mulching under different water supply and weather conditions. Agric. Water Manag. 188, 91–100. doi: 10.1016/j.agwat.2017.04.006
Cui, Y., Jiang, S., Jin, J., Ning, S., and Feng, P. (2019). Quantitative assessment of soybean drought loss sensitivity at different growth stages based on S-shaped damage curve. Agric. Water Manag. 213, 821–832. doi: 10.1016/j.agwat.2018.11.020
Cui, Y., Lin, H., Xie, Y., and Liu, S. (2021). Application study of crop yield prediction based on AquaCrop model in black soil region of Northeast China. Acta Agron. Sin. 47, 159–168. doi: 10.3724/SP.J.1006.2021.03016
Cui, Y., Ning, S., Jin, J., Jiang, S., Zhou, Y., and Wu, C. (2020). Quantitative lasting effects of drought stress at a growth stage on soybean evapotranspiration and aboveground biomass. Water 13:18. doi: 10.3390/w13010018
Cui, Y., Tang, H., Jin, J., Zhou, Y., Jiang, S., and Chen, M. (2022). System structure—based drought disaster risk assessment using remote sensing and field experiment data. Remote Sens. 14:5700. doi: 10.3390/rs14225700
Cui, Y., Zhou, Y., Jin, J., Jiang, S., Wu, C., and Ning, S. (2023). Spatiotemporal characteristics and obstacle factors identification of agricultural drought disaster risk. A case study across Anhui Province, China. Agric. Water Manag. 289:108554. doi: 10.1016/j.agwat.2023.108554
Daniel, C. (2012). One-at-a-time plans. J. Am. Stat. Assoc. 68, 353–360. doi: 10.1080/01621459.1973.10482433
Dao, J., Xing, Y., Chen, C., Chen, M., and Wang, Z. (2023). Adaptability of rhizosphere bacterial communities of drought-resistant sugarcane varieties under different degrees of drought stress. Microbiol. Spectr. 11:e0118423. doi: 10.1128/spectrum.01184-23
Dercas, N., Dalezios, N. R., Stamatiadis, S., Evangelou, E., Glampedakis, A., Mantonanakis, G., et al. (2022). AquaCrop simulation of winter wheat under different N management practices. Hydrology. 9:56. doi: 10.3390/hydrology9040056
Ge, D., Gao, X., and Wei, X. (2024). Changes in spatiotemporal drought characteristics from 1961 to 2017 in northeastern maize-growing regions, China. Irrig. Sci. 42, 163–177. doi: 10.1007/s00271-023-00893-4
Ge, W., Han, J., Zhang, D., and Wang, F. (2021). Divergent impacts of droughts on vegetation phenology and productivity in the Yungui plateau. Southwest China. Ecol. Indic. 127:107743. doi: 10.1016/j.ecolind.2021.107743
Greaves, G. E., and Wang, Y. (2016). Assessment of FAO AquaCrop model for simulating maize growth and productivity under deficit irrigation in a tropical environment. Water 8:557. doi: 10.3390/w8120557
Guo, E., Zhang, J., Wang, Y., Si, H., and Zhang, F. (2016). Dynamic risk assessment of waterlogging disaster for maize based on CERES-maize model in Midwest of Jilin Province, China. Nat. Hazards 83, 1747–1761. doi: 10.1007/s11069-016-2391-0
Gupta, A., Rico-Medina, A., and Caño-Delgado, A. I. (2020). The physiology of plant responses to drought. Science 368, 266–269. doi: 10.1126/science.aaz7614
Gusain, S., Kumari, K., and Joshi, R. (2024). Physiological, hormonal and molecular dynamics of root system architectural response to drought stress signaling in crops. Rhizosphere. 31:100922. doi: 10.1016/j.rhisph.2024.100922
Hao, S., Guo, X., and Wang, W. (2010). Aftereffects of water stress on corn growth at different stages. Trans. Chin. Soc. Agric. Eng. 26, 71–75. doi: 10.3969/j.issn.1002-6819.2010.07.012
Hou, M., Li, Y., Biswas, A., Chen, X., Xie, L., Liu, D., et al. (2024). Concurrent drought threatens wheat and maize production and will widen crop yield gaps in the future. Agric. Syst. 220:104056. doi: 10.1016/j.agsy.2024.104056
Hsiao, T., Heng, L., Steduto, P., Rojas-Lara, B., Raes, D., and Fereres, E. (2009). AquaCrop—the FAO crop model to simulate yield response to water: III. Parameterization and testing for maize. Agron. J. 101, 448–459. doi: 10.2134/agronj2008.0218s
Hu, J., Zhao, X., Gu, L., Liu, P., Zhao, B., Zhang, J., et al. (2023). The effects of high temperature, drought, and their combined stresses on the photosynthesis and senescence of summer maize. Agric. Water Manag. 289:108525. doi: 10.1016/j.agwat.2023.108525
Hussain, A., Jadoon, K. Z., Rahman, K. U., Shang, S., Shahid, M., Ejaz, N., et al. (2023). Analyzing the impact of drought on agriculture: evidence from Pakistan using standardized precipitation evapotranspiration index. Nat. Hazards 115, 389–408. doi: 10.1007/s11069-022-05559-6
Irmak, S., Amiri, E., Bazkiaee, P. A., and Araji, H. A. (2024). Evaluation of CERES-maize model for simulating maize phenology, grain yield, soil-water, evapotranspiration, and water productivity under different nitrogen levels and rainfed, limited, and full irrigation conditions. Irrig. Sci. 42, 551–573. doi: 10.1007/s00271-023-00909-z
Jing, L., Weng, B., Yan, D., Zhang, S., Bi, W., and Yan, S. (2023). The persistent impact of drought stress on the resilience of summer maize. Front. Plant Sci. 14:1016993. doi: 10.3389/fpls.2023.1016993
Kim, K. H., and Lee, B. M. (2023). Effects of climate change and drought tolerance on maize growth. Plan. Theory 12:3548. doi: 10.3390/plants12203548
Kinoshita, T., Toh, S., and Torii, K. U. (2021). Chemical control of stomatal function and development. Curr. Opin. Plant Biol. 60:102010. doi: 10.1016/j.pbi.2021.102010
Lamin-Samu, A. T., Mohamed, F., Muhammad, A., and Lu, G. (2021). Morpho-physiological and transcriptome changes in tomato anthers of different developmental stages under drought stress. Cells 10:1809. doi: 10.3390/cells10071809
Li, B., Qin, L., Qi, H., Wang, J., Dang, Y., Lv, M., et al. (2024). Assessing the effects of drought on rainfed maize water footprints based on remote sensing approaches. J. Sci. Food Agric. 104, 1154–1165. doi: 10.1002/JSFA.13000
Li, F., Zhang, M., and Liu, Y. (2022). Quantitative research on drought loss sensitivity of summer maize based on AquaCrop model. Nat. Hazards 112, 1065–1084. doi: 10.1007/S11069-022-05218-W
Liu, L. (2015). The influence of drought on the growth and yield of maize. Agric. Sci. Technol. Equipment 11, 6–7. doi: 10.3969/j.issn.1674-1161.2015.11.003
Lu, Y., Wei, C., McCabe, M. F., and Sheffild, J. (2022). Multi-variable assimilation into a modified AquaCrop model for improved maize simulation without management or crop phenology information. Agric. Water Manag. 266:107576. doi: 10.1016/j.agwat.2022.107576
Mondol, M. A. H., Zhu, X., Dunkerley, D., and Henley, B. J. (2021). Observed meteorological drought trends in Bangladesh identified with the effective drought index (EDI). Agric. Water Manag. 255:107001. doi: 10.1016/j.agwat.2021.107001
Munnus, R., and Millar, A. H. (2023). Seven plant capacities to adapt to abiotic stress. J. Exp. Bot. 74, 4308–4323. doi: 10.1093/jxb/erad179
Ors, S., Ekinci, M., Yildirim, E., Sahin, U., Turan, M., and Dursun, A. (2021). Interactive effects of salinity and drought stress on photosynthetic characteristics and physiology of tomato (Lycopersicon esculentum L.) seedlings. S. Afr. J. Bot. 137, 335–339. doi: 10.1016/j.sajb.2020.10.031
Peng, J., Lu, L., Noor, M., Li, S., Ma, W., and Wang, J. (2023b). Mid-season lodging modulates photosynthesis, evapotranspiration, and dry matter accumulation and distribution simulated by the optimized model in maize. Front. Ecol. Evol. 11:1178609. doi: 10.3389/fevo.2023.1178609
Peng, Q., Shen, R., Li, X., Ye, T., Dong, J., Fu, Y., et al. (2023a). A twenty-year dataset of high-resolution maize distribution in China. Sci. Data. 10:658. doi: 10.1038/S41597-023-02573-6
Razzaghi, F., Zhou, Z., Andersen, M., and Plauborg, F. (2017). Simulation of potato yield in temperate condition by the AquaCrop model. Agric. Water Manag. 191, 113–123. doi: 10.1016/j.agwat.2017.06.008
Ru, C., Hu, X., Chen, D., and Wang, W. (2023). Droughts and thermo-priming enhance acclimation to later drought and heat stress in maize seedlings by improving leaf physiological activity. Agronomy 13:1124. doi: 10.3390/agronomy13041124
Salgotra, R. K., and Chauhan, B. S. (2023). Ecophysiological responses of rice (Oryza sativa L.) to drought and high temperature. Agronomy 13:1877. doi: 10.3390/agronomy13071877
Sato, H., Mizoi, J., Shinozaki, K., and Yamaguchi-Shinozaki, K. (2024). Complex plant responses to drought and heat stress under climate change. Plant J. 117, 1873–1892. doi: 10.1111/tpj.16612
Shafi, S., Shafi, I., Zaffar, A., Zaffar, S. M., Shikari, A. B., Ranjan, A., et al. (2023). The resilience of rice under water stress will be driven by better roots: evidence from root phenotyping, physiological, and yield experiments. Plant Stress 10:100211. doi: 10.1016/j.stress.2023.100211
Tang, D., Feng, Y., Gong, D., Hao, W., and Cui, N. (2018). Evaluation of artificial intelligence models for actual crop evapotranspiration modeling in mulched and non-mulched maize croplands. Comput. Electron. Agric. 152, 375–384. doi: 10.1016/j.compag.2018.07.029
Tojo Soler, C. M., Suleiman, A., Anothai, J., Flitcroft, I., and Hoogenboom, D. (2013). Scheduling irrigation with a dynamic crop growth model and determining the relation between simulated drought stress and yield for peanut. Irrig. Sci. 31, 889–901. doi: 10.1007/s00271-012-0366-9
Voloudakis, D., Karamanos, A., Economou, G., Kalivas, D., Vahamidis, P., Kotoulas, V., et al. (2015). Prediction of climate change impacts on cotton yields in Greece under eight climatic models using the AquaCrop crop simulation model and discriminant function analysis. Agric. Water Manag. 147, 116–128. doi: 10.1016/j.agwat.2014.07.028
Wang, C., Guo, E., Wang, Y., Jirigala, B., Kang, Y., and Zhang, Y. (2023). Spatiotemporal variations in drought and waterlogging and their effects on maize yields at different growth stages in Jilin Province, China. Nat. Hazards 118, 155–180. doi: 10.1007/s11069-023-05996-x
Wang, Y., Lv, J., Sun, H., Zuo, H., Gao, H., Qu, Y., et al. (2022). Dynamic agricultural drought risk assessment for maize using weather generator and APSIM crop models. Nat. Hazards 114, 3083–3100. doi: 10.1007/s11069-022-05506-5
Wei, Y., Jin, J., Cui, Y., Ning, S., Fei, Z., Wu, C., et al. (2021). Quantitative assessment of soybean drought risk in Bengbu city based on disaster loss risk curve and DSSAT. Int. J. Disaster Risk Reduc. 56:102126. doi: 10.1016/j.ijdrr.2021.102126
Wei, Y., Jin, J., Jiang, S., Ning, S., Cui, Y., and Zhou, Y. (2019). Simulated assessment of summer maize drought loss sensitivity in Huaibei plain, China. Agronomy 9:78. doi: 10.3390/agronomy9020078
Wu, Y., Leng, P., and Ren, C. (2024). Assessing net irrigation needs in maize–wheat rotation farmlands on the North China plain: implications for future climate scenarios. Agronomy 14:1144. doi: 10.3390/agronomy14061144
Xie, Z., Kong, J., Tang, M., Luo, Z., Li, D., Liu, R., et al. (2023). Modelling winter rapeseed (Brassica napus L.) growth and yield under different sowing dates and densities using AquaCrop model. Agronomy 13:367. doi: 10.3390/agronomy13020367
Yao, C., Zhang, S., and Yan, X. (2012). Effects of drought and re-watering on photosynthetic characteristics of maize leaf. Res. Soil Water Conserv. 19, 278–283.
Yuan, H., Jiang, S., Yang, J., and Liu, J. (2019). The response regularity of maize under drought stress based on physiological indexes. Water Saving Irrigation. 5, 5–10. doi: 10.3969/j.issn.1007-4929.2019.05.002
Zhang, C., Chao, J., Tang, M., Lin, W., Ding, D., and Feng, H. (2023). Improving maize growth and development simulation by integrating temperature compensatory effect under plastic film mulching into the AquaCrop model. Crop J. 11, 1559–1568. doi: 10.1016/j.cj.2023.05.008
Zhang, J., He, Y., Wang, J., Zhao, Y., and Wang, C. (2015). Impact simulation of drought at different growth stages on grain formation and yield of maize. Chin. J. Agrometeorol. 36, 43–49. doi: 10.3969/j.issn.1000-6362.2015.01.006
Zhang, X., Lei, L., Lai, J., Zhao, H., and Song, W. (2018). Effects of drought stress and water recovery on physiological responses and gene expression in maize seedlings. BMC Plant Biol. 18:68. doi: 10.1186/s12870-018-1281-x
Zhang, J., Okada, N., Tatano, H., and Hayakawa, S. (2004). Damage evaluation of agro-meteorological hazards in the maize-growing region of Songliao plain, China: case study of Lishu county of Jilin Province. Nat. Hazards 31, 209–232. doi: 10.1023/B:NHAZ.0000020263.98345.a0
Zhang, Q., Yao, Y., Li, Y., Huang, J., Ma, Z., Wang, Z., et al. (2020). Causes and changes of drought in China: research progress and prospects. J. Meteorol. Res. 34, 460–481. doi: 10.1007/s13351-020-9829-8
Zhou, R., Jin, J., Cui, Y., Ning, S., Bai, X., Zhang, L., et al. (2022). Agricultural drought vulnerability assessment and diagnosis based on entropy fuzzy pattern recognition and subtraction set pair potential. Alex. Eng. J. 61, 51–63. doi: 10.1016/j.aej.2021.04.090
Zhou, D., Wang, H., Wang, X., Wang, F., Zhang, J., and Ma, D. (2024). Evaluation of AquaCrop’s ability to simulate water stress based on 2-year case study of maize crop. Agronomy 14:354. doi: 10.3390/agronomy14020354
Zhu, X., Xu, K., Liu, Y., Guo, R., and Chen, L. (2021). Assessing the vulnerability and risk of maize to drought in China based on the AquaCrop model. Agric. Syst. 189:103040. doi: 10.1016/j.agsy.2020.103040
Zizinga, A., Mwanjalolo, J. G. M., Tietjen, B., Martins, M. A., and Bedadi, B. (2024). Maize yield under a changing climate in Uganda: long-term impacts for climate smart agriculture. Reg. Environ. Chang. 24:34. doi: 10.1007/s10113-024-02186-8
Keywords: responses to continuous drought stress, accumulative effect, adaptive effect, food security, sustainable agricultural production, summer maize, Huaibei Plain
Citation: Cui Y, Tang H, Zhou Y, Jin J and Jiang S (2024) Accumulative and adaptive responses of maize transpiration, biomass, and yield under continuous drought stress. Front. Sustain. Food Syst. 8:1444246. doi: 10.3389/fsufs.2024.1444246
Edited by:
Aliza Pradhan, National Institute of Abiotic Stress Management (ICAR), IndiaReviewed by:
Jiban Shrestha, Nepal Agricultural Research Council, NepalHuailin Zhou, Chinese Academy of Meteorological Sciences, China
Wuxia Bi, China Institute of Water Resources and Hydropower Research, China
Copyright © 2024 Cui, Tang, Zhou, Jin and Jiang. This is an open-access article distributed under the terms of the Creative Commons Attribution License (CC BY). The use, distribution or reproduction in other forums is permitted, provided the original author(s) and the copyright owner(s) are credited and that the original publication in this journal is cited, in accordance with accepted academic practice. No use, distribution or reproduction is permitted which does not comply with these terms.
*Correspondence: Juliang Jin, SklOSkw2NkBoZnV0LmVkdS5jbg==