- 1Department of Agricultural Economics, Wolaita Sodo University, Wolaita Sodo, Ethiopia
- 2Department of Economics, Addis Ababa University, Addis Ababa, Ethiopia
Introduction: Low adoption rates of digital tools in agricultural extension services persisted among smallholder ginger producers in Southern and Central Ethiopia, despite their recognized benefits. This study investigated the factors that drove or hindered digital tool adoption in this context.
Methods: A mixed-methods approach was used, combining qualitative interviews and quantitative data analysis. The Endogenous Switching Regression model was applied to examine the socio-economic, institutional, and technological factors affecting adoption.
Results: The results showed that digital tool adoption significantly enhanced both agricultural productivity and household income among smallholder farmers. Key determinants included access to digital infrastructure, availability of ICT resources, and tailored extension services.
Discussion: The findings suggested a need for policies that promoted digital adoption, emphasizing infrastructure investment, expanded ICT access, and the development of specialized extension programs. These actions were seen as crucial for advancing rural livelihoods, supporting sustainable agriculture, and stabilizing the regional economy.
1 Introduction
In an era marked by rapid technological advancements, digital tools have emerged as pivotal resources for agricultural extension services, offering innovative ways to disseminate information and empower farm households (Daniso et al., 2020; Krell et al., 2021). Digital tools hold transformative potential for agriculture in Ethiopia, offering innovative solutions to boost productivity and reduce environmental impact (Smith, 2018). The agricultural sector in Ethiopia, particularly among smallholder ginger producers in Southern and Central regions, plays a vital role in the country’s economy, livelihoods, and food security (Tilore et al., 2024). With the increasing penetration of digital technologies, there is growing interest in understanding the determinants of digital tool adoption in agricultural extension services and their welfare impact on smallholder farmers (Abdulai et al., 2023).
Despite Ethiopia’s progress toward digital agriculture, challenges such as weak digital infrastructure, limited data sharing policies, and low digital literacy among farmers hinder widespread adoption (Kropff et al., 2023). Additionally, ginger production faces numerous challenges, including limited access to information, input markets, and extension services, which hinder productivity and income generation (Kifile et al., 2023). While digital tools offer promising solutions, low adoption rates among smallholder ginger farmers persist, limiting the sector’s potential growth and impact. This gap underscores the need for urgent research to identify the barriers to adoption and to provide actionable insights for promoting the uptake of digital technologies in rural agricultural communities. In response, digital tools such as mobile applications, agronomic advisory platforms, and remote sensing technologies offer innovative solutions to address these challenges and enhance agricultural extension services (Agnihotri et al., 2023).
Despite the potential benefits, the adoption of digital tools among smallholder ginger producers in Ethiopia remains critically low (Haile et al., 2019), creating an urgent need for targeted research into the socio-economic, technological, and institutional factors affecting technology uptake.
Recent empirical studies highlighted the transformative potential of digital technology in agricultural extension services but identified gaps such as overlooking factors like motivation and ability in technology adoption (Oyinbo et al., 2020), lack of comprehensive examination of potential barriers (Abebe and Mammo Cherinet, 2019), and reliance on self-reported (Bolfe et al., 2020) or secondary data (Thakur et al., 2019). This study aimed to fill this gap by investigating the determinants of the adoption of digital technology as extension tools among farm households in South and Central Ethiopia and assessing their welfare impact on income and yield. By employing a mixed-methods approach that combined quantitative surveys and qualitative interviews, the research sought to uncover the socio-economic, institutional, and technological factors shaping farmers’ decisions to adopt digital tools and their subsequent effects on welfare outcomes.
The findings of this study contributed valuable insights into the role of digital tools in agricultural extension services and their potential to enhance the livelihoods of smallholder ginger producers in Ethiopia. By elucidating the determinants of digital tool adoption and assessing their welfare impact, this research aimed to guide policymakers, development practitioners, and extension service providers in designing targeted interventions to promote the uptake of digital technologies and support sustainable agricultural development in the region.
In the following sections, we reviewed the existing literature, detailed the study’s methodology, presented the empirical results, and discussed the implications for policymakers, development practitioners, and the agricultural community.
2 Literature review
2.1 Theoretical approach
The theory of utility maximization served as a foundational framework for understanding the decision-making process of farm households regarding the adoption of digital technology (Awunyo-Vitor, 2018). According to this theory, individuals sought to maximize their utility or satisfaction from available resources, subject to various constraints such as income, prices, and technological opportunities (Rosen et al., 2019). In the context of adopting digital technology for agricultural extension, farm households were expected to evaluate the potential benefits and costs of using these tools in terms of enhancing their agricultural productivity, knowledge acquisition, and overall welfare (Takahashi et al., 2020). By applying the principle of utility maximization, this study aimed to elucidate the factors that influenced farmers’ decisions to adopt digital technology, considering their preferences, resource endowments, and institutional environments.
Comparatively, other impact study theories, such as the Technology Acceptance Model (TAM) and the Diffusion of Innovations theory, offered complementary perspectives on understanding technology adoption and its effects. TAM posited that individuals’ acceptance and usage of new technology were determined by perceived usefulness and ease of use (Davis, 1987). Meanwhile, the Diffusion of Innovations theory emphasized the role of social networks and communication channels in the spread of innovations within a community (Rogers, 1983). Each theory provides a unique lens for analyzing adoption: utility maximization looks at the individual decision-making process, while TAM focuses on perceptions of technology, and Diffusion of Innovations highlights the social dynamics of adoption. Integrating these theories with the utility maximization framework allowed for a comprehensive analysis of the adoption process, incorporating both individual-level motivations and social dynamics shaping technology uptake among farm households.
The theory of utility maximization was applied to understand the adoption of digital technology among farm households using mathematical formulations. The basic premise was that households made decisions to maximize their utility subject to budget constraints. In the context of digital technology adoption, households allocated their resources (e.g., time, money) to obtain the maximum utility from using these technologies.
One way to represent utility maximization mathematically was through the following optimization problem (Equation 1):
Where: represented the utility function, which captures the satisfaction or wellbeing derived from consuming a bundle of goods or services (including digital technology). , was a vector of quantities of different goods or services consumed (including digital technology). , represented the price of each good or service. , was the household’s income or budget constraint. In the context of digital technology adoption, included the quantities of different digital tools (e.g., text messages, phone calls, YouTube) used by farm households. The utility function, , captured the perceived benefits or satisfaction derived from using these technologies, which could be influenced by factors such as improved access to information, increased productivity, or enhanced communication.
In this study, the utility maximization framework provided a lens through which to examine the cost–benefit considerations underlying farmers’ adoption decisions regarding digital technology. By conducting empirical analyses that accounted for factors such as farmers’ socio-economic characteristics, access to information and communication technologies (ICTs), and perceptions of technology usefulness, the study aimed to uncover the drivers and barriers to adoption. Furthermore, by assessing the impact of digital technology adoption on farm household welfare indicators such as income and yield, the research sought to demonstrate the practical implications of utility-maximizing behavior in the context of agricultural extension. By integrating these three theoretical frameworks, this study offers a comprehensive analysis of the factors influencing digital tool adoption, encompassing both individual and collective decision-making processes. Through this approach, the study endeavored to generate actionable insights for policymakers and development practitioners seeking to promote the effective use of digital tools in agricultural development strategies.
2.2 Conceptual framework
Our conceptual framework for studying the adoption of digital extension tools among farm households in South and Central Ethiopia incorporated various influential variables categorized into socioeconomic, demographic, institutional, and information-related factors (Figure 1). Socioeconomic variables included education, income, farm size, access to credit, and access to market information (Sikundla et al., 2018). Demographic variables encompassed age and gender (Lampé, 2006). Institutional variables consisted of access to extension services, social networks, technological literacy, and access to ICTs (Gebrehiwot and van der Veen, 2021). Information variables included the availability and accessibility of information regarding digital technology (Lampé, 2006).
Key determinants such as education, income, and farm size were expected to positively influence technology adoption, reflecting higher levels of education and income, as well as larger farm sizes (Sikundla et al., 2018). Additionally, factors such as access to extension services, credit, social networks, information, technological literacy, access to market information, and access to ICTs were anticipated to facilitate technology adoption by providing crucial resources, information, and support to farm households (Gebrehiwot and van der Veen, 2021). Furthermore, demographic factors such as age and gender were integrated into the framework, acknowledging their potential impact on technology adoption patterns (Lampé, 2006). By considering these variables and their hypothesized relationships, our framework aimed to offer a comprehensive understanding of the multifaceted determinants shaping technology adoption among farm households in Ethiopia. This framework served as a guiding tool for our analysis, informing variable selection and facilitating the exploration of their interactions in shaping technology adoption behaviors within agricultural communities.
3 Methodology
3.1 Description of the study area
The study was conducted in two districts, Boloso-Bombe and Hadaro-Tunto, located in the Wolaita zone and Kembata-Tembaro zone, respectively. These zones were reorganized under different regional states as a result of the Ethiopian government’s reformation in 2018. The Wolaita zone is now part of Southern Ethiopia, serving as an administrative and political center, while the Kembata-Tembaro zone situated in Central Ethiopia. The administrative structure comprises 22 districts in the Wolaita zone and eight districts in the Kembata-Tembaro zone. The selected districts are known for their predominant reliance on agriculture, with ginger production being a significant contributor to the local economy.
Agriculture, especially crop production, is the main livelihood of both districts, with ginger production taking a lion’s share, followed by cereals like maize, teff, etc.; root crops such as taro and sweet potato; and animal husbandry. The rationale for selecting ginger producers specifically over other crop producers stems from the unique economic and climatic significance of ginger in these regions. Unlike other crops, ginger faces distinct challenges, such as market volatility and vulnerability to disease, making it an ideal case for studying digital tool adoption to enhance productivity and food security. Figure 2 below showed map of the study area.
3.2 Selection of sample size and sample size design
To ensure a robust sampling strategy, we employed a multistage sampling approach to select our sample respondents. Firstly, we identified two agricultural zones in the study area: the Wolaita zone, comprising Boloso-Bombe district, and the Kembata-Tembaro zone, including Hadaro-Tunto district. These zones were chosen due to their significant ginger production activities. The two districts, Boloso-Bombe and Hadaro-Tunto, from the Wolaita and Kembata-Tembaro zones, respectively, were purposively selected in the first stage as they are known for ginger production in terms of ginger land area coverage and total ginger production in the southern and central regions, respectively (Prameela and Suseela Bhai, 2020). Moving to the second stage, we employed a quota sampling method to select kebeles within each district. Randomly, we chose four kebeles from the 18 kebeles in Boloso-Bombe district and two kebeles from the 16 rural kebeles in Hadaro-Tunto district. This selection was based on the proportion of ginger producing kebeles in each district, ensuring representation across the study area. Bidin (2017) Provides several sample size determination formulas based on different statistical considerations (Equation 2).
where n represents the sample size; Z represents the cumulative standard distribution, which corresponds to the confidence level with a value of 1.96; e is the desired precision level, as suggested by Freeman et al. (1992); a p value of 0.5 indicates the estimated proportion of an attribute present in the population required to obtain the desired minimum level of sample size at the 95% confidence level and 5% precision; q = 1 – p; and N represents the total size of the population from which the sample is drawn.
Subsequently, within the selected kebeles, we utilized a simple random sampling technique to choose households for participation. From the total of 3,208 households in the selected kebeles, we randomly selected 343 households. The distribution of the sample within each kebele was proportional to the number of ginger farmers. For instance, in Boloso-Bombe district, 64 households were selected from Gamo Walalna, 29 from Matala Walana, 67 from Parawocha, and 50 from Adila. Similarly, in Hadaro-Tunto district, 66 households were chosen from Mukurunja and 67 from Ajora. The sample size distribution is presented in Table 1.
3.3 Data types sources and methods of collection
This study utilized quantitative, cross-sectional data to explore the adoption of digital technology among farm households in South and Central Ethiopia. Quantitative data, crucial for measuring variables like income, yield, and education levels, enabled precise statistical analysis. The data was collected at a single point in time, providing a snapshot of current technology adoption and its impact on household welfare. The primary source of data was a structured household survey conducted in the Wolaita and Kembata-Tembaro zones, chosen for their significant ginger production activities. Supplementary data from local agricultural offices and secondary sources enhanced the analysis by providing additional context.
The data collection employed a multistage sampling approach to ensure a representative sample. The Wolaita and Kembata-Tembaro zones were purposively selected, followed by a quota sampling method to choose specific kebeles, and simple random sampling to select households within each kebele. A structured survey was administered to 343 randomly selected households, capturing detailed information on socioeconomic and demographic characteristics, access to credit and extension services, social networks, technological literacy, and welfare outcomes. Trained enumerators conducted face-to-face interviews to ensure data accuracy. Ethical considerations included obtaining oral permission from local authorities and community leaders, ensuring adherence to ethical guidelines and prioritizing participants’ rights and welfare.
3.4 Variable definition and hypothesis
In our investigation into the adoption of digital technology among farm households in South and Central Ethiopia, we recognized a multitude of influential variables. Alongside education and farm size, access to extension services, credit, social networks, information, and market information emerged as crucial factors shaping adoption decisions (Sikundla et al., 2018). Notably, access to extension services offered valuable insights and training on digital tools, potentially swaying adoption choices (Gebrehiwot and van der Veen, 2021). Similarly, access to credit facilitated technology investment, especially among financially constrained households (Ouma et al., 2017). Moreover, social networks and technological literacy played pivotal roles, with information dissemination and effective utilization of digital tools influencing adoption behaviors within communities (Jaeger et al., 2012). Considering these alongside demographic factors like age and sex, which also impacted technology adoption patterns, ensured a holistic analysis of adoption dynamics (Rojas-Méndez et al., 2015).
Furthermore, the availability and accessibility of ICTs significantly contributed to adoption tendencies. Households with greater access to smartphones and internet connectivity were more likely to adopt digital agricultural tools, driven by familiarity and reliance on digital devices (Michels et al., 2020). Additionally, the perceived benefits and barriers associated with technology adoption, alongside the level of innovativeness within households, warranted attention in understanding adoption behaviors (Neumeyer et al., 2021). By incorporating these variables into our analysis, we aimed to provide a comprehensive understanding of the multifaceted determinants influencing technology adoption among farm households in Ethiopia, contributing to the existing body of knowledge on rural development and technology adoption. All variables used in the study were defined in Appendix I.
The endogenous switching regression (ESR) model was employed to estimate the impact of various factors on welfare outcomes. To ensure consistent, unbiased, and valid results, checks for multicollinearity, heteroscedasticity, and omitted variables were conducted (see Appendixes I, II, and IV respectively). The variance inflation factor (VIF) values were all below 10, indicating no significant multicollinearity issues, with a mean VIF of 1.532. Breusch-Pagan/Cook-Weisberg tests for heteroscedasticity indicated significant heteroscedasticity (Prob > chi2 = 0.0000 for Yield/ha and Prob > chi2 = 0.0005 for Total income), suggesting the need for robust standard errors. The Ramsey RESET test indicated omitted variable problems (Prob > F = 0.0000 for both Total income and Yield/ha), suggesting that additional variables might be necessary. Detailed results are available upon request, providing a comprehensive overview of the statistical assessments conducted to ensure the robustness of the ESR model’s outcomes.
3.5 Model specification
The study aimed to understand the impact of technology adoption on the yield of smallholder ginger farmers. The Endogenous Switching Regression (ESR) model consisted of two stages. The first stage employed a probit model to assess the likelihood of smallholder ginger farmers adopting digital technology. This stage aimed to determine the probability of technology adoption based on various predictor variables, considering socio-economic and demographic characteristics. The first stage employed a probit model to assess the likelihood of smallholder ginger farmers adopting digital technology (Equation 3).
Where, is the cumulative distribution function of the standard normal distribution, represents a vector of exogenous variables affecting technology adoption, and is a vector of coefficients to be estimated.
The use of the Endogenous Switching Regression (ESR) model is appropriate because it specifically addresses both endogeneity and selection bias. Endogeneity refers to the potential correlation between the independent variables and the error terms, while selection bias arises from non-random assignment of units to treatment and control groups. The ESR model handles these issues by modeling the decision process of technology adoption and estimating the corresponding outcomes for adopters and non-adopters.
The Full Information Maximum Likelihood (FIML) method is an estimation technique employed to jointly estimate the parameters of both the selection equation and the outcome equations in the Endogenous Switching Regression (ESR) model. FIML is particularly useful when dealing with potential endogeneity and selection bias, as it allows for the simultaneous estimation of the entire system of equations, which helps to account for the correlation between the unobserved factors influencing both adoption decisions and outcomes (such as productivity or income).
In this study, FIML was used in the second stage to estimate the outcomes of digital tool adopters and non-adopters. By modeling the decision to adopt digital tools in the first stage (using a probit selection equation) and estimating the corresponding outcome equations in the second stage, FIML provides more efficient and consistent estimates compared to limited information methods like Two-Stage Least Squares (2SLS). Additionally, FIML is able to handle missing data more effectively, ensuring that all available information is used in the estimation process.
Once farmers were classified into treatment groups (adopters) and control groups (non-adopters) based on their predicted probabilities of adoption, the second stage estimated the outcome equation for each group (Equations 4, 5) separately:
For the treatment group :
For the control group ( ):
Where and denote potential outcomes for the adopters and non-adopters, respectively; represents a vector of exogenous variables influencing the yield; and are vectors of coefficients to be estimated; and and are the error terms assumed to be normally distributed.
To account for potential endogeneity in the technology adoption equation, instrumental variables were introduced. These instrumental variables, such as age and extension contact, were correlated with the endogenous explanatory variable (technology adoption) but not with the error term in the outcome equation, thereby isolating exogenous variability in the technology adoption decisions were used as instruments in this study. The validity of the instruments was assessed using the Sargan test (see Appendix III), which evaluates whether the instruments are uncorrelated with the error term and correctly excluded from the outcome equation (Kertesz, 2017). This approach ensures that the instruments are valid and that the estimated effects of technology adoption on yield are not biased by endogeneity issues (Wossen et al., 2019).
Understanding the treatment effect is crucial in research and policy-making to evaluate the impact of interventions and assess their outcomes. This study focused on the Average Treatment Effect on the Treated (ATT), a key measure calculated by examining the conditional expectations of individuals who have adopted digital technology.
Let be a binary variable indicating whether each unit adopted the technology or not, where for adopters and for non-adopters. The average welfare for adopters, that is, the average value of the welfare outcomes (e.g., income, yield, and food security) for units that adopted the technology, was calculated as follows (Equation 6):
Where is the number of adopters, Yi is the welfare outcomes for unit i, and is an indicator function that equals 1 if (units an adopter) and 0 otherwise.
The average welfare for non-adopters, that is, the average value of the welfare outcomes for units that did not adopt the technology, was calculated as follows (Equation 7):
Where is the number of non-adopters, Yi is the welfare outcomes for unit i, and is an indicator function that equals 1 if (units non-adopter) and 0 otherwise.
Therefore, the average treatment effect (ATE) was computed by taking the difference between the average welfare for the adopters and the average welfare for the non-adopters (Equation 8):
The ATE represents the average change in welfare outcomes due to the adoption of digital technology. If the adoption had a positive effect, the ATE would be positive, indicating an improvement in welfare outcomes. Conversely, if the adoption had a negative effect, the ATE would be negative.
4 Results and discussion
4.1 Socio-economic and demographic characteristics of households in adoption of digital technology categories
Household socio-economic and demographic traits exhibited notable distinctions between adopters and non-adopters of digital technology (Table 2). Equipped households were younger (41.17 vs. 43.90 years), with larger farms (1.43 vs. 0.78 hectares) and more livestock (6.45 vs. 3.24 TLU), all significantly different (p < 0.001). They also enjoyed more extension services (3.32 vs. 1.90). Family size, however, showed no significant difference. These findings underscored the link between digital technology adoption and enhanced agricultural resources and support.
In the analysis of dummy variables, significant associations between digital technology adoption and various factors were evident. Gender exhibited no statistically significant difference (p = 0.1485), suggesting equitable access among male and female household heads. Conversely, education level strongly correlated with adoption (p < 0.000), indicating higher education linked to increased digital technology adoption. Moreover, access to information on ginger production, ICT availability, social institution membership, and market information significantly predicted digital technology adoption (all p < 0.000). Additionally, access to credit and participation in digital technology training were positively associated with adoption (both p < 0.000). These findings underscored the pivotal role of education, information access, social networks, and financial resources in fostering digital technology adoption among smallholder farmers.
4.2 Types of digital extension service used by farmers
The bar chart in Figure 3 below, illustrates the types of digital extension services utilized by farmers. The most frequently used tool is phone calls, which account for 65.79% of the total usage. Text messages are the second most common method, used by 22.81% of the farmers. A smaller proportion, 11.40%, access extension services through YouTube or Google. These figures suggest that while traditional communication methods like phone calls dominate, there is still a notable use of other digital tools among farmers, reflecting a diverse approach to accessing agricultural information.
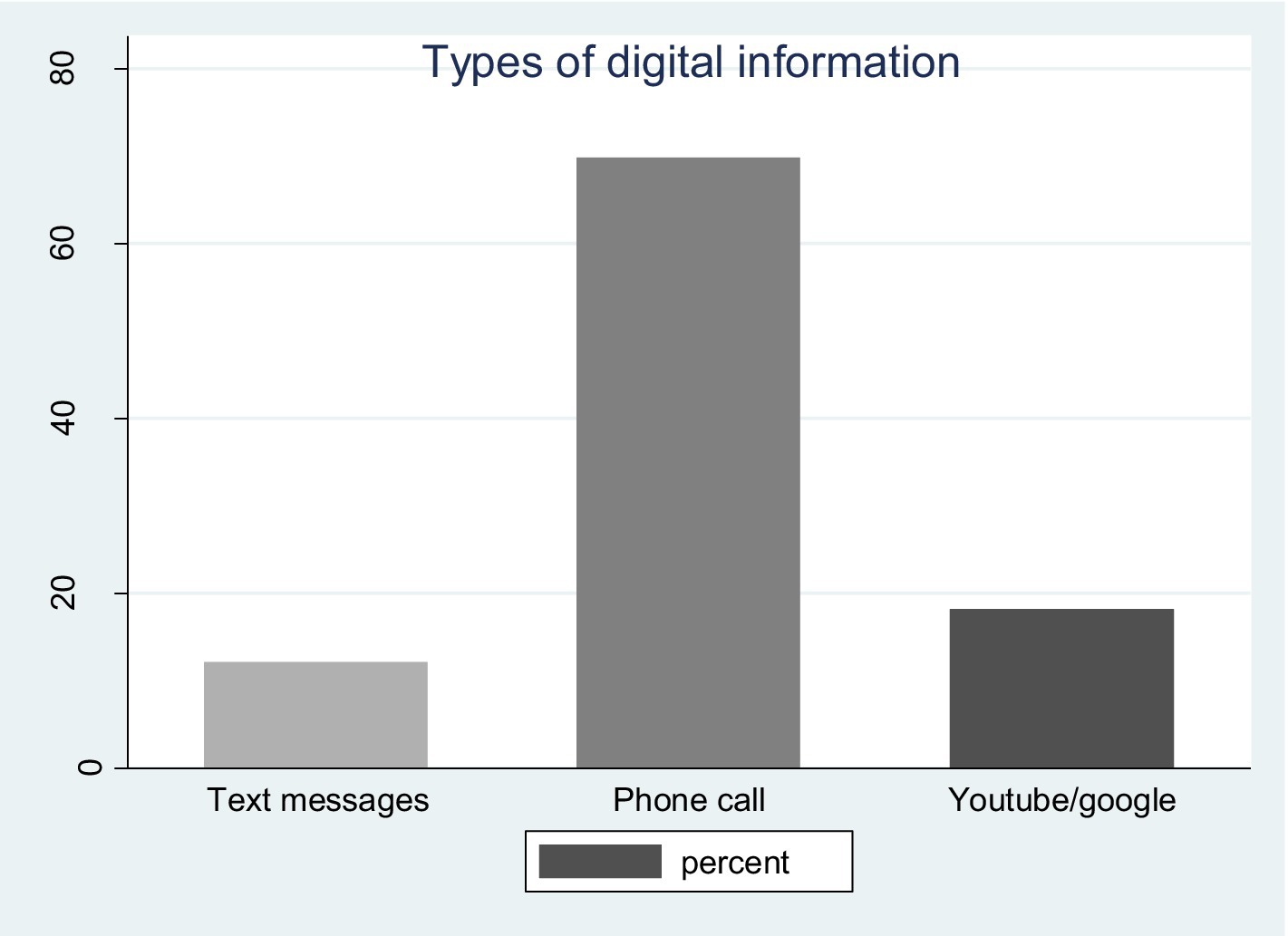
Figure 3. Types of digital extension tool used. Source: Authors’ computation from survey data, 2023.
4.3 Mean difference in welfare indicators between adopters and non-adopters of digital extension tools
The t-tests for total annual income and yield per hectare revealed significant differences between adopters and non-adopters of digital extension tools (Table 3). Adopters had a higher mean annual income (65,531.69 vs. 51,726.99) and yield per hectare (150.8604 vs. 125.7319), both differences statistically significant (p = 0.0000). These findings underscore the positive impact of digital extension tools on income and productivity.
4.4 Thematic results for focus group discussions (FGD) and key informant interviews (KII)
The study on the role of digital tools in agricultural extension among smallholder ginger producers was conducted in Boloso-Bombe district (Adila Kebele) and Hadaro-Tunto district (Ajora Kebele), Southern and Central Ethiopia, respectively. A total of 2FGDs were held in these areas which comprises eight households, and the Key Informant Interviews (KII) involved discussions with district offices of concerned bodies. The following themes emerged.
4.4.1 Awareness and perception of digital tools
From the FGDs, it became evident that many farmers in both districts had limited awareness of available digital tools for agricultural purposes. While younger and more educated farmers were somewhat aware of mobile-based applications that provide weather forecasts or market price information, most participants had not been exposed to these tools or were unaware of their potential to improve farming practices. This highlights a critical gap in outreach and education regarding digital agricultural solutions. In contrast, key informants from district offices acknowledged that awareness campaigns regarding the benefits of digital tools were insufficient, emphasizing the need for tailored communication strategies to increase digital tool adoption among smallholder farmers.
4.4.2 Benefits and challenges of adoption
Farmers who had adopted digital tools reported improved decision-making due to better access to market prices and weather forecasts, resulting in more efficient farming operations. However, the challenges of poor internet connectivity and the high cost of smartphones were frequently mentioned barriers. Non-adopters, particularly in remote areas like Ajora and Adila Kebeles, viewed these tools as impractical and unaffordable, reinforcing the need for infrastructural improvements. Key informants corroborated these challenges, suggesting that inadequate digital infrastructure and high costs are significant barriers to adoption. They called for increased investment in rural connectivity and more affordable digital technologies to make them accessible to smallholder farmers.
4.4.3 Socio-economic and institutional factors
The FGDs revealed that socio-economic factors, such as age, education, and income level, played a significant role in digital tool adoption. Younger farmers and those with higher education levels were more likely to use digital tools, while older, less educated farmers were more resistant. Additionally, wealthier households, especially those with diversified income sources, were more inclined to adopt digital technologies. Key informants from district offices confirmed these socio-economic disparities, stressing the need for targeted interventions that consider the socio-economic backgrounds of farmers. The lack of institutional support was also a recurring theme, with both farmers and district officials noting that agricultural extension workers did not consistently promote digital tools or provide necessary training.
4.4.4 Cultural and behavioral barriers
Cultural resistance to technology adoption, particularly among older farmers, emerged as a significant barrier. FGDs participants, especially in Adila Kebele, expressed skepticism about the reliability of digital tools, preferring traditional farming practices. Key informants recommended involving community leaders and trusted figures in training programs to help bridge this cultural gap, suggesting that demonstrations of the practical benefits of digital tools could foster greater acceptance among conservative farmers.
4.4.5 Policy implications and recommendations
Both FGDs and KIIs indicated that farmers and local officials believed policy interventions were needed to overcome the barriers to digital tool adoption. Farmers suggested that government initiatives should focus on reducing the costs of digital tools and improving network coverage. They also emphasized the need for frequent training programs tailored to specific agricultural practices, such as ginger farming. District officials echoed these concerns, urging policymakers to prioritize investments in digital infrastructure and develop more farmer-friendly digital extension programs. Additionally, they recommended providing financial incentives, such as subsidies or low-interest loans, to support smallholder farmers in adopting digital technologies.
4.5 Results from the endogenous switching regression model
4.5.1 First stage (probit) result of endogenous switching regression
The probit model estimated the determinants of adoption of digital extension tools in Table 4, showed that several factors significantly influence this access. Information access, ICTs, family size, and extension contact positively affect the likelihood of adopting digital tools, whereas age has a negative impact. This suggests that individuals with better information access, more extensive use of ICTs, larger families, and more contact with extension services are more likely to adopt digital tools. Recent empirical studies support these findings. For instance, Kamal and Bablu (2023) indicated that improved information access significantly boosts digital tool adoption among farmers by enhancing their knowledge and decision-making abilities. Additionally, Van Campenhout et al. (2021) found that ICTs like mobile phones substantially enhance agricultural productivity and access to markets, thereby encouraging digital tool adoption. These determinants highlight the critical role of information and communication technologies and support services in promoting digital tool usage.
The economic significance of these results underscores the importance of policy interventions aimed at enhancing information access and digital infrastructure in rural areas. By targeting extension services and providing support for ICT adoption, governments and development agencies can significantly improve farmers’ ability to adopt digital tools, leading to better productivity and welfare outcomes.
4.5.2 Second stage (FIML) estimates from the ESR for welfare outcomes
The second stage employed Full Information Maximum Likelihood (FIML) to estimate the impact of digital tool adoption on welfare outcomes, specifically yield and total annual income (Table 4). The results revealed significant differences between adopters and non-adopters of digital tool access. The likelihood ratio test for joint independence confirms that the endogenous switching model effectively controls for self-selection and inherent differences between groups. This aligns with (Lokshin and Sajaia, 2004), who emphasized the necessity of accounting for selection bias in evaluating program impacts. The error correlation coefficients alternate in signs (ρ1 = 0.519, ρ2 = −0.884), indicating differing unobserved factors affecting yield and income equations.
In terms of economic significance, the results show a meaningful impact on both yield and income, which are critical measures of household welfare. Adopters of digital tools experienced a significant income increase, while non-adopters, if they were to adopt, would see similar benefits. The model shows that the average treatment effect (ATT) on income for adopters was an increase of 4,495.58 units, suggesting that digital tools substantially enhance financial wellbeing. This translates into better livelihood outcomes and potential improvements in household economic stability, especially for smallholder farmers in rural areas.
For yield, the results indicate that adopters have a slightly lower yield by 6.086 units compared to their hypothetical yield without adoption, suggesting that there may be external factors limiting the full realization of yield gains. However, non-adopters would see a yield increase of 6.086 units if they adopted digital tools, underscoring the positive potential of these tools to improve productivity. These findings align with the notion that adoption of digital tools requires complementary inputs and support, such as better access to markets or inputs, to fully capitalize on the technology’s benefits.
The heterogeneous effects (TH) for yield and income emphasize the digital tool’s overall positive impact on household welfare, particularly in terms of income. The total yield effect (TH) was 12.172 units, and the total income effect (TH) was 8,991.16 units, which are economically meaningful figures, demonstrating that digital extension tools not only contribute to higher productivity but also lead to significant improvements in household income.
Gender played a significant role in agricultural productivity, particularly in the adoption of digital tools. Female farmers, when provided with equal access to resources and technologies, demonstrated higher yields in the presence of digital tools. This observation aligned with (Doss and Quisumbing, 2020), who delved into gender dynamics in agricultural productivity in Sub-Saharan Africa. This highlights the importance of addressing gender disparities in technology access to maximize the economic benefits for all households.
Another critical factor that influenced productivity was farm size. Larger farms were associated with higher yields, indicative of the economies of scale and better resource allocation at play. Van Campenhout et al. (2021) in South Africa supported this notion, emphasizing the efficiency gains and improved access to inputs and markets that larger farms enjoyed, ultimately leading to higher overall productivity.
Access to information emerged as a vital catalyst for yield enhancement. Kumar and Ali (2011) in India demonstrated the transformative impact of information access on farming practices and technology adoption, resulting in substantial yield improvements. This highlighted the indispensable role of knowledge in driving agricultural productivity.
In terms of income outcomes, gender continued to exert a significant influence, particularly for female farmers leveraging digital tools. Bansal et al. (2021) highlighted the income gains experienced by women in agriculture through the adoption of digital technologies, enhancing their economic empowerment and household welfare in India.
Educational attainment also played a pivotal role in income generation, with educated farmers better positioned to leverage digital tools for economic benefits. Amudavi and Obura (2017) in Kenya underscored this relationship, showcasing how education enabled farmers to adopt and benefit from new technologies more effectively. Family size positively correlated with income, attributed to the availability of more labor, particularly in smallholder farming systems. Asfaw et al. (2012) in Ethiopia highlighted the contributions of larger families to farming activities, enhancing productivity and income levels.
Similarly, larger farm sizes significantly boosted income, reflecting the benefits of scale. Ogundari and Aklnbogun (2010) in Nigeria emphasized the higher income potential of larger farm operations due to increased production capacity and improved market access.
Ownership of livestock emerged as another income-enhancing factor, providing diversified income sources and improving food security. Barrett et al. (2008) in Kenya underscored the significant economic benefits derived from livestock ownership among rural households. Access to information continued to play a pivotal role in income generation, reaffirming the criticality of knowledge in economic activities. Aker et al. (2011) in Niger highlighted the positive impact of information access on economic outcomes in agriculture, enabling farmers to make better selling decisions and ultimately increasing their income.
Lastly, access to market information significantly boosted income by enhancing farmers’ bargaining power and enabling them to time their sales better for higher prices. Dillon and Dambro (2017) in Madagascar illustrated the transformative effects of market information on farmers’ income levels, emphasizing its importance in improving sales and profitability.
4.5.3 Economic implications of the ESR results
The economic significance of the results was crucial for policymakers, development agencies, and rural development programs. The adoption of digital tools presented an opportunity for significant improvements in household income and food security, particularly in rural areas where agricultural productivities closely linked to welfare outcomes. However, the trade-offs observed in yield outcomes suggested that adoption alone is insufficient; farmers need complementary support such as access to better inputs, training, and market information to fully benefit from digital tools.
These findings supported the development of integrated rural development programs that focus on improving information access, ICT infrastructure, and extension services, especially for female farmers and smallholder farms. Such programs could significantly contribute to enhancing the welfare of farming households and improving overall economic stability in rural areas. The potential for digital tools to close the productivity gap and increase income underscores their relevance in meeting Sustainable Development Goals (SDGs), particularly those related to poverty reduction, food security, and gender equality.
Table 5 below, presented the expected outcomes for both yield and income, comparing the actual and counterfactual scenarios, and highlights the average treatment effects (ATE) for those adopters and non-adopters of digital tools. The result was obtained by following the conditional expectations derived from the estimation of the switching model presented above.
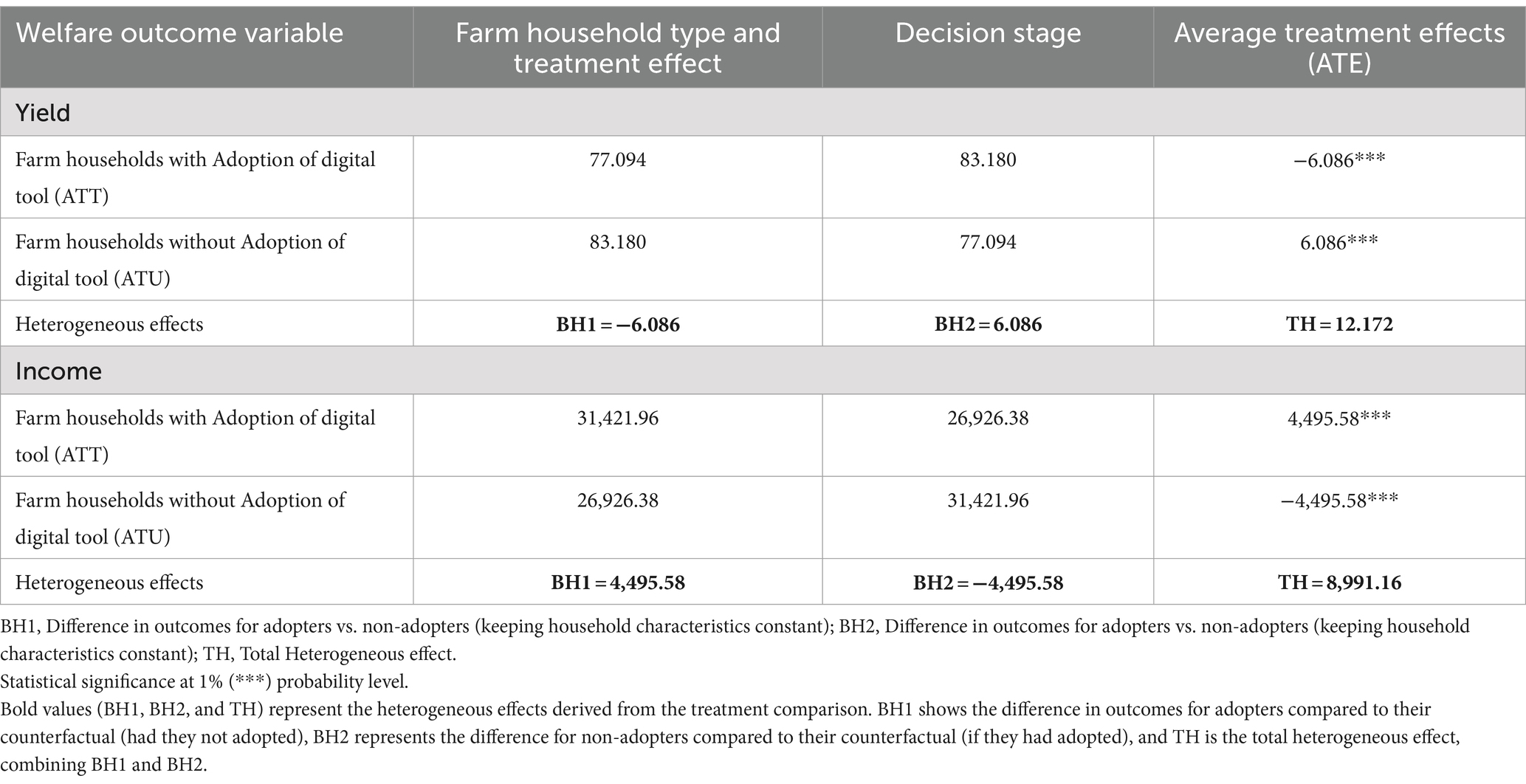
Table 5. ESR based average treatment effects of digital extension tool on welfare outcome variables.
The analysis of the ESR-based average treatment effects (ATE) of the digital extension tool on welfare outcome variables revealed significant impacts on both yield and income for smallholder ginger farmers. For adopters, the yield was slightly lower by 6.086 units compared to their hypothetical yield without adoption, indicating a potential trade-off or external factors influencing yield despite digital adoption. Conversely, non-adopters would see a yield increase of 6.086 units if they adopted the tool, underscoring its positive potential in enhancing yield. Regarding income, adopters experienced a substantial increase of 4,495.58 units, highlighting the digital tool’s efficacy in improving financial outcomes. In contrast, non-adopters would face a decrease of the same amount if they adopted the tool, suggesting differences in how households utilize the tool or other unobserved factors. The heterogeneous effects showed a total yield effect (TH) of 12.172 units and a total income effect (TH) of 8,991.16 units, emphasizing the digital tool’s overall positive impact on household welfare, particularly in terms of income, which could lead to better livelihood outcomes and economic stability for the farmers.
5 Conclusion and policy implication
5.1 Conclusion
This study illuminated the critical role digital tools play in agricultural extension, particularly among smallholder ginger producers in Southern and Central Ethiopia. Despite their potential, the adoption of these tools remained low due to weak digital infrastructure and limited access to information. By investigating the determinants of adoption and their impact on welfare outcomes, this research addressed key gaps in the literature. Using a mixed-methods approach, the study identified socio-economic, institutional, and technological factors shaping adoption decisions, providing valuable insights for policymakers.
Grounded in utility maximization theory, the research offered a nuanced understanding of adoption dynamics, taking into account individual and social influences. The study employed a robust multistage sampling approach, involving 343 randomly selected households, to assess the factors influencing digital technology adoption. Through the use of an Endogenous Switching Regression (ESR) model, the research demonstrated the significant positive impact of digital tool adoption on welfare outcomes, notably improving agricultural productivity and income.
The econometric analysis provided strong evidence that access to digital tools substantially benefited smallholder farmers. Factors such as access to information, ICT usage, family size, and agricultural extension services significantly influenced the likelihood of adoption. Moreover, the analysis revealed considerable differences in yield and income between households that adopted digital tools and those that did not, highlighting the transformative potential of technology adoption in enhancing agricultural productivity and financial wellbeing.
These findings carried important implications for policymakers and development practitioners, emphasizing the need for targeted interventions to promote digital technology adoption. By leveraging digital tools, policymakers could foster sustainable agricultural development, enhance income generation, and contribute to the economic stability of smallholder farmers in Ethiopia.
5.2 Recommendations
To enhance the adoption of digital technology and improve agricultural productivity among smallholder ginger producers in Ethiopia, several critical steps were recommended. First, the government needed to prioritize investments in strengthening digital infrastructure, particularly in rural areas where access remained limited. Successful models, such as Kenya’s M-Farm, which connects farmers to market prices and digital extension services through mobile platforms, provided valuable lessons for addressing infrastructure challenges.
In addition to infrastructure, expanding access to information and communication technologies (ICTs) was crucial. Broadening mobile platform and internet accessibility would empower farmers by improving their decision-making processes. India’s e-Choupal initiative, which provided agricultural information and market data through internet kiosks, served as a practical example for enhancing digital access and fostering adoption in Ethiopia.
Moreover, agricultural extension services should have been redesigned to focus on digital literacy and the effective use of digital tools. Uganda’s Grameen Foundation FarmerLink, which provided agricultural advice directly to farmers via mobile phones, illustrated how such services could improve farmers’ capacity to adopt new technologies and enhance their productivity.
Improving access to credit was also vital, as it directly influenced smallholder farmers’ ability to invest in digital tools. Bangladesh’s BRAC program, which offered financial products tailored to agricultural technology investments, demonstrated how providing affordable financial services could encourage technology adoption. Similarly, expanding microfinance and other financial services to smallholder farmers in Ethiopia would have accelerated the adoption process.
Lastly, public-private partnerships played a key role in scaling up digital interventions. Collaborative efforts between the government, private sector, and NGOs, similar to Ghana’s Esoko platform, which delivered real-time market information via mobile phones, could have facilitated wider adoption of digital tools in Ethiopia. Learning from successful interventions in countries like Kenya, Uganda, and Ghana offered valuable insights for developing localized solutions to overcome barriers to adoption and promote sustainable agricultural development in the region.
Data availability statement
The raw data supporting the conclusions of this article will be made available by the authors without undue reservation.
Ethics statement
Ethical review and approval was not required for the study on human participants in accordance with the local legislation and institutional requirements. Oral informed consent from the [patients/participants OR patients/participants legal guardian/next of kin] was obtained to participate in this study in accordance with the national legislation and the institutional requirements.
Author contributions
FG: Writing – review & editing, Writing – original draft. BK: Writing – review & editing. AT: Writing – review & editing. AL: Writing – review & editing.
Funding
The author(s) declare that no financial support was received for the research, authorship, and/or publication of this article.
Acknowledgments
The authors extend their gratitude to the study participants for their cooperation and valuable contributions.
Conflict of interest
The authors declare that the research was conducted in the absence of any commercial or financial relationships that could be construed as a potential conflict of interest.
Publisher’s note
All claims expressed in this article are solely those of the authors and do not necessarily represent those of their affiliated organizations, or those of the publisher, the editors and the reviewers. Any product that may be evaluated in this article, or claim that may be made by its manufacturer, is not guaranteed or endorsed by the publisher.
Supplementary material
The Supplementary material for this article can be found online at: https://www.frontiersin.org/articles/10.3389/fsufs.2024.1443775/full#supplementary-material
References
Abdulai, A. R., Kc, K. B., and Fraser, E. (2023). What factors influence the likelihood of rural farmer participation in digital agricultural services? Experience from smallholder digitalization in northern Ghana. Outlook Agric. 52, 57–66. doi: 10.1177/00307270221144641
Abebe, A., and Mammo Cherinet, Y. (2019). Factors affecting the use of information and communication technologies for cereal marketing in Ethiopia. J. Agric. Food Inf. 20, 59–70. doi: 10.1080/10496505.2018.1438290
Agnihotri, A., Madon, T., and Gadgil, A. J. (2023). “Introduction to development engineering” in Introduction to development engineering. Cham, Switzerland: Springer. doi: 10.1007/978-3-030-86065-3_1
Aker, J., Clemens, M., and Ksoll, C. (2011). Mobiles and mobility: the effect of mobile phones on migration in Niger. Proceedings on the German development economics conference, 2, 22.
Amudavi, D. M., and Obura, R. K. (2017). Determinants of choice of agricultural information sources and pathways among sorgum farmers in Ndhiwa Sub-County, Western Kenya. Int. J. Agr. Ext. 5, 5–6.
Asfaw, S., Lipper, L., Dalton, T. J., and Audi, P. (2012). Market participation, on-farm crop diversity and household welfare: micro-evidence from Kenya. Environ. Dev. Econ. 17, 579–601. doi: 10.1017/S1355770X12000277
Awunyo-Vitor, D. (2018). Theoretical and conceptual framework of access to financial services by farmers in emerging economies: implication for empirical analysis. Acta Univ. Sapientiae Econ. Bus. 6, 43–59. doi: 10.1515/auseb-2018-0003
Bansal, V., Das, L., Joshi, V., and Meena, S. C. (2021). Attitude & perceived effects of ICT tools used by farm women attitude & perceived effects of ICT tools used by farm women. Turkish Online J. Qual. Inq. (TOJQI) 12, 4632–4643.
Barrett, C. B., Carter, M. R., and Ikegami, M. (2008). Poverty Traps and Social ProtectionWorking Paper No. 0804, University of Wisconsin-Madison, Department of Agricultural and Applied Economics.
Bidin, A. (2017). “Опыт аудита обеспечения качества и безопасности медицинской деятельности в медицинской организации по разделу «Эпидемиологическая безопасностьNo Title” in Вестник Росздравнадзора, vol. 4.
Bolfe, É. L., Jorge, L. A. d. C., Sanches, I. D., Júnior, A. L., Costa, C. C. d., Victoria, D. d. C., et al. (2020). Precision and digital agriculture: adoption of technologies and perception of Brazilian farmers. Agriculture (Switzerland) 10, 1–16. doi: 10.3390/agriculture10120653
Daniso, B., Muche, M., Fikadu, B., Melaku, E., and Lemma, T. (2020). Assessment of rural households’ mobile phone usage status for rural innovation services in Gomma Woreda, Southwest Ethiopia. Cogent Food Agric. 6:1728083. doi: 10.1080/23311932.2020.1728083
Davis, F. D. (1987). User acceptance of information systems: the technology acceptance model (TAM) (Doctoral dissertation, Sloan School of Management, Massachusetts Institute of Technology).
Dillon, B., and Dambro, C. (2017). How competitive are crop markets in sub-Saharan Africa? Am. J. Agric. Econ. 99, 1344–1361. doi: 10.1093/ajae/aax044
Doss, C. R., and Quisumbing, A. R. (2020). Understanding rural household behavior: beyond Boserup and Becker. Agric. Econ. (United Kingdom) 51, 47–58. doi: 10.1111/agec.12540
Freeman, L. C., White, D. R., and Romney, A. K. (1992). Methods in social network. Vancouver, Canada: Sociological Inquiry.
Gebrehiwot, T., and van der Veen, A. (2021). Farmers’ drought experience, risk perceptions, and behavioural intentions for adaptation: evidence from Ethiopia. Clim. Dev. 13, 493–502. doi: 10.1080/17565529.2020.1806776
Haile, M. G., Wossen, T., and Kalkuhl, M. (2019). Access to information, price expectations and welfare: the role of mobile phone adoption in Ethiopia. Technol. Forecast. Soc. Chang. 145, 82–92. doi: 10.1016/j.techfore.2019.04.017
Jaeger, P. T., Bertot, J. C., Thompson, K. M., Katz, S. M., and DeCoster, E. J. (2012). Digital divides, digital literacy, digital inclusion, and public libraries. Public Libr. Q. 31, 1–20. doi: 10.1080/01616846.2012.654728
Kamal, M., and Bablu, A. (2023). Mobile applications empowering smallholder farmers: an analysis of the impact on agricultural development. Int. J. Soc. Anal. 8, 36–52.
Kertesz, B. (2017). Discrete response models. Adv. Stud. Theor. Appl. Economet. 50, 163–194. doi: 10.1007/978-3-319-60783-2_6
Kifile, A., Bekele, A., Tefera, T., Alemu, D., Koomen, I., and Diro, M. (2023). Importance of ginger in Ethiopia: recent trends and challenges, vol. 1 doi: 10.18174/635326
Krell, N. T., Giroux, S. A., Guido, Z., Hannah, C., Lopus, S. E., Caylor, K. K., et al. (2021). Smallholder farmers’ use of mobile phone services in Central Kenya. Clim. Dev. 13, 215–227. doi: 10.1080/17565529.2020.1748847
Kropff, W., Ortiz-crespo, B., Tesfaye, L., Alemayehu, S., Ramirez-Villegas, J., Ghosh, A., et al. (2023). Mainstreaming Digital Approaches for Adaptation in Agriculture in Kenya. International Development Research Centre (IDRC).
Kumar, S., and Ali, J. (2011). Analyzing the factors affecting consumer awareness on organic foods in India. 21st annual IFAMA world forum and symposium on the road to 2050: sustainability as a business opportunity, February, 1–12, Frankfurt, Germany
Lokshin, M., and Sajaia, Z. (2004). Maximum likelihood estimation of endogenous switching regression models. Stata J. 4, 282–289. doi: 10.1177/1536867X0400400306
Michels, M., Fecke, W., Feil, J. H., Musshoff, O., Pigisch, J., and Krone, S. (2020). Smartphone adoption and use in agriculture: empirical evidence from Germany. Precis. Agric. 21, 403–425. doi: 10.1007/s11119-019-09675-5
Neumeyer, X., Santos, S. C., and Morris, M. H. (2021). Overcoming barriers to technology adoption when fostering entrepreneurship among the poor: the role of technology and digital literacy. IEEE Trans. Eng. Manag. 68, 1605–1618. doi: 10.1109/TEM.2020.2989740
Ogundari, K., and Aklnbogun, O. O. (2010). Modeling technical efficiency with production risk: a study of fish farms in Nigeria. Mar. Resour. Econ. 25, 295–308. doi: 10.5950/0738-1360-25.3.295
Ouma, S. A., Odongo, T. M., and Were, M. (2017). Mobile financial services and financial inclusion: is it a boon for savings mobilization? Rev. Dev. Finance 7, 29–35. doi: 10.1016/j.rdf.2017.01.001
Oyinbo, O., Chamberlin, J., and Maertens, M. (2020). Design of digital agricultural extension tools: perspectives from extension agents in Nigeria. J. Agric. Econ. 71, 798–815. doi: 10.1111/1477-9552.12371
Prameela, T. P., and Suseela Bhai, R. (2020). Bacterial wilt of ginger (Zingiber officinale Rosc.) incited by Ralstonia pseudosolanacearum - a review based on pathogen diversity, diagnostics and management. J. Plant Pathol. 102, 709–719. doi: 10.1007/s42161-020-00487-5
Rogers, E. M. (1983). “Diffusion of innovations: attributes of innovations and their rate of adoption” in The tree press, vol. 49. 4th ed, 204–251.
Rojas-Méndez, J. I., Parasuraman, A., and Papadopoulos, N. (2015). “Consumers’ technology readiness in a developing country: the role of demographics and attitudes” in Developments in marketing science: proceedings of the academy of marketing science, vol. 2015 (Cham: Springer International Publishing), 2.
Rosen, S., Horinek, K., Odeh, A., Servi, L., and Tolk, A. (2019). Foundations for a game theoretic framework for agile acquisition. Defense Acquisit. Res. J. 26, 250–289. doi: 10.22594/dau.18-812.26.03
Sikundla, T., Mushunje, A., and Akinyemi, B. E. (2018). Socioeconomic drivers of mobile phone adoption for marketing among smallholder irrigation farmers in South Africa. Cogent Soc. Sci. 4, 1–12. doi: 10.1080/23311886.2018.1505415
Takahashi, K., Muraoka, R., and Otsuka, K. (2020). Technology adoption, impact, and extension in developing countries’ agriculture: a review of the recent literature. Agric. Econ. (United Kingdom) 51, 31–45. doi: 10.1111/agec.12539
Thakur, D., Kumar, Y., Kumar, A., and Singh, P. K. (2019). “Applicability of wireless sensor networks in precision agriculture: a review” in Wireless personal communications, vol. 107 (Cham: Springer). doi: 10.1007/s11277-019-06285-2
Tilore, F. G., Shano, B. K., Shirko, A. T., and Hawitibo, A. L. (2024). Effect of credit constraint on yield: the case of ginger producers in southern and central Ethiopia. Front. Sustain. Food Syst. 8:1334799. doi: 10.3389/fsufs.2024.1334799
Van Campenhout, B., Spielman, D. J., and Lecoutere, E. (2021). Information and communication technologies to provide agricultural advice to smallholder farmers: experimental evidence from Uganda. Am. J. Agric. Econ. 103, 317–337. doi: 10.1002/ajae.12089
Keywords: digital technology adoption, ginger farming, welfare, South Ethiopia, Central Ethiopia
Citation: Gizachew F, Kuma B, Tafesse A and Lambamo A (2024) Digital tools and welfare: adoption determinants among ginger producers in Southern and Central Ethiopia. Front. Sustain. Food Syst. 8:1443775. doi: 10.3389/fsufs.2024.1443775
Edited by:
Isabelle Piot-Lepetit, INRAE Occitanie Montpellier, FranceReviewed by:
Anup Pradhan, University of Johannesburg, South AfricaShemei Zhang, Sichuan Agricultural University, China
Copyright © 2024 Gizachew, Kuma, Tafesse and Lambamo. This is an open-access article distributed under the terms of the Creative Commons Attribution License (CC BY). The use, distribution or reproduction in other forums is permitted, provided the original author(s) and the copyright owner(s) are credited and that the original publication in this journal is cited, in accordance with accepted academic practice. No use, distribution or reproduction is permitted which does not comply with these terms.
*Correspondence: Fetagn Gizachew, ZmV0YWdudGlsb3JlQGdtYWlsLmNvbQ==; ZmV0YWduLmdpemFjaGV3QHdzdS5lZHUuZXQ=