- 1Business School, Yangzhou University, Yangzhou, China
- 2Center for Social Survey Research, Xuzhou Administration Institute, Xuzhou, China
- 3Ningbo Branch, The People’s Bank of China, Ningbo, China
- 4Office of Scientific Research, Party School of Kuitun Municipal Committee of CPC, Kuitun, China
Dietary diversity is an important indicator used to measure family dietary quality and food safety status. Against the backdrop of the transformation of China’s agricultural production model from a diversified, small-scale approach to specialization, this paper presents a comprehensive investigation into the complex interrelationship between crop specialization and dietary diversity. It employs a multi-method approach, integrating theoretical insights with empirical evidence. The analysis is based on a micro-survey of 866 rural households, utilizing mediation, moderation, and unconditional quantile regression model. The results indicated that crop specialization had a notable negative impact on dietary diversity, particularly among II part-time and mountainous households. Further analysis of the mechanisms involved reveals that income exerts a mediating effect, while education and market factors exert a moderating effect. Such factors may serve to mask or reduce the negative effects of crop specialization on dietary diversity. In the reality that the trend of production specialization is irreversible, government interventions to ensure dietary diversity and food security for rural households, particularly II part-time households and those in mountainous regions, can be implemented in three key areas: income support, education enhancement, and market access improvement.
1 Introduction
In the face of the complex international situation and extreme climates on the food safety of the global population, particularly the rural vulnerable people, how to ensure that rural residents achieve the “Zero Hunger” development goal has drawn unprecedented attention and concern (Chen et al., 2023; Langer et al., 2024; FAO, IFAD, UNICEF, WFP, WHO, 2024). China has 477 million farmers, and its rural area are the largest weak points of national economic and social growth, which are facing more severe food insecurity problems (Yu et al., 2017). According to the Agricultural and Rural Development Notes of World Bank, farmers’ crop production strategies are closely connected with their household food security (Hawkes and Ruel, 2008). Internationally, dietary diversity is an important indicator used to measure family dietary quality and food safety status (Jones et al., 2014; Tchuente et al., 2024), while crop production strategies in a broad sense mainly include two types of crop specialization and corresponding diversification. Among them, crop specialization reflects agricultural specialization from a micro perspective. As specialized labor division in China’s agricultural production is enhanced and the extent of marketization of agricultural products is improved, most farmers have gradually shifted from the conventional diversified production pattern to the specialized production of crops (Luo, 2017; Huang et al., 2019); a series of policies promulgated by the Chinese government are also actively encouraging farmers to engage in specialized production of crops. There is no denying that from the perspective of classical economics, emerging classical economics, and traditional agricultural economic theories (Yang and Ng, 1993; Schultz, 1993), crop specialization can strengthen farmers’ production efficiency and income via the effect of “practice makes perfect.” Thus, this paper mainly focuses on the following questions:
i. In the context of China’s agricultural transformation towards specialization, what impact does crop specialization have on farmers’ dietary diversity?
ii. What role does farmers’ income play in this?
iii. Are there any other factors besides farmers’ income that play a role in this?
Currently, China has completely realized the poverty alleviation target with “do not worry about food” as the central requirement, but “eating enough” is insufficient to guarantee that the residents have enough nutrition and health, and a balanced and diverse diet plays a key role in this (Pellegrini and Tasciotti, 2014). According to the classic thesis proposed by Ellis earlier, a diversified production pattern is an optimal approach for small farmers in developing countries to guarantee the survival and security of their families in the face of severe external shocks (Ellis, 1998). In practice, production diversification is regarded as the agricultural development strategy in developing countries like Thailand, Zambia, and Bangladesh (Mofya-Mukuka and Hichaambwa, 2018; Rahman, 2009; Kasem and Thapa, 2011). Consequently, the academic community mainly starts from the view-angle of crop production diversification to analyze how crop production strategies influence the dietary diversity of farmers. Therefore, this paper incorporates both types of crop production strategies into the research scope and systematically reviews the relevant literature on how crop specialization or diversification affect farmers’ dietary diversity. Pellegrini and Tasciotti (2014) and Jones et al. (2014) found that while the vast majority of farmers rely on crop production and management to maintain household livelihood security and dietary diversity, few studies have examined the relationship between the two. Given this, they examined the impact of crop diversification on farmers’ dietary diversity based on cross-sectional data of farmers in eight developing countries, revealing that crop diversification has a significant positive impact on farmers’ dietary diversity. In other words, crop specialization reduces dietary diversity. Subsequently, the same conclusion was reached in empirical research by Ecker (2018) based on the 2006 and 2013 residential living survey data in Ghana, Tobin et al. (2019) based on the cross-sectional data of farmers in 11 sub-Saharan African countries, Sekabira and Nalunga (2020) based on the three rounds of farmer survey data in Uganda from 2009 to 2012, and Isbell et al. (2024) based on the Demographic and Health Survey (DHS) data from 10 sub-Saharan African countries.
Nevertheless, the associations between crop diversity and dietary diversity have also been found to vary significantly by country (Nandi et al., 2021). For example, Sibhatu et al. (2015) based on the farmer household survey data from 4 developing countries, found that while crop diversification positively affected the dietary diversity of farmers in Indonesia and Malawi, it had no impact on the dietary diversity of farmers in Kenya and Ethiopia, This revealed that the positive relationship between crop diversification and farmers’ dietary diversity is not universal. In this regard, Berti (2015) pointed out that the selection of the measurement method of crop diversification in Sibhatu et al.’s paper was the major cause of the uncertainty of the relationship between the two. Sibhatu and Qaim (2018) further used several measurement methods such as a simple counting method and a food group score method to conduct empirical tests. They clarified that different measurement methods indeed induce uncertainty in the impact of crop diversification or specialization on farmers’ dietary diversity. Other scholars have found through research on farmer household survey data in Kenya and Burkina Faso that the correlation between crop specialization and farmers’ dietary diversity is not negative or uncertain, but irrelevantt (Ng’endo et al., 2015; Lourme-Ruiz et al., 2016). In addition, some studies have shown that crop specialization can improve farmers’ dietary diversity. For example, Chinnadurai et al. (2016) using data from a national sample survey in India, noticed that the diversified production of vegetable crops exerts a significant adverse influence on farmers’ dietary diversity, indicating that the specialization of vegetable crop production will make farmers’ diets more diversified. Argyropoulou (2016) focused on a group of children in rural northern Ghana, and the results also showed that crop specialization can significantly enrich the dietary diversity of rural children.
In general, there are rich research results on the relationship between crop specialization (or diversification) and farmers’ dietary diversity, providing an important reference for this paper, but there are still some deficiencies. First, the research conclusions drawn by various literature using micro-data of farmers in different countries or different index measurement methods still vary dramatically. No consensus has been reached on whether crop specialization or diversification is the best strategy to ensure farmers’ dietary diversity and achieve the “Zero Hunger” development goal, so further discussions are needed. Second, effectively ensuring the dietary diversity of Chinese residents, who take up a high proportion of the global population, especially the Chinese rural residents, is crucial to the realization of the “Zero Hunger” development goal at the global level. However, in the context of China’s rapid agricultural transformation towards specialization, little research has explored the impact of crop specialization on farmers’ dietary diversity based on a sample of Chinese farmers. In recent literature, although Huang et al. (2019) analyzed the relationship between farmers’ planting and breeding diversity and farmers’ dietary diversity based on 395 farmer household survey data, unfortunately, they failed to further clarify how the specialization of crop production (or diversification) affects farmers’ dietary diversity. Third, as socialization and commercialization trends of agricultural production become more prominent in China and even in the world, farmers can influence the purchase of family food through the income obtained from the sale of agricultural products (Hawkes and Ruel, 2008). This unveils that income can play a certain indirect role in the process of crop specialization affecting farmers’ dietary diversity, but existing studies often solely pay attention to the direct relationship between the two and ignore the indirect role of income. Furthermore, education (Abokyi et al., 2023; Adugna et al., 2024) and market (Nandi et al., 2021; Kihiu and Kydd, 2021) also have important effects on dietary diversity, but the role of these factors in the relationship between crop specialization and dietary diversity have also been ignored in existing research. Finally, in the empirical strategy, the existing literature mainly applies the linear mean regression model to explore the average impact of crop specialization on farmers’ dietary diversity. There is no mention of the heterogeneity of the effects of crop specialization on farmers at different dietary diversity quantiles or different groups within a group under virtual translational transformations.
This paper intends to enrich the existing research from the three perspectives listed below. To begin with, with the micro-survey data of crop growers from the rural survey team of the Hubei Survey Team of the National Bureau of Statistics of China (HRST) as samples, this paper used Feasible Generalized Least Squares (FGLS) and Unconditional Quantiles Regression (UQR) estimation method to comprehensively examine the average effect of crop specialization on household dietary diversity and the heterogeneity of the impact across quantiles. Second, the farmers were grouped according to the terrain features of the villages and the production types of the farmers, and the grouped estimation and Seemingly Uncorrelated Model Test (SUEST) method was applied to analyze the realistic scenario of the disparity in the influence of crop specialization on dietary diversity of various groups among the farmers. Third, this research introduced farmers’ income, education and market as mediating or regulating variables in the theoretical analysis framework of sustainable livelihoods, and constructed a test model of mediating and moderating effects, thereby deeply exploring the internal impact mechanism and action path of crop specialization on farmers’ dietary diversity. The research in this study can provide decision-making reference for promoting the process of crop specialization in China, ensuring the food security of farmers and taking the lead in realizing the development goal of “Zero Hunger” of the United Nations.
The rest of the paper is organized as follows: Section 2 is the theoretical framework and research hypothesis of the research; Section 3 is the research design; Section 4 is the estimated results of the model; Section 5 is the discussion and presentation of the research shortcomings and future research directions; Section 6 is the conclusion.
2 Research hypotheses
The theoretical framework of sustainable livelihoods proposed by Scoones (1998) and the Department for International Development (DFID, 1999) provides a good analytical tool for understanding and analyzing how crop specialization affects farmers’ livelihoods. As suggested by sustainable livelihood theory, household livelihood outcomes are affected by household livelihood capital and livelihood strategies. This research argues that the agricultural production strategy of crop specialization will exert a direct or indirect impact on the dietary diversity of farmers’ livelihood outcomes, particularly for small farmers whose major livelihood is crop production and management. Moreover, the Agricultural and Rural Development Notes of World Bank claimed that the production of food crops for direct household consumption is the major way in which agricultural production activities influence farmers’ dietary diversity (Hawkes and Ruel, 2008), as farmers mainly eat crops directly produced by themselves (Lourme-Ruiz et al., 2016). Nevertheless, limited by the given resource endowments (labor, land, etc.), farmers can only conduct a limited range of production activities. In this way, when farmers specialize in the production and operation of one or several crops, it will have a “crowding-out effect” on the input of other crops, leading to a decline in the category of food produced by farmers for direct family consumption. This indicates that the absence of a diversified agricultural production system will reduce farmers’ dietary diversity (Sibhatu et al., 2015), and on the contrary, the rise in crop specialization extent will improve this diversity.
Furthermore, the Agricultural and Rural Development Notes of World Bank also suggested another way that agricultural production activities influence farmers’ household diversity, namely, the income drawn from selling agricultural products influences the purchase of family food (Hawkes and Ruel, 2008), but only if crop specialization can bring about more economic benefits for farmers (Sekabira and Nalunga, 2020; Habtemariam et al., 2021). Consequently, it remains a question whether crop specialization can improve farmers’ income. Based on the analytical framework of emerging classical economics (Yang and Ng, 1993; Schultz, 1993; Yang, 2000), the specialized development level of farmers’ crop production is divided into three phases: self-sufficiency, partial specialization, and complete specialization.
As shown in Figure 1 (where n represents the number of crops grown, F denotes an individual farmer), it is assumed that there are only four farmers who are both producers and consumers in the economic system, Each farmer chooses to grow four crops (to simplify the analysis, this paper does not consider agriculture production seasonality and multiple cropping issues) and, has a diversified consumption tendency to consume these four crops. In the self-sufficiency state of Figure 1a, each farmer consumes only the four crops he or she produces. At this time, the level of production specialization of each farmer is very low, there is no market in the whole society, farmers do not trade with each other, the family is in a closed-type small-scale peasant economy of “men farming and women weaving,” falling into the “low-level equilibrium trap.” According to emerging classical economics (Yang and Ng, 1993; Schultz, 1993; Yang, 2000), a low level of specialization corresponds to a low level of productivity and income. Under the partial specialization state manifested in Figures 1b,c, the number of crops grown by each farmer changed from 4 to 3 or 2. At this time, the level of production specialization of farmers has been lifted, the agricultural product trade market has grown from nothing, and the agricultural productivity of farmers and the income level of planting have been improved to a certain extent. In the state of complete specialization in Figure 1d, each farmer only grows one crop with a comparative advantage, and the production specialization of each farmer, the degree of marketization of the society, and the degree of economic integration have been dramatically enhanced. This is the same case for farmers’ agricultural productivity and planting income level, which have also increased. Thus, with the rising of the crop specialization extent, farmers will acquire higher income to purchase diverse food from the agricultural product market. Through this process, a “substitution effect” can be generated for the food given up by farmers due to specialized production, thereby continuing to maintain and improve the intake of diverse nutrients in the family.
From the above exploration of the “crowding-out effect” and the “substitution effect” of crop specialization on the number of food categories consumed by farmers, this paper makes the hypotheses below:
H1: The influence of crop specialization on farmers’ dietary diversity is not certain.
H2: Crop specialization can exert an indirect impact on farmers’ dietary diversity by improving farmers’ income.
In addition, since heterogeneity is common in social science research, even if different individual units in the same population have certain commonalities, there are still significant differences in their specific characteristics (Sun and Chen, 2019). Especially in the context of the continuous widening of development gaps between regions and groups in China, there are bound to be disparities in the livelihood capital or livelihood background of different groups within farmers, leading to the heterogeneous influence of crop specialization on the dietary diversity of different households. As pointed out by Sibhatu and Qaim (2018) and Habtemariam et al. (2021), the relationship between crop production and dietary diversity are mixed and context-specific. So, what factors are causing the difference in results? We believe that education and market are two important factors. Theoretically, increased education is expected to have an enhanced dietary knowledge and shape the food consumption habits of the households (Abokyi et al., 2023). Also, more educated households are more likely to use the internet and other sources such as mobile phones, and other communication methods to access more and relevant nutrition and health knowledge that could guide their food choices and consumptions (Hou et al., 2021). The market is another factor that can play an important role for farm households who act as both sellers and buyers of food and other agricultural commodities (Koppmair et al., 2017). On the one hand, increased market access and involvement in market activities enable smallholder farmers to sell a portion of their harvested crops and use the proceeds to acquire more diverse food (Hawkes and Ruel, 2008). On the other hand, residents who reside near markets have easier access to a wider variety of foods throughout the year (Morrissey et al., 2024), thereby regulating or altering the intensity of the impact of crop specialization on household dietary diversity. Therefore, this paper proposes the following hypothesis:
H3: There is significant heterogeneity in the effect of crop specialization on the dietary diversity of different households.
H4: Education and market accessibility have moderating effect on the relationship between crop specialization and dietary diversity, which can alter the intensity of the impact of crop specialization on dietary diversity.
To sum up, the conceptual framework for the relationship between crop specialization and farmers’ dietary diversity can be represented in Figure 2. This conceptual framework strictly complies with the core viewpoints of the theoretical framework of sustainable livelihoods and points out the directness, indirectness, and heterogeneity of the impact of farmers’ adoption of crop-specialized production strategies on household dietary diversity in the context of the transformation of agricultural production methods.
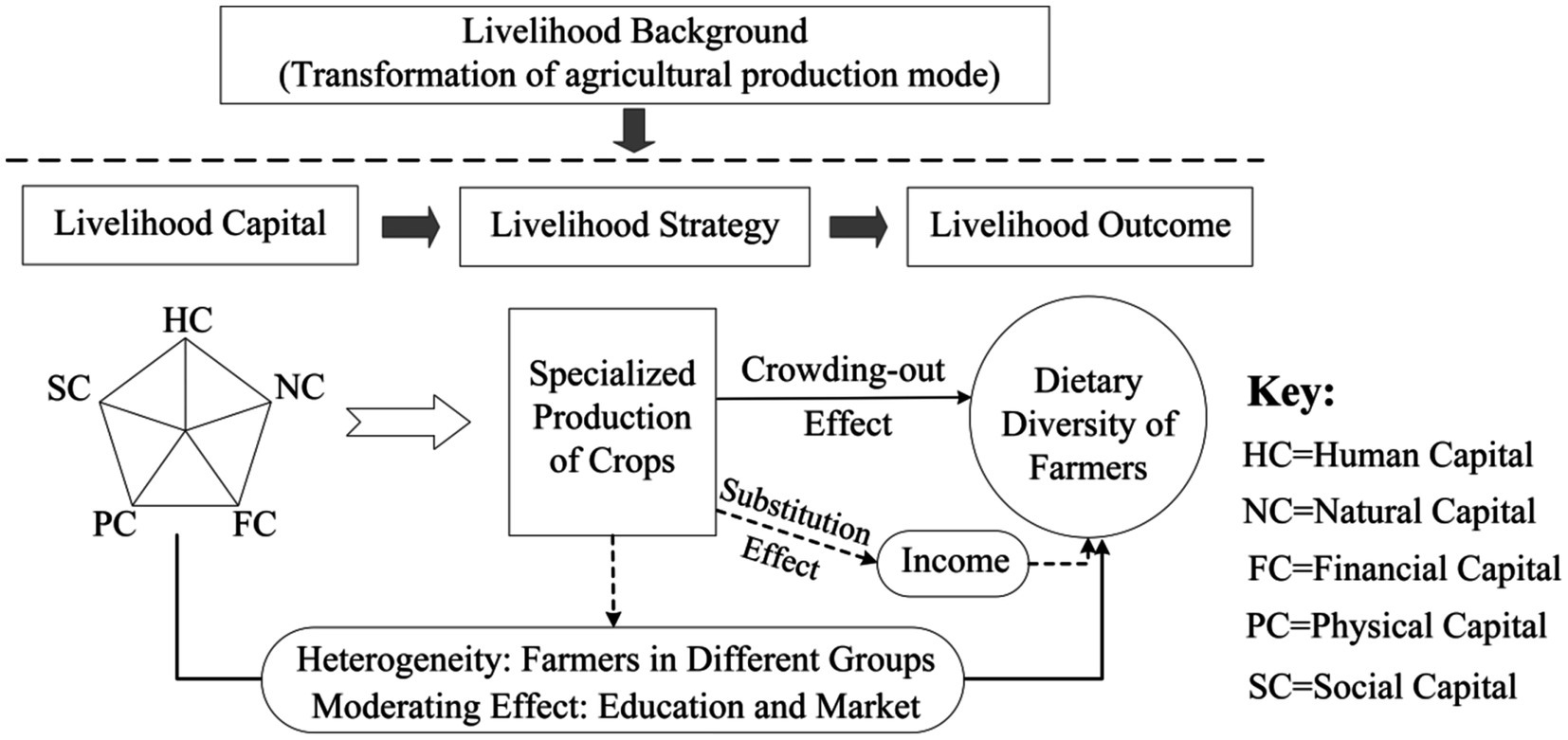
Figure 2. The conceptual framework for the association between crop specialization and dietary diversity.
3 Materials and methods
3.1 Data
This paper uses the rural household micro-survey data collected by HRST from 56 counties and cities in Hubei Province in 2016. Previous research has introduced the acquisition, contents, and management of this data (Chen and Ravallion, 1996), and used the data to analyze farmers’ nutritional intake (Sun et al., 2022). In this survey, stratified random sampling method and daily bookkeeping method were utilized to collect data. When selecting farmers, 1–7 villages in each county and 8–12 households in each village were selected. In 2016, a total of 2,564 households were investigated, and a wide range of data indicators were collected. Compared with other database, this survey data could address the “seasonal deviation” issue caused by the cyclical characteristics of agricultural production (Sibhatu and Qaim, 2017), and it could better address the “recall bias” issue that potentially exists when applying the 24-h retrospective approach or the food frequency approach to gather data.
The survey is based on the sampling method of the national population census, which includes all households in the sample box. However, the main purpose of this paper is to explore the impact of farmers’ livelihood strategies on their household livelihood outcomes, that is, the impact of crop specialization on household dietary diversity (Figure 2). For farmers who are no longer engaged in crop production, there is no relationship between their family dietary diversity and crop specialization. Therefore, in order to accurately identify the net effect and mechanism of crop specialization on household dietary diversity, this paper classifies farmers into four categories [Pure agricultural households, I part-time households, II part-time households, and Non-agricultural households refer to households where the proportion of income from the primary industry in their net income is (80%, 100%], (50%, 80%], (20%,50%], and [0,20%], respectively] based on the classification method adopted by the Ministry of Agriculture and Rural Affairs of China, firstly, Non-agricultural households in the database are excluded, followed by the exclusion of households where crop production income accounts for <50% of the household’s primary industry income. After multiple rounds of screening, 866 valid observation samples are finally obtained for this paper. In addition, we also illustrate the selection of the research sample through Figure 3.
According to the survey caliber of HRST, the types of crops planted by farmers included fall into 19 categories: wheat, rice, corn, sorghum, other grains, sweet potatoes, potatoes, other tubers, soybeans, other legumes, cotton, vegetables, melons and fruits, sugar crops, peanut, sesame, rapeseed, sunflower, and other oil crops. Table 1 shows the crop planting types and sown area of the sample farmers. In terms of the crop planting types of the sample farmers, the farmers who choose to grow vegetables are the most, accounting for 83.49% of the total number of sample farmers, followed by rice, rapeseed, corn, wheat, and other crops. Regarding the per capita crop sown area of the sample farmers, the per capita sown area of rice, wheat, corn, and other crops is larger, which are 0.81, 0.58, and 0.40 hectares, respectively, accounting for 53.73, 29.67, and 27.86% of the total sown area of household crops. For the vegetables that farmers generally plant, their per capita planting area and proportion to the total planting area of household crops are 0.04 hectares and 6.19%, respectively, revealing that most farmers currently plant a small number of vegetables mainly for self-sufficiency.
HRST collects food data (including self-produced food for self-use and food purchased from the market) according to food categories and by way of bookkeeping. Among them, the food categories included in this paper mainly include 10 broad categories and 40 specific categories. Table 2 demonstrates the food consumption of the sample farmers throughout the year. It can be seen that grain crops are their main food source, and the average annual consumption of the households reaches 442.51Kg, taking up 39.23% of the total consumption. Concerning the total number of food categories consumed by the sample farmers, the average number of food categories consumed by the households per year is 21.29. Moreover, from the grouping situation, the variance of the categories of food consumed by farmers in various village terrain feature groups and production type groups is significant at the 1 and 5% levels, revealing significant differences in the number of food categories consumed by different groups within farmers. Specifically, the number of food categories consumed by farmers in mountainous area and I part-time households is below that of farmers in plains and hills, as well as pure agricultural households and II part-time households.
3.2 Model
3.2.1 Mediation effect model
According to the previous theoretical analysis and research hypothesis, and referring to the existing research of Argyropoulou (2016) and Habtemariam et al. (2021), this paper sets up the following measurement models to test the direct and mediating effects of crop specialization, farmers’ income and farmers’ dietary diversity:
In Equations 1–3, is the crop specialization level of the farmer; , represent the dietary diversity and total household disposable income of the farmer; , , , , are the five major livelihood capitals, namely human capital, natural capital, physical capital, financial capital and social capital, which affect farmers’ livelihood results (referring to farmers’ dietary diversity in this article) in the DFID sustainable livelihood theoretical analysis framework; where, , , are the parameters to be estimated, respectively, , and are the random error terms of each model. Regarding the test method and judgment criteria of the mediation effect, this paper adopts the Causal Steps Approach proposed by Baron and Kenny (1986) and is widely used for analysis, and the specific analysis process will not be repeated here.
In the process of investigating the impact of crop specialization on farmers’ dietary diversity and its mechanism, the problem of data heteroscedasticity is the primary measurement problem. In order to overcome the estimation bias caused by using traditional Ordinary Least Squares (OLS) in the presence of heteroscedasticity, according to the suggestion of Reed and Ye (2011), Feasible Generalized Least Squares (FGLS) was used to estimate the above benchmark model. This method can allow the existence of heteroscedasticity and autocorrelation in the data, which is very suitable for the analysis of farmer household micro-survey data.
3.2.2 Moderation effect model
In order to test whether education and market can moderate the impact of crop specialization on farmers’ dietary diversity, this paper constructs the following moderation effect model by adding interaction terms between education and crop specialization, and market and crop specialization based on Equation 1:
In Equations 4, 5, and are the education level and market accessibility of the farmer, respectively. The meaning of is same as Equation 1, and includes the five major livelihood capital in Equation 1. and are the parameters to be estimated, and and are the random error terms of each model. is the interaction term between education and crop specialization, and is the interaction term between market and crop specialization. If the parameters to be estimated for these interaction terms are statistically significant, it indicates the presence of a moderating effect. The estimation method for the aforementioned model remains FGLS.
3.2.3 Unconditional quantile regression model
Both OLS estimation and FGLS estimation are conditional mean regression, which describe only the average influences among variables, and cannot deeply analyze the heterogeneity influence of crop specialization on farmers with different dietary diversity levels. In order to solve this problem, this paper further uses the Unconditional Quantile Regression (UQR) model proposed by Firpo et al. (2009) to comprehensively investigate the effect of crop specialization on the quantile of the unconditional distribution of household dietary diversity under virtual location Shift. UQR assumes that each influencing factor including crop specialization (that is, all independent variable in Equation 1) is exogenous, and the basic definition is as follows:
In Equation 6, is the recentralization influence function corresponding to the quantile of and represents the unconditional quantile of the level of dietary diversity of farmers.
3.3 Variable
3.3.1 Dependent variable
Farmers’ dietary diversification is the dependent variable. Referring to the approach of Min et al. (2019), the Shannon Index (SI) was used as a variable to measure dietary diversity at the household level. According to the definition, assuming that the total number of food types (including self-produced and purchased food) evenly consumed by household is , then the Shannon index of household is:
In Equation 7, ( ) represents the proportion of the consumption of the nth food category to the total food consumption of farmer in the whole year, and its logarithm is , in which the larger the , the richer and more diverse the farmers’ diets, and the higher the level of dietary diversity.
3.3.2 Independent variable
Crop specialization is the independent variable. Currently, the academic community utilizes the Herfindahl Index (HI) to reflect agricultural specialization or diversification (Mofya-Mukuka and Hichaambwa, 2018; Chinnadurai et al., 2016). The HIi uses the square sum of the proportion of the sown area of each crop in farmer to the total sown area to express the level of crop specialization (see Equation 8). The value range of is between 0 and 1, and the smaller the value, the lower the level of crop specialization; otherwise, the greater the value. If the value of is 1, it means that the farmer only grows one type of crop.
3.3.3 Mediating variable
The farmer’s income is the mediating variable, and it may be collected directly from the farmer’s household survey data. It should be emphasized that the income of farmers includes cash income (before deducting production expenses), total income (before deducting production costs), and disposable income, according to the statistical standards of the HRST’s rural survey team. Among these, disposable income, also known as net income, can better reflect farmers’ living standards and consuming ability. As a result, the statistical quality of disposable income is used as a mediating variable in this work.
3.3.4 Moderating variables
Education and market are the moderating variables in this paper. Among them, education is measured by the education level of the household head. Although the education level of the mother might be a better indicator (Abokyi et al., 2023), research has also demonstrated that the educational level of the household head similarly exerts a significant influence on household livelihood strategies and outcomes (Vo, 2024). Market is proxied by the distance from home to the nearest market, and it is unquestionable that a shorter distance to the market facilitates easier access to a more diverse array of foods, thereby promoting dietary diversity (Nandi et al., 2021; Kihiu and Kydd, 2021).
3.3.5 Control variables
According to the analytical framework of DFID sustainable livelihood theory, this study uses the five major livelihood capitals of farmers as other control variables that affect dietary diversity in farmers’ livelihood outcomes. Referring to the practice of existing research (Gu et al., 2016), this paper selects indicators such as the size of the permanent resident population to represent the human capital status of the farmer, and the quantity of household durable goods and the type of toilets to represent the physical capital. The total area of farmland actually operated represents natural capital, and the status of household deposits and loans, as well as gift expenditures such as family weddings and funerals, represent the financial capital and social capital owned by farmers. The description and descriptive statistics of all variables are shown in Table 3.
4 Results
4.1 Baseline regression
Using Stata17.0 software, the estimation results of the benchmark model are presented in Table 4. During the estimation process, regression 1 in Table 4 only examines the marginal impact of the crop specialization level, the core independent variable on farmers’ dietary diversity and regression 2 involves other control variables. Evidently, the estimated coefficients of the sample farmers all reach a significant negative level. This means that the crop specialization of the sample farmers significantly adversely affects farmers’ dietary diversity. In other words, the greater the extent of farmers’ crop specialization, the smaller the extent of farmers’ dietary diversity, which echoes relevant research (Jones et al., 2014; Tobin et al., 2019). Regression 3–5 reported the estimation results of farmers’ dietary diversity at the low quantile (Q25), the middle quantile (Q50), and the high quantile (Q75) under the unconditional quantile regression, respectively. It is clear that except for the high quantile (Q75), crop specialization has a significant negative effect on farmers’ dietary diversity at both the low quantile (Q25) and the middle quantile (Q50). Moreover, the absolute value of the estimated coefficient is larger at the low quantile (Q25), suggesting the more prominent adverse influence of crop specialization on farmers with low levels of dietary diversity. That is, from the perspective of dietary diversity, crop specialization is a production decision detrimental to farmers with low dietary diversity levels.
As for other control variables, farmland size had a significant positive impact on the dietary diversity of farmers at the 1% statistical level in both FGLS mean regression and UQR. Clearly, the larger the farmland management area of farmers, the higher the level of farmers’ dietary diversity, indicating that large-scale land management is indeed a vital way to enhance farmers’ dietary diversity. The livelihood capital variables such as population size and toilet type are only significant in the FGLS mean regression or the low (Q25) and middle quantiles (Q50), and the impact on farmers in the high quantiles (Q75) is not statistically significant. For farmers with a higher level of dietary diversity, their dietary diversity is no longer affected by the above-mentioned changes in livelihood capital.
4.2 Robustness testing and endogeneity treatment
In order to ensure the robustness of the above estimation results, this part adopts the method of replacing the core independent variable, the dependent variable and the estimation model to test. Specifically, referring to existing research, the maximization index (MI) was used to replace the HI to measure the level of crop specialization as the core independent variable; adopt a classification method for the dependent variable, and replace the household dietary diversity with a dichotomous variable with a mean boundary (a value greater than or equal to the mean is set to 1, and a value less than the mean is set to 0), and the Probit model is selected to estimate it according to the processing method of the dependent variable. Table 5 reports the results of the robustness test of the substitution variables and model.
The estimation results of Table 5 regression 1, which only replaces the HI, show that the coefficient value of the MI is consistent with the benchmark model estimation results in terms of sign and significance, indicating that crop specialization does have a negative impact on farmers’ dietary diversity. Regression 2 simultaneously replaces crop specialization, household food diversity, and regression models, and it should be pointed out that the coefficient values directly estimated by the probit model do not have economic meanings in the usual regression sense, such as OLS or FGLS. Therefore, referring to the practice of previous studies (Greene, 2018), regression 3 gives the marginal effect value transformed by the Probit model [regression result (2)], and the results show that after replacing variables and models, crop specialization also had a negative impact on farmers’ dietary diversity at a significant level of 5%. The estimation results (4–6) of the UQR also showed that, in addition to the high quantile (Q75), crop specialization has a significant negative effect on farmers’ dietary diversity at both the low quantile (Q25) and the middle quantile (Q50), and the absolute value of the estimated coefficient is larger at the low quantile (Q25). To sum up, it can be seen that the estimation results of the benchmark model will not change with the changes of the independent variable, the measurement methods of the dependent variable, and the model, indicating that the main conclusions above are robust to a certain extent.
In addition, it is undeniable that endogeneity is an important measurement issue that cannot be ignored in empirical research. Generally speaking, the sources of endogeneity mainly include three aspects: measurement error, omitted variables and simultaneous causality. This article uses HRST’s micro-survey data of farmer households. The basic data of this survey comes from survey households’ bookkeeping and survey personnel’s household visits. At the end of each quarter, the investigators will code, enter and review the sample information, accounting data and questionnaire data of the surveyed households, which effectively avoids measurement errors during the data collection process, and the data quality is high; moreover, the results of the robustness test show that the measurement method of replacing the core independent variable and the dependent variable will still not affect the main conclusions of the benchmark regression part, so this part can eliminate the measurement error type endogeneity problem.
For the endogeneity problem caused by omitted variables and simultaneous causality, this paper uses the bounding argument method (Oster, 2019) and simultaneous equation method (Wang, 2016) to test, and the results are shown in Table 6. Among them, the test results of omitted variables show that the -value of the ratio between the selection of non-observable variables and the selection of observable variables is −7.4048, and its absolute value is >1. According to the suggestions put forward by Oster (2019), it can be considered that the model in this paper does not have endogeneity problems caused by missing variables. The results of the simultaneous causality test show that the coefficient of the residual value is significant at the 1% level. According to the test criteria of the simultaneous equation method, it is shown that crop specialization and diet diversity are causality to each other, that is, the model in this paper has the endogeneity problem caused by the simultaneous causality.
In order to overcome the estimation bias caused by endogenous, the two-stage least squares method (IV-2SLS) and generalized quantile regression method (IV-GQR) with the introduction of instrumental variables were used to estimate the benchmark model. This paper selected the aggregation data —crop specialization index at village level— as an instrumental variable. It is one of the most common ideas to use instrumental variables from regional agglomeration data to solve endogenous problems (Porter, 2024). Before using instrumental variable analysis, the effectiveness of village-level crop specialization of instrumental variable should be tested, including underidentification test and weak instrumental variable test. The test results are shown in Table 7 (lower part). According to the validity criteria of instrumental variables, there is no problem of unrecognizable and weak instrumental variables in the selected instrumental variables. Table 7 (upper part) reports the estimated results of IV-2SLS and IV-GQR. It can be observed that after overcoming the endogeneity problem, the estimated coefficient values of crop specialization are always statistically significant and negative on mean regression (FGLS or IV-2SLS), low quantile regression (Q25), and middle quantile regression (Q50), which suggests that crop specialization does have a negative impact on dietary diversity.
4.3 Heterogeneity analyses based on grouping of farmers
The above confirms that crop specialization has a significant negative impact on dietary diversity, especially for low quantile farmers. In the context of the continuous expansion of development imbalance between regions and groups in China, further analysis of the impact of crop specialization on the dietary diversity of different groups within farmers has important reference value for the government to formulate targeted policy measures. Therefore, this part adopts the grouping estimation method to compare and analyze the farmers according to the production type (including pure agricultural households, I part-time households, II part-time households, and non-agricultural households), and village terrain features (including plains, hills, and mountainous areas). It should be noted that, since the differential impact of crop specialization is only judged by simply comparing the estimated value of the coefficient and its significance level between different farmer groups, and lack of statistical test support, on the basis of grouping estimation, the method of Seemingly Uncorrelated Model Testing (SUEST) model was used to test the difference of regression coefficients. The results of group estimation and coefficient difference test are shown in Table 8.
The regressions 1–3 in Table 8 show the estimated results grouped by farmers’ production types. The results show that crop specialization has a significant negative impact on I part-time households and II part-time households at the statistical level of 5 and 1%, respectively, but the effect on pure agricultural households is not significant. As far as the absolute value of the estimated coefficient is concerned, the absolute value of the estimated coefficient of the II part-time households group is larger. From the test results of the coefficient difference between groups, the group difference between II part-time households and pure agricultural households passed the 5% statistical test, which indicates that crop specialization has a stronger negative effect on the dietary diversity of II part-time farmers than the pure agricultural household group.
In this paper, dietary diversity is measured based on the dietary status of the resident population in the household. For II part-time households, also known as non-agricultural part-time households, the resident population of the family is the left-behind population (mainly including left-behind women, children, and the elderly), whose are relatively disadvantaged groups arising from the rapid development of China’s new urbanization and industrialization (Min et al., 2019). One possible reason for the greater negative impact of crop specialization on their dietary diversity is that, compared with pure agricultural households, the education level (including nutritional knowledge) of the resident population such as women, children and the elderly in II part-time households is not high. Education changes the intensity of the impact of crop specialization on dietary diversity, that is, education plays a moderating effect in it. Of course, further testing is needed to verify whether education does indeed have a moderating effect.
Regression 4–6 gives the estimation results grouped by village terrain features. It can be seen that the estimation coefficient in mountainous areas is significant at the level of 1%, and the absolute value is also the largest. At the same time, from the results of inter-group coefficient difference test, mountains and plain, mountain and hills all passed the statistical test, which indicates that crop specialization has different effects on the dietary diversity of farmers with different village terrain features, and the negative impact on mountainous areas is the largest. A possible reason is that markets play a moderating role. The typical feature of mountainous areas is that the transportation is not convenient enough, the marketization level of agricultural products is not high, and the distance from home to market is also far, which makes it difficult for farmers in mountainous areas to conveniently obtain various kinds of food through the market. As a result, mountain farmers cannot buffer or mitigate the negative impact of crop specialization on dietary diversity through the market as effectively as those in plain and hills areas. Similarly, whether the market has a moderating effect still requires further examination.
4.4 Mechanism analyses
After identifying the causal relationship between crop specialization and dietary diversity, as well as the heterogeneity of its impact, in order to further clarify the complex relationship and underlying mechanisms between the two, we will next test the mediating effect of income and the moderating effects of education and market based on the model established in Part Three. Firstly, the Causal steps approach was used to test whether the income of farmers played a mediating role in the impact of crop specialization on their dietary diversity. The results are shown in Table 9 (the upper part), and the regression results (1–2) in Table 4 need to be discussed together in the analysis process.
First of all, without considering the mediating variable, it can be seen from the regression results (1–2) in Table 4 that the regression coefficient of crop specialization is statistically significant whether or not the control variable is added, so it can be transferred to the follow-up test. Then, the Equations 2, 3 are estimated and the coefficients and are tested in turn, and the regression results (1–2) in Table 9 are the estimation of the Equation 2. The results show that the regression coefficient of crop specialization is significantly positive at the 1% level whether or not the control variable is added, indicating that crop specialization has a significant positive impact on farmers’ in9667come. The regression result (3) in Table 9 is an estimation of Equation 3, indicating that is still significantly positive at the 1% level, so the complete mediation effect test can be carried out at this time. According to the judgment criterion of mediating effect, the regression coefficient of crop specialization in regression result (3) is significantly positive at the level of 1%, indicating that farmers’ income has played a partial mediating effect in the impact of crop specialization on farmers’ dietary diversity. The sign of is opposite to that of , so the mediating effect of farmers’ income here is a masking effect, that is, farmers’ income can alleviate the negative impact of crop specialization on farmers’ dietary diversity through indirect effects.
In addition, although the Causal steps approach is the most popular analytical method to test the mediation effect, in recent years, it has also been criticized and questioned more and more for rationality and validity. Some scholars even called for the application of the nonparametric Bootstrap method with higher test potency instead of the Causal steps approach. Therefore, in order to ensure the reliability of the results of the mediation effect test, the nonparametric Bootstrap method was further used to test the masking effect of farmers’ income. According to the different ways of estimating confidence intervals, the nonparametric Bootstrap method can be divided into the nonparametric percentile Bootstrap method and the bias-corrected nonparametric percentile Bootstrap method. In this section, the number of repeated sampling is set to 5,000 times, the confidence interval is 95%, the estimation results of the nonparametric percentile Bootstrap method and the bias-corrected nonparametric percentile Bootstrap method are shown in Table 9 (lower part). It can be seen that no matter which confidence interval estimation method is adopted, the confidence intervals of the direct effect, indirect effect (i.e., masking effect) and the ratio of indirect effect to direct effect do not contain 0. Therefore, it can be concluded that farmers’ income plays a masking effect on the negative impact of crop specialization on farmers’ dietary diversity, that is, it alleviates the negative impact of crop specialization on farmers’ dietary diversity. From the specific value of the point estimate, it can be seen that the masking effect of farmers’ income accounts for 25.10% of the direct effect.
According to the testing method of moderation effects, this section incorporates the interaction terms between education level and crop specialization, as well as between market accessibility and crop specialization, into the regression model. The test results are shown in Table 10. As can be observed, the estimated coefficients of both interaction terms are significant at the 5% level and positive, indicating that there is a positive moderating effect between education level and market accessibility. In other words, improving education level and market accessibility can reduce the negative impact of crop specialization on dietary diversity, and vice versa. This result also supports the research hypothesis 3 proposed in this paper, as well as the explanation in the heterogeneity analysis section that crop specialization has a greater negative impact on both II part-time households and mountainous households.
5 Discussion
Admittedly, the relationship between crop specialization or diversity and dietary diversity is mixed and context-specific (Sibhatu and Qaim, 2018; Habtemariam et al., 2021), and a few studies found that crop specialization is a livelihood strategy that is beneficial for improving farmers’ dietary diversity (Argyropoulou, 2016; Chinnadurai et al., 2016). However, this paper uses data from Chinese farmers demonstrates that crop specialization reduces farmers’ dietary diversity, which is consistent with other studies using survey data from developing countries (Ecker, 2018; Sekabira and Nalunga, 2020; Isbell et al., 2024; Morrissey et al., 2024).
With the development of socialization and marketization of agricultural production in China, agricultural households are inevitably involved in a highly open and specialized division of labor system, and the trend of farmers getting rid of the traditional “small-scale and diversified” production mode and entering the development track of modern agriculture cannot be reversed. It is no longer realistic to maintain or increase the level of crop diversification. Therefore, in order to ensure the food security of farmers and achieve the development goal of “Zero Hunger” of the United Nations, what we should do is to ascertain, as much as possible, which groups will suffer greater negative impacts from crop specialization, and what the potential mechanisms of crop specialization affecting dietary diversity are, so that governments can implement more targeted interventions.
Through the heterogeneity analysis, we found that crop specialization would have a greater negative impact on II part-time households and mountainous households. Among them, most of the permanent residents of II part-time households are left-behind women, children and the elderly, and most of the rural farmers in mountainous areas are in a relatively poor state. They are all vulnerable groups in China, so more and more people begin to pay attention to the food safety and health problems of such groups (Min et al., 2019; Wang et al., 2024). After all, if the health of all people cannot be guaranteed, comprehensive prosperity is impossible. Through further mechanism analysis, it was found that income has a mediating effect on the impact of crop specialization on dietary diversity, which is consistent with the basic viewpoint of classical economics that higher specialization leads to higher production efficiency and income (Yang and Ng, 1993; Schultz, 1993; Yang, 2000), and higher income enables farmers to purchase a wider variety of foods (Hawkes and Ruel, 2008; Abokyi et al., 2023), thus masking the negative effects of crop diversification on household dietary diversity.
Education and the market have a moderating effect on the impact of crop specialization on dietary diversity. Although some studies suggest that there is no correlation between the market and dietary diversity (Jones, 2017; Curtin et al., 2024), more scholars have found that both education and market not only enhance farmers’ subjective willingness to consume diversified foods (Hou et al., 2021; Abokyi et al., 2023; Sato et al., 2024), but also provide them with the objective convenience to do so (Koppmair et al., 2017; Morrissey et al., 2024), thereby reducing the negative impact of crop specialization on dietary diversity.
It should be noted that there are some limitations in this paper. First of all, due to the limitation of data, this paper only uses the survey data of Hubei Province, China, and the scope of investigation should be expanded in the future research. However, it is undeniable that Hubei Province is a typical agricultural province in China (Sun et al., 2022), with a total area of 185,900 square kilometers and a total population of 58.38 million, with plains, hills and mountains accounting for 20, 24 and 56% of the total area, respectively. In 2023, the tertiary industrial structure of Hubei Province was 9.1:36.2:54.7, which was generally in line with the national industrial structure (7.1:38.3:54.6). Additionally, the urbanization rate was 65.47%, which was also basically consistent with the national average level (66.16%). The aforementioned characteristics of Hubei Province render the principal findings of this study of significant reference value for other regions of China.
Furthermore, although this paper analyzes the mechanism of income, education and market between crop specialization and dietary diversity at both theoretical and empirical levels, this is only a preliminary exploration. Consequently, further questions need to be addressed. For instance, what is the effect of income generated through crop specialization on dietary diversity? Are the education levels of household heads and market accessibility the most appropriate indicators for representing education and market? What other potential mechanisms, beyond those already discussed, may influence the relationship between crop specialization and dietary diversity? These are also directions for our future research and discussion.
6 Conclusion
Dietary diversity is an important indicator used to measure family dietary quality and food safety status (Jones et al., 2014; Tchuente et al., 2024). In the context of the transformation of China’s agricultural production model from “small-scale and diversified” to specialization, this paper systematically studies the complex relationship between crop specialization and dietary diversity from both theoretical and empirical aspects based on the micro-survey data of farmers collected by HRST in 56 counties and urban areas. It was found that crop specialization had a significant negative effect on dietary diversity, especially on II part-time households and mountainous households. Further mechanism analysis shows that income has mediating effect, education and market has moderating effect, which could mask or reduce the negative effects of crop specialization in the process of affecting diet diversity.
In the reality that the trend of production specialization is irreversible, government intervention measures can be carried out from three aspects to ensure the dietary diversity and food safety of rural households, especially II part-time households and mountainous households. Firstly, by implementing the rural household income doubling plan, we can broaden their sources of income and enable farmers to have sufficient purchasing power to buy a diverse range of foods. Secondly, improve the education level of rural households. For the left-behind women and the elderly who are responsible for cooking in II part-time households, informal education channels such as television and short videos on mobile phones can also be used to promote dietary nutrition knowledge and guide them to establish diverse dietary habits. Thirdly, improve market access conditions. It is necessary to optimize the spatial layout of rural agricultural markets and supermarket outlets, especially in mountainous regions, and enhance transportation conditions between farmers and these outlets. This will enable all farmers to conveniently purchase various types of food needed for their households, thereby reducing the negative effects of crop specialization.
Data availability statement
The micro-survey data of this paper collected by the Hubei Survey Team of the National Statistics Bureau of China. The authors accessed the data with their institution’s membership. The authors had no special access privileges to the data others would not have. While the data on population, economy, terrain and land area of Hubei Province and China is freely available on http://www.hubei.gov.cn/jmct, and https://data.stats.gov.cn/easyquery.htm?cn=C01.
Author contributions
SC: Conceptualization, Formal analysis, Software, Writing – original draft. FS: Data curation, Funding acquisition, Methodology, Software, Writing – review & editing. LW: Data curation, Formal analysis, Writing – review & editing. WH: Writing – review & editing.
Funding
The author(s) declare that financial support was received for the research, authorship, and/or publication of this article. This research was funded by Jiangsu Federation of Philosophy and Social Sciences (Award no.: 23SYC-113).
Acknowledgments
The authors would like to thank the reviewers for their constructive comments on improving this research.
Conflict of interest
Author LW was employed by the company The People’s Bank of China.
The remaining authors declare that the research was conducted in the absence of any commercial or financial relationships that could be construed as a potential conflict of interest.
Publisher’s note
All claims expressed in this article are solely those of the authors and do not necessarily represent those of their affiliated organizations, or those of the publisher, the editors and the reviewers. Any product that may be evaluated in this article, or claim that may be made by its manufacturer, is not guaranteed or endorsed by the publisher.
References
Abokyi, E., Asante, B. O., and Wongnaa, C. A. (2023). Women’s role of caregiving for under-five children: implications for dietary diversity and food security in Ghana. Cogent Food Agric. 1, 1–22. doi: 10.1080/23311932.2022.2153415
Adugna, G., Egata, G., Fufa, D. A., and Desta, D. T. (2024). Effect of nutrition education on improving dietary diversity of children aged 6-23 months in Horo district, Oromia region, Ethiopia. Hum. Nutr. Metab. 35:200244. doi: 10.1016/j.hnm.2024.200244
Argyropoulou, S. E. (2016). The association between the diversity of crop production and nutritional indicators of rural households in northern Ghana. Wageningen: Wageningen University.
Baron, R. M., and Kenny, D. A. (1986). The moderator-mediator variable distinction in social psychological research: conceptual, strategic, and statistical considerations. J. Pers. Soc. Psychol. 51, 1173–1182. doi: 10.1037/0022-3514.51.6.1173
Berti, P. R. (2015). Relationship between production diversity and dietary diversity depends on how number of foods is counted. Proc. Natl. Acad. Sci. USA 42:5656. doi: 10.1073/pnas.1517006112
Chen, S. H., and Ravallion, M. (1996). Data in transition: assessing rural living standards in southern China. China Econ. Rev. 7, 23–56. doi: 10.1016/S1043-951X(96)90018-8
Chen, X., Shuai, C. Y., and Wu, Y. (2023). Global food stability and its socio-economic determinants towards sustainable development goal 2 (zero hunger). Sust. Dev. 31, 1768–1780. doi: 10.1002/sd.2482
Chinnadurai, M., Karunakaran, K. R., Chandrasekaran, M., Balasubramanian, R., and Umanath, M. (2016). Examining linkage between dietary pattern and crop diversification: an evidence from Tamil Nadu. Agric. Econ. Res. Rev. 29, 149–160. doi: 10.22004/ag.econ.253177
Curtin, I. J., Tobin, D., Reynolds, T., and Li, M. E. (2024). Do wealth and market access explain inconsistent relationships between crop diversity and dietary diversity? Evidence from 10 sub-Saharan African countries. Sustain. For. 3:1040. doi: 10.3390/su16031040
Ecker, O. (2018). Agricultural transformation and food and nutrition security in Ghana: does farm production diversity (still) matter for household dietary diversity? Food Policy 8, 271–282. doi: 10.1016/j.foodpol.2018.08.002
Ellis, F. (1998). Household strategies and rural livelihood diversification. J. Dev. Stud. 35, 1–38. doi: 10.1080/00220389808422553
FAO, IFAD, UNICEF, WFP, WHO (2024). The state of food security and nutrition in the world 2024. Rome: Food and Agriculture Organization of the United Nations (FAO).
Firpo, S., Fortin, N. M., and Lemieux, T. (2009). Unconditional quantile regressions. Econometrica 77, 953–973. doi: 10.3982/ECTA6822
Gu, R., Nie, F. Y., Bi, J. Y., and Huang, J. Q. (2016). Effect of livelihood assets on food security: Evidence from survey in six western counties of rural China. Food Nutr. China 1, 10–15. doi: 10.3969/j.issn.1006-9577.2016.01.002
Habtemariam, L. T., Gornott, C., and HandSieber, S. (2021). Farm production diversity and household dietary diversity: panel data evidence from rural households in Tanzania. Front. Sust. Food Syst. 5, 1–10. doi: 10.3389/fsufs.2021.612341
Hawkes, C., and Ruel, M. T. (2008). From agriculture to nutrition: Pathways, synergies and outcomes. Washington, DC: Agricultural and Rural Development Notes of World Bank.
Hou, M., Qing, P., and Min, S. (2021). Multiple indicators of household dietary diversity in rural China: effects of income and dietary knowledge. Nutrition 91:111406. doi: 10.1016/j.nut.2021.111406
Huang, Z. Y., Sun, J. M., Guo, Y. Z., Wang, X. L., and Ma, Y. Q. (2019). Influence of farmers’ farm production diversity on their own dietary diversity and nutritional health. Sci. Agric. Sin. 18, 3108–3121. doi: 10.3864/j.issn.0578-1752.2019.18.004
Isbell, C., Tobin, D., Thiede, B. C., Jones, K., and Reynolds, T. (2024). The association between crop diversity and children’s dietary diversity: multi-scalar and cross-national comparisons. Food Secur. 16, 883–897. doi: 10.1007/s12571-024-01458-9
Jones, A. D. (2017). Critical review of the emerging research evidence on agricultural biodiversity, diet diversity, and nutritional status in low- and middle-income countries. Nutr. Rev. 75, 769–782. doi: 10.1093/nutrit/nux040
Jones, A. D., Shrinivas, A., and Bezner-Kerr, R. (2014). Farm production diversity is associated with greater household dietary diversity in Malawi: findings from nationally representative data. Food Policy 46, 1–12. doi: 10.1016/j.foodpol.2014.02.001
Kasem, S., and Thapa, G. B. (2011). Crop diversification in Thailand: status, determinants, and effects on income and use of inputs. Land Use Policy 28, 618–628. doi: 10.1016/j.landusepol.2010.12.001
Kihiu, E. N., and Kydd, J. (2021). Agricultural market access and dietary diversity in Kenya: gender considerations towards improved household nutritional outcomes. Food Policy 100:102004. doi: 10.1016/j.foodpol.2020.102004
Koppmair, S., Kassie, M., and Qaim, M. (2017). Farm production, market access and dietary diversity in Malawi. Public Health Nutr. 2, 325–335. doi: 10.1017/S1368980016002135
Langer, B., Gupta, R. K., and Kumari, R. M. R. (2024). Food insecurity and its association with dietary diversity: a cross-sectional study in rural households in Jammu. Indian J. Community Med. 1, 70–75. doi: 10.4103/ijcm.ijcm_811_22
Luo, B. L. (2017). Farming family business: Towards a division of labor economy. Beijing: China Agricultural Press.
Lourme-Ruiz, A., Dury, S., and Martin-Prével, Y. (2016). Do you eat what you sow? Linkages between farm production diversity, agricultural income and dietary diversity in Burkina Faso. Cahiers Agric. 6, 65001–65011. doi: 10.1051/cagri/2016038
Min, S., Hou, L. L., Hermann, W., Huang, J. K., and Mu, Y. Y. (2019). The impact of migration on the food consumption and nutrition of left-behind family members: evidence from a minority mountainous region of southwestern China. J. Integr. Agric. 18, 1780–1792. doi: 10.1016/S2095-3119(19)62588-8
Mofya-Mukuka, R., and Hichaambwa, M. (2018). Livelihood effects of crop diversification: a panel data analysis of rural farm households in Zambia. Food Secur. 10, 1449–1462. doi: 10.1007/s12571-018-0872-6
Morrissey, K., Reynolds, T., Tobin, D., and Isbell, C. (2024). Market engagement, crop diversity, dietary diversity, and food security: evidence from small-scale agricultural households in Uganda. Food Secur. 16, 133–147. doi: 10.1007/s12571-023-01411-2
Nandi, R., Nedumaran, S., and Ravula, P. (2021). The interplay between food market access and farm household dietary diversity in low- and middle-income countries: a systematic review of literature. Glob. Food Sec. 28:100484. doi: 10.1016/j.gfs.2020.100484
Ng’endo, M., Keding, G. B., Bhagwat, S., and Kehlenbeck, K. (2015). Variability of on-farm food plant diversity and its contribution to food security: a case study of smallholder farming households in Western Kenya. Agroecol. Sustain. Food Syst. 39, 1071–1103. doi: 10.1080/21683565.2015.1073206
Oster, E. (2019). Unobservable selection and coefficient stability: theory and evidence. J. Bus. Econ. Stat. 2, 187–204. doi: 10.1080/07350015.2016.1227711
Pellegrini, P., and Tasciotti, L. (2014). Crop diversification, dietary diversity and agricultural income: empirical evidence from eight developing countries. Canadian J. Dev. Stu. 35, 211–227. doi: 10.1080/02255189.2014.898580
Porter, S. (2024). Understanding the counterfactual approach to instrumental variables: a practical guide. Asia Pac. Educ. Rev. 25, 673–683. doi: 10.1007/s12564-024-09982-1
Rahman, S. (2009). Whether crop diversification is a desired strategy for agricultural growth in Bangladesh? Food Policy 34, 340–349. doi: 10.1016/j.foodpol.2009.02.004
Reed, W. R., and Ye, H. (2011). Which panel data estimator should I use? Appl. Econ. 8, 985–1000. doi: 10.1080/00036840802600087
Sato, Y., Khamphithoun, S., Saiyachak, K., Ando, H., Ishizuka, T., et al. (2024). Enhancing child dietary diversity through cooking demonstration and nutritional education in rural Lao PDR. Trop. Med. Health. 52:8. doi: 10.1186/s41182-023-00571-3
Scoones, I. (1998). Sustainable rural livelihoods: A framework for analysis. Falmer: The Institute of Development Studies (IDS).
Sekabira, H., and Nalunga, S. (2020). Farm production diversity: is it important for dietary diversity? Panel data evidence from Uganda. Sustain. For. 2, 1–21. doi: 10.3390/su12031028
Sibhatu, K. T., Krishna, V. V., and Qaim, M. (2015). Production diversity and dietary diversity in smallholder farm households. Proc. Natl. Acad. Sci. USA 34, 10657–10662. doi: 10.1073/pnas.1510982112
Sibhatu, K. T., and Qaim, M. (2017). Rural food security, subsistence agriculture, and seasonality. PLoS One 10, 1–15. doi: 10.1371/journal.pone.0186406
Sibhatu, K. T., and Qaim, M. (2018). Farm production diversity and dietary quality: linkages and measurement issues. Food Secur. 1, 47–59. doi: 10.1007/s12571-017-0762-3
Sun, F., and Chen, Y. P. (2019). Fuzzy evaluation of the development level of Chinese farmers. J. South China Agric. Univ. Soc. Sci. Ed. 5, 50–63. doi: 10.7671/j.issn.1672-0202.2019.05.005
Sun, F., Qian, P., Cao, S., Chen, Y., and Feng, Z. (2022). The impact of crop specialization on nutritional intake: evidence from farm households in China. PLoS One 17:e0272347. doi: 10.1371/journal.pone.0272347
Tchuente, T. B. R., Hagbe, P. V., Eyenga, E. F., Dibacto, K. R. E., Ngoumen, N. D. J., Youovop, F. J. A., et al. (2024). Dietary diversity and food security status among cameroonian adults living in semi-urban areas: a cross-sectional study. Food Nutr. Sci. 7, 568–564. doi: 10.4236/fns.2024.157036
Tobin, D., Jones, K., and Thiede, B. C. (2019). Does crop diversity at the village level influence child nutrition security? Evidence from 11 sub-Saharan African countries. Popul. Environ. 2, 74–97. doi: 10.1007/s11111-019-00327-4
Vo, D. H. (2024). Drivers of domestic migration in Vietnam: the characteristics of the households and their heads, environmental factors and living conditions. PLoS One 6:e0304321. doi: 10.1371/journal.pone.0304321
Wang, G., Hao, Y., and Ma, J. (2024). Family income level, income structure, and dietary imbalance of elderly households in rural China. Food Secur. 2:190. doi: 10.3390/foods13020190
Wang, R. Q. (2016). Expectations of children's education and willingness of migrant workers to settle in cities: based on survey data from 7 cities across the country. J. Agrotech. Econ. 3, 77–86. doi: 10.13246/j.cnki.jae.2016.03.008
Yang, X. K., and Ng, Y. K. (1993). Specialization and economic organization: A new classical microeconomics framework. North-Holland: North-Holland Publishing Company.
Yang, X. K. (2000). Economics: New classical versus neoclassical frameworks. Oxford: Blackwell Publishing Ltd.
Keywords: crop specialization, dietary diversity, farmers’ income, education level, market accessibility
Citation: Cao S, Sun F, Wang L and Hong W (2024) “Less is more?” The association between crop specialization and dietary diversity in China. Front. Sustain. Food Syst. 8:1439989. doi: 10.3389/fsufs.2024.1439989
Edited by:
Q. Yuan, Chinese Academy of Sciences (CAS), ChinaReviewed by:
Sukhwinder Singh, University of Winnipeg, CanadaAmar Razzaq, Huanggang Normal University, China
Copyright © 2024 Cao, Sun, Wang and Hong. This is an open-access article distributed under the terms of the Creative Commons Attribution License (CC BY). The use, distribution or reproduction in other forums is permitted, provided the original author(s) and the copyright owner(s) are credited and that the original publication in this journal is cited, in accordance with accepted academic practice. No use, distribution or reproduction is permitted which does not comply with these terms.
*Correspondence: Fei Sun, c3VuZmVpNTczQHNpbmEuY29t