- 1Wageningen University & Research, Animal Breeding and Genomics, Wageningen, Netherlands
- 2International Livestock Research Institute (ILRI), Addis Ababa, Ethiopia
- 3The Roslin Institute and Royal (Dick) School of Veterinary Studies, Division of Quantitative Biology, University of Edinburgh, Easter Bush, Edinburgh, United Kingdom
- 4School of Life Sciences, University of Nottingham, Nottingham, United Kingdom
- 5AgResearch, Invermay Agricultural Centre, Mosgiel, New Zealand
Smallholder chicken production system is dominant in tropical developing countries and it contributes significantly to the livelihoods of farmers. Performance of flocks is often too low to meet growing demands for meat and eggs. Unavailability of productive and adaptive breeds that match suitably with the environment is a major limitation. Breeds developed for low- or medium-input systems elsewhere can be evaluated for their performances and introduced at scale to enhance productivity and improve socioeconomic outcomes. Such genetic interventions require conducting multi-environment performance analysis (MEPA) of candidate breeds. However, analytical frameworks and methods are not readily available to identify the best performing breeds considering agroecological differences. Methods used in plant breeding to predict productivity and yield stability of genotypes across environments are theoretically applicable to smallholder livestock systems. In the present study, we adapted two modeling approaches of MEPA to evaluate growth performance of chicken breeds across different agroecologies in Ethiopia. Contrary to the conventional classification system that relies on the types of plants grown and other agronomic variables to delineate agroecological classes, we utilized classes defined by Species Distribution Models (SDMs). SDM defined agroecologies take into account the most relevant environmental predictors that influence suitability of habitats for a livestock species and are ideal for breed performance evaluations. Additive main effects multiplicative interaction model (AMMI) and linear mixed-effects models (LMM) were fitted on three agroecologies and five improved chicken breeds to evaluate growth performance until 180-days-of-age (W180) and yield stability (environmental sensitivity). A total of 21,562 chickens were evaluated in 1,557 smallholder flocks. Our results show that LMM had the best model fit on productivity and yield stability. In both methods of MEPA, Sasso and Kuroiler dual-purpose commercial hybrid chickens were the most productive breeds for W180. Indexes based on LMM consistently identified these two breeds also as the most yield stable. Our results demonstrate that the existing methods of MEPA that are being used in plant breeding are applicable to breed performance comparisons and prediction of genotype by environment interactions (GxE). Moreover, the present study validated that SDM-defined agroecologies are useful for undertaking MEPA in smallholder livestock systems.
Introduction
Rapid human population growth, steady economic development, urbanization, and a shift in dietary patterns in Africa have induced high demands for animal-sourced foods. In 2022, the size of human population in sub-Saharan Africa grew at an annual rate of 2.5% (DESA, 2022). As of 2013, the average annual per capita consumption of meat in Africa reached 19 kg —this is projected to increase to 26 kg in 2050 (FAO, 2019). With no sustainable selective breeding scheme in place in the last decades to improve growth rate and egg number under smallholder systems, current levels of production mainly based on indigenous chickens dragged far behind the growing demands. Mean body weight at 16-weeks-of-age for an individual local chicken kept in smallholder (low- to medium-input management) systems can be as low as 621 g, with mean annual egg production below 60 eggs/hen (Dana, 2011; Getachew et al., 2016). Genetically improved dual-purpose chicken breeds (developed for meat and eggs), on the other hand, weigh around 1,600 g at the same age with mean annual production of around 180 eggs (Alemu et al., 2021; Guni et al., 2021) under smallholder semi-scavenging system. Genetically improved chicken breeds constitute diverse genetic background, which is also reflected on their productive performance and environmental sensitivity. Dual purpose breeds can be an outcome of selective breeding on indigenous chicken populations from the tropics (Dana, 2011). They may be composites that created from multiple parental breeds but are genetically stable enough to reproduce across generations (Grobbelaar et al., 2010). Dual-purpose commercial hybrids are produced by crossing two or more purebred lines to take advantage of heterosis (hybrid vigor) and are not genetically stable to be maintained over generations (Gura, 2007). Some dual-purpose breeds introduced into tropical regions have their origin in temperate areas (Hassen et al., 2006).
Thus, far, attempts to enhance livestock productivity in Africa by introducing improved chicken breeds did not bring about the desired results mainly due to a mismatch between genotype and environmental conditions (Tadelle et al., 2000; Safalaoh, 2001; Magothe et al., 2012; Wurzinger et al., 2014; Birhanu et al., 2021). Heterogenous production environments (e.g., influenced by different climatic conditions) require robust experimental designs and analytical frameworks which can identify breeds that perform optimally in multiple environments or those utilizable only in specific production contexts.
Evidence for differential performance of livestock breeds in response to environmental factors, such as extremes of temperature, solar radiation, relative humidity and wind speed have already been documented in poultry (Mazzi et al., 2003; Lara and Rostagno, 2013; Lan et al., 2016) (Lozano-Jaramillo et al., 2019; Kebede et al., 2021, 2023, 2024; Gebru et al., 2023); in cattle (Brown-Brandl, 2013; Bagath et al., 2019); and in swine (Ross et al., 2015; Mayorga et al., 2019).
Apart from their level of performance for the trait of interest, candidate breeds need to be evaluated under different environmental conditions for their yield stability (stability) before they are introduced at scale to smallholder farmers. Stability is the ability of a genotype to be less “sensitive” to environmental influences (Finlay and Wilkinson, 1963; Eberhart and Russell, 1966; Shukla, 1972; Lin et al., 1986; Becker and Leon, 1988). Stable genotypes or breeds are populations that show less plasticity across environments (De Jong and Bijma, 2002). Animals that combine high production potential with resilience to external stressors, allowing for unproblematic expression of high production potential and less phenotypic plasticity in a wide variety of environmental conditions are regarded as “robust” (Knap and Su, 2008; Kebede et al., 2024). GxE measures the extent to which genotypes differ in their plastic responses to environmental changes for a given trait (Sultan, 2021).
As a field of study, agroecology can be seen as the application of ecological concepts and principles in the management of plants, animals and their interactions with other life forms in the environment to enhance food security and nutrition (Francis et al., 2003). In the present study, the application of agroecology as a scientific discipline has been exploited to delineate environmental classes or agroecosystems for a livestock species of interest based on the most relevant bioclimatic predictors having strong effects on habitat suitability, productive performance and adaptability.
Livestock species-specific definition of agroecologies based on species distribution models (SDMs) were proposed by Kebede et al. (2023). In their study, SDM-defined agroecologies delineated based on environmental predictors which influence habitat and phenotypic differentiation resulted in better model fit in predicting GxE compared to conventional agroecologies. The conventional agroecologies in Ethiopia are defined primarily based on environmental predictors which influence plant growth (e.g., the length of growing season, cropping pattern, and land use type; Dove, 1890; MoA, 1998) and are not suitable to serve as a basis for breed performance comparison.
The level of productivity and yield stability of improved breeds is considerably affected by the environment they are introduced into. The definition of agroecologies based on SDM identified predictors has a potential utility in Multi-environment performance analysis (MEPA) of livestock. Unfortunately, analytical frameworks are not readily available for smallholder livestock breed evaluations to make use of SDM-identified agroecologies. On-farm experimental designs and statistical methods used in plant breeding trials to predict GxE and to test yield stability across environments could be adapted to evaluate breed performances across SDM-defined agroecologies.
Two popular methods of MEPA that are used in plant breeding are additive main effects and multiplicative interaction models (AMMI; Gauch, 2013) and linear mixed-effects models (LMM; Piepho, 1994; Piepho and Möhring, 2005). AMMI is a family of powerful multi-environment analytical techniques which is widely used to study GxE interactions (Barhdadi and Dubé, 2010; Culman et al., 2009; Mukherjee et al., 2012; Rodrigues et al., 2016; Rincent et al., 2019). While AMMI models provide more accurate estimates compared to traditional ANOVA (Van Eeuwijk et al., 2016), LMMs through REML/BLUP are predictively even more accurate than AMMI (Piepho, 1994; Van Eeuwijk et al., 2016). Combining different analytical approaches helps identify stable and productive breeds across environments (Olivoto et al., 2019a).
The relative efficiency of different MEPA models in analyzing productivity levels and yield stability among livestock breeds has not been investigated. The objectives of the present study are to (1) evaluate two commonly used methods of MEPA for their applicability in livestock breed performance comparisons in smallholder livestock systems by taking chicken breeds as an example; (2) validate applicability of SDM-defined agroecologies in MEPA; and (3) apply MEPA to identify the most productive and yield stable chicken breeds for wider use by smallholder farmers.
Materials and methods
Experimental design
The present study was designed in a Randomized Complete Block Design (RCBD) in Ethiopia. Five chicken breeds were assigned as treatments (GEN) into each of the three agroecologies or environmental class (ENV). Performance testing sites (PTSs) within each environment were considered as environmental replicates (REP) or blocks denoted with b. The administrative concepts of village and districts were not relevant in the design of present study and were ignored (Supplementary Table 3).
The number of households receiving a breed of chicken in each PTS varies across regions (Supplementary Table 3). In total, 25 chicks of an improved breed, vaccinated against major diseases (Newcastle disease, Gumboro or infectious bursal disease, fowl pox, and Marek's) and brooded to the end of 42 day-of-age were given to each household. The assignment of breeds into households within a PTS was at random. The sampling framework for the present study comprised a total of 56 PTSs or smallholder chicken keeping villages. Performance data was collected on a total of 21,562 female chickens distributed to 1,557 households (Table 1; Supplementary Table 3). Individual households at each PTS were georeferenced and linked with their respective agroecologies (see Supplementary Tables 1, 2).
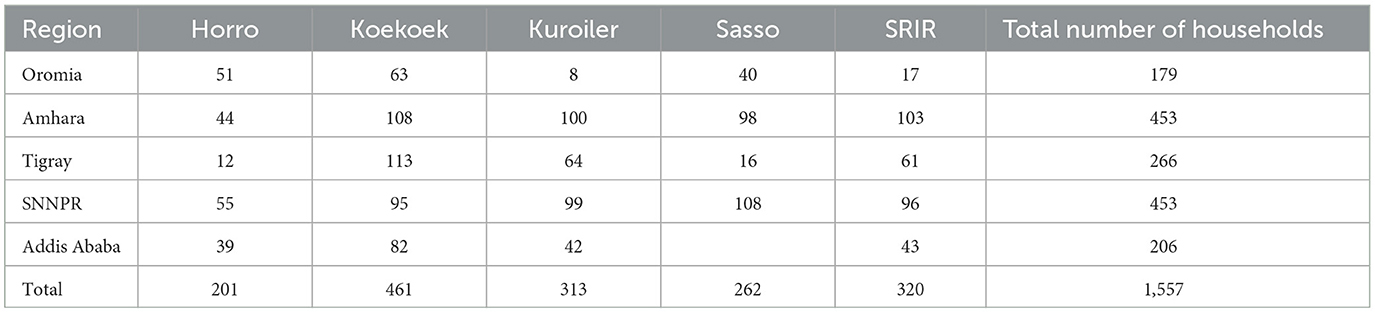
Table 1. Number of participant households receiving a breed of chicken for a multi-environment performance analysis of live body weight (W180) by region in Ethiopia.
Phenotypic data and management of chickens
Improved Horro/Horro, Kuroiler, Potchefstroom Koekoek/Koekoek, Sasso, and SRIR breeds of chicken were evaluated for their growth performance until 180-days-of-age (W180) per PTS (Table 1; Supplementary Table 3). A household received only one of the five breeds assigned to it at random.
Prior to the onset of the on-farm experiment, participant farmers were trained on improved management of chickens (e.g., feeding, biosecurity measures) to achieve reasonable uniformity among experimental units. Enumerators monitored farmers to ensure that they had constructed night shelters for their birds and provided 30% of their daily feed requirement as a supplement, on top of scavenging. Every bird was individually identified with a wing-tag and its live body weight was measured every 2 weeks with digital weighing scale (10 g accuracy). The scale was calibrated to “0.0” after hanging a plastic bucket and before each chicken was placed inside for measurement. Once all chickens in a household were measured, the average weight of the flock at that specific datapoint was reported in near real time to a central computer server via ODK collect, an Android based mobile application (Hartung et al., 2010).
Environmental data
Multi-environment performance testing of breeds in the present study was based on the three agro-ecologies (AEI, AEII, and AEIII) defined for chicken (Gallus gallus) in Ethiopia by Kebede et al. (2023) through species distribution modeling. Each agroecology in their study represented a group of performance testing sites within which environmental conditions are relatively homogenous. Out of 34 environmental predictors obtained from WorldClim database (http://www.worldclim.org/; version 2) at a spatial resolution of 30 s (~1 Km2; Fick and Hijmans, 2017), and tested by maximum entropy modeling (Phillips et al., 2017), a final set of six least correlated predictors (|r| < 0.6) that contributed the most to suitability of habitat for chickens were used as a basis of agroecological classification (Kebede et al., 2023). These included elevation, solar radiation in May, precipitation of the driest month (Bio14), water vapor pressure of May, precipitation of the coldest quarter (Bio19), and precipitation of the wettest month (Bio13).
Data preparation for MEPA
Since the focus of our study was on comparison of breed performance within and between agroecologies, we used average flock performance of chickens in each household for analysis. A reasonable level of uniformity was expected within commercial chicken populations and there was no reason to take the weights of individual animals for a breed assigned in each household. Supplementary Figure 1 shows the results of data inspection to verify assumptions of parametric analysis have been met prior to MEPA. Interquartile range (IQR) is not affected by extreme values and has an advantage over standard deviation as a measure of dispersion. The first quartile (Q1) represents the value below which 25% of the data falls. Low outliers (below Q1 - 1.5*IQR) and high outliers (above Q1 + 1.5*IQR) of live body weight at a specific age were removed from further analysis. No outlier was detected for W180. A visual inspection of performance data (Figure 1) showed change in productivity levels (W180) of breeds across agroecologies and warranted a more detailed analysis.
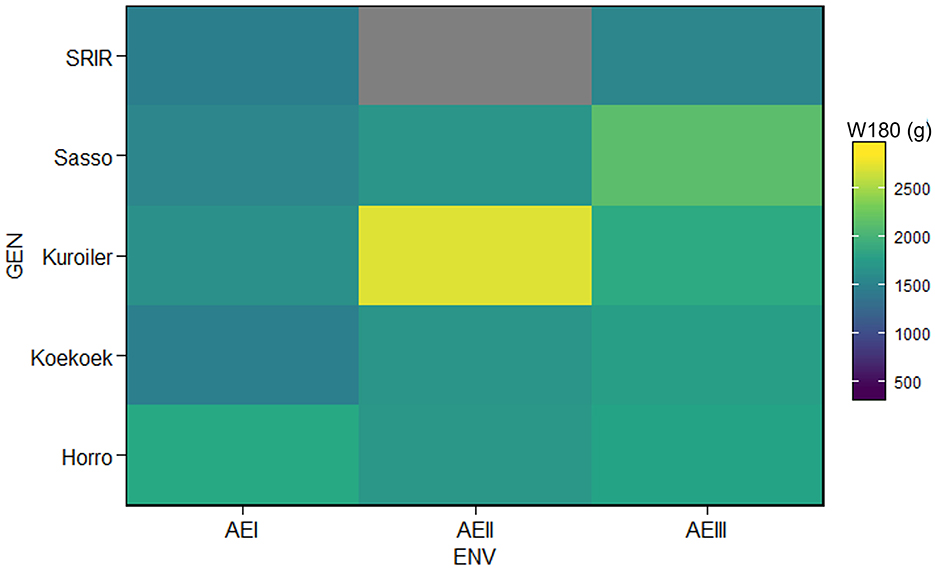
Figure 1. Visual inspection of performance data to see a change in the productivity of breeds (GEN) across environments.
Modeling of multi-environment performance data
The term “stability” in the present study is used to describe the level of fluctuation in W180 of breeds across production environments (i.e., SDM-defined agroecosystems: AEI, AEII, and AEIII). A breed that is less sensitive to environmental variations will be considered more stable. The level of productive performance and yield stability for W180 are evaluated through two different approaches, discussed below, with the R package metan (Olivoto and Lúcio, 2020).
AMMI-based productivity and stability analysis
Additive Main effects and Multiplicative Interaction (AMMI) model works under a fixed-model framework and is fitted at two stages (Zobel et al., 1988; Gauch, 1992; Rodrigues et al., 2014). First, the main effects of the model (i.e., the effects of breeds and environments) are estimated using the additive two-way analysis of variance (ANOVA) least squares. Then, PCA is applied to the residuals of the ANOVA, which includes the interaction, to obtain the multiplicative terms of the AMMI model. The mean response of individual breeds averaged over b replications within each environment was computed. The estimate of the response variable in a completely randomized design, can be obtained by the following model:
where Yi,j is the response variable (i.e., W180 in grams) of the ith breed (i= 1, 2, 3, 4, 5) in the jth environmental class or agroecosystem (j = 1, 2, 3); μ is the grand mean; βi is the main effect of the ith breed; τj is the main effect of the jth environment; λk is the singular value for the kth principal component (PC) axis; γi,k is the ith element of the kth eigenvector; δj,k is the jth element of the kth eigenvector; ρi,j is the residual, containing all multiplicative terms not included in the model; ϵi,j is the experimental error assuming normal, identical and independent distribution ; and n is the number of principal components retained in the model.
We have chosen the AMMI stability value (ASV) and Yield and Stability Index (YSI) to analyse our data out of several AMMI based stability measures available in the plant breeding literature. The ASV is a relatively simple estimate based on PC1 and PC2 scores and can be more clearly explained in terms of environmental and/or biological factors. Moreover, according to Purchase et al. (2000), ASV is highly correlated with other stability measures such as those of Eberhart and Russell (1966), Wricke (1964), and Shukla (1972). AMMI stability indexes were computed using the R package agricolae (De Mendiburu, 2021). The AMMI stability value (ASV) was computed from the first and the second principal components (PC1 and PC2) of the AMMI model according to Purchase et al. (2000), , where b is the number of blocks.
The yield and stability index (YSI) was calculated by adding together the AMMI stability value (ASV) with the rank of mean growth yield of breeds (RY) across environments (i.e., YSI = rASV + RY). The advantage of YSI is that it incorporates both mean yield and stability into a single criterion. Low values of both parameters (i.e., ASV and YSI) show desirable breeds with high stability. Graphic nominal AMMI yield plot (Gauch and Zobel, 1997) as a function of the environmental PC1 scores was used to visualize yield stability of the five improved chicken breeds across three agroecologies for W180.
LMM-based productivity and stability analysis
We have analyzed our productivity data with linear mixed-effects models (LMMs). Deciding whether to treat genotype, environment, or their interaction (GxE) as fixed or random effects is often subject to debate in GxE analysis. Hence, we used Akaike information criterion (AIC) to resolve the issue by providing a way to compare the goodness of fit of the different mixed-effects models (Akaike, 1987). The model with the lowest AIC value will be considered as the most appropriate model as it provides the best trade-off between fit and complexity.
In model 1, we fitted environment (ENV) as a fixed effect and included breed (GEN) and breed by environment interactions (GxE) as random effects. In this model, the interest was to see how specific environments (agroecologies) influence chicken performance regardless of breed. The equation can be written in a standard linear mixed model (Yang, 2007; Olivoto et al., 2019a) to estimate W180 as follows:
where y is a vector of response variable (i.e., body-weight-at-180-days-of-age); b is a vector of unknown and observable fixed effects; u is vector of unobservable random effects; X is a design matrix of 0 s and 1 s relating y to b; Z is a design matrix of 0 s and 1 s relating y to u; and e is a vector of random errors. The vectors b and e in mixed model are estimated using the equation by Henderson (1975).
In model 2, we fitted breed (GEN) as a fixed effect and included replication (ENV), environment (ENV) and breed by environment interactions (GxE) as random effects. Here our focus is on making specific conclusions about the performance of particular breeds, while allowing for generalization across environments and understanding how the interaction between breeds and environments behaves across the different conditions. In model 3, we fitted breed (GEN), replication (ENV), environment (ENV) and breed by environment interactions (GxE) as random effects. This model was fitted to see general patterns regardless of specific breed and environments. In model 4, we fitted breed (GEN), replication (ENV), environment (ENV) and breed by environment interactions (GxE) as fixed effects to get insights into how performance varies across specific breeds, environments, and their interactions.
We computed the harmonic mean of the relative performance of genotypic values (HMRPGV) of the five improved chicken breeds for W180. HMRGV proposed by Resende (2007) was used efficiently as LMM-based stability index to select genotypes with high adaptability and stability in other studies (Colombari Filho et al., 2013; Azevedo Peixoto et al., 2018; Dias et al., 2018). The HMRPGV to evaluate the yield stability was estimated according to the equation (Resende, 2007; Olivoto and Lúcio, 2020):
where: HMRPGVi is the harmonic mean of the relative performance for breed i; Gvij is the growth performance (W180) of ith breed in the j agroecosystem; and E is the number of environments in which breed i was tested (j = 1, 2, 3); uj is the mean performance of all breeds in environment j; Gvij is the relative performance of breed i in environment j. In the HMRPGV method for stability analysis, the breeds are simultaneously sorted by values for yield and stability using the harmonic means of the LMM so that the smaller the standard deviation of breed performance among the agroecologies, the greater the HMRPGV.
WAASB biplots (WAASBY stability index biplots) were generated based on LMM predictions (Olivoto et al., 2019a,b). WAASBY stability index biplots allow weighing and visualizing between stability (y-axis) and performance (x-axis) of the different chicken breeds across three agroecologies.
Results
AMMI-based productivity and stability analysis
The AMMI analysis of variance indicated highly significant (p < 0.001) effects of genotype, environment, and interaction for W180 (Table 2). The treatment sum of squares (SS) for growth performance was partitioned into three sources: the genotype main effect (GEN), the environment main effect (ENV), and the interaction (GxE). Environment (ENV SS) had higher contribution (47.3%; p < 0.001) to variability of performance in live body weight across chicken breeds at 180-days-of-age. W180 was also significantly influenced by the type of breed (40.4%); p < 0.001).The AMMI analysis had identified only two principal components for having effects on productivity (Table 2). Both the first and the second principal component (PCs) had significant effect (p < 0.001) on productivity. PC1 explained most of the GxE interaction (68.4%). Higher values of principal component (PC1) indicate GxE is important in explaining phenotypic variation in the dataset.
AMMI nominal yield plots presented in Figure 2 show the stability of the five improved chicken breeds for W180 in three different agroecologies (AEI, AEII, and AEIII). The nominal yield plots have a complementary advantage over AMMI stability indexes because they integrate and visualize information on productivity and stability. Sasso an Kuroiler were the most productive breeds across agroecologies (AEI and AEIII). However, in terms of stability alone (without considering body weight), SRIR had a gentle slope and was observed to be the most stable across agroecologies. When the five breeds were evaluated at three different ages (W90, W120, and W180), we still observe that Sasso and Kuroiler had the highest weight and gentle slopes showing higher stability at W90 compared to W180 (Supplementary Figure 2).
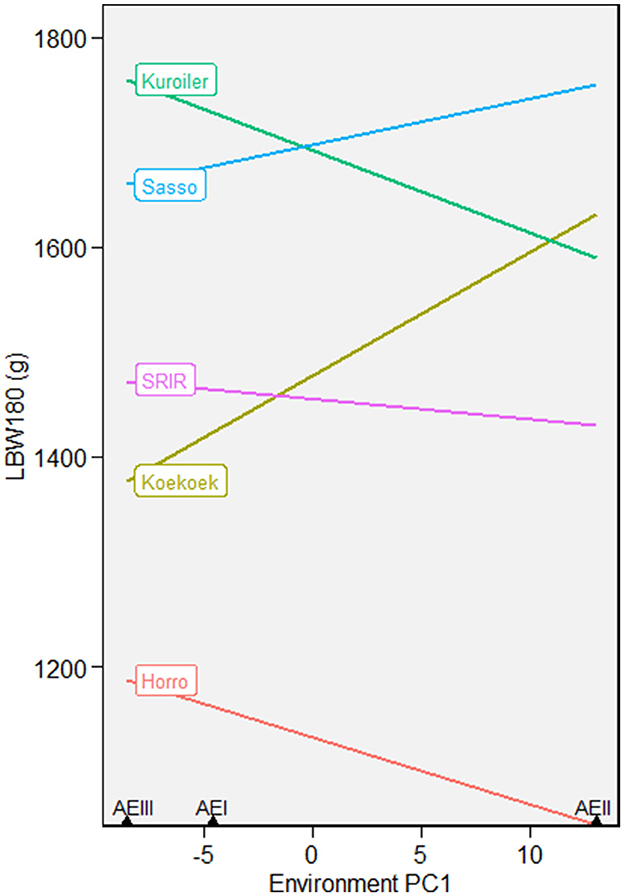
Figure 2. AMMI nominal yield plots showing mean growth performance and stability of five improved chicken breeds introduced into three different agroecologies for growth until 180-days-of-age (W180).
Table 3 shows the AMMI stability indexes (rASV and rYSI) computed for W180. Sasso, the breed with the highest live body weight performance was also identified as the most stable breed by both indexes. We can easily see that these stability indexes are influenced more by productivity levels than the slope of the lines for individual breeds.
LMM-based productivity and stability analysis
The linear mixed-effects model (LMM) comparisons for W180 of improved chicken breeds using AIC are presented in Table 4. The model with the best fit (lowest AIC value) had environment (ENV) and environmental replicates (REP) as a fixed effect, and breed (GEN) and breed by environment interaction (GxE) as random effects (model 1).
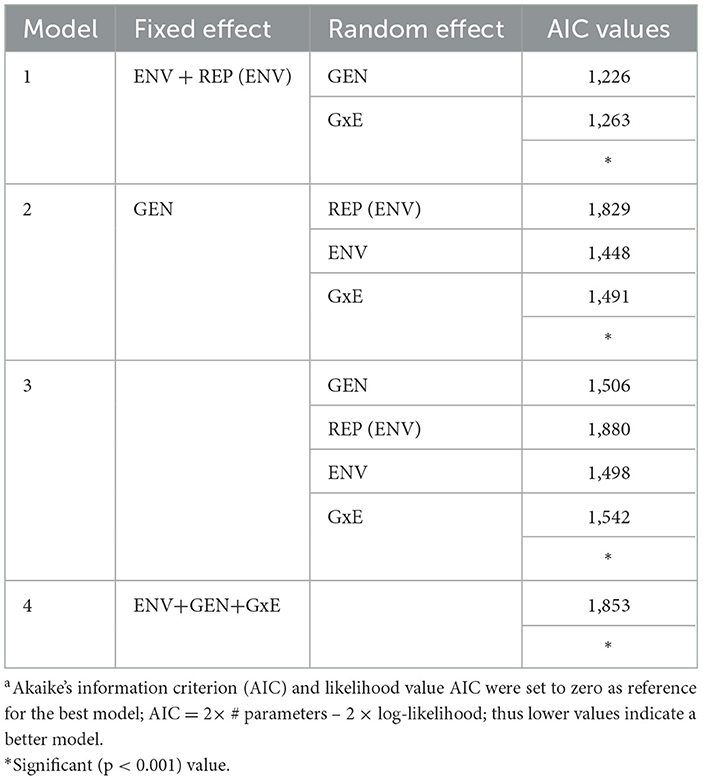
Table 4. Comparison of models fitted with linear mixed-effects model (REML) for W180 of improved chicken breeds using AICa.
The interaction between breed and environment was significant (p < 0.001) for W180 (Table 5). Sasso had the highest body weight in AEI and AII, while Kuroiler excelled in AEIII. Koekoek had moderate performance that falls between the two top performers and SRIR. Horro yielded the lowest body weight in all the three environmental classes.
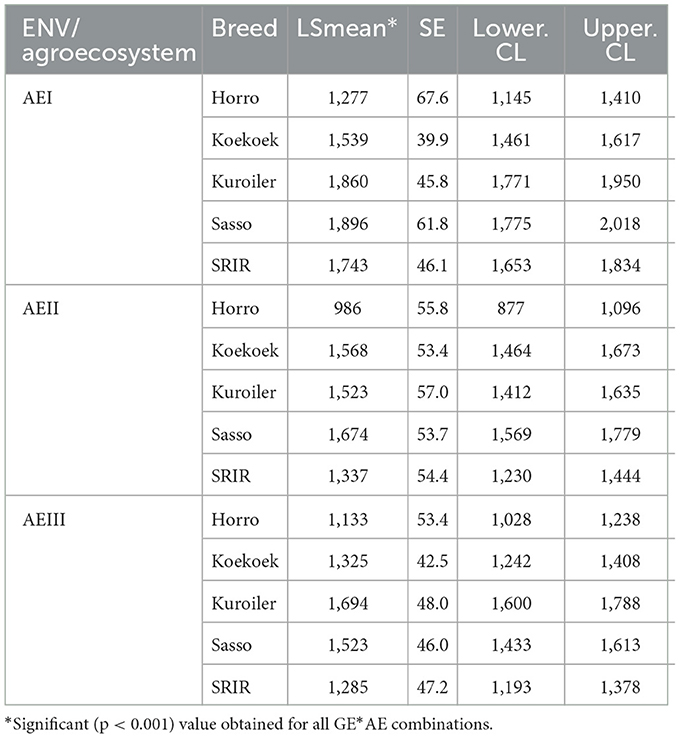
Table 5. W180 (LSMean ± SE) of female chickens by breed (GEN) and environmental class (ENV) from linear mixed-effects model analysis.
Table 6 shows harmonic mean of the relative performance of genotypic values (HMRPGV) stability index based on the best fit linear mixed-effect model (model 1). The breeds with the highest stability (highest HMRPGV) for W180 were Sasso (1.14) and Kuroiler (1.13). Horro had the lowest stability (0.76).
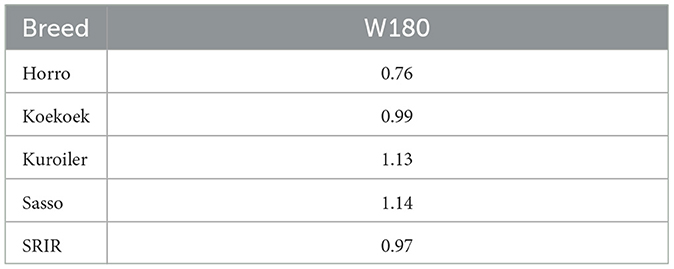
Table 6. Harmonic mean of the relative performance of genotypic values (HMRPGV) stability index based on linear mixed-effect model.
The results of WAASB stability analysis based on the best fit LMM (model 1) are displayed in Figure 3. The four quadrants in each of the three biplots represent classifications regarding the joint interpretation of mean performance and stability. The lower the WAASB value, the more stable the breed can be considered. Quadrant I contained unstable breeds, agroecologies with high discrimination ability, and productivity level of below the grand mean. The breed within quadrant III (i.e., SRIR) had low productivity but could be considered stable due to the lower value of WAASB. Horro and Koekoek in quadrant I are far from the origin on the y-axis and are also below the midpoint on the x-axis showing below average performance and least stability. The environment included in the third quadrant was considered as unfavorable and having low discrimination ability of breed performance. Sasso and Kuroiler breeds positioned on right side of the biplot in quadrant IV exhibit the highest performance for W180 and are favored in that specific environmental class (AEI). These two breeds have low WAASB values indicating their greater stability.
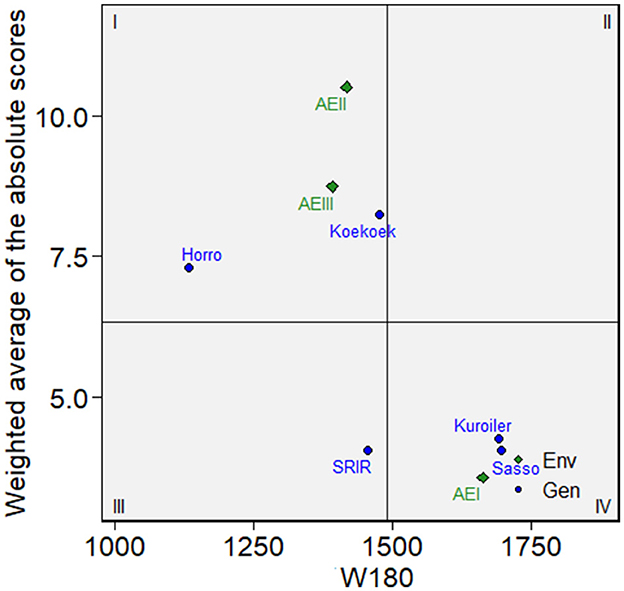
Figure 3. WAASB biplots generated based on LMM analysis showing mean growth performance until 180-days-of-age (W180) of five improved chicken breeds introduced into three different agroecologies in Ethiopia. The x-axis represents the mean performance of the breeds (body weight in grams), while y-axis measures the stability of the genotypes across environments.
Discussion
Smallholder chicken production plays significant role in poverty reduction in developing countries by improving household income, empowering women, creating jobs, and increasing the consumption of nutrient rich animal source foods (ASFs; Birhanu et al., 2023; Passarelli et al., 2020). Introduction of already developed productive and adaptive chicken breeds holds opportunities to enhance current levels of productivity and production in a short time compared to developing new ones through selective breeding. In the last 20 years, the poultry production landscape has seen positive changes in terms of access to inputs, support services, and in the development of tailored business models (Birhanu et al., 2021, 2022, 2023; Birhanu and Jensen, 2023) justifying the technical feasibility of scaling improved dual-purpose chicken breeds for the betterment of rural and urban livelihoods.
GxE studies in livestock most commonly use reaction norms to describe the response of breeds to environmental variation (Oliveira et al., 2018; Cheruiyot et al., 2020; Chen et al., 2021). We adapted two methods of MEPA (AMMI and LMM) to evaluate productivity (live body weight) and stability. Our results demonstrate that MEPA has several advantages over conventional methods such as Analysis of Variance (ANOVA) and linear regression. MEPA captures both additive and multiplicative components, improves prediction accuracy through inclusion of random components, provides visual tools (biplots) that are helpful to assess stability and adaptability of genotypes across multiple environments, and better accommodates unbalanced data due to missing observations. In MEPA, it is possible to include environmental covariates to study how specific variables influence performance of genotypes.
Our results from AMMI and LMM methods showed the presence of breed by environment interaction (GxE). Based on WAASB biplots of LMM, Kuroiler and Sasso were identified as the two breeds which combined the most high growth performance (W180) with stability across agroecologies. AMMI (rASV and rYSI) and LMM (HMRPGV) stability indexes have jointly identified Sasso as the most stable of the five chicken breeds evaluated. A change in rank was observed between Sasso and Kuroiler whereby Kuroiler excelled in body weight in the third environment (AEIII) but not in the first two. This implies that the two breeds responded differently to environmental challenges and exhibited varying phenotypic plasticity probably owing to their different genetic background. The two breeds indeed have different origins. Both Sasso and Kuroiler are commercial hybrids developed to suit semi-scavenging management systems in Africa and Southeast Asia (Van Arendonk et al., 2019; Duijvesteijn et al., 2022). Sasso chicken is a blend of many breeds including the Rhode Island Red from USA and a strain of indigenous chicken from France that contribute to its hardiness and adaptability (OSU Breeds of Livestock, 2024). Kuroiler originates from crossing the Rhode Island Red, the White Leghorn, the Barred Plymouth Rock and two Indian indigenous chicken breeds, with some introgression of broilers to obtain specific broiler characteristics (Ahuja et al., 2008a,b; Isenberg, 2007). The environmental predictors which served as the basis for definition of the three agroecosystems (e.g., elevation, solar radiation, and precipitation) probably had some effects on availability of feeds and prevalence of diseases (parasites) inducing direct or indirect impacts on live body weight our test breeds.
Improved Horro had the lowest body weight, and this contributed to poor stability indexes by AMMI and LMM. Horro is a breed under development and has so far been selected for 10 generations from the population of indigenous Horro chickens (Kebede et al., 2021). The Horro chicken selective breeding programme focuses on improving age at first egg, egg production, body weight, and survival (Dana et al., 2010, 2011; Wondmeneh et al., 2014).
Koekoek and SRIR had average performance in terms of W180 and stability. The SRIR (Sasso x Rhode Island Red) is a commercial hybrid closely related with the Sasso (Kebede et al., 2023). The Koekoek (Potchefstroom Koekoek) is a composite of the White Leghorn, Black Australorp, and the Barred Plymouth Rock, developed during the 1950's in the Republic of South Africa (Grobbelaar et al., 2010; Kebede et al., 2023). It is very popular among rural farmers in South Africa and neighboring countries for egg and meat production as well as their ability to hatch their own offspring in medium input production systems (Grobbelaar et al., 2010).
The highest model fit in LMM in the present study was obtained when environmental class (agroecosystem) was fitted as a fixed and genotypic effects and their interactions with the environment were fitted as random (model 1). Fitting breed as random might be useful to make conclusion on suitability of environments to a broader range of breeds. Apart from model fit (based on AIC), however, fitting breed as random may not be biologically meaningful considering that we are not evaluating breeds that are random samples of large population of potential breeds. Hence, if the interest is to recommend breeds adapted to specific environments, fitting breed as a fixed effect might be more appropriate. In contrast to traditional definitions that classify genotypic effects as fixed, the highest model fit in plant breeding trials was obtained when genotypic effects were considered as random (Stroup and Mulitze, 1991; Olivoto et al., 2019a). More studies are needed on model validation in livestock for implementation of MEPA under different scenarios, such as when the interest is to identify breeds for specific environments and when the intention is to identify breeds which are broadly adapted to multiple environments under smallholder systems.
Studies comparing the different methods of MEPA in plant breeding are documented by Balestre et al. (2009) and Sa'diyah and Hadi (2016). After comparing Eberhart-Russel, AMMI, and mixed-models, Ferraudo and Perecin (2014) concluded that the three methods detected GxE differently but effectively, with mixed models showing higher sensitivity. Some of the indexes (e.g., WAASB) can even integrate the features of AMMI and LMM techniques and display their results on performance using biplots (Olivoto et al., 2019a). Biplot-based interpretation of MEPA on LMM results helps easily identify “which breed wins where” and facilitates recommendation of specific breeds for specific agroecologies. LMMs such as the best linear unbiased prediction (BLUP) and restricted maximum likelihood estimation (REML) are more predictively accurate than AMMI and the main advantages of these methods can be combined to recommend highly productive and stable individuals or populations (Van Eeuwijk et al., 2016; Olivoto et al., 2019a).
There were some limitations regarding the study we carried out on yield stability that could be addressed to get better results in the future. We have observed that the stability indexes in all methods of MEPA evaluated in the present study are biased toward more productive genotypes. SRIR, which had a moderate yield but the most gentle slope for W180, did not achieve the highest stability indexes on AMMI and LMM because of its inferior performance when compared to Sasso and Kuroiler. The locally improved Horro had the lowest productive performance and the least stability index. This is contrary to genetic and physiological evidence in the literature supporting that local (indigenous) populations exhibit better local adaptation to environmental stressors than introduced breeds.
Methods for multi-environment performance evaluation should consider economic efficiency apart from productivity and stability. Socio-economic parameters applicable to MEPA include farm income, farmers' trait and breed preferences (likability), culture, and consumer preferences. Although breeds such as Kuroiler and Sasso perform better than most local ecotypes in terms of productivity, the feed utilization efficiency of these breeds is far lower than current commercial breeds (Van Arendonk et al., 2019). Pedigree, individual phenotype, and genomic information were not available in the present study to perform animal evaluation and select the best performing individuals within a breed. In circumstances where phenotypic, pedigree, environmental and genomic information are available, random regression models via reaction norms (Veerkamp and Goddard, 1998; Oliveira et al., 2018) are applicable to estimate the breeding value of individual animals for traits such as growth and survival under varying environmental conditions. Spatial analysis considering environmental (climatic) factors was conducted by Sæbø and Frigessi (2004) and Tiezzi et al. (2017) to improve genetic prediction in smallholder dairy cattle evaluation. Challenges affecting genetic evaluations across environments in smallholder systems due to poor data structure (e.g., small herd size, low genetic connectedness, and no pedigree) and ways to address them are discussed in several studies (Powell et al., 2021; Jorjani et al., 2001; Foulley et al., 1990). Kebede et al. (2023) followed a different approach to reaction norms to visualize the influence of bioclimatic predictors on breed performance by using partial dependence plots produced by General Additive Models (GAMs). Models applicable to phenotypic plasticity in evolutionary biology and animal breeding are reviewed by De Jong and Bijma (2002) and can also be evaluated for their use in in multi-environment performance evaluations.
The importance of reducing potential confounding between the effects of management practices of individual households (e.g., feeding, health, and housing) and challenges from the physical environment (e.g., climate, geography) need to be carefully considered in multi-environment performance evaluations. We tried to standardize the management practices of the farmers participating in the present study by taking certain precautionary measures. By implementing RCBD, we tried to control for variability among experimental units by grouping them into blocks that are as homogeneous as possible. Within each block (environmental replicate) treatments (breeds) were randomly assigned to experimental units (households). The amount of feed supplemented per flock (on top of scavenging) among households was regulated, animal health practices were reasonably kept uniform, and night enclosures were put in place in all the households to reduce experimental errors. Taking large sample size of participants has also helped in accounting for variations in the management of flocks among households with different socio-economic backgrounds (e.g., literacy levels, farm resources). Once the right breeds suitable for technological scaling have been identified, based on their productive performance and stability, introduction of the breeds has to be accompanied by improved access to other essential inputs and support services/infrastructure (e.g., feeds, flock health, extension, marketing, and financial models) to achieve sustained adoption of innovations.
Conducting MEPA in farm animals has its own peculiarities compared to multi-environment trials in plants. In the field of plant breeding, the analysis of genotype by environment interactions (GxE) is well-supported by extensive data, largely because plants can be easily grown across multiple environments with repeated measures for each genotype at specific locations. Such large datasets allow precise measurements of GxE, providing clear insights into performance of genotypes under varying environmental conditions. However, the scenario is markedly different in animal breeding. The mobile nature of animals and their cost impedes the replication of genotypes across diverse environments to undertake MEPA at individual and population level under on-farm conditions.
Apart from its practical applicability in developing countries (Africa and Southeast Asia), MEPA can potentially contribute to promotion of sustainable food systems in developed regions of the world by identifying and scaling more productive and better adapted breeds within a framework of circular economy. Matching breeds of optimal performance with the right agroecosystem improves resource efficiency (lowers the amount of farm inputs required), reduces waste, improves economic viability, and builds resilience to environmental changes and shocks.
Equal emphasis should be given to the improvement of local (indigenous) populations alongside the testing of already developed improved breeds. Selective breeding on local populations requires more resources than breed introduction but holds many advantages for smallholder farmers compared to the scaling of commercial hybrids. To mention but a few (1) animals can be used by farmers without intellectual property right (IP) restrictions; (2) purebreds are genetically stable their eggs can be used to sustain future generations; (3) they have well-established consumer and producer preference or niche market for their appearances, product quality and sociocultural values; (4) they can be used in cross-breeding programmes with improved or other local breeds to develop robust genotypes; (5) they have certain adaptive qualities making them suitable for smallholder systems (e.g., alertness, scavenging ability, disease tolerance, and adaptability to harsh environmental conditions); and (6) the development of such breeding programs enhances sustainable utilization and conservation of local genetic resources in food systems.
Issues of biodiversity conservation, sustainable utilization, and intellectual property rights should be carefully considered when performance testing is conducted across wider geographies or regions. The adverse impacts of market consolidation and vertical integration from mergers of multinational animal breeding corporations involved in supply of improved livestock genetic material in Africa and Asia are well-documented. These include limited access to breeding stock, high price of animal products and feed, removal of potential competition in the value chain, and restriction of product choices of consumers (Bagopi et al., 2016; Goga and Roberts, 2023; Karamchedu and Syndicus, 2022).
Finally, addressing the pressing challenges in food system of developing countries (nutritional security, climate change, gender equality, and employment opportunities) requires strategic implementation of different but complementary approaches. The choice of the right genetic intervention depends on the prevailing socio-economic and environmental contexts of the specific country.
Conclusion
The performance of animals in smallholder livestock systems of the tropics are significantly influenced by the environment. Apart from productivity levels, candidate improved strains should be evaluated for yield stability to increase production efficiency and address nutritional security. The present study revealed that existing methods of multi-environment performance analysis from plant breeding literature are applicable to chicken (livestock) breed evaluations. Our results also demonstrate that species-specific agroecologies defined by SDMs are useful to undertake MEPA in livestock. LMM-based productivity and stability indexes/biplots combining information on productivity with yield stability showed superiority over the other MEPA models. The present study illustrated that there is a strong need to address limitations of existing methods and develop robust analytical framework and methodologies for spatial analysis of within and between breed performance. Such methods need to integrate environmental, genetic/genomic, phenotypic and socio-economic information. Advances in geographic information system, ecology, machine learning, genomic prediction, and food systems should be harnessed to optimize MEPA and increase animal productivity and environmental efficiency.
Data availability statement
Publicly available datasets were analysed in this study. The datasets used can be found in online repository: https://data.ilri.org/portal/dataset/acggonfarmeth.
Ethics statement
The animal studies were approved by Institutional Research Ethics Committee (IREC) of International Livestock Research Institute (ILRI; Ref: ILRI-IREC2015-08). The studies were conducted in accordance with the local legislation and institutional requirements. Written informed consent was obtained from the owners for the participation of their animals in this study.
Author contributions
FK: Conceptualization, Data curation, Formal analysis, Investigation, Methodology, Resources, Software, Validation, Visualization, Writing – original draft, Project administration. HK: Conceptualization, Funding acquisition, Methodology, Project administration, Resources, Supervision, Writing – review & editing. TD: Conceptualization, Funding acquisition, Investigation, Methodology, Project administration, Resources, Supervision, Writing – review & editing. OH: Funding acquisition, Project administration, Resources, Supervision, Writing – review & editing. SK: Conceptualization, Funding acquisition, Project administration, Resources, Writing – review & editing. SA: Data curation, Methodology, Writing – review & editing. JB: Conceptualization, Funding acquisition, Investigation, Methodology, Project administration, Resources, Supervision, Writing – review & editing.
Funding
The author(s) declare financial support was received for the research, authorship, and/or publication of this article. The research was funded by the African Chicken Genetic Gains (ACGG) project under the Tropical Poultry Genetic Solutions (TPGS) Program of the International Livestock Research Institute (ILRI) with grant received from the Bill and Melinda Gates Foundation (Investment IDs: OPP1112198 and INV-042538). Additional funding was obtained from the CGIAR Initiative on Sustainable Animal Productivity or SAPLING.
Acknowledgments
We thank the Ethiopian Agricultural Research Institute (EIAR) and Regional Agricultural Research Institutes in Oromia, Amhara, Southern and Tigray Regional States for their crucial involvement in on-farm implementation of ACGG activities. We also thank the farmers and enumerators who participated in chicken performance evaluation. ILRI, Animal Breeding and Genomics of Wageningen University and Research (WUR), and the Koepon Foundation provided a Ph. D. scholarship to FK. The findings and conclusions contained within are those of the authors and do not necessarily reflect positions or policies of the Bill & Melinda Gates Foundation, WUR, ILRI, and the Kopoen Foundation.
Conflict of interest
The authors declare that the research was conducted in the absence of any commercial or financial relationships that could be construed as a potential conflict of interest.
Publisher's note
All claims expressed in this article are solely those of the authors and do not necessarily represent those of their affiliated organizations, or those of the publisher, the editors and the reviewers. Any product that may be evaluated in this article, or claim that may be made by its manufacturer, is not guaranteed or endorsed by the publisher.
Supplementary material
The Supplementary Material for this article can be found online at: https://www.frontiersin.org/articles/10.3389/fsufs.2024.1441295/full#supplementary-material
References
Ahuja, V., Dhawan, M., Punjabi, M., and Maarse, L. (2008a). “Chickening out of poverty? Story of “kuroiler” from India,” in International Conference (Ahmedabad: Indian Institute of Management).
Ahuja, V., Dhawan, M., Punjabi, M., and Maarse, L. (2008b). Poultry Based Livelihoods of Rural Poor: Case of Kuroiler in West Bengal. South Asia Pro Poor Livestock Policy Programme, initiative of NDDB and FAO: Document 12. New Delhi: NDDB.
Alemu, S. W., Hanotte, O., Kebede, F. G., Esatu, W., Abegaz, S., Bruno, J. E., et al. (2021). Evaluation of live-body weight and the number of eggs produced for introduced and local chickens in Ethiopia. Acta Agriculturae Scandinavica Sect. A Anim. Sci. 70, 71–77. doi: 10.1080/09064702.2021.1891278
Azevedo Peixoto, L. D., Teodoro, P. E., Silva, L. A., Rodrigues, E. V., Laviola, B. G., and Bhering, L. L. (2018). Jatropha half-sib family selection with high adaptability and genotypic stability. PLoS ONE 13:e0199880. doi: 10.1371/journal.pone.0199880
Bagath, M., Krishnan, G., Devaraj, C., Rashamol, V., Pragna, P., Lees, A., et al. (2019). The impact of heat stress on the immune system in dairy cattle: a review. Res. Vet. Sci. 126, 94–102. doi: 10.1016/j.rvsc.2019.08.011
Bagopi, E., Chokwe, E., Halse, P., Hausiku, J., Humavindu, M., Kalapula, W., et al. (2016). Competition, Agro-processing and Regional Development: The Case of the Poultry Section in South Africa, Botswana, Namibia and Zambia. Competition in Africa: Insights From Key Industries (Cape Town: HSRC Press), 66–101.
Balestre, M., Von Pinho, R., Souza, J., and Oliveira, R. (2009). Genotypic stability and adaptability in tropical maize based on AMMI and GGE biplot analysis. Genet. Mol. Res. 8, 1311–1322. doi: 10.4238/vol8-4gmr658
Barhdadi, A., and Dubé, M. P. (2010). Testing for gene-gene interaction with AMMI models. Statist. Appl. Genet. Mol. Biol. 9:2. doi: 10.2202/1544-6115.1410
Becker, H., and Leon, J. (1988). Stability analysis in plant breeding. Plant Breed. 101, 1–23. doi: 10.1111/j.1439-0523.1988.tb00261.x
Birhanu, M. Y., Alemayehu, T., Bruno, J. E., Kebede, F. G., Sonaiya, E. B., Goromela, E. H., et al. (2021). Technical efficiency of traditional village chicken production in Africa: entry points for sustainable transformation and improved livelihood. Sustainability 13:8539. doi: 10.3390/su13158539
Birhanu, M. Y., Bruno, J. E., Alemayehu, T., Esatu, W., Geremew, K., Yemane, T., et al. (2022). Beyond diffusion to sustained adoption of innovation: a case of smallholder poultry development in sub-Saharan Africa. Int. J. Agricult. Sustainabil. 20, 1028–1046. doi: 10.1080/14735903.2022.2041235
Birhanu, M. Y., and Jensen, N. (2023). Dynamics of improved agricultural technologies adoption: the chicken and maize paradox in Ethiopia. Sustain. Fut. 5:100112. doi: 10.1016/j.sftr.2023.100112
Birhanu, M. Y., Osei-Amponsah, R., Yeboah Obese, F., and Dessie, T. (2023). Smallholder poultry production in the context of increasing global food prices: roles in poverty reduction and food security. Anim. Front. 13, 17–25. doi: 10.1093/af/vfac069
Brown-Brandl, T. (2013). Managing thermal stress in feedlot cattle: environment, animal susceptibility and management options from a US perspective. Livestock Hous. 189:10. doi: 10.3920/978-90-8686-771-4_10
Chen, S.-Y., Freitas, P. H., Oliveira, H. R., Lázaro, S. F., Huang, Y. J., Howard, J. T., et al. (2021). Genotype-by-environment interactions for reproduction, body composition, and growth traits in maternal-line pigs based on single-step genomic reaction norms. Genet. Select. Evol. 53, 1–18. doi: 10.1186/s12711-021-00645-y
Cheruiyot, E., Nguyen, T., Haile-Mariam, M., Cocks, B., Abdelsayed, M., and Pryce, J. (2020). Genotype-by-environment (temperature-humidity) interaction of milk production traits in Australian Holstein cattle. J. Dairy Sci. 103, 2460–2476. doi: 10.3168/jds.2019-17609
Colombari Filho, J. M., De Resende, M. D. V., De Morais, O. P., De Castro, A. P., Guimaraes, E. P., Pereira, J. A., et al. (2013). Upland rice breeding in Brazil: a simultaneous genotypic evaluation of stability, adaptability and grain yield. Euphytica 192, 117–129. doi: 10.1007/s10681-013-0922-2
Culman, S. W., Bukowski, R., Gauch, H. G., Cadillo-Quiroz, H., and Buckley, D. H. (2009). T-REX: software for the processing and analysis of T-RFLP data. BMC Bioinformat. 10:171. doi: 10.1186/1471-2105-10-171
Dana, N. (2011). Breeding Programs for Indigenous Chicken in Ethiopia: Analysis of Diversity in Production Systems and Chicken Populations. Wageningen: Wageningen University and Research.
Dana, N., Van Der Waaij, L. H., Dessie, T., and Van Arendonk, J. A. (2010). Production objectives and trait preferences of village poultry producers of Ethiopia: implications for designing breeding schemes utilizing indigenous chicken genetic resources. Trop. Anim. Health Prod. 42, 1519–1529. doi: 10.1007/s11250-010-9602-6
Dana, N., Vander Waaij, E., and Van Arendonk, J. A. (2011). Genetic and phenotypic parameter estimates for body weights and egg production in Horro chicken of Ethiopia. Trop. Anim. Health Prod. 43, 21–28. doi: 10.1007/s11250-010-9649-4
De Jong, G., and Bijma, P. (2002). Selection and phenotypic plasticity in evolutionary biology and animal breeding. Livest. Prod. Sci. 78, 195–214. doi: 10.1016/S0301-6226(02)00096-9
De Mendiburu, F. (2021). Agricolae Tutorial (Version 1.3-5). La Molina: Universidad Nacional Agraria.
DESA, U. (2022). United Nations Department of Economic and Social Affairs, Population Division. World Population Prospects 2022: Summary of Results. UN DESA/POP/2022/TR. New York, NY: UN DESA.
Dias, P. C., Xavier, A., Resende, M. D. V. D., Barbosa, M. H. P., Biernaski, F. A., and Estopa, R. A. (2018). Genetic evaluation of Pinus taeda clones from somatic embryogenesis and their genotype x environment interaction. Crop Breed. Appl. Biotechnol. 18, 55–64. doi: 10.1590/1984-70332018v18n1a8
Duijvesteijn, N., Perrault, L., Atikpakpe, R., Garcia Martinez, N., Renckly, J., and Arbeau, T. Van Arendonk, J. (2022). “Improving growth performance for dual purpose chickens for challenging conditions in Africa,” in Proceedings of 12th World Congress on Genetics Applied to Livestock Production (WCGALP) Technical and Species Orientated Innovations in Animal Breeding, and Contribution of Genetics to Solving Societal Challenges, 2022 (Wageningen: Wageningen Academic Publishers), 1791–1794.
Eberhart, S. T., and Russell, W. (1966). Stability parameters for comparing varieties 1. Crop Sci. 6, 36–40. doi: 10.2135/cropsci1966.0011183X000600010011x
FAO (2019). Africa Sustainable Livestock 2050 - Livestock Sector Development in Asia and sub-Saharan Africa—A Framework for Comparative Analysis. Rome: FAO.
Ferraudo, G. M., and Perecin, D. (2014). Mixed model, AMMI and Eberhart-Russel comparison via simulation on genotype × environment interaction study in sugarcane. Appl. Math. 2014:514205. doi: 10.4236/am.2014.514205
Fick, S. E., and Hijmans, R. J. (2017). WorldClim 2: new 1-km spatial resolution climate surfaces for global land areas. Int. J. Climatol. 37, 4302–4315. doi: 10.1002/joc.5086
Finlay, K., and Wilkinson, G. (1963). The analysis of adaptation in a plant-breeding programme. Austral. J. Agricult. Res. 14, 742–754. doi: 10.1071/AR9630742
Foulley, J., Bouix, J., Goffinet, B., and Elsen, J. (1990). Connectedness in genetic evaluation. Adv. Statist. Methods Genet. Improv. Livest. 7:13. doi: 10.1007/978-3-642-74487-7_13
Francis, C., Lieblein, G., Gliessman, S., Breland, T. A., Creamer, N., Harwood, R., et al. (2003). Agroecology: the ecology of food systems. J. Sustain. Agricult. 22, 99–118. doi: 10.1300/J064v22n03_10
Gauch Jr, H. (1992). Statistical Analysis of Regional Yield Trials: AMMI Analysis of Factorial Designs. Washington, DC: Elsevier Science Publishers.
Gauch Jr, H. G. (2013). A simple protocol for AMMI analysis of yield trials. Crop Sci. 53, 1860–1869. doi: 10.2135/cropsci2013.04.0241
Gauch Jr, H. G., and Zobel, R. W. (1997). Identifying mega-environments and targeting genotypes. Crop Sci. 37, 311–326. doi: 10.2135/cropsci1997.0011183X003700020002x
Gebru, G., Belay, G., Dessie, T., Zenebe Kelkay, M., Birhanie Dagnhegn, M., and Hanotte, O. (2023). Morphological and osteological characterization of indigenous domestic chickens (Gallus gallus domesticus): validation of Rensch's, Bergmann's and Allen's rules. Front. Ecol. Evol. 11:1032082. doi: 10.3389/fevo.2023.1032082
Getachew, F., Abegaz, S., Assefa, A., Misganaw, M., Emshaw, Y., Hailu, A., et al. (2016). Multivariate analyses of morphological traits in indigenous chicken populations of Metekel zone, Northwestern Ethiopia. Anim. Genet. Resour. 59, 15–25. doi: 10.1017/S2078633616000084
Goga, S., and Roberts, S. (2023). Multinationals and Competition in Poultry Value Chains in South Africa, Zambia, and Malawi. Johannesburg Centre for Competition, Regulation and Economic Development (CCRED). CCRED Working Paper Series 2023/11, University of Johannesburg, South Africa.
Grobbelaar, J. A. N., Sutherland, B., and Molalakgotla, N. (2010). Egg production potentials of certain indigenous chicken breeds from South Africa. Anim. Genet. Resour. 46, 25–32. doi: 10.1017/S2078633610000664
Guni, F., Mbaga, S., Katule, A., and Goromela, E. (2021). Performance evaluation of Kuroiler and Sasso chicken breeds reared under farmer management conditions in highland and lowland areas of Mvomero district, Eastern Tanzania. Trop. Anim. Health Product. 53:245. doi: 10.1007/s11250-021-02693-4
Gura, S. (2007). Livestock Genetics Companies. Concentration and Proprietary Strategies of an Emerging Power in the Global Food Economy. League for Pastoral Peoples and Endogenous Livestock Development. Ober-Ramstadt: Livestock Genetics Companies.
Hartung, C., Lerer, A., Anokwa, Y., Tseng, C., and Brunette, W. Borriello, G. (2010). “Open data kit: tools to build information services for developing regions,” in Proceedings of the 4th ACM/IEEE International Conference on Information and Communication Technologies and Development (New York, NY: Association for Computing Machinery), 1–12.
Hassen, H., Neser, F., De Kock, A., and Marie-Köster, V. (2006). Growth performance of indigenous chickens under intensive management conditions in Northwest Ethiopia. South Afri. J. Anim. Sci. 36, 71–73. doi: 10.13140/2.1.1701.9522
Henderson, C. R. (1975). Best linear unbiased estimation and prediction under a selection model. Biometrics 1975, 423–447. doi: 10.2307/2529430
Isenberg, D. (2007). Keggfarms (India)-Which came first, the Kuroiler or the Kegg. Harv. Bus. Sch. Case Study 2007, 9–807. Available at: https://www.hbs.edu/faculty/Pages/item.aspx?num=33933 (accessed November 30, 2023).
Jorjani, H., Philipsson, J., and Mocquot, J. (2001). Interbull guidelines for national and international genetic evaluation systems in dairy cattle with focus on production traits. Interbull Bull. 28, 1–27. Available at: https://journal.interbull.org/index.php/ib/issue/view/24 (accessed November 30, 2023).
Karamchedu, A., and Syndicus, I. (2022). Identifying economic and financial drivers of industrial livestock production-the case of the global chicken industry. Tiny Beam Fund 2022:40548. doi: 10.15868/socialsector.40548
Kebede, F. G., Derks, M. F., Dessie, T., Hanotte, O., Barros, C. P., Crooijmans, R. P., et al. (2024). Landscape genomics reveals regions associated with adaptive phenotypic and genetic variation in Ethiopian indigenous chickens. BMC Genom. 25:284. doi: 10.1186/s12864-024-10193-6
Kebede, F. G., Komen, H., Dessie, T., Alemu, O. H., and Ja, B. (2021). Species and phenotypic distribution models reveal population differentiation in ethiopian indigenous chickens. Front. Genet., 12:723360. doi: 10.3389/fgene.2021.723360
Kebede, F. G., Komen, H., Dessie, T., Hanotte, O., Kemp, S., Alemu, S. W., et al. (2023). Agroecologies defined by species distribution models improve model fit of genotype by environment interactions to identify the best performing chicken breeds for smallholder systems. Front. Sustain. Food Syst. 7:1305799. doi: 10.3389/fsufs.2023.1305799
Knap, P., and Su, G. (2008). Genotype by environment interaction for litter size in pigs as quantified by reaction norms analysis. Animal 2:1742. doi: 10.1017/S1751731108003145
Lan, X., Hsieh, J. C., Schmidt, C. J., Zhu, Q., and Lamont, S. J. (2016). Liver transcriptome response to hyperthermic stress in three distinct chicken lines. BMC Genom. 17:955. doi: 10.1186/s12864-016-3291-0
Lara, L. J., and Rostagno, M. H. (2013). Impact of heat stress on poultry production. Animals 3, 356–369. doi: 10.3390/ani3020356
Lin, C.-S., Binns, M. R., and Lefkovitch, L. P. (1986). Stability analysis: where do we stand?. Crop Sci. 26, 894–900. doi: 10.2135/cropsci1986.0011183X002600050012x
Lozano-Jaramillo, A., Alemu, S., Dessie, T., Komen, H., and Bastiaansen, J. (2019). Using phenotypic distribution models to predict livestock performance. Sci. Rep. 9, 1–11. doi: 10.1038/s41598-019-51910-6
Magothe, T., Okeno, T., Muhuyi, W., and Kahi, A. (2012). Indigenous chicken production in Kenya: I. Current status. World's Poult. Sci. J. 68, 119–132. doi: 10.1017/S0043933912000128
Mayorga, E. J., Renaudeau, D., Ramirez, B. C., Ross, J. W., and Baumgard, L. H. (2019). Heat stress adaptations in pigs. Anim. Front. 9, 54–61. doi: 10.1093/af/vfy035
Mazzi, C. M., Ferro, J. A., Ferro, M. I. T., Savino, V. J. M., Coelho, A. A. D., and Macari, M. (2003). Polymorphism analysis of the hsp70 stress gene in Broiler chickens (Gallus gallus) of different breeds. Genet. Mol. Biol. 26, 275–281. doi: 10.1590/S1415-47572003000300010
MoA (1998). Agro-Ecological Zones of Ethiopia. Ministry of Agriculture. Natural Resources Management & Regulatory Department. Addis Ababa, Ethiopia.
Mukherjee, B., Ko, Y.-A., Vanderweele, T., Roy, A., Park, S. K., and Chen, J. (2012). Principal interactions analysis for repeated measures data: application to gene-gene and gene-environment interactions. Statist. Med. 31, 2531–2551. doi: 10.1002/sim.5315
Oliveira, D., Lourenco, D., Tsuruta, S., Misztal, I., Santos, D., De Araújo, N. E. T. O., et al. (2018). Reaction norm for yearling weight in beef cattle using single-step genomic evaluation. J. Anim. Sci. 96, 27–34. doi: 10.1093/jas/skx006
Olivoto, T., Lúcio, A. D., Da Silva, J. A., Marchioro, V. S., De Souza, V. Q., and Jost, E. (2019a). Mean performance and stability in multi-environment trials I: combining features of AMMI and BLUP techniques. Agron. J. 111, 2949–2960. doi: 10.2134/agronj2019.03.0220
Olivoto, T., Lúcio, A. D., Da Silva, J. A., Sari, B. G., and Diel, M. I. (2019b). Mean performance and stability in multi-environment trials II: selection based on multiple traits. Agron. J. 111, 2961–2969. doi: 10.2134/agronj2019.03.0221
Olivoto, T., and Lúcio, A. D. C. (2020). metan: an R package for multi-environment trial analysis. Methods Ecol. Evol. 11, 783–789. doi: 10.1111/2041-210X.13384
OSU Breeds of Livestock (2024). 13 October 2024 ed. Stillwater, OK: Department of Animal and Food Sciences, Oklahoma State University.
Passarelli, S., Ambikapathi, R., Gunaratna, N. S., Madzorera, I., Canavan, C. R., Noor, A. R., et al. (2020). A chicken production intervention and additional nutrition behavior change component increased child growth in Ethiopia: a cluster-randomized trial. J. Nutr. 150, 2806–2817. doi: 10.1093/jn/nxaa181
Phillips, S. J., Anderson, R. P., Dudík, M., Schapire, R. E., and Blair, M. E. (2017). Opening the black box: an open-source release of Maxent. Ecography 40, 887–893. doi: 10.1111/ecog.03049
Piepho, H., and Möhring, J. (2005). Best linear unbiased prediction of cultivar effects for subdivided target regions. Crop Sci. 45, 1151–1159. doi: 10.2135/cropsci2004.0398
Piepho, H.-P. (1994). Best linear unbiased prediction (BLUP) for regional yield trials: a comparison to additive main effects and multiplicative interaction (AMMI) analysis. Theoret. Appl. Genet. 89, 647–654. doi: 10.1007/BF00222462
Powell, O., Mrode, R., Gaynor, R. C., Johnsson, M., Gorjanc, G., and Hickey, J. M. (2021). Genomic evaluations using data recorded on smallholder dairy farms in low-to middle-income countries. JDS Commun. 2, 366–370. doi: 10.3168/jdsc.2021-0092
Purchase, J., Hatting, H., and Van Deventer, C. (2000). Genotype × environment interaction of winter wheat (Triticum aestivum L.) in South Africa: II. Stability analysis of yield performance. South Afri. J. Plant Soil 17, 101–107. doi: 10.1080/02571862.2000.10634878
Resende, M. D. (2007). SELEGEN-REML/BLUP: sistema estatístico e seleção genética computadorizada via modelos lineares mistos. Colombo: Embrapa Florestas.
Rincent, R., Malosetti, M., Ababaei, B., Touzy, G., Mini, A., Bogard, M., et al. (2019). Using crop growth model stress covariates and AMMI decomposition to better predict genotype-by-environment interactions. Theoret. Appl. Genet. 132, 3399–3411. doi: 10.1007/s00122-019-03432-y
Rodrigues, P. C., Malosetti, M., Gauch Jr, H. G., and Van Eeuwijk, F. A. (2014). A weighted AMMI algorithm to study genotype-by-environment interaction and QTL-by-environment interaction. Crop Sci. 54, 1555–1570. doi: 10.2135/cropsci2013.07.0462
Rodrigues, P. C., Monteiro, A., and Lourenço, V. M. (2016). A robust AMMI model for the analysis of genotype-by-environment data. Bioinformatics 32, 58–66. doi: 10.1093/bioinformatics/btv533
Ross, J., Hale, B., Gabler, N., Rhoads, R., Keating, A., and Baumgard, L. (2015). Physiological consequences of heat stress in pigs. Anim. Prod. Sci. 55, 1381–1390. doi: 10.1071/AN15267
Sæbø, S., and Frigessi, A. (2004). A genetic and spatial Bayesian analysis of mastitis resistance. Genet. Select. Evol. 36, 1–16. doi: 10.1186/1297-9686-36-5-527
Sa'diyah, H., and Hadi, A. F. (2016). AMMI Model for yield estimation in multi-environment trials: a comparison to BLUP. Agricult. Agricult. Sci. Proc. 9, 163–169. doi: 10.1016/j.aaspro.2016.02.113
Safalaoh, A. (2001). Village chicken upgrading programme in Malawi. World's Poult. Sci. J. 57, 179–188. doi: 10.1079/WPS20010013
Shukla, G. (1972). Some statistical aspects of partitioning genotype environmental components of variability. Heredity 29, 237–245. doi: 10.1038/hdy.1972.87
Stroup, W., and Mulitze, D. (1991). Nearest neighbor adjusted best linear unbiased prediction. Am. Statist. 45, 194–200. doi: 10.1080/00031305.1991.10475801
Sultan, S. E. (2021). Phenotypic plasticity as an intrinsic property of organisms. Phenot. Plast. Evol. 2021:2. doi: 10.1201/9780429343001-2
Tadelle, D., Alemu, Y., and Peters, K. J. (2000). Indigenous chickens in Ethiopia: genetic potential and attempts at improvement. World's Poult. Sci. J. 56, 45–54. doi: 10.1079/WPS20000005
Tiezzi, F., De Los Campos, G., Gaddis, K. P., and Maltecca, C. (2017). Genotype by environment (climate) interaction improves genomic prediction for production traits in US Holstein cattle. J. Dairy Sci. 100, 2042–2056. doi: 10.3168/jds.2016-11543
Van Arendonk, J., Bink, M. M., Peeters, K., Visser, B., Duijvesteijn, N., and Van As, P. (2019). “Investments in breeding technologies and organization to meet global needs,” in Proceedings of the 23rd AAABG Conference (Perth: AAABG).
Van Eeuwijk, F. A., Bustos-Korts, D. V., and Malosetti, M. (2016). What should students in plant breeding know about the statistical aspects of genotype × environment interactions? Crop Sci. 56, 2119–2140. doi: 10.2135/cropsci2015.06.0375
Veerkamp, R., and Goddard, M. (1998). Covariance functions across herd production levels for test day records on milk, fat, and protein yields. J. Dairy Sci. 81, 1690–1701. doi: 10.3168/jds.S0022-0302(98)75736-4
Wondmeneh, E., Van Der Waaij, E., Tadelle, D., Udo, H., and Van Arendonk, J. (2014). Adoption of exotic chicken breeds by rural poultry keepers in Ethiopia. Acta Agric. Scand. A Anim. Sci. 64, 210–216. doi: 10.1080/09064702.2015.1005658
Wricke, G. (1964). Zur berechnung der okovalenz bei sommerweizen und hafer. Zeitschrift Fur Pflanzenzuchtung 52:127.
Wurzinger, M., Mirkena, T., and Sölkner, J. (2014). Animal Breeding Strategies in Africa: Current Issues and the Way Forward. Hoboken, NJ: Wiley Online Library.
Yang, R. C. (2007). Mixed-model analysis of crossover genotype-environment interactions. Crop Sci. 47, 1051–1062. doi: 10.2135/cropsci2006.09.0611
Keywords: smallholder systems, agroecology, mixed-effects models, AMMI models, improved breeds, yield stability, growth, genotype by environment interactions (GxE)
Citation: Kebede FG, Komen H, Dessie T, Hanotte O, Kemp S, Alemu SW and Bastiaansen JWM (2024) Multi-environment performance analysis identifies more productive and widely adapted chicken breeds for smallholder farmers. Front. Sustain. Food Syst. 8:1441295. doi: 10.3389/fsufs.2024.1441295
Received: 30 May 2024; Accepted: 22 November 2024;
Published: 13 December 2024.
Edited by:
Vassilios D. Litskas, Independent Researcher, Lefkosia, CyprusReviewed by:
Wuttigrai Boonkum, Khon Kaen University, ThailandThiruvenkadan Aranganoor Kannan, Tamil Nadu Veterinary and Animal Sciences University, India
Eva Armero, Polytechnic University of Cartagena, Spain
Copyright © 2024 Kebede, Komen, Dessie, Hanotte, Kemp, Alemu and Bastiaansen. This is an open-access article distributed under the terms of the Creative Commons Attribution License (CC BY). The use, distribution or reproduction in other forums is permitted, provided the original author(s) and the copyright owner(s) are credited and that the original publication in this journal is cited, in accordance with accepted academic practice. No use, distribution or reproduction is permitted which does not comply with these terms.
*Correspondence: Fasil Getachew Kebede, ZmFzaWxnZXRhY2hldzdAZ21haWwuY29t