- College of Economics and Management, South China Agricultural University, Guangzhou, China
Introduction: Specialty agricultural farmers have widely adopted digital technologies in all aspects of their specialty agricultural operations. However, the effect of digital technology application (DTA) on specialty agricultural farmers’ income has not been fully evaluated. Moreover, it remains unclear whether DTA enhances farmers’ income (FI) by improving their varied capabilities. To fill this gap, we analyzed the intrinsic relationship and mechanism between DTA, farmers’ capability (FC), and specialty agricultural farmers’ income.
Methods: Using field survey data from 635 litchi farmers in China, we employed OLS regression models and mediation effect models to empirically investigate DTA’s direct and indirect impacts on litchi farmers’ income. Additionally, we examined the group heterogeneity and regional heterogeneity.
Results and discussion: The empirical results show that DTA can promote specialty agricultural farmers’ income by enhancing their production capacity (PC) and transaction capacity (TC). Heterogeneity analysis showed that the empowering effect of DTA is especially significant for disadvantaged farmers, and farmers who are in specialty agricultural resources-rich regions.
Conclusion: This study contributes to the body of research on the empowerment of specialty agricultural farmers by examining the effect of DTA from a capability perspective. Consequently, to better play the empowering effect of DTA for specialty agricultural farmers, policymakers should account for regional disparities in promoting digital agriculture, and enhance heterogeneous farmers’ DTA capability.
1 Introduction
Promoting industrial revitalization to help farmers achieve sustainable and stable income growth is an essential path for China to consolidate the achievement of poverty alleviation and realize common prosperity. Specialty agriculture, as a vital element of industrial revitalization, makes a significant contribution to farmers’ income growth (Li and Gan, 2022). This paper defines specialty agriculture as agriculture distinguished by varietal, regional, and cultural characteristics, in addition to the foundational attributes of general agriculture. It typically encompasses unique product categories, exceptional varieties, and superior quality, and is closely connected to the regional environment (Wan, 2022). Compared with general agriculture, specialty agriculture can generate sales revenue that is three to five times higher (Garg et al., 2023). The prices of protected geographical indication (GI) products are 11.5% higher than those of non-protected (Duvaleix et al., 2021). China has been making great efforts to advance specialty agriculture development. In 2017, the Chinese Government’s Central Document No. 1 clearly emphasized the need to transform local specialties and small varieties into major industries to promote farmers’ income. The 20th Party Congress report also advocated developing rural specialty industries and expanding farmers’ income channels. This focus was emphasized in the Chinese Government’s Central Document No. 1 for three consecutive years from 2022 to 2024. Driven by policies, specialty agriculture fosters rural industrial revitalization, resulting in increased incomes for local farmers. For example, by 2023, China has developed 3,274 “one village, one product” model villages and towns.1 Farmers’ per capita disposable income in these model villages and towns is about 10% higher than the national average (see footnote 1). However, official data are often average which can obscure the significant disparities in individual incomes. Our field survey data from 635 litchi farmers shows that the gap between the highest and lowest average net income can reach up to 400 times. More significantly, the foundation of some specialty agriculture is not solid in some regions. The monitoring result for the first six batches of national “one village, one product” model villages and towns shows that about 5.88% of villages and towns failed to meet the required standards (see footnote 1), which not only failed to empower farmers but also potentially remove farmers’ original ability. Thus, focusing on the issue of empowering specialty agricultural farmers and increasing their incomes is of great practical significance.
This task arises within two crucial contexts. Firstly, digitization has become a vital component of China’s economy. Since the 1990s, when Internet technology kicked off China’s digitization, the country has witnessed rapid growth in digital technology development. According to the latest data from the China Office of the Central Cyberspace Affairs Commission, China’s digital economy reached 50.2 trillion yuan in 2022,2 making up 41.5% of its GDP. Digital technology, as a new element, is now deeply embedded in agriculture. For example, the Internet, which is widely accessible to farmers, had a penetration rate of 66.5% in China’s rural areas in 2023.3 The integration of digital technologies with agriculture has revolutionized farmers’ production and business models (Abiri et al., 2023), creating new opportunities for increasing their incomes. We plotted the relationship between internet penetration rate in rural China (data from CNNIC) and per capita disposable income of rural residents in China (data from CNBS) from 2013 to 2023 (Figure 1). The results indicate a positive correlation between these two variables. In other words, as internet penetration rate rises, so does per capita disposable income of rural residents. This implies that the DTA of farmers may positively impact their income. Thus, it is important to provide more empirical evidence on the relationship between DTA and FI to address the challenge of maintaining stable income growth for farmers.
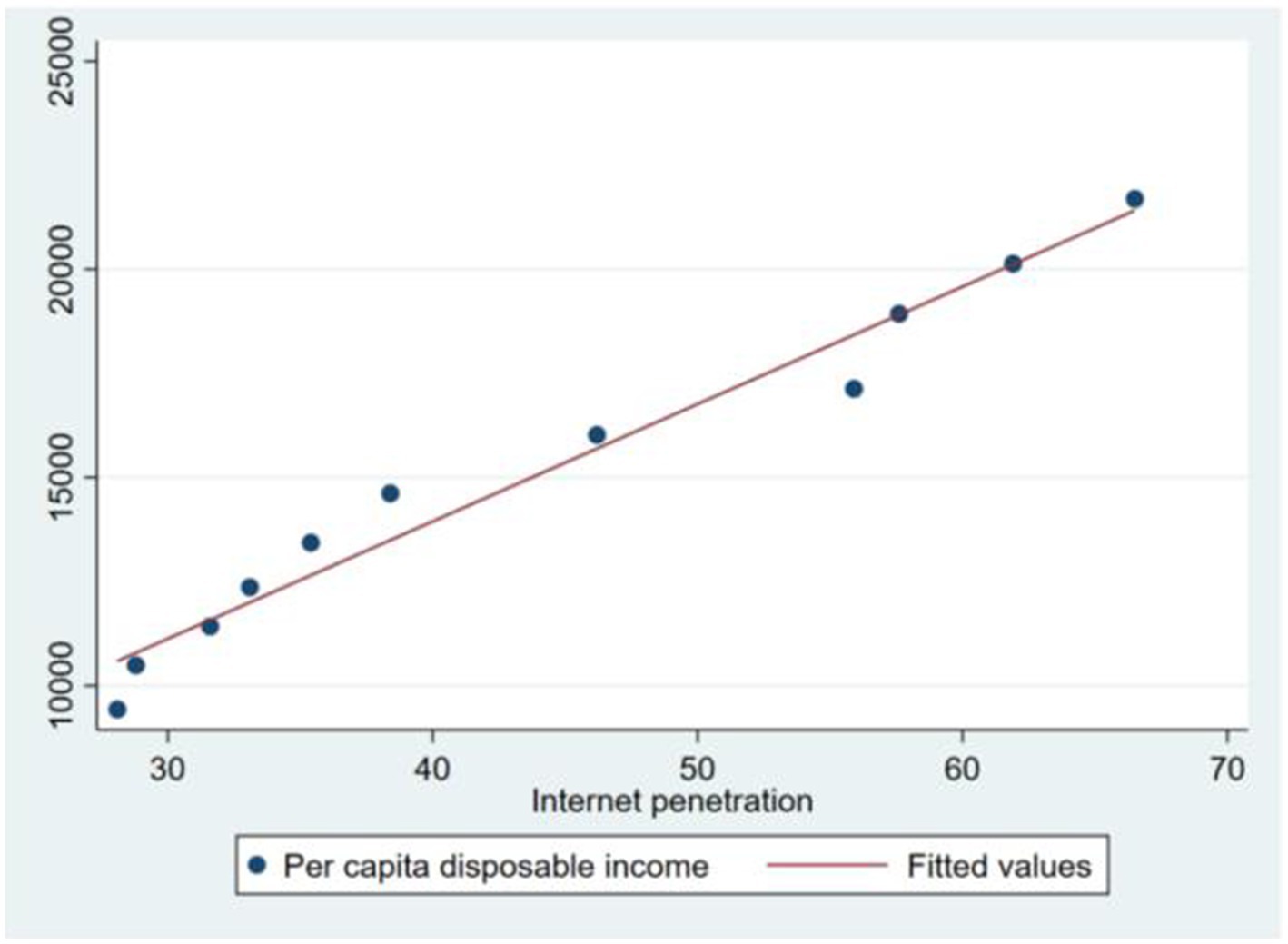
Figure 1. Internet penetration rate in rural China and per capita disposable income of rural residents in China from 2013 to 2023.
The second context is, specialty agricultural farmers are in dire need of digital technology empowerment. In reality, Many of China’s farmers are located in traditional agricultural areas, often remote and inaccessible but possess unique natural and cultural resources (Lin et al., 2023), suitable for developing specialty agriculture. Compared to general agriculture, specialty agricultural farmers face unique production risks, such as high perishability and high climate dependence (Flores et al., 2019), and are also vulnerable to extreme fluctuations in temperature and humidity (Zhao and Yue, 2020). Consumers expect high levels of freshness and flavor from specialty produce (Ruiz-Altisent et al., 2010), so specialty produce needs to be delivered to market immediately after harvest. However, unlike grain crops, specialty crops generally do not receive government protection, requiring farmers to bear their risks, and cope with high production and trading uncertainties, and it is challenging for specialty agricultural farmers to enter larger markets independently. Therefore, there is a growing demand from specialty agricultural farmers for digital technologies that can link small farmers to larger markets. To maximize the role of digital technology in empowering specialty agricultural farmers, the Chinese government has implemented several substantial decisions and deployments. In 2019, the government issued the Outline of the Strategy for Digital Countryside Development, explicitly proposing the “promotion of deep integration of the internet and specialty agriculture.” In 2022, the Central Office of the Internet, along with ten other departments, issued the Action Plan for the Development of the Digital Countryside (2022–2025), emphasizing the “continuous implementation of ‘digital business for agricultural development’… to promote mobile payment projects in rural areas, specialty industries….” Therefore, examining how DTA can empower specialty agricultural farmers to increase their incomes is crucial for designing better policy tools to promote common prosperity.
Recently, the issue of how DTA empowers farmers has gained considerable attention. There are two main strands of literature relevant to this paper. First, it relates to the ongoing debate concerning the impact of DTA on farmers’ performance. Before assessing these impacts, it is essential to clarify the definitions and categories of DTA. Existing literature varies in these respects due to differing research contexts. Many studies categorize and define DTA based on technical attributes (Kvam et al., 2022), constituent elements (Ancillai et al., 2023), and usage intentions (Schnebelin, 2022). On this basis, researchers have examined how DTA influences FC and FI. They believe that DTA works by influencing farmers’ market sales (Aker and Ksoll, 2016), intra-household resource allocation (Diiro et al., 2021), production factor allocation (Carrer et al., 2015), green production (Wang et al., 2024), technology adoption (Zheng et al., 2022) and other production behaviors, thereby enhancing FC. However, regarding the impact of DTA on FI, there are different research conclusions. Some scholars believe that DTA can increase FI (Zhou et al., 2020) and significantly benefit low-income groups (Zhang, 2022). Conversely, DTA is considered a “double-edged sword” that might accelerate group polarization (Scheerder et al., 2017). This is mainly because effectively using digital technology requires certain competencies, such as digital skills and literacy (Kabbiri et al., 2018). Some farmers lack these competencies, making it difficult for them to benefit from digital technology (Marshall et al., 2022). In addition, this paper is relevant to literature that investigates the mechanisms of farmer empowerment. The existing literature evaluates the influence of external factors, such as agricultural extension services (Yitayew et al., 2023), agricultural policy subsidies (Biagini et al., 2023), agricultural land titling (Séogo and Zahonogo, 2023), collective action (Lin et al., 2022), and farmer field schools (Luther et al., 2018) on empowering farmers. Some scholars have also looked at intra-farmer factors, such as new technology adoption (Abate et al., 2018), farmer risk management (Lien et al., 2022), precision agriculture adoption (Carrer et al., 2022), and crop diversification (Hoang et al., 2021) on farmer empowerment.
Although the existing literature has delved deeply into the DTA and farmer empowerment, three critical aspects remain significantly under-explored. First, existing evidence regarding the impact of DTA is mainly concentrated within general agricultural farmers (e.g., Kai et al., 2023; Ma et al., 2023; Zheng and Ma, 2023), leaving the influence of DTA on specialty agricultural farmers relatively unclear. While specialty agriculture possesses attributes common to general agriculture, it also exhibits distinct characteristics. Consequently, the conclusions derived from research on general agricultural farmers may not be directly applicable to specialty agricultural farmers. Second, much of the extant empirical literature concerning DTA has primarily focused on the overall farmers’ income effects (e.g., Zhang H. et al., 2023; Yi et al., 2023), with limited attention to substantiating the underlying mechanisms. Lastly, assessing DTA behavior on a micro level is particularly challenging, and there is a lack of a widely accepted system for measuring DTA. Current literature typically employs two main methods to illustrate DTA. Due to a shortage of micro-level field surveys about farmers’ DTA, most empirical research depends on secondary databases, which often have time delays and accuracy issues, leading to scholars using proxy variables like “internet use” to indirectly assess DTA (Wang et al., 2024). The other method focuses on specific forms of DTA, such as digital financial inclusion and digital e-commerce (Guo et al., 2023), without considering the diverse scenarios of DTA throughout the agro-industrial chain.
Referring to these research gaps, this study aims to contribute to the existing body of literature by providing farmers’ micro-level evidence for the impact of DTA on FI and delving into the potential mechanisms. We use survey data from lychee growers in China to estimate the impact of DTA on FI. Our findings indicate an increase in FI as a consequence of DTA, particularly among disadvantaged farmers and farmers in areas with rich specialty agricultural resources. The results of the mechanism analysis suggest that this positive effect can be primarily attributed to the production-empowering and transaction-empowering mechanisms of DTA, in which DTA enhances farmers’ PC and TC, thereby contributing to increased FI.
The contributions of this study to the existing literature are three-fold. First, we present new evidence on farmers’ DTA, expanding on the causal impact of DTA on farmers’ economic performance. The measurement of DTA in the existing literature may not adequately and accurately reflect the actual status of DTA among farmers. Using rich household-level survey data, we measure farmers’ DTA throughout the agricultural chain of pre-production, production, and post-production behaviors that are often challenging to observe at the macro level. This approach provides a more comprehensive picture of farmers’ DTA and allows us to explore potential channels behind the observed effects.
Second, by focusing on the specialty agriculture context, we add new empirical facts empowered by DTA. The production process of grain crops is often easily outsourced to socialized agricultural service organizations, which provide equal digital technology for farmers, resulting in narrowing the gap driven by farmers’ own DTA behaviors. In contrast, specialty crops that require intensive cultivation tend to have a lower degree of socialized agricultural service development. The performance differences stemming from farmers’ own DTA behaviors are more pronounced in specialty crops. Furthermore, China’s grain crops are backed by guaranteed price purchases, exposing grain farmers to limited market risks and lower requirements for transaction capability. Conversely, most specialty crops offer higher economic benefits than grain crops, with relatively less government subsidization, but face higher production and transaction risks. Thus, our findings provide valuable insights into the relatively under-explored empowering role of DTA among specialty agricultural farmers.
Last, we assess the income effect of DTA in farmers’ micro-level from a capability perspective, which provides new insights into the analysis of digital technology empowerment. Previous studies have recognized several effects of DTA on FI, including the social network effect (Kai et al., 2023), the technology adoption effect (Zheng et al., 2022), and the transaction cost effect (Yao et al., 2022). However, they do not adequately reveal the underlying cause of different performances among farmers with similar DTA behavior. Given that participation in specialty agriculture presents multiple risks (Neill and Morgan, 2021), requires a certain capacity of farmers, and capability and income performance display a significant correlation (Li et al., 2022), The variations in FC may be a key reason for the discrepancies among specialty agricultural FI. Nevertheless, existing studies have neglected the intrinsic impact of FC on FI. Although some research has attended to the capability issue, the difficulty of measuring capacity has led scholars to measure it relying on proxy variables like production and operational efficiency (Mauki et al., 2023), which do not adequately capture the diversity and complexity of FC. To address this gap, we explore the internal logic of DTA empowerment of specialty agricultural farmers from a capability perspective, unveiling the embedded mechanisms of production empowerment and transaction empowerment, and fostering the FC indicator system applicable to the specialty agricultural operation context.
2 Theoretical analysis and research hypotheses
This study draws on the resource-based theory (RBT) and empowerment theory to explore the mechanism of digital technology empowering farmers in specialty agriculture. The development of RBT can be summarized into three primary stages: “traditional RBT—dynamic capability theory—resource orchestration theory (Penrose, 1959; Wernerfelt, 1984; Teece et al., 1997; Sirmon et al., 2011). The traditional RBT suggests that firms possessing VRIN resources can gain a competitive advantage, highlighting the impact of resources on organizational performance (Penrose, 1959; Wernerfelt, 1984). However, scholars such as Eisenhardt and Martin (2000) argue that in a dynamic and rapidly changing market environment, the competitive advantage derived from such resources may not be sustainable. The dynamic capability view, represented by Teece et al. (1997), suggests that how a firm utilizes its resources is as critical as the nature of the resources themselves and that even without VRIN resources, firms can achieve performance through resource utilization, coordination, and other capabilities. This remedies the limitation of the traditional RBT, which focuses on the impact of resource stock on competitive advantage from a static perspective. Later, scholars such as Sirmon et al. (2011) put forward the resource orchestration theory by integrating the concepts of “resource management” and “asset orchestration” into the dynamic capability framework. This theory aims to open the “black box” of the process of resource influence on performance. It believes that the effective combination of resources, capabilities, and managerial behavior is an important way to enhance the creativity of enterprises. The theoretical logic of “resource-capability-performance” in these three stages provides a theoretical basis for this study to explore the mechanisms by which DTA empowers specialty agricultural farmers.
In the logical chain of “resource-capability-performance,” the empowerment theory explores the specific mechanism of resource empowerment based on a process perspective, which can explain the process mechanism of transforming resources into performance. Originating in psychology, empowerment theory has expanded to become a fundamental framework in sociology and organizational management. Definitions of empowerment abound (Rappaport, 1987; Thomas and Velthouse, 1990; Perkins and Zimmerman, 1995; Peterson et al., 2005; Llorente-Alonso et al., 2024). A common definition is that the empowering subject gives the empowered person some kind of power or ability (Thomas and Velthouse, 1990) to gain greater control or self-efficacy (Peterson et al., 2005; Santos et al., 2019). Consequently, the core focus of empowerment research is on identifying capabilities (Perkins and Zimmerman, 1995). In the digital economy era, digital technology has profoundly impacted human production and daily life. It has also become a new path and tool for empowerment by its advantages of high efficiency, low cost, and ability to cross the limitations of time and space. The symbiotic fusion of digital technology with empowerment theory has given rise to the new concept of “digital technology empowerment,” which has become a prominent topic in the academic community. Essentially, digital technology empowerment extends and enriches the empowerment theory’s core connotation, and its core is still empowerment. Therefore, digital technology empowerment emphasizes that the empowered subject obtains new abilities through DTA (Mäkinen, 2006).
The nature of DTA empowerment differs based on the context of its application and the target audience. On the individual level, various forms of empowerment exist, such as psychological empowerment, cultural empowerment, production empowerment (Xu Z. et al., 2023), and transaction empowerment (Zheng and Wu, 2024). Given that cultural and psychological empowerment typically rely on specific behaviors, and considering that farmers’ agricultural business behaviors involve both production decisions and transaction interactions (Zhu and Luo, 2016), the primary mechanisms of DTA empowering farmers to increase their incomes are production empowerment and transaction empowerment. Production empowerment generally enhances farmers’ capability to work with “objects,” that is PC, while transaction empowerment improves their abilities to work with “people,” that is TC. These specific mechanisms are depicted in Figure 2.
On the one hand, DTA can substantially enhance agricultural production practices (Karanasios and Slavova, 2019; Schnebelin, 2022). First, DTA assists specialty agricultural farmers in allocating production factors. Specialty crops tend to produce higher value outputs on smaller land areas compared to other crops (Astill et al., 2020), making land issues less significant. While labor issue is the primary challenge (Rihn et al., 2023). By DTA, farmers can address labor shortages and improve labor quality. For instance, automated and intelligent digital technologies can replace simple and repetitive labor, which can transform traditional production methods that rely on physical labor, and free up the labor force. Through online learning platforms, farmers can access open educational resources more affordably and rapidly, acquire advanced production technologies, and engage in modernized agricultural production and management. Second, DTA aids specialty agricultural farmers in managing the production process. In the production process, satellite remote sensing, ground sensing, and other Internet of Things technology enable farmers to monitor specialty crop growth and assess the production environment data in real-time (Ruiz-Altisent et al., 2010). In the manufacturing process, automated mechanical digital technology allows farmers to perform standardized processes such as refrigeration, packaging, and transport, along with timely warnings of safety indicators (Zhong et al., 2023). In the distribution process, using a traceability system, farmers can monitor product flow in real-time, ensuring that specialty agricultural products maintain high quality (Tao and Chao, 2024).
Finally, DTA assists specialty agricultural farmers in mitigating production risks. Specialty agricultural operations encounter various production risks. DTA helps farmers effectively address these risks. Implementing smart weather, pest, and disease monitoring systems allows farmers to scientifically predict the adverse effects of extreme weather (Zhao and Yue, 2020) and timely adopt preventive measures, helping farmers make the right decisions in production and management. Furthermore, the use of digital technologies such as variable-rate applicators enables farmers to adjust the frequency and timing of pesticide and chemical fertilizer applications based on growing conditions, thus minimizing agricultural product contamination issues (Khanna, 2021). Additionally, adopting digital technologies like big data intelligent temperature control systems and cold chain technology offers precise temperature control and preservation treatment for specialty agricultural products, thereby preventing quality deterioration issues such as loss of freshness and nutrient content (Flores et al., 2019).
On the other hand, DTA can assist farmers in entering differentiated markets (Hidalgo et al., 2023) by expanding social capital and achieving economies of scale, thereby enhancing farmers’ transaction capacity and boosting their income. Firstly, DTA aids farmers in expanding their social networks, establishing cooperative relationships with other trading entities, and increasing their access to cooperating organizations. Farmers use digital tools such as WeChat and the Internet to facilitate long-distance, real-time exchanges, and communications, fostering trust and cooperation with other trading partners (Ali-Hassan et al., 2015). By leveraging sensor-embedded digital technologies, farmers can accurately record and report the production process of specialty agricultural products, and transmit and store information, which helps cooperative entities capture real-time quality data about the products, enhancing their trust in the farmers (Qureshi et al., 2021). This cultivates stable contractual relationships and a community of interest among farmers and trading entities. To achieve a win-win situation, other trading entities might offer unified purchasing of production materials, processing, and centralized warehousing for farmers (Gramzow et al., 2018). This can significantly reduce the search and negotiation costs for farmers in both factor and product markets, enabling them to cross market thresholds, enhance their transaction capacity, and secure higher-value distribution. Secondly, DTA has encouraged farmers to scale up their operations, leveraging economies of scale to enhance their transaction capacity and promote income growth. When transaction costs are fixed, increased operational scale lowers the transaction cost per unit of specialty agricultural products, driving farmers to expand their cultivation through continuous planting and land transfer. Digital technology facilitates large-scale operations by allowing farmers to easily access digital financial services for capital loans, easing the credit constraints associated with land transfer (Smidt and Jokonya, 2022). Furthermore, farmers use digital technologies such as Internet platforms to obtain land transfer information timely (Wang et al., 2023), aiding them to make land transfer decisions. As the scale of their operations expands, farmers increasingly tend to invest in digital technologies that enhance production efficiency (Tamirat et al., 2018), enabling them to consistently provide high-quality, high-priced specialty agricultural products in the market, which bolsters their market power and increases sales prices.
Based on the discussion of theory and literature, this study draws on the resource-based theory and empowerment theory, follows the “resource-capability-performance” theoretical framework, and establishes the theoretical analysis framework of “DTA-FC-FI” (shown in Figure 2). Operating within this research framework, we seek to investigate the production empowerment and transaction empowerment effects on farmers stemming from the DTA throughout the entire industrial chain, to realize the enhancement of PC and TC, and ultimately affect FI. Thus, the following hypothesis is formulated:
H1: The DTA has a positive impact on FI.
H2: Production empowerment and transaction empowerment are two mechanisms through which DTA empowers specialty agricultural farmers to raise their income.
3 Methodology
3.1 Data source
China is a country with a vast expanse, and each place has unique resource conditions, which gave birth to a variety of specialty agricultural products. Among the many specialty agricultural products, this paper focuses on the litchi industry, due to litchi’s strong dependence on geographic and climatic conditions. China’s litchi has a unique planting history and cultural heritage, with over 2,000 years of cultivation, making it one of the nation’s most culturally specialty agricultural products. As the leading global litchi producer, China accounted for about 65% of the world’s litchi planting area in 2022 (Qi et al., 2023). However, uneven resource distribution has resulted in varied development levels of the litchi industry across regions, and in some areas, litchis may not develop into a specialty agriculture with comparative advantages. GI agricultural products are defined by specific qualities, reputations, or other attributes linked to their origin (Albayram et al., 2014). These products typically possess unique flavors, textures, or nutritional values rooted in their specific geographical areas. GI agricultural products reflect a region’s natural resources, cultural history, and other unique traits, and are a symbol of high-quality, distinctive agricultural products (Albayram et al., 2014). Therefore, this paper focuses on litchi growers within GI agricultural production areas as the primary research subjects. On this basis, this research involves randomly selecting litchi growers in the GI agricultural production areas of Guangdong Province of China for the survey. The reasons are multifaceted. Firstly, Guangdong Province is renowned for its development of specialty agriculture, with 194 designated “Guangdong” brand demonstration bases (Wu and Zhao, 2023). Secondly, the litchi industry is a key specialty industry in Guangdong. In 2023, the litchi planting area in the province expanded to 4.1 million mu,1 producing a total yield of 1.60 million tons and supporting over 1.8 million litchi farmers (Xu and Wang, 2023). Moreover, in 2023, Guangdong Province’s fresh litchi exports ranked first in the country and constituted 62.90% of the national market share.2 Thirdly, the litchi industry in Guangdong Province has a high degree of DTA. As litchi is a perennial specialty crop, lending itself well to digital technologies such as drones and sensors. Guangdong Province has thus far developed several digital platforms, including the China (Guangdong) Litchi Industry Big Data Center and the Conghua Litchi Open Big Data Platform. Consequently, the subject of our research is both exemplary and representative.
To ensure the representativeness and accuracy of our sample, we employed a combination of stratified random sampling. Initially, we selected two counties or districts with GI agricultural products from each of the Pearl River Delta, Eastern Guangdong, and Western Guangdong, considering regional differences and economic development levels. Subsequently, we randomly selected 1–2 towns from each sample county or district. Following this, four sample villages were randomly chosen from each sample town, resulting in a total of 44 sample villages. Finally, trained master’s and doctoral students, serving as investigators and assisted by local agricultural management officials and village cadres, randomly selected 12–20 litchi farmers in each sample village for one-on-one questionnaire surveys, leading to a total of 655 sample households. Additionally, to ensure the validity of the farmer questionnaires, we also designed a village-level questionnaire for village cadres and selected one village or township cadre from each sample village to complete it.
The questionnaire aimed to collect essential data for the study, including detailed information on individual farmer characteristics, family traits, specialty agricultural businesses, and DTA. This survey mainly targeted the head of the household or principal family member to ensure a thorough understanding of the farmers’ family businesses. Furthermore, village-level questionnaires covered information on village characteristics, agricultural resources, and village-specific agricultural business. After the questionnaire interviews, we carefully collated and checked the collected raw data, obtaining 655 original questionnaires. After excluding those farmer questionnaires with missing or anomalous data, we obtained 635 valid questionnaires, yielding a validity rate of 96.95%. The village questionnaires achieved a validity rate of 100%.
3.2 Variable description
3.2.1 Dependent variable
Drawing on Cha et al. (2024), the dependent variable in this study is the income of litchi farmers, represented by the household profits from lychee cultivation alone, calculated as total revenue minus total costs, with a logarithmic transformation applied for empirical analysis.
3.2.2 Independent variable
The independent variable in this study is the extent of DTA by litchi farmers. DTA is embedded in every facet of specialty agriculture, spanning the entire industry chain. We synthesized the research of Peng et al. (2022) and Abdulai et al. (2023) to capture farmers’ DTA across seven dimensions: digital procurement, digital production, digital processing, digital sales, digital information access, digital training participation, and digital financial use. Digital procurement is assessed by asking farmers if they use digital technologies such as WeChat and e-commerce platforms for agricultural procurement. Digital production is assessed by asking farmers if they use digital technologies such as automatic weeders, sprayers, systems for water and fertilizer integration, remote connections, intelligent monitoring, etc., in their processes for weeding, dosing, irrigation, pest control, monitoring, etc. Digital processing is determined by asking farmers if they implement digital technologies such as automatic sorting machines, smart cold storage units, automatic drying machines, etc., in their sorting, storage, drying, or other processing procedures. Digital marketing is measured by asking farmers if they employ digital technologies such as the Internet, WeChat, live sales platforms, product traceability codes, and smart cold chain logistics in their sales, product traceability, cold chain transportation, and other sales-related processes. Access to digital information is evaluated by asking farmers if they use digital technologies like WeChat, Tik Tok, the Internet, cell phone apps, etc., to access policy information, technical data, agricultural information, weather updates, market data, loan information, and insurance details related to their specialty agricultural business. Participation in digital training is assessed by asking farmers if they utilize digital technologies such as WeChat, Tik Tok, the Internet, cell phone apps, etc., to engage in training on agricultural policies and regulations, production skills, processing techniques, e-commerce operations, live broadcasting of goods, and the use of network information technology, as well as rural civilization or farming culture related to their specialty agricultural business. Digital financial use is measured by asking farmers if they use digital technologies such as WeChat, Alipay, mobile banking, online banking, etc., to perform payment transactions, purchase insurance for specialty agricultural products, and access Internet credit. All of the above questions are assigned a value of 1 if farmers answer “do,” otherwise it is 0.
Field research data reveal that 379 farm households utilize digital technology in the procurement process, representing 59.69%; 540 farm households employ digital technology in the production process, constituting 85.04%; 63 farm households apply digital technology in the processing process, accounting for 9.92%; 343 farm households leverage digital technology in the sales process, making up 54.02%; 557 farm households use digital technology for information access, corresponding to 87.72%; 543 farm households engage digital technology in training, equating to 85.51%; and 529 farm households incorporate digital technology in the financial process, comprising 83.31%. Focusing merely on whether farmers use digital technology does not adequately address the real issue. Hence, we employed entropy weighting to quantify the extent of DTA by farmers across the seven identified categories.
3.2.3 Mechanism variable
To delve deeper into the pathways through which DTA affects litchi farmers’ income, we conducted a mechanism analysis. In line with our research hypothesis, we selected farmers’ capacity as the mechanism variable, encompassing PC and TC. PC was broken down into three sub-variables: production factor allocation capacity, production process management capacity, and production risk resistance capacity. Drawing from the work of Wassie et al. (2023), Liang and Jiao (2022), and Li et al. (2020), and considering the practical context of specialty agriculture, we developed a 12-item five-level Likert scale to measure these factors. The production factor allocation capacity was gauged by three items related to resource allocation and utilization; the production process management capacity was measured by five items associated with variety selection, field management, and post-harvest processing; and the production risk resistance capacity was evaluated through four items designed to measure resilience to specific production risks. TC was assessed in two areas: factor market transaction capacity and product market transaction capacity. Based on the research by Wassie et al. (2023), Dias et al. (2021), and Ansah et al. (2020), we designed a 9-item five-point Likert scale, focusing on relational governance involving farmers’ access to factor market resources, selling products in the product market, and the relational governance in product sales. We used SPSS 22.0 software to conduct exploratory factor analysis on these indexes and constructs PC and TC indexes,1 which are used as measures of PC and TC, respectively.
3.2.4 Control variable
Referring to the existing literature (Zhou and Shen, 2022; Cheng et al., 2016), We introduced the following four types of control variables to avoid model estimation bias caused by missing variables. Firstly, individual characteristic variables, including age, gender, years of education, health status, years of experience in specialty agriculture, and the frequency of training participation by the head of household. Secondly, household characteristic variables, cooperative membership, land area dedicated to specialty agriculture, labor force involved in specialty agriculture, total inputs in specialty agriculture, and social networks. Village characteristics variables, including topography, transportation infrastructure, village income, clan relations, and the presence of private courier services and 5G networks. Moreover, in the context of specialty agriculture, we also consider variables related to regional specialty agricultural resources, measured by the unique natural and sociocultural resources available in the village. Natural resources are assessed based on whether the village possesses national geographic indications, or possesses the “one village, one product” or “one town” model. Sociocultural resources are evaluated based on whether the village is recognized as a Chinese traditional village, an ancient village, a location of significant agricultural cultural heritage in China, or a site of national intangible cultural heritage in the county. The final value indicating the level of specialty agricultural resources is calculated using entropy weighting of these six indices. Variable definitions and descriptive statistics are summarized in Table 1.
3.3 Econometric model
3.3.1 Baseline model
To explore the relationship between DTA and FI, we referenced Cha et al. (2024), using the OLS to estimate the following model:
In Equation 1, LnIncomei is the dependent variable, which represents the logarithm of the income level of farmers involved in specialty agricultural operations. Digitali is the core independent variable, indicating the level of DTA by these farmers. Controli is the control variable. ∂0 is a constant term; ∂1 and ∂2 are the regression coefficients of each variable respectively; εi is a random disturbance term.
3.3.2 Mechanism analysis model
The primary aim of this paper is to examine the impact of DTA on FI and to explore the underlying mechanisms of PC and TC. Consequently, we need to establish a mediation model in which DTA influences farmers’ PC and TC, which in turn affects FI. This study referenced Cha et al. (2024) to develop the following model.
In Equation 2, Capacityi represents a mechanism variable encompassing PC and TC. The meanings of other variables are the same as those of Equation 1. Given that the dependent variables in Equation 2 are continuous, the OLS model was chosen for empirical analysis.
4 Results and discussion
4.1 Baseline results
Table 2 shows the baseline regression results of the impact of DTA on litchi farmers’ income after progressively introducing control variables. The results from Models (1) and (2) indicate a significant positive impact of DTA on litchi farmers’ income at the 1% significance level. This suggests that DTA substantially increases litchi farmers’ income, thereby confirming research hypothesis 1. Moreover, the regression results show that DTA can increase the per FI by 0.603 in general. Our results extend the current literature on the effect of DTA on FI. There is a growing body of literature focusing on the effects of DTA on FI, but the findings are frequently inconsistent. Some researchers highlight the positive impacts of DTA on FI (Karanasios and Slavova, 2019; Schnebelin, 2022; Zheng and Ma, 2023), whereas others have expressed concerns (Wyche and Steinfield, 2016; Scheerder et al., 2017). We suggest that the primary reason for such discrepancies is that researchers typically focus on specific DTA behaviors, inevitably resulting in varied outcomes. This paper provides a systematic analysis of the effects of farmers’ DTA across the entire industry chain of specialty agriculture on their incomes and concludes that the positive effect of DTA on FI. This is a response to the previous divergent findings. This finding suggests that DTA covering the entire process of specialty agricultural production and operations, can improve the PC and TC of specialty agricultural farmers, which in turn leads to income growth.
4.2 Endogeneity discussion
There might be endogeneity concerns between DTA and litchi farmers’ income. Firstly, despite our efforts to control for factors influencing FI, it is challenging to account for all variables, resulting in potential endogeneity problems due to omitted variables. Additionally, the model may face reverse causality, where farmers with higher incomes might have greater access to DTA, thereby increasing their DTA levels, which could skew the findings. To mitigate endogeneity issues, the instrumental variable (IV) approach is a commonly used method. Based on relevant research (Qiu et al., 2024), this study used the average DTA level of other farmers in the village except the respondent as an instrumental variable. Due to the herd effect, the DTA of other farmers in the village will affect whether the respondent uses DTA or not. However, they will not directly affect the respondent’s income. Therefore, the average DTA level of other farmers in the village satisfies the correlation and exclusion constraints required by the instrumental variables. The first-stage regression results, presented in Table 3, reveal that the regression coefficients for the instrumental variables are significantly positive at the 1% level, indicating a substantial positive correlation between the instrumental variable and the respondent’s DTA. Additionally, the F-statistic for the first-stage regression is 20.28, which exceeds the conventional threshold of 10, suggesting no weak instrument issue. The first-stage regression results reveal that the higher the average DTA level of other farmers, the higher the digital technology use by the respondent. Specifically, a 1% increase in the average DTA level of other farmers is associated with 0.308% increase in the respondent’s DTA. The second-stage regression results indicate that farmers’ DTA has a significantly positive effect on the specialty agricultural farmers’ income. After controlling for other factors, the DTA can increase the per FI by 5.3071 in general. These findings suggest that controlling for potential endogeneity using instrumental variables does not negate the significant impact of DTA on the specialty agricultural farmers’ income. This underscores the robustness of the previous regression results and confirms research hypothesis H1.
4.3 Robustness test
To ensure the reliability of the above analysis results, we conducted robustness tests by changing the measurement method of the core dependent variable. Specifically, based on the research of Liu (2022), we replaced the dependent variable with “average return per mu of specialty agricultural farmers” for a new regression analysis. As indicated in column 2 of Table 4, the coefficient of the core dependent variable remains significantly positive.
Secondly, we conducted a robustness check by altering the independent variable. Following the study of Mao et al. (2023), we employed the weighting method to measure farmers’ DTA. The result in column 3 of Table 4 shows that the coefficient of DTA is significantly positive, reinforcing the robustness of the findings.
Thirdly, we excluded part of the sample for regression. To further eliminate the influence of age, We draw on the research of Xu and Wang (2022) and excluded farm households over 65 years old from the sample. The results in column 4 of Table 4 affirm that even with this exclusion, DTA still significantly enhances specialty agricultural farmers’ income.
Furthermore, to address potential outliers in continuous variables, we followed Xu J. et al. (2023) and shrank the values of continuous variables in the sample by 1%. As observed in column 5 of Table 4, the coefficient of DTA on specialty agricultural farmers’ income is significant at the 1% level, further affirming that the benchmark regression results are robust and valid.
4.4 Influence mechanism analysis
The theoretical analysis provided in this study reveals that DTA can boost specialty agricultural farmers’ income through production empowerment and transaction empowerment. Therefore, we identified PC and TC as mechanism variables to further verify this research hypothesis. Drawing on the work of Cha et al. (2024), the focus of this section is on the causal connections between DTA and these mechanism variables (PC and TC). The results in Table 5 show that the regression coefficients of DTA on PC or TC are both significantly positive at the 1% level, signifying that DTA can indeed enhance PC and TC. Therefore, hypothesis H2 is confirmed. By comparing the regression coefficients, we observe that the mediating effect of TC is stronger than that of PC. This result is in line with the operational demands of Chinese farmers. As stated by Chen (2019), most Chinese farmers have adapted well to agricultural production conditions, demonstrating strong resilience and vitality. Specialty agricultural farmers are no exception. Relying on traditional production experience, these farmers can maintain the basic agricultural product production. However, a significant constraint for specialty agricultural farmers is their lack of trading capacity. One issue is the farmers’ limited ability to connect to the market (Ma et al., 2024). In reality, poor sales of specialty agricultural products are occasionally observed, and even some GI agricultural products encounter sales difficulties (Wan, 2022). Furthermore, many specialty agricultural farmers lack adequate negotiation skills, making it challenging for them to engage in high-value industrial chain activities that offer higher added value (Wang et al., 2021). Consequently, the need for TC enhancement among specialty agricultural farmers is more urgent than that for PC enhancement. Farmers are thus more willing to adopt digital technologies that can bolster their TC. This, to some extent, results in a more significant marginal effect of DTA on TC compared to PC.
4.5 Heterogeneity analysis
4.5.1 Heterogeneity analysis of farm households
Although the previous analysis has demonstrated that DTA can help litchi farmers increase their income, this conclusion only applies to the average effect on the overall litchi farmer population. Due to the different characteristics of farmers, it is necessary to further explore the heterogeneity of the impact of DTA on increasing litchi farmers’ income. We draw on the studies of Wang and Zhao (2023), Mao et al. (2023), and Zhang et al. (2023), grouped the estimation based on farmers’ income, social network, and the number of family-specific agricultural input labor, using the mean value of each variable as the grouping threshold. Table 6 reports the regression results for these three farm household types. The findings indicate that DTA has a universal and inclusive nature, and its empowering and income-generating effect is more pronounced for disadvantaged farm households with low social capital, fewer laborers, and lower income levels. For disadvantaged farmers, DTA can alleviate the problems of low production efficiency and marketing difficulties due to their lack of PC and TC, making it easier for them to obtain and distribute production factors, as well as smoothly connect to factor and product markets. This allows them to expand their land scale and sales channels, thereby realizing income enhancement. In contrast, the advantaged farmers have multiple paths to improve their incomes and do not rely solely on DTA. Moreover, the stock of DTA is higher among the advantaged farmers, and the marginal effect of incremental DTA on their income improvement is already diminished, the income-boosting effect of DTA is more significant for the disadvantaged farmers. The findings of this study indicate that DTA does not significantly impact the FI of the advantaged farmers, which is quite different from the findings of Lajoie-O'Malley et al. (2020) and Qi et al. (2022), who argued that large-scale farmers, due to their substantial capital, are more adept at adopting digital technology in comparison to small-scale farmers. The possible reason for the divergence in findings could be that previous studies have not accounted for the varying levels of dependency on digital technologies among different farmers. Smaller farmers, possessing weaker PC and TC, are more dependent on digital technologies that enhance these capacities. Along with increased government efforts to bolster digital technology infrastructure and the rise of organizations offering digital agricultural socialization services, disadvantaged farmers now have better access to digital technologies. As a result, digital technologies are increasingly playing a crucial role in boosting the incomes of these disadvantaged farmers.
4.5.2 Heterogeneity analysis of region
There is significant variation in specialty agricultural resource endowment in China, resulting in different development levels of specialty agriculture across regions. This variation creates different application scenarios for digital technology, which may lead to regional variability in its empowering effects. Accordingly, we divided the sample into two regional groups: areas abundant in specialty agricultural resources and areas relatively deficient, based on the average value of these resources within the research sample. The regression results in Table 7 demonstrate that DTA has heterogeneous effects on different specialty agricultural resource areas. The DTA significantly empowers farmers to increase income in areas relatively rich in specialty agricultural resources, but it does not empower farmers to increase income in areas with poor specialty agricultural resources. This finding supports the resource evangelization theory (Yanıkkaya and Turan, 2018; Zhang et al., 2023), which suggests that regions endowed with specialty agricultural resources can better utilize their resource advantages in the digital economy era. The possible explanations for this are twofold: Firstly, areas with rich agricultural resources typically receive more extensive policy and resource support, which can attract more industrial and commercial capital. These industrial and commercial capitals often bring digital technology to rural areas, leveraging the technology diffusion effect, which helps facilitate local digital infrastructure improvement and enhance digital literacy among farmers, thereby enhancing the effect of DTA on farmers’ income. In contrast, regions with scarce resources often have inadequate digital infrastructure, hindering the empowerment effects of DTA. Secondly, the development of specialty agriculture in resource-scarce regions tends to lag, and the returns from specialty agricultural businesses are lower, prompting more farmers to seek off-farm employment. Consequently, even when farmers in these areas use digital technology, it is often for accessing external employment information rather than enhancing specialty agricultural production and management, resulting in minimal impact on the specialty agricultural farmers’ income.
5 Conclusions and implications
This study utilizes the resource-based theory and empowerment theory and follows the “resource-capability-performance” theoretical framework to examine the effect of DTA on FI, particularly focusing on specialty agricultural farmers. The findings of this study indicate: (1) The DTA increases specialty agricultural farmers’ income. (2) The DTA increases specialty agricultural farmers’ income mainly through enhancements in PC and TC. (3) The impact of DTA on FI exhibits varying intensities across different regions and farmers. On the whole, the hypotheses put forth in this study are validated.
This study makes important theoretical contributions. First, this study utilizes the resource-based theory and empowerment theory to explore the role of DTA in empowering specialty agricultural farmers, and effectively expanding the research boundaries of the empowerment theory. Previous studies have extensively explored the empowering effects of DTA on corporate employees, consumers, etc. However, prior research has paid little attention to the empowering effect of DTA on farmers. In other words, DTA empowering farmers is a neglected field. This study explains the path of DTA empowering farmers based on “resource-capability-performance” and builds an action strategy to empower farmers. Second, this study analyzes the internal mechanisms through which DTA promotes FI, thereby opening the “black box” of their relationship. This study explicates the pathway of DTA empowering farmers within the “resource-capability-performance” framework, formulates an action strategy for farmer empowerment, and reveals the process mechanism of DTA promoting FI. Last, this paper’s methods of measuring DTA behavior and FC advance the current research knowledge system and lay a solid foundation for the digital technology empowerment theory. Unlike previous literature that typically defines DTA on a single dimension, this paper conceptualizes DTA by considering activities along the entire agricultural industry chain, which is of great theoretical significance in revealing the connotation of different types of farmers’ DTA behaviors. Moreover, this paper refines the concept of FC by focusing on PC and TC, and identifies the intrinsic dimensions of each of the two different capabilities. This helps to improve the understanding of the FC, and makes an important theoretical contribution to the ongoing research on farmer empowerment.
Our findings offer valuable insights for governments seeking to guide farmers in the effective use of digital technologies to empower themselves and achieve higher incomes. First, this study confirms that DTA can enhance FI, which confirms the beneficial impact of DTA on farmers’ economic performance. Therefore, the government should strengthen investments in digital technology infrastructure, and at the same time intensify efforts to publicize and educate farmers about DTA, to make farmers understand the benefits of DTA. Second, this study indicates that DTA promotes FI by enhancing FC. This implies that governments should prioritize boosting FC. Therefore, governments should support the development of more digital technologies that apply to agricultural operations, and guide farmers to use digital technology in agricultural production and transactions, especially in the transaction process, to improve their PC and TC. Finally, this study confirms the differences in digital technology empowerment among different farmers and regions. Therefore, in promoting digital agriculture, the government should fully account for regional differences in specialty agricultural resources, and encourage relevant parties to use digital technology to explore and develop these resources. Moreover, the government should improve the relevance and precision of digital skills training for different farmers to enhance their ability to effectively use digital technology.
Three issues need further exploration in future research. First, the impact of different DTA patterns on farmers may not always be consistent, so in the future, there is a need to distinguish different DTA patterns when discussing their impacts on the various capacities of farmers, to understand the effectiveness of DTA empowerment comprehensively. Second, the cross-sectional data employed in this study is limited in its ability to identify causal effects. The effects of DTA may manifest with a delay and are not instantly observable. This study uses data from only 1 year, potentially hindering the revelation of the dynamic changes in the empowering capacity of DTA. Future research should conduct a more thorough empirical investigation using longitudinal tracking data. Last, this study did not deeply examine the impact of personal traits (e.g., self-efficacy, digital literacy, etc.), and situational variables (e.g., environmental dynamics, peer effects, etc.) on the process of digital technology empowerment for farmers. Future studies could explore deeper into the mechanisms of digital technology empowerment for farmers by considering these personal and situational factors.
Data availability statement
The raw data supporting the conclusions of this article will be made available by the authors, without undue reservation.
Author contributions
JX: Writing – review & editing, Writing – original draft, Methodology, Investigation, Formal analysis, Data curation. JW: Writing – original draft, Software, Investigation, Formal analysis, Writing – review & editing, Supervision, Resources, Project administration, Methodology, Funding acquisition, Conceptualization. ZD: Writing – original draft, Software, Conceptualization, Writing – review & editing, Methodology.
Funding
The author(s) declare that financial support was received for the research, authorship, and/or publication of this article. This research was funded by The National Social Science Fund Project of China (grant number 21&ZD090), The Guangdong Province Natural Science Foundation of China (grant number 2024A1515011302), The Guangdong Province Ordinary University Innovative Team Project of China (grant number 2023WCXTD003).
Conflict of interest
The authors declare that the research was conducted in the absence of any commercial or financial relationships that could be construed as a potential conflict of interest.
Publisher’s note
All claims expressed in this article are solely those of the authors and do not necessarily represent those of their affiliated organizations, or those of the publisher, the editors and the reviewers. Any product that may be evaluated in this article, or claim that may be made by its manufacturer, is not guaranteed or endorsed by the publisher.
Footnotes
1. ^Data source: The Ministry of Agriculture and Rural Affairs of the People’s Republic of China.
2. ^Yuan is a Chinese currency; 1 yuan is equivalent to USD 0.14 in 2024.
3. ^Data source: The China Internet Network Information Center (CNNIC).
1. ^Mu is a Chinese unit. One hectare is equivalent to 15 mu.
2. ^Data source: The General Administration of Customs of the People’s Republic of China.
1. ^The scales measuring PC and TC successfully passed reliability and validity tests. Specifically, Cronbach’s alpha coefficients for the measurement items of both PC and TC were 0.851 and 0.841 respectively, well above the acceptable threshold of 0.6. Additionally, the alpha coefficients for each individual measurement item were greater than 0.7, indicating high reliability in the measurement variables. Factor analysis confirmed that the overall KMO values for PC and TC were 0.830 and 0.839, respectively. The probability values for Bartlett’s test of sphericity were 0.000, showing excellent correlation and validity among the scale items.
References
Abate, G., Bernard, T., and Minot, N. (2018). The impact of the use of new technologies on farmers’ wheat yield in Ethiopia: evidence from a randomized control trial. Agric. Econ. 49, 409–421. doi: 10.1111/agec.12425
Abdulai, A., Gibson, R., and Fraser, E. (2023). Beyond transformations: zooming in on agricultural digitalization and the changing social practices of rural farming in northern Ghana, West Africa. J. Rural. Stud. 100:103019. doi: 10.1016/j.jrurstud.2023.103019
Abiri, R., Rizan, N., Balasundram, S., Shahbazi, A., and Abdul-Hamid, H. (2023). Application of digital technologies for ensuring agricultural productivity. Heliyon 9:e22601. doi: 10.1016/j.heliyon.2023.e22601
Aker, J., and Ksoll, C. (2016). Can mobile phones improve agricultural outcomes? Evidence from a randomized experiment in Niger. Food Policy 60, 44–51. doi: 10.1016/j.foodpol.2015.03.006
Albayram, Z., Mattas, K., and Tsakiridou, E. (2014). Purchasing local and non-local products labeled with geographical indications (GIs). Oper. Res. 14, 237–251. doi: 10.1007/s12351-014-0154-9
Ali-Hassan, H., Nevo, D., and Wade, M. (2015). Linking dimensions of social media use to job performance: the role of social capital. J. Strateg. Inf. Syst. 24, 65–89. doi: 10.1016/j.jsis.2015.03.001
Ancillai, C., Sabatini, A., Gatti, M., and Perna, A. (2023). Digital technology and business model innovation: a systematic literature review and future research agenda. Technol. Forecast. Soc. Chang. 188:122307. doi: 10.1016/j.techfore.2022.122307
Ansah, I., Lambongang, M., and Donkoh, S. (2020). Ghana’s planting for food and jobs programme: a look at the role of capability in farmers’ participation. J. Hum. Develop. Capabil. 21, 161–182. doi: 10.1080/19452829.2020.1745162
Astill, G., Perez, A., and Thornsbury, S. (2020). Developing automation and mechanization for specialty crops: a review of US Department of Agriculture Programs: a report to Congress. U.S. Department of Agriculture, Econ. Res. Rep. AP-082, 1–26. doi: 10.22004/ag.econ.320792
Biagini, L., Antonioli, F., and Severini, S. (2023). The impact of CAP subsidies on the productivity of cereal farms in six European countries: a historical perspective (2008–2018). Food Policy 119:102473. doi: 10.1016/j.foodpol.2023.102473
Carrer, M., De Souza Filho, H., Batalha, M., and Rossi, F. (2015). Farm management information systems (FMIS) and technical efficiency: an analysis of citrus farms in Brazil. Comput. Electron. Agric. 119, 105–111. doi: 10.1016/j.compag.2015.10.013
Carrer, M., Filho, H., Vinholis, M., and Mozambani, C. (2022). Precision agriculture adoption and technical efficiency: an analysis of sugarcane farms in Brazil. Technol. Forecast. Soc. Chang. 177:121510. doi: 10.1016/j.techfore.2022.121510
Cha, J., Deng, Y., Zheng, S., and Li, F. (2024). Crop insurance, factor allocation, and farmers’ income: evidence from Chinese pear farmers. Front. Sustain. Food Syst. 8:1378382. doi: 10.3389/fsufs.2024.1378382
Chen, J. (2019). Resilient smallholder farming: historical continuity and modern conversion - the vitality and autonomous responsibility mechanism of smallholder farmers in China. China Soc. Sci. 12, 82–99.
Cheng, M., Gai, Q., Jin, Y., and Shi, Q. (2016). Focusing on human capital improvement and income growth. Econ. Res. J. 1, 168–181.
Dias, C., Gouveia, R., and Ferreira, J. (2021). Small agricultural businesses’ performance-what is the role of dynamic capabilities, entrepreneurial orientation, and environmental sustainability commitment? Bus. Strateg. Environ. 30, 1898–1912. doi: 10.1002/bse.2723
Diiro, G., Fisher, M., Kassie, M., Muriithi, B., and Muricho, G. (2021). How does adoption of labor saving agricultural technologies affect intrahousehold resource allocations? The case of push-pull technology in Western Kenya. Food Policy 102:102114. doi: 10.1016/j.foodpol.2021.102114
Duvaleix, S., Emlinger, C., Gaigné, C., and Latouche, K. (2021). Geographical indications and trade: firm-level evidence from the French cheese industry. Food Policy 102:102118. doi: 10.1016/j.foodpol.2021.102118
Eisenhardt, K., and Martin, A. (2000). Dynamic capabilities: what are they? Strateg. Manag. J. 21, 1105–1121. doi: 10.1002/1097-0266(200010/11)21:10/11<1105::AID-SMJ133>3.0.CO;2-E
Flores, H., Villalobos, J., Ahumada, O., Uchanski, M., Meneses, C., and Sanchez, O. (2019). Use of supply chain planning tools for efficiently placing small farmers into high-value, vegetable markets. Comput. Electron. Agric. 157, 205–217. doi: 10.1016/j.compag.2018.12.050
Garg, S., Jha, G., Kim, S., Miller, Z., and Kuo, W. (2023). The need and development for a value-added toolkit—a case study with Montana specialty fruit growers. Front. Sustain. Food Syst. 7:1084750. doi: 10.3389/fsufs.2023.1084750
Gramzow, A., Batt, P., Afari-Sefa, V., Petrick, M., and Roothaert, R. (2018). Linking smallholder vegetable producers to markets-a comparison of a vegetable producer group and a contract-farming arrangement in the Lushoto District of Tanzania. J. Rural. Stud. 63, 168–179. doi: 10.1016/j.jrurstud.2018.07.011
Guo, X., Wang, L., Meng, X., Dong, X., and Gu, L. (2023). The impact of digital inclusive finance on farmers’ income level: evidence from China’s major grain production regions. Financ. Res. Lett. 58:104531. doi: 10.1016/j.frl.2023.104531
Hidalgo, F., Quiñones-Ruiz, X., Birkenberg, A., Daum, T., Bosch, C., Hirsch, P., et al. (2023). Digitalization, sustainability, and coffee. Opportunities and challenges for agricultural development. Agric. Syst. 208:103660. doi: 10.1016/j.agsy.2023.103660
Hoang, V., Nguyen, T., Wilson, C., Ho, T., and Khanal, U. (2021). Scale and scope economies in small household rice farming in Vietnam. J. Integr. Agric. 20, 3339–3351. doi: 10.1016/S2095-3119(21)63612-2
Kabbiri, R., Dora, M., Kumar, V., Elepu, G., and Gellynck, X. (2018). Mobile phone adoption in Agri-food sector: are farmers in sub-Saharan Africa connected? Technol. Forecast. Soc. Chang. 131, 253–261. doi: 10.1016/j.techfore.2017.12.010
Kai, L., Yu, J., and Zhou, J. (2023). Are vulnerable farmers more easily influenced? Heterogeneous effects of internet use on the adoption of integrated pest management. J. Integr. Agric. 22, 3220–3233. doi: 10.1016/j.jia.2023.08.005
Karanasios, S., and Slavova, M. (2019). How do development actors do “ICT for development”? A strategy-as-practice perspective on emerging practices in Ghanaian agriculture. Inf. Syst. J. 29, 888–913. doi: 10.1111/isj.12214
Khanna, M. (2021). Digital transformation of the agricultural sector: pathways, drivers and policy implications. Appl. Econ. Perspect. Policy 43, 1221–1242. doi: 10.1002/aepp.13103
Kvam, G., Hårstad, R., and Stræte, E. (2022). The role of farmers’ micro AKIS at different stages of uptake of digital technology. J. Agric. Educ. Ext. 28, 671–688. doi: 10.1080/1389224X.2022.2046617
Lajoie-O'Malley, A., Bronson, K., Van Der Burg, S., and Klerkx, L. (2020). The future (s) of digital agriculture and sustainable food systems: an analysis of high-level policy documents. Ecosyst. Serv. 45:101183. doi: 10.1016/j.ecoser.2020.101183
Li, E., Deng, Q., and Zhou, Y. (2022). Livelihood resilience and the generative mechanism of rural households out of poverty: an empirical analysis from Lankao County, Henan Province, China. J. Rural. Stud. 93, 210–222. doi: 10.1016/j.jrurstud.2019.01.005
Li, W., and Gan, Y. (2022). Can specialty agriculture enrich farmers? A perspective based on the relationship between herbal medicine cultivation, Chinese medicine revitalization and farmers’ income increase. Rural Econ. 11, 134–143.
Li, T., Sun, X., and Feng, K. (2020). The design of food security system based on feasible ability theory in China: labor transfer, logic of factor substitution and feasible ability improvement. On Econ. Problem 9, 100–108. doi: 10.16011/j.cnki.jjwt.2020.09.013
Liang, W., and Jiao, L. (2022). Research on improving the development ability of population lifted out of poverty from the perspective of capacity poverty. J. Huazhong Agric. Univ. 6, 99–109. doi: 10.13300/j.cnki.hnwkxb.2022.06.010
Lien, G., Kumbhakar, S., Mishra, A., and Hardaker, J. (2022). Does risk management affect productivity of organic rice farmers in India? Evidence from a semiparametric production model. Eur. J. Oper. Res. 303, 1392–1402. doi: 10.1016/j.ejor.2022.03.051
Lin, B., Wang, X., Jin, S., Yang, W., and Li, H. (2022). Impacts of cooperative membership on rice productivity: evidence from China. World Dev. 150:105669. doi: 10.1016/j.worlddev.2021.105669
Lin, H., Zhao, L., and Hu, Y. (2023). Whether digital village construction can promote common wealth in old revolutionary areas. China Rural Econ. 5, 81–102. doi: 10.20077/j.cnki.11-1262/f.2023.05.005
Liu, Y. (2022). Evaluation of the effect of rural digitalization in improving farmers’ living standards and research on the mechanis. Guizhou Soc. Sci. 2, 160–168. doi: 10.13713/j.cnki.cssci.2022.02.006
Llorente-Alonso, M., Garcia-Ael, C., and Topa, G. (2024). A meta-analysis of psychological empowerment: antecedents, organizational outcomes, and moderating variables. Curr. Psychol. 43, 1759–1784. doi: 10.1007/s12144-023-04369-8
Luther, G., Mariyono, J., Purnagunawan, R., Satriatna, B., and Siyaranamual, M. (2018). Impacts of farmer field schools on productivity of vegetable farming in Indonesia. Nat. Res. Forum 42, 71–82. doi: 10.1111/1477-8947.12144
Ma, W., McKay, A., Rahut, D., and Sonobe, T. (2023). An introduction to rural and agricultural development in the digital age. Rev. Dev. Econ. 27, 1273–1286. doi: 10.1111/rode.13025
Ma, W., Rahut, D., Sonobe, T., and Gong, B. (2024). Linking farmers to markets: barriers, solutions, and policy options. Econ. Analysis Policy 82, 1102–1112. doi: 10.1016/j.eap.2024.05.005
Mäkinen, M. (2006). Digital empowerment as a process for enhancing citizens’ participation. E Learn. Digital Media 3, 381–395. doi: 10.2304/elea.2006.3.3
Mao, H., Liu, S., Peng, P., and Yang, Z. (2023). Digital extension and farmers’ fertilizer reduction: an empirical analysis of China’ s major apple producing areas in Shaanxi. Chinese Rural Econ. 2, 66–84. doi: 10.20077/j.cnki.11-1262/f.2023.02.006
Marshall, A., Turner, K., Richards, C., Foth, M., and Dezuanni, M. (2022). Critical factors of digital AgTech adoption on Australian farms: from digital to data divide. Inf. Commun. Soc. 25, 868–886. doi: 10.1080/1369118X.2022.2056712
Mauki, C., Jeckoniah, J., and Massawe, G. (2023). Smallholder rice farmers profitability in agricultural marketing co-operative societies in Tanzania: a case of Mvomero and Mbarali districts. Heliyon 9:e17039. doi: 10.1016/j.heliyon.2023.e17039
Neill, C., and Morgan, K. (2021). Beyond scale and scope: exploring economic drivers of US specialty crop production with an application to edamame. Front. Sustain. Food Syst. 4:582834. doi: 10.3389/fsufs.2020.582834
Peng, Y., Zhou, H., and Su, L. (2022). Does the participation in digital economy improve farmers’ social class identity? Empirical evidence from Ningxia, Chongqing, and Sichuan Province. Chin. Rural Econ. 10, 59–81.
Perkins, D., and Zimmerman, M. (1995). Empowerment theory, research, and application. Am. J. Commun. Psychol. 23, 569–579. doi: 10.1007/BF02506982
Peterson, N., Lowe, J., Aquilino, M., and Schneider, J. (2005). Linking social cohesion and gender to intrapersonal and interactional empowerment: support and new implications for theory. J. Community Psychol. 33, 233–244. doi: 10.1002/jcop.20047
Qi, W., Chen, H., and Li, J. (2023). Development status, trend and countermeasures of litchi industry in mainland China in 2022. Guangdong Agric. Sc. 50, 147–155. doi: 10.16768/j.issn.1004-874X.2023.02.016
Qi, Y., Han, J., Shadbolt, N., and Zhang, Q. (2022). Can the use of digital technology improve the cow milk productivity in large dairy herds? Evidence from China’s Shandong Province. Front. Sustain. Food Syst. 6:1083906. doi: 10.3389/fsufs.2022.1083906
Qiu, H., Feng, M., Tang, W., Luo, M., Wang, J., and Ye, F. (2024). Analysis of the impact of farm machinery outsourcing services on farmers’ cropping structure and grain production: an empirical evidence from Henan Province, China. Front. Sustain. Food Syst. 8:1365576. doi: 10.3389/fsufs.2024.1365576
Qureshi, I., Pan, S., and Zheng, Y. (2021). Digital social innovation: an overview and research framework. Inf. Syst. J. 31, 647–671. doi: 10.1111/isj.12362
Rappaport, J. (1987). Terms of empowerment/exemplars of prevention: toward a theory for community psychology. Am. J. Community Psychol. 15, 121–148. doi: 10.1007/BF00919275.3604997
Rihn, A., Velandia, M., Warner, L., Fulcher, A., Schexnayder, S., and LeBude, A. (2023). Factors correlated with the propensity to use automation and mechanization by the US nursery industry. Agribusiness 39, 110–130. doi: 10.1002/agr.21763
Ruiz-Altisent, M., Ruiz-Garcia, L., Moreda, G., Lu, R., Hernandez-Sanchez, N., Correa, E., et al. (2010). Sensors for product characterization and quality of specialty crops-a review. Comput. Electron. Agric. 74, 176–194. doi: 10.1016/j.compag.2010.07.002
Santos, S., Neumeyer, X., and Morris, M. (2019). Entrepreneurship education in a poverty context: an empowerment perspective. J. Small Bus. Manag. 57, 6–32. doi: 10.1111/jsbm.12485
Scheerder, A., Van Deursen, A., and Van Dijk, J. (2017). Determinants of internet skills, uses and outcomes. A systematic review of the second-and third-level digital divide. Telematics Inform. 34, 1607–1624. doi: 10.1016/j.tele.2017.07.007
Schnebelin, É. (2022). Linking the diversity of ecologisation models to farmers’ digital use profiles. Ecol. Econ. 196:107422. doi: 10.1016/j.ecolecon.2022.107422
Séogo, W., and Zahonogo, P. (2023). Do land property rights matter for stimulating agricultural productivity? Empirical evidence from Burkina Faso. Land Use Policy 125:106475. doi: 10.1016/j.landusepol.2022.106475
Sirmon, D., Hitt, M., Ireland, R., and Gilbert, B. (2011). Resource orchestration to create competitive advantage: breadth, depth, and life cycle effects. J. Manag. 37, 1390–1412. doi: 10.1177/0149206310385695
Smidt, H., and Jokonya, O. (2022). Factors affecting digital technology adoption by small-scale farmers in agriculture value chains (AVCs) in South Africa. Inf. Technol. Dev. 28, 558–584. doi: 10.1080/02681102.2021.1975256
Tamirat, T., Pedersen, S., and Lind, K. (2018). Farm and operator characteristics affecting adoption of precision agriculture in Denmark and Germany. Acta Agric. Scandinavica 68, 349–357. doi: 10.1080/09064710.2017.1402949
Tao, Z., and Chao, J. (2024). The impact of a blockchain-based food traceability system on the online purchase intention of organic agricultural products. Innovative Food Sci. Emerg. Technol. 92:103598. doi: 10.1016/j.ifset.2024.103598
Teece, D., Pisano, G., and Shuen, A. (1997). Dynamic capabilities and strategic management. Strateg. Manag. J. 18, 509–533. doi: 10.1002/(SICI)1097-0266(199708)18:7<509::AID-SMJ882>3.0.CO;2-Z
Thomas, K., and Velthouse, B. (1990). Cognitive elements of empowerment: an “interpretive” model of intrinsic task motivation. Acad. Manag. Rev. 15, 666–681. doi: 10.5465/amr.1990.4310926
Wan, J. (2022). Developing rural specialty industries and broadening the channels for farmers to increase income and become rich. Agric. Econ. Manag. 6, 19–22.
Wang, H., Wang, X., Sarkar, A., and Qian, L. (2021). Evaluating the impacts of smallholder farmer’s participation in modern agricultural value chain tactics for facilitating poverty alleviation-a case study of kiwifruit industry in Shaanxi, China. Agriculture 11:462. doi: 10.3390/agriculture11050462
Wang, Y., Yang, A., Li, Y., and Yang, Q. (2023). Effect of e-commerce popularization on farmland abandonment in rural China: evidence from a large-scale household survey. Land Use Policy 135:106958. doi: 10.1016/j.landusepol.2023.106958
Wang, J., Yang, G., and Zhou, C. (2024). Does internet use promote agricultural green development? Evidence from China. Int. Rev. Econ. Finance 93, 98–111. doi: 10.1016/j.iref.2024.03.009
Wang, X., and Zhao, Y. (2023). Competition of bank and common prosperity of rural households: based on the perspectives of absolute income and relative income. Econ. Res. J. 9, 98–115.
Wassie, S., Mengistu, D., and Birlie, A. (2023). Agricultural livelihood resilience in the face of recurring droughts: empirical evidence from Northeast Ethiopia. Heliyon 9:e16422. doi: 10.1016/j.heliyon.2023.e16422
Wernerfelt, B. (1984). A resource-based view of the firm. Strateg. Manag. J. 5, 171–180. doi: 10.1002/smj.4250050207
Wu, L., and Zhao, W. (2023). Small cuts to realize big changes in the agricultural industry: sample of Guangdong’s practice in creating specialty agricultural products advantage zones. Available at: https://www.rmzxb.com.cn/c/2023-11-01/3436029.shtml (Accessed November 1, 2023).
Wyche, S., and Steinfield, C. (2016). Why don’t farmers use cell phones to access market prices? Technology affordances and barriers to market information services adoption in rural Kenya. Inf. Technol. Dev. 22, 320–333. doi: 10.1080/02681102.2015.1048184
Xu, J., Wan, J., and Han, L. (2023). Digital technology usage, socialization services for agricultural production and farmers’ operating income gap-empirical study based on the CLDS data. J. Shanxi Univ. Finance Econ. 6, 33–44. doi: 10.13781/j.cnki.1007-9556.2023.06.003
Xu, X., and Wang, W. (2022). Can digital infrastructure deliver inclusive growth? Based on the perspective of capital accumulation. Soft Sci. 11, 9–14. doi: 10.13956/j.ss.1001-8409.2022.11.02
Xu, Y., and Wang, L. (2023). Guangdong’s 1.6 million tons of lychees sold for 16 billion yuan. Available at: https://ep.ycwb.com/epaper/ycwb/html/2023-07/29/content_6_589976.htm (Accessed July 29, 2023).
Xu, Z., Zhang, C., and Liu, Z. (2023). How agricultural technology is reshaping the countryside is based on? A three-dimensional investigation of individuals, families, and villages. Rural Econ. 3, 108–117.
Yanıkkaya, H., and Turan, T. (2018). Curse or blessing? An empirical re-examination of natural resource-growth nexus. J. Int. Dev. 30, 1455–1473. doi: 10.1002/jid.3374
Yao, B., Shanoyan, A., Schwab, B., and Amanor-Boadu, V. (2022). Mobile money, transaction costs, and market participation: evidence from Côte d’ Ivoire and Tanzania. Food Policy 112:102370. doi: 10.1016/j.foodpol.2022.102370
Yi, F., Yao, L., Sun, Y., and Cai, Y. (2023). E-commerce participation, digital finance and farmers’ income. China Agric. Econ. Rev. 15, 833–852. doi: 10.1108/CAER-03-2023-0053
Yitayew, A., Abdulai, A., and Yigezu, Y. (2023). The effects of advisory services and technology channeling on farm yields and technical efficiency of wheat farmers in Ethiopia. Food Policy 116:102436. doi: 10.1016/j.foodpol.2023.102436
Zhang, Z. (2022). Internet use and income inequality among farmers. Econ. Survey 39, 45–54. doi: 10.15931/j.cnki.1006-1096.2022.03.009
Zhang, B., Liu, F., Hou, X., Zhu, H., Niu, W., Jin, Y., et al. (2023). The impact mechanism of “one household one plot” cultivated land fragmentation governance on farmers’ income: an empirical analysis based on data of Yuyang District in Shaanxi Province. China Land Sci. 4, 73–83.
Zhang, H., Ma, W., Li, J., and Yang, W. (2023). Can citrus farmers earn more from selling online? Econ. Analysis Policy 80, 1549–1560. doi: 10.1016/j.eap.2023.10.026
Zhao, S., and Yue, C. (2020). Risk preferences of commodity crop producers and specialty crop producers: an application of prospect theory. Agric. Econ. 51, 359–372. doi: 10.1111/agec.12559
Zheng, H., and Ma, W. (2023). Smartphone-based information acquisition and wheat farm performance: insights from a doubly robust IPWRA estimator. Electron. Commer. Res. 23, 633–658. doi: 10.1007/s10660-021-09481-0
Zheng, K., and Wu, X. (2024). Discussion on the entrepreneurial effect and heterogeneity of internet-empowered family farms under the background of the “three-level digital divide”. Rural Economy 3, 16–30.
Zheng, Y., Zhu, T., and Wei, J. (2022). Does internet use promote the adoption of agricultural technology? Evidence from 1449 farm households in 14 Chinese provinces. J. Integr. Agric. 21, 282–292. doi: 10.1016/S2095-3119(21)63750-4
Zhong, X., Zhang, M., Tang, T., Adhikari, B., and Ma, Y. (2023). Advances in intelligent detection, monitoring, and control for preserving the quality of fresh fruits and vegetables in the supply chain. Food Biosci. 56:103350. doi: 10.1016/j.fbio.2023.103350
Zhou, X., Cui, Y., and Zhang, S. (2020). Internet use and rural residents’ income growth. China Agric. Econ. Rev. 12, 315–327. doi: 10.1108/CAER-06-2019-0094
Zhou, L., and Shen, K. (2022). Impact of the rural land system reform on rural households’ income growth in China: evidence from the three rights separation. Econ. Res. J. 5, 141–157.
Keywords: digital technology application, specialty agricultural farmers, productive capacity, transaction capacity, China
Citation: Xu J, Wan J and Dai Z (2024) How does digital technology application empower specialty agricultural farmers? Evidence from Chinese litchi farmers. Front. Sustain. Food Syst. 8:1444192. doi: 10.3389/fsufs.2024.1444192
Edited by:
Isabelle Piot-Lepetit, INRAE Occitanie Montpellier, FranceReviewed by:
Ghulam Rasool Madni, University of Education Lahore, PakistanIndira Devi Puthussery, Kerala Agricultural University, India
Anup Pradhan, University of Johannesburg, South Africa
Copyright © 2024 Xu, Wan and Dai. This is an open-access article distributed under the terms of the Creative Commons Attribution License (CC BY). The use, distribution or reproduction in other forums is permitted, provided the original author(s) and the copyright owner(s) are credited and that the original publication in this journal is cited, in accordance with accepted academic practice. No use, distribution or reproduction is permitted which does not comply with these terms.
*Correspondence: Junyi Wan, V2FuanlzY2F1QDE2My5jb20=