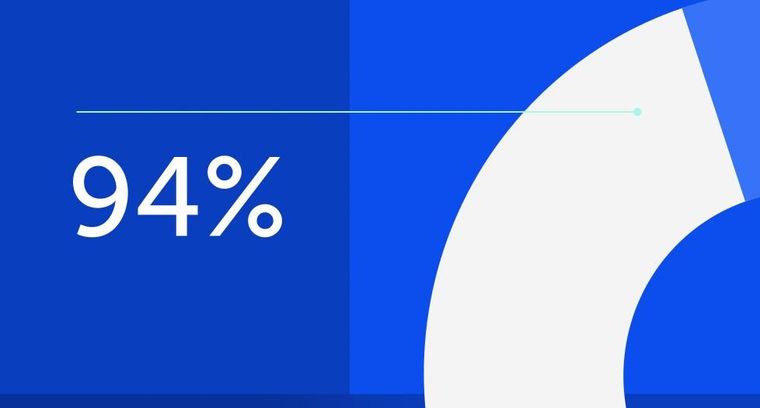
94% of researchers rate our articles as excellent or good
Learn more about the work of our research integrity team to safeguard the quality of each article we publish.
Find out more
ORIGINAL RESEARCH article
Front. Sustain. Food Syst., 25 September 2024
Sec. Agricultural and Food Economics
Volume 8 - 2024 | https://doi.org/10.3389/fsufs.2024.1431294
This article is part of the Research TopicStrategies Of Digitalization And Sustainability In Agrifood Value ChainsView all 23 articles
Green is the foundation of agricultural development. By leveraging modern information technology, digital village construction injects new momentum into the green growth of agriculture. Using balanced panel data from 31 provinces in China from 2011 to 2022, this paper employs the entropy approach and SBM-GML index to measure the indicators of digital village construction and agricultural green total factor productivity (AGTFP). The impacts and mechanisms of digital village construction on AGTFP are examined through fixed effect, mediating effect, and threshold models. The findings are as follows: (1) AGTFP in China shows an overall increasing trend from 2011 to 2022, with significant growth in the western region compared to the eastern and central regions. (2) Digital village construction significantly accelerates the improvement of AGTFP, a conclusion supported by robustness tests. (3) Mechanism analysis indicates that digital village construction advances AGTFP by fostering agricultural technology innovation, enhancing agricultural human capital, and improving agricultural productive services. (4) Digital village construction has a more significant impact on major grain-producing areas, economically underdeveloped areas, and northern region compared to other regions. (5) Environmental regulation acts as a threshold effect on the impact of digital village construction on AGTFP. Digital village construction substantially impacts AGTFP when environmental regulation is below the threshold, with the positive effect continuing beyond this point but at a reduced intensity.
Advancing the greening of agriculture is essential for mitigating climate change, maintaining food security, and safeguarding the well-being of the planet’s ecosystems. In 2015, the United Nations unveiled the Sustainable Development Goals. The urgency of supporting sustainable agricultural growth and guaranteeing global food security is particularly emphasized by the second of these goals, Zero Hunger. In 2023, China’s total grain output reached 695.41 billion kilograms, while the per capita grain possession reached 493 kilograms, surpassing the internationally recognized food security line of 400 kilograms. As a populous developing country, China has achieved self-sufficiency in agricultural output. However, China’s previous agricultural development model relied heavily on chemical fertilizers and pesticides, making it unsustainable. The over-reliance on chemical fertilizers and pesticides in agricultural production has caused worldwide environmental hazards, threatening human health and food security (Dou et al., 2016; Rockström et al., 2017). Achieving the harmony and unity of agricultural productivity and environmental sustainability is an urgent issue in modern agricultural development (Koiry and Huang, 2023). To address this issue, agricultural green development is a practical solution.
There is a consensus among nations, with a general increase in focus and funding directed toward agricultural green development. In March 2020, the European Union introduced its Farm to Fork strategy. Implementing this plan is an excellent start in reducing agricultural carbon footprints and stimulating a sustainable transition in the food system. Agricultural green development is also a top priority for the Chinese government. According to the Communist Party of China’s report to the 19th National Congress, efforts should be made to address major environmental issues, tighten regulations on non-point source pollution from agriculture, and implement measures to enhance the quality of life in rural areas. Agricultural green development has also been emphasized numerous times in Central Document No. 1 in recent years. Agricultural Green Total Factor Productivity (AGTFP) fully reflects the comprehensive competitiveness of agricultural green development and has become an important indicator for measuring it. Enhancing AGTFP is a crucial step in advancing the green transformation of agriculture, which has become the predominant path of China’s agricultural development.
Traditional Total Factor Productivity (TFP) primarily focuses on improving production efficiency while ignoring the ecological impact of agriculture, which is not conducive to modern agricultural practices (Baležentis et al., 2021). AGTFP seeks sustainable and ecologically friendly economic growth by incorporating environmental considerations into TFP. AGTFP is crucial for managing the pressure of an ever-increasing population (Myeki et al., 2023), safeguarding global food security (Zhang et al., 2021), adapting to climate change, and ensuring the sustainability of agriculture (Akzar and Amandaria, 2021). Research has confirmed the beneficial impact of factors including crop insurance (Fang et al., 2021), rural industrial integration (Chen et al., 2024), and agricultural mechanization (Zhu et al., 2022b) on AGTFP. However, scholars have given less attention to the impact of recent changes in China’s village environment on AGTFP. Therefore, this paper innovatively explores the impacts and mechanisms of AGTFP from the perspective of digital village construction.
Digital technology significantly aids the advancement of rural and agricultural development. The rapid progress of information and communication technology has been the primary catalyst for the global trend of smart villages, which has emerged in recent years. In 2017, the European Commission introduced the EU Smart Village Initiative to promote balanced regional development, rural prosperity, and growth. Digital village construction has been identified as a critical tactic to solve the numerous obstacles to sustainable agricultural growth (Zhang and Zhang, 2020). In 2018, Central Document No. 1 put forth proposals for executing the digital countryside strategy. In December 2023, the National Development and Reform Commission and the National Data Bureau issued the Implementation Programme on Digital Economy for Common Wealth, making digital village construction a critical task to empower rural revitalization. The all-encompassing development of digital village construction has boosted agricultural and rural development, a prerequisite for encouraging agricultural modernization and a calculated move toward realizing the objective of rural revitalization (Shen and Ye, 2021). Its essence is to empower the whole process of agricultural production and marketing through technology and to achieve the organic combination of the new paradigm of the digital economy and the traditional production organization of agriculture (Guo and Liu, 2023). This paper argues that digital village construction is critical in promoting AGTFP. Digital village construction may effectively enhance the intelligence and precision of agricultural production and its efficiency in utilizing resources and energy. It will ultimately strengthen AGTFP. Despite previous studies examining the multifaceted consequences on rural areas (Mei et al., 2022) and farmers’ lives (Chen et al., 2022), there is a lack of thorough research on the implications of digital village construction on AGTFP. In light of this, the objective of this paper is to investigate the impacts of digital village construction on AGTFP and its underlying mechanisms. Given the current push for green development, these concerns merit investigation. This paper will provide valuable references for promoting global ecological balance, mitigating climate change, and ensuring social well-being.
This paper experimentally analyzes the influence of digital village construction on AGTFP and its underlying mechanisms using balanced panel data for 31 Chinese regions from 2011 to 2022. This paper’s contributions are outlined below. (1) Regarding research content, this work investigates how digital village construction affects AGTFP, adding to the body of literature on the subject and discussing the elements that influence AGTFP. (2) In terms of the impact mechanism, studies have verified the mediating effects of factors such as resource misallocation (Guo and Liu, 2023), agricultural scale operation, and agricultural informatization (Du J. et al., 2023) in the process of digital village construction empowering AGTFP. This paper explores how digital village construction fosters AGTFP through agricultural technology innovation, agricultural human capital, and agricultural social services. Consequently, the impact pathway of digital village construction on AGTFP is more fully recognized. (3) Previous studies have not considered the potential threshold effect of digital village construction on AGTFP. This paper uses environmental regulation as a threshold variable to investigate the threshold effect. In light of the aforementioned conclusions, targeted recommendations are provided for policymakers to optimize digital village construction to promote environmental sustainability while enhancing agricultural productivity.
The digital village strategy was initially introduced in the central government’s No. 1 document in 2018. Since then, scholarly investigations into digital village construction have evolved from qualitative and theoretical interpretations to quantitative evaluations and empirical analyses. First, given that digital village construction is an emerging research field, most recent studies have been theoretical, exploring the connotations of digital village construction, practical dilemmas, and path selection. The literature defines the concept of digital village construction. By using modern information networks as the primary platform and contemporary information technology as the primary engine, digital village construction refers to a set of initiatives, plans, and procedures designed to entirely reinvent rural economic development based on the advancement of the digital economy (Wang S. et al., 2021). Second, the Institute for New Rural Development at Peking University introduced the County Digital Village Index in 2022 to quantitatively measure digital village construction. The index uses Four primary dimensions to construct an extensive assessment framework: digital infrastructure, digitalization of economic activity, digitalization of governance procedures, and digitalization of citizens’ lives. Subsequently, there was a steady surge in the exploration of how digital village construction can empower rural communities and agriculture. From a broad viewpoint, digital village construction can promote rural economic growth (Wang P. et al., 2023) and sustainable rural development (Adamowicz and Zwolińska-Ligaj, 2020). The divide between towns and the countryside tends to close as the level of the digital village rises (Zhao and Zhao, 2024). At the micro level, digital village construction can significantly reduce the likelihood that rural households in China will alter their current secure situation in response to disturbances and increase their resilience (Cai et al., 2023). Additionally, by encouraging employment in other industries and asset conversion, digital village construction can significantly boost the revenue of farmers (Chen et al., 2022).
The domain of AGTFP research is constantly expanding and deepening. Initially, research concentrated on AGTFP measurement techniques and outcome analysis, striving to establish and improve quantitative instruments for evaluating AGTFP. Over time, scholars broadened their perspectives and initiated investigations into the diverse elements influencing AGTFP. In 1957, Robert Solow first proposed the notion of TFP, also known as the Solow residual. Since its introduction to the agricultural industry, TFP has become a crucial metric for assessing the state of the agricultural economy (Jorgenson and Gollop, 1992). With the increasing contradiction between resources and the environment, the level of resource utilization and the degree of ecological damage are involved in the evaluation index (Oskam, 1991). There are currently two categories of AGTFP research. Measuring and analyzing AGTFP comes first. Parametric and non-parametric approaches are the categories into which their measurement methodologies fall. Research on AGTFP drivers is the second. Agricultural credit inputs have been shown by researchers to considerably boost AGTFP within a region while inhibiting its growth in adjacent areas (Wang et al., 2022). Fang et al. (2021) found that increasing agricultural insurance coverage will facilitate AGTFP, and this effect will increase with the growing operation scale.
Furthermore, the researcher discovered that adopting the carbon trading pilot program boosts AGTFP (Yu et al., 2022). AGTFP’s driving force has grown over time due to the reduction of carbon quotas. In addition, some scholars have explored the drivers of AGTFP from the perspectives of integration of rural industries (Chen et al., 2024), industrial agglomeration (Luo et al., 2023), and digital inclusive finance (Gao et al., 2022).
Digital village construction has become a significant concern at the national strategic level due to the ongoing advancement of digital technology and its deep integration with agriculture and rural areas. Simultaneously, indicators for evaluating agricultural digitization have evolved. Agricultural digitization indicators were initially single-dimensional but have since developed into a multi-dimensional indicator system. In previous research, academics primarily utilized the notion of agricultural informatization to determine the state of digitalization in rural regions. The association between agricultural informatization and TFP has been the leading research subject. Digital village construction is now a crucial component for the excellent growth of agriculture due to the profound advancement of the current technological revolution (Mei et al., 2022). The advent of the digital village signifies a new phase in the advancement of agricultural digitalization. In addition to positively impacting agriculture’s TFP (Fang et al., 2024), digital village construction has some ecological effects. Some studies have found an inverted U-shaped curve association between digital village construction and carbon emissions in rural areas. This effect will vary depending on the stage of regional economic development (Hao et al., 2022). While Tang and Chen (2022) confirmed that the development of digital villages can increase the effectiveness of greening arable land, a thorough empirical examination of its underlying influence mechanism is lacking. On this basis, Lin and Li (2023) found that digital village construction can enhance agroecological efficiency through agricultural scale operation and optimizing agricultural planting structure. However, other researchers have discovered that digital village construction lowers ecological performance by using the e-commerce into rural comprehensive demonstration zone policy as a quasi-natural experiment (Zhang and Zhong, 2023).
By combining the relevant literature in this field, it was found that the literature has conducted relevant studies on the evaluation methods and driving elements of AGTFP. Scholars have begun concentrating on the impact of digital village construction on agriculture as a result of the progression of the digital village strategy. The findings of previous studies serve as a valuable guide for this paper. Nonetheless, there is still space for the current study to be improved and expanded upon: (1) Existing research mostly starts from a single indicator, such as access to the internet and the degree of agricultural information, to explore its impact on agricultural development, and less research has focused focus on the effect of digital village construction on agriculture. (2) Research on the mechanisms influencing AGTFP is not well-represented in the literature currently, and a relatively small amount of studies have empirically examined the connection between digital village construction and AGTFP. Thus, this work investigates the effect of digital village construction on AGTFP and provides additional clarification on the impact mechanism of digital village construction on AGTFP based on previous research. Ultimately, policy proposals for China’s digital village construction are proposed in light of the paper’s findings to support rural green development while accomplishing agricultural modernization.
Digital village construction has greatly influenced several facets, such as agricultural production, rural life, and village governance, by utilizing modern technologies, particularly the web, big data, artificial intelligence, and other tools. The following points illustrate how digital village construction affects agriculture’s green development. First, digital village construction reduces the transaction costs faced by farmers due to information asymmetry and other factors by providing efficient information services (Zanello, 2012). Using this platform, agricultural producers can quickly and accurately grasp the dynamics of land supply and demand, improve land resource allocation, and promote agricultural development toward large-scale operation. The large-scale management of agriculture fosters the popularization of environmental protection agricultural technology and the optimization of agricultural structure (Du J. et al., 2023), achieving the efficient use of resources, increasing production efficiency, and boosting the green transformation of agriculture (Wei et al., 2022). Second, the efficiency and transparency of information sharing have progressively increased with the ongoing development and application of information technology (Ji et al., 2023). The information-based regulatory capacity of agricultural green production has strengthened (Arts et al., 2016). The application of artificial intelligence, remote sensing satellites, and Beidou navigation to agricultural production has accelerated (Shin and Choi, 2015), improving agricultural production efficiency and precision and reducing resource waste and environmental pollution. In addition, digital village construction promotes establishing an information-based regulatory system for agricultural green production, thus strengthening the supervision and management of environmental protection standards for agricultural production (Granell et al., 2016) and guaranteeing the quality of agricultural goods and the safety of the natural environment. Thus, hypothesis 1 is put out in this work.
Hypothesis 1. Digital village construction can contribute to AGTFP.
The permeability of digital technology is driving the digital transformation of production elements and production relations, thereby altering the model of social and economic growth. Digital village construction can empower agriculture in the agricultural sector through three dimensions. First, technological empowerment: digital village construction can lead to technological agricultural innovations. These innovations can optimize agricultural production processes and are critical for raising AGTFP. Second, labor empowerment: digital village construction can attract talent to the countryside and improve the comprehensive literacy of farmers, thus enhancing rural human capital. Human capital, as a carrier of information and expertise, is vital for the progress of AGTFP. Third, management empowerment: digital village construction accelerates the growth of agricultural productive services through technological support. Agricultural productive services, which provide crucial information and managerial guidance, facilitate AGTFP growth. Collectively, these three approaches have boosted AGTFP and offered a fresh outlook for the sustainable development of agriculture.
Technological innovation is the core of improving food output and enhancing agricultural productivity, sustainability, and resilience (Liu et al., 2021). Digital village construction has expedited the agricultural sector’s technical advancement rate. The adoption of advanced technologies could enhance AGTFP while lowering the detrimental effects of agricultural production on the environment (Wang H. et al., 2021). First, digital village construction is a significant component in advancing the creation of a digital China and a strategic path for rural rejuvenation. This objective has sped up the adoption of digital technology in agriculture and encouraged the invention of innovative agricultural technologies. Second, digital village construction has reinforced the rural advancement of information infrastructure. The popularization of broadband and mobile communication networks has established a strong hardware basis for technology innovation and accelerated the application and popularisation of advanced agricultural technologies. Innovation in agricultural technology greatly maximizes the distribution of production elements, enhances the efficiency of traditional energy use, and reduces the carbon footprint from agricultural production (Zhu et al., 2022b). In addition to significantly increasing the productivity of production factors, the use of advanced technology in agriculture can spur the development of novel agricultural production practices. For instance, it supports the growth of ecological agriculture. It ensures that agricultural production activities and the natural environment remain harmonious. Consequently, hypothesis 2 is put out in this work.
Hypothesis 2. Digital village construction can enhance AGTFP by promoting agricultural technology innovation
Human capital is a crucial and scarce resource for digital village construction. Enhancing agricultural human capital is instrumental in elevating the quality and efficiency of agricultural management. This enhancement is essential for advancing AGTFP. Farmers’ digital quality improves and highly qualified talent returns, both of which are indicators of increased agricultural human capital. While promoting innovation and entrepreneurship in villages (Audretsch et al., 2015), digital village construction also opens up diverse employment avenues for residents (Ma and Han, 2023). The widespread use of digital technology provides a new employment direction and career development opportunities for high-skilled labor, promotes the return of talent to the agricultural field, and injects new vitality into the sustainable development of agriculture. Highly skilled personnel can not only develop and promote new agricultural technologies but also spread the concept and knowledge of green agriculture to more agricultural producers and enhance farmers’ environmental awareness and technical level, thus improving agricultural production efficiency and ecological friendliness. As digital village construction continues, rural communities’ digital infrastructure has steadily improved, providing farmers more diversified access to knowledge and information. Farmers can now more easily access market trends and learn advanced agricultural technologies and management strategies, enhancing their information literacy and agricultural production management skills. It has positively impacted the promotion of green production practices and AGTFP (Du F. et al., 2023). Accordingly, hypothesis 3 is put out in this work.
Hypothesis 3. Digital village construction can enhance AGTFP by enhancing agricultural human capital.
The agricultural productive service industry refers to the industry that specializes in providing intermediate services for the producers of agricultural products, and its services cover the entire agricultural production process. Promoting agricultural productive services is essential for accelerating the process of agricultural modernization and promoting rural industrial revitalization. Along with the depth of digital village construction, agricultural productive services will also improve. On the one hand, digital village construction provides a perfect infrastructure for agricultural productive services, providing accurate data supply and thus improving the productivity and accuracy of agricultural productive services. On the other hand, the adoption of modern digital techniques dramatically expands the service scope of the agricultural productive service industry. It stimulates innovation in its service mode and content to address farmers’ diverse demands more accurately and upgrade efficiency and quality of service. To pursue maximum economic returns, farmers tend to apply agricultural inputs, including pesticides, chemical fertilizers, and agro-film in excess during farming, to increase agricultural output, which places a significant burden on the ecological environment (Xu et al., 2022). Agricultural productive services are a vital way to alleviate these problems.
First, agricultural productive service organizations can popularize and promote water-saving irrigation, organic farming, and other environmentally friendly agricultural technologies to farmers and guide agricultural business entities to adopt scientific and environmentally friendly production technologies and management methods (Yang et al., 2013), thereby reducing agricultural pollution related to the agricultural production process and reducing agricultural non-desired output. Second, enhancing the level of expertise at each link in the agriculture value chain is agricultural productive services (Zhu et al., 2022a). The benefits of specialization brought about by the division of labor enhance agricultural productivity and alleviate the excessive use of agrochemicals (Sims and Kienzle, 2017). In addition, accompanied by technological innovation, agricultural productive services can be digitized and intelligently upgraded, allowing farmers to access information more conveniently and effectively, feeding back the market demand for green agricultural goods to farmers and incentivizing them to carry out green production. Accordingly, hypothesis 4 is put out in this work.
Hypothesis 4. Digital village construction can enhance AGTFP by promoting agricultural productive services.
Environmental regulation aims to safeguard the environment by controlling different activities that contaminate the public space, and it is an essential element of social regulation. Using a range of legislative and policy initiatives, environmental regulation effectively reduces pollutant emissions, thus weakening ecological damage. Two major groups of academics have different perspectives on how environmental regulations affect agriculture. Some scholars advocate innovation compensation theory, arguing that appropriate environmental regulation can stimulate agricultural farmers to incorporate more environmentally friendly technologies and methods (Porter and Linde, 1995), promote ecological and sustainable agriculture, and improve AGTFP. Other scholars follow the cost theory, emphasizing that environmental regulation may bring additional costs to agricultural production (Barbera and McConnell, 1990), putting pressure on agricultural production, especially for small-scale farmers and economically underdeveloped areas, and affecting their competitiveness. In addition, the increase in environmental management costs will crowd out the R&D expenditures of cleaner agricultural technologies, increasing the financial burden on agricultural producers. It may lead to a decline in AGTFP in the short term. The role of environmental regulation in digital village construction to promote AGTFP depends on the dynamic relationship between innovation compensation and the cost of compliance under different intensities. Therefore, a non-linear link exists between digital village construction and AGTFP. Thus, hypothesis 5 is put out in this work.
Hypothesis 5. Environmental regulation has a threshold effect on the impact of digital village construction on AGTFP.
Figure 1 depicts the theoretical framework of this paper.
This paper selects labor, land, water, energy, chemical fertilizers, agrochemicals, and agro-films as indicators of agricultural inputs. Among them, labor input is measured by primary industry employees. The crop sown area serves as a gauge for land input. The quantity of water utilized in agriculture is used to calculate water input. Agriculture’s energy intake is calculated by how much power is used. Chemical fertilizer, agrochemical, and agro-film are measured by the number of pure chemical fertilizers used, the agrochemicals used, and the amount of agro-films used, respectively.
The gross value of forestry, livestock, fishery, and agriculture deflated for the 2011 base period is used to calculate the desired output.
This paper identifies six primary sources of agricultural carbon emissions by extensively investigating agricultural production practices and reviewing pertinent research findings. First, carbon emissions are generated by the use of agricultural films, pesticides, and fertilizers. Second, plowing alters soil structure and emits greenhouse gases. Third, burning fossil fuels during irrigation and using agricultural machinery contributes to carbon emissions. To more accurately assess the effects of these actions on nature, an indirect pollution quantification can be achieved by using carbon emissions as a proxy variable. Undesired output was measured using carbon emissions from agriculture. The type of carbon source, its quantity, and the related carbon emission coefficient are the three components required for estimating total carbon emissions, according to Li et al. (2011). Equation 1 presents the equations.
In Equation 1, is the sum of carbon emissions. shows the carbon emissions per source selected for this paper. represents the quantity of each carbon source. represents the carbon emission factor. Table 1 offers the individual carbon emission factors.
Methods for measuring production efficiency can be categorized into parametric and non-parametric methods. The stochastic frontier production function (SFA) represents the former and the latter using the data envelopment analysis (DEA) method. The SBM model (Tone, 2001) and the super-efficiency SBM model (Tone, 2002) are extensions and enhancements of the traditional DEA model. Compared with other models, the super-efficiency SBM model has several outstanding advantages. First, compared with SFA, the super-efficiency SBM model avoids presetting the production function form, reducing subjectivity in the model setting process and enhancing the efficiency assessment’s objectivity. Second, compared with the traditional DEA model (represented by the CCR model and BCC model), the non-radial and non-angle super-efficiency SBM model simultaneously considers the slack variables of inputs and outputs, reflecting the actual situation of each factor more objectively, thereby avoiding the measurement errors caused by the radial and angle selection problems of the traditional DEA model. Third, the super-efficiency SBM model can handle situations containing undesired outputs, demonstrating its unique application value in environmental efficiency assessment. Fourth, the super-efficiency SBM model can assess the efficiency of effective units in a more detailed way, identifying slight differences in AGTFP across each Chinese province. Nonetheless, the super-efficiency SBM model cannot handle cases where input and output variables exhibit radial and non-radial characteristics. Considering the above factors, this paper assesses AGTFP using the super-efficiency SBM model.
In Equation 2, is the decision unit efficiency value. x, y, and b are the input, desired, and undesired output vectors. and are slack variables. is a vector of weights in the model. When , the system is considered to be efficient.
The super-efficiency SBM model evaluates individual decision-making units’ production efficiency values at a given technology level. The efficiency value determined by the model, however, does not visualize the dynamics of productivity over time; instead, it is a static indicator. Therefore, based on the super-efficiency SBM model, the Global Malmquist-Luenberger (GML) index is used to measure AGTFP to achieve an inter-period comparison of productivity. The formula is as follows.
In Equation 3, represents the change in AGTFP from period t to t + 1. and denote the values of the efficiency distance function at times t and t + 1, respectively, which measure the relative efficiency of the decision-making unit at times t and t + 1. When the GML index surpasses 1, the efficiency of the decision unit at time t + 1 has increased relative to time t. If the GML index is below 1, the production efficiency has decreased compared to the base period. The case where the GML index is precisely 1, on the other hand, reflects that the productivity of the decision-making module has remained stable over the assessment period.
Using 2011 as the base year, the AGTFP of 31 provinces in China was measured from 2011 to 2022. Three areas were created out of the provinces by considering their geographic locations: East, Central, and West. Figure 2 depicts the findings of the computation. At the national level, the GML index consistently remained above 1 during the decade from 2012 to 2022. Specifically, the GML index increased from 1.017 in 2012 to 1.072 in 2017. It then increased to 1.150 in 2022, showing an overall upward trend. It reflects that Chinese agriculture is gradually transforming toward a more environmentally friendly and sustainable green development path. Notably, under the influence of the COVID-19 epidemic in 2020, AGTFP in regions other than the east of China decreased to varying degrees, especially in the West. The AGTFP in the east, central, and west at the regional level increased steadily during the sample period. As shown in Figure 2, the West performs exceptionally well in terms of the growth of AGTFP. Specifically, in 2012, the region’s GML index was 0.994, lower than other regions. However, by 2022, the index had increased significantly to 1.178, not only catching up but also jumping above the national average. This jump shows that although the economic and agricultural progress of the West is slightly lagging behind that of the East and Central, this phenomenon provides the region with a unique late-comer advantage, indicating its vast growth potential. In transitioning to a green economic system, the West can actively change its traditional agricultural production mode and structure. In addition, the West can reach the target of specialization and scale in production by learning and adopting advanced green production technology to catch up with other regions and show a strong development momentum in upgrading the greening of agriculture.
The present study develops an econometric model to investigate the influence of digital village construction on AGTFP through empirical means, depending on the theoretical analysis presented above.
In Equation 4, i indicates the region. t indicates the year. is the AGTFP of province i in period t. is the digital village construction degree of province i in period t. is the coefficient to be estimated, indicating the direction and magnitude of the effect of digital village construction on AGTFP. stands for control variables. represents the constant term. and represent the province and year fixed effects, respectively. represents the potential random error term.
In this paper, the mediating effect was adopted according to Jiang (2022), and agricultural technology innovation, agricultural human capital, and agricultural productive services were selected as the mediating variables. The modeling is as follows.
In Equation 5, represents the mediating variable. is the coefficient to be estimated, indicating the degree and direction of the influence of digital village construction on the mediating variable.
This paper uses AGTFP as the dependent variable. Since the AGTFP calculated above is a chained growth index from time t to t + 1, this paper transforms it into a cumulative value with 2011 as 1. Hence, the AGTFP in 2012 was the product of the AGTFP in 2011 and the GML index in 2012. The AGTFP was calculated analogously for subsequent years.
Digital village construction aims to advance digital transformation across all rural and agricultural facets. This construction process involves a wealth of content and covers various participating subjects. Therefore, when assessing the state of digital village construction, the academic community tends to establish an integrated system of evaluation indicators. According to the relevant literature, combined with data availability, this paper creates a system of indicators containing three dimensions, and the detailed metrics are listed in Table 2. The comprehensive score of each province’s digital village construction level is then determined using the entropy approach, and it serves as the independent variable for this paper. Among these indicators, weather stations are devices that gather and quantify data concerning atmospheric phenomena, which is crucial for forecasting weather conditions, analyzing climate change, and evaluating the state of the environment. However, these statistics are insufficient for agricultural needs. Consequently, agrometeorological stations were established. Agrometeorological stations track meteorological features directly related to agriculture, specialize in meeting agricultural production needs, and assist farmers in promptly updating their agricultural production strategies.
Agricultural technology innovation: The quantity of patents is usually regarded as an essential gauge of technical advancement. According to Liu et al. (2021), the number of patents per capita is valuable for gauging agricultural technology innovation in rural settings. Agricultural human capital: The actual per capita human capital data of rural areas in China Human Capital Report 2023 was adopted. Agricultural productive services: According to Tang et al. (2023), the gross value of services in forestry, agriculture, livestock, and fisheries per unit of sown area is a proxy for the degree of productive agricultural services. In empirical evidence, logarithms are utilized.
In this work, environmental regulation serves as the threshold variable. Referring to Lv et al. (2021), the amount of environmental investment divided by the annual gross agricultural product was used to assess the state of environmental regulation.
To exclude the impact of external factors on AGTFP, drawing on the relevant literature (Sun, 2022; Bai et al., 2023), the indicators of urban–rural income distribution, fiscal expenditure on agriculture, agricultural disaster rate, agricultural machinery density, and agricultural cropping structure are picked as control variables. The ratio of per capita disposable income in urban areas to that in rural areas is utilized to indicate the income distribution. The logarithm of local financial expenditure on forestry, water affairs, and agriculture is used to estimate the amount of financial expenditure on agriculture. The disaster area to total sown area ratio represents the agricultural disaster rate. Agricultural machinery density is measured by the power of agricultural machinery per unit sown area. The proportion of the area devoted to food crops to the overall area of crops is employed to express the agricultural crop structure.
For the independent variables, data on Taobao villages originate from the China Taobao Village Research Report from previous years. The Peking University Digital Financial Inclusion Index report sourced the digital financial inclusion index. Data on farmers’ consumption levels of digital services come from the China Statistical Yearbook. The rest of the indicators, such as agrometeorological stations, derive from the National Bureau of Statistics. For the dependent variable, all data come from the National Bureau of Statistics website, except for those employed in the primary sector, which come from provincial statistical yearbooks. All data were sourced from the National Bureau of Statistics website for control variables. Human capital statistics were sourced from the China Human Capital Report 2023 for the mediating variables. Data on patents were collected from the China National Knowledge Infrastructure Patent Database. Raw data on agricultural productive services come from the China Tertiary Industry Statistical Yearbook. The data for the threshold variables were obtained from the China Environmental Statistics Yearbook. For missing data for individual years in some provinces, the paper employed linear interpolation to supplement the data.
Table 3 shows the characteristics of the variables. From 2011 to 2022, the standard deviation of China’s AGTFP was 0.480, and the maximum and minimum values were 3.210 and 0.587, respectively, indicating a significant difference in each province’s AGTFP. For digital village construction, the values range from 0.015 at the lowest point to 0.767 at the highest. The standard deviation is 0.113, which reflects that the overall level of digital village construction needs to be improved and that there is uneven development among regions. Analysis of the mean and standard deviation of the mediating and threshold variables reveals significant disparities among Chinese provinces regarding productive services, technological innovation capacity, and environmental regulation. Analysis of the control variables shows that Chinese provinces have relatively balanced urban–rural income distribution, more significant financial support for agriculture, higher mechanization density, and a relatively balanced agricultural structure, all providing the necessary conditions for green agricultural development.
Table 4 reports the baseline regression results for the impact of digital village construction on AGTFP, which are regressed empirically without and with control variables. Column (1) of Table 4 shows the results without adding control variables, which shows that the estimated coefficient is 1.464 and is significantly positive at the 1% level, which indicates that digital village construction can promote AGTFP; thus, hypothesis 1 is initially verified. On this basis, by introducing the control variables of the income distribution, financial expenditure on agriculture, agricultural disaster rate, agricultural machinery density, and agricultural structure, the regression coefficient becomes 0.817. Its effect on AGTFP is still significant at the 1% level. Regardless of the insertion of control factors, the results demonstrate that the influence of digital village construction on AGTFP is considerably positive at the 1% level, revealing that digital village construction is essential for encouraging AGTFP. Hypothesis 1 is proved. The extant literature supports this conclusion. Using a sample of 1740 county-level administrative units in 2019, Du J. et al. (2023) demonstrated the role of digital village construction in enhancing AGTFP. Despite some differences in the data samples used, the consistency of the findings strongly supports the reliability of the conclusions presented in this paper.
Regarding the control variables, AGTFP is significantly improved by the income distribution gap between urban and rural areas. This finding reveals that moderate income disparity can effectively incentivize agricultural production to shift to greener and more efficient production methods, thus enhancing AGTFP. However, the effects of financial expenditure on agricultural support and machinery density on AGTFP are significantly adverse. The possible reason is that the current development model in China still relies mainly on traditional methods of resource consumption. In this context, financial support for agriculture may inadvertently intensify inputs to the traditional agricultural model, which not only exacerbates the demand for natural resources but also exacerbates the problem of environmental pollution, which is not conducive to enhancing AGTFP. The use of agricultural machinery increases fuel consumption, which in turn increases greenhouse gas emissions, adversely affecting the ecology and thus limiting the positive role of agricultural mechanization in promoting AGTFP. In addition, the effect of the agricultural disaster rate on AGTFP is negatively correlated. Besides, the influence of agricultural structure shows a positive correlation, but neither effect reaches a statistically significant level.
The following five robustness tests are used to verify the robustness of the influence of digital village construction on AGTFP.
The previous section used the GML index method to calculate China’s AGTFP. To examine the robustness of the results, AGTFP is re-measured in this section using the CCR model, a more traditional DEA model for evaluating the size and technical efficiency of DMUs, which has strong feasibility and applicability in practical applications. By adopting the new calculation method to estimate AGTFP and including it as the dependent variable in the regression model, the results are displayed in column (1) of Table 5. The results show that the digital village construction regression coefficient on AGTFP drops to 0.175, a reduction compared to the baseline regression results. This phenomenon is attributed to the inherent differences in the measurement of efficiency values between the CCR model and the super-efficiency SBM model, highlighting the precision differences between the models in measuring efficiency. Nonetheless, the regression coefficients for digital village construction remain positive and significant at the 1% level even after adjusting for the different measures. This result confirms that the benchmark regression results are reliable.
The entropy method measures the core independent variable digital village construction in the benchmark regression. To avoid interference from different measurement methods in the results, this section uses principal component analysis to measure the degree of digital village construction again. Principal component analysis is a multivariate statistical analysis technique that captures the critical information embedded in the raw variables by extracting a few linear combinations. This approach effectively avoids the repetition of information owing to the increased number of indicators, reduces redundancy, and improves the efficiency of data processing, leading to a more precise assessment of the characteristics and evolutionary trends of the research object. After being re-measured via principal component analysis, the variables related to digital village construction are substituted into the model and re-examined. As indicated in column (2) of Table 5, the regression coefficient for digital village construction on AGTFP is 0.186. It is significantly positive at the 1% level. The difference in the results stems from the differing data processing methods of the two approaches. The entropy method emphasizes the informativeness and balance of the indicators. In contrast, the principal component analysis method prioritizes extracting the central variability of the data. Even with different measurement methods, the positive effect of digital village construction on AGTFP is still significant, indicating the robustness of the above findings.
Compared with other general provinces, municipalities have unique characteristics in terms of administrative level, geographic factors, economic positioning, and agricultural development conditions. To ensure the comparability and dependability of the results and to avoid the potential interference of these unique factors on the model estimation, the municipalities in China, Beijing, Tianjin, Chongqing, and Shanghai, were removed from the model analysis in this paper. Using the new samples, the model is re-estimated. Column (3) of Table 5 gives the results. After excluding the municipalities’ sample, the digital village construction’s positive impact on AGTFP becomes significantly higher, with the regression coefficient rising to 1.145. The possible reason is that municipalities have limited environmental carrying capacity. Thus, their agricultural policies may emphasize ecological protection and sustainable development more. While digital village construction is essential for promoting green agricultural development, its impact on municipalities may be weaker due to existing local environmental policies. The results above reaffirm the reliability of the paper’s conclusions.
Extreme values or outliers may greatly impact statistical analyses and model estimation. The disturbance of these outliers on the analytical results can be reduced by shrinking the tails. To ensure the dependability of the analytical results and reduce the possible disturbance of outliers on model estimation, the main variables were subjected to tailoring at the 1% quartile. After the trimming of the variables, the model was re-estimated with parameters. As indicated in column (4) of Table 5, the coefficient for digital village construction remains significantly positive even after accounting for possible bias from extreme values. This finding suggests that digital village construction positively enhances AGTFP and that this effect is stable and reliable.
Within the framework of the existing control variables, the degree of industrialization and the state of rural roads are further introduced as control variables to enhance the explanatory power and robustness of the model. The percentage of industrial-added value to gross regional product signifies industrialization. The state of rural roads is represented by the ratio of (total highway mileage of the province—highway mileage—first-class highway mileage) to the province’s area. After re-estimating the model, column (5) of Table 5 depicts the regression results. The regression coefficient remains significantly positive even after incorporating a more comprehensive set of control variables. This finding further strengthens the previous conclusion that digital village construction positively affects AGTFP and that this effect is robust across different model settings.
The Endogeneity issues may challenge the findings of the previous study. Digital village construction uses information technology to increase AGTFP. However, regions with greater AGTFP are also likely to have higher requirements for infrastructure, information technology, etc., which drives digital village construction. To mitigate the impact of this reverse causation on the results as much as possible, this paper adopts an instrumental variables(IV) approach by employing the two-stage least squares.
Referring to Bartik (2009), the Bartik IV is constructed, where is the degree of digital village construction lagged by one period and is the first-order difference at the national level of digital village construction over time. Bartik’s instrumental variables can effectively mitigate endogeneity problems caused by reverse causality. This instrumental variable theoretically satisfies the two essential properties required of an instrumental variable. In terms of correlation, this variable includes one lagged period of digital village construction, and the level of digital village construction in the current period is inevitably affected by the level of digital village construction in the lagged period. Therefore, this instrumental variable is highly correlated with the endogenous variables in this paper. From the perspective of exogeneity, the exogeneity of this instrumental variable mainly derives from different terms. Since the level of digital village construction at the national level is derived from the mean value synthesized by the 31 provincial administrative regions, its trend will not be significantly affected by individual provincial administrative areas. The difference term is exogenous relative to individual regions. In summary, the only channel through which this instrumental variable affects the dependent variable is the associated endogenous independent variable, ensuring the original endogenous independent variable is estimated consistently.
The Bartik IV regression results are illustrated in columns (1) and (2) of Table 6. Apart from this, this paper adopted the method of Chen and Chu (2023) to estimate the first-order difference value of the digital village construction level in each year of the country. It implements the de-one method (LOO) processing. This step is intended to reduce the effect of the increase in digital village construction in a single region on the overall national growth rate. The regression results using the LOO method are displayed in columns (3) and (4) of Table 6.
The initial stage regression results are displayed in columns (1) and (3). The hypothesis of weak IV is rejected since the F values of the two instrumental factors in the first stage are more significant than 10. The Cragg-Donald Wald F-statistic is bigger than the crucial value of 16.38 under 10% bias. Columns (2) and (4) show the results for the second stage IV, demonstrating that after identifying the endogeneity issue, digital village construction still significantly impacts AGTFP, further verifying hypothesis 1. The estimation results in Table 6 indicate that digital village construction can promote AGTFP, regardless of whether the IV is constructed using the Bartik or LOO methods.
To circumvent the problems of overuse and endogeneity bias present in the traditional step-by-step test of mediating effects, this paper relies on the two-step method proposed by Jiang (2022) to conduct the mediating effects test. The impact of each mediating variable on AGTFP has already been verified through the literature review. Therefore, this section focuses on whether digital village construction can influence the mediating variables, thus verifying the mediatings effect.
Agricultural technology innovation csan minimize the consumption of resources by improving resource use efficiency while lowering the utilization of pesticides and fertilizers to reduce environmental pollution. Numerous studies have confirmed that agricultural technology innovation has an advantageous influence on AGTFP (Zhang et al., 2022; Dai et al., 2023; Liu Y. et al., 2023). Thus, this investigation covers only the effect of digital village construction on agricultural technology innovation. As shown in column (1) of Table 7, the main regression coefficient is 2.652, indicating that digital village construction can significantly promote agricultural technology levels. Through the integration and application of modern information technology, digital village construction has provided significant impetus to agricultural technological innovation and accelerated the practical application of these innovations. The enhancement of agricultural technology innovation improves the efficiency of agricultural resources and energy use, thereby promoting the enhancement of AGTFP. Therefore, digital village construction significantly promotes agricultural technology innovation, thus facilitating the improvement of AGTFP. It verifies hypothesis 2.
As an endogenous driver of agricultural growth, human capital in agriculture plays a crucial part in promoting the green transformation of agriculture. It constitutes an innovative starting point for sustainable agricultural development and an essential component in realizing this transformation. The favorable effect of agricultural human capital accumulation on agricultural green growth has been confirmed by the lSterature (Ren et al., 2022; Wang Y. et al., 2023). As shown in column (2) of Table 7, the regression coefficient is 0.389, indicating that digital village construction can enhance rural human capital. Digital village construction has not only attracted a large number of technical and managerial talents but also provided opportunities for knowledge updating and skills upgrading in rural areas. By leveraging network platforms, digital village construction can promote the cross-regional sharing of educational resources and improve the accessibility of rural education. Through these channels, rural human capital has been gradually upgraded, thereby providing intellectual support for the green and intelligent transformation of agriculture. Therefore, digital village construction enhances AGTFP by facilitating the accumulation of agricultural human capital. It verifies hypothesis 3.
Strengthening the construction and improvement of agricultural productive services is of great significance in promoting the process of agricultural modernization. Numerous academics have studied the relationship between agricultural productive services and agricultural sustainable growth, and they all concur that these services can increase AGTFP (Zhu et al., 2022a; Bai et al., 2023; Tang et al., 2023). Column (3) of Table 7 shows the results, and the coefficient is 0.796 and is positive at the 1% level, showing that digital village construction can improve agricultural productive services. Digital village construction further encourages the growth of AGTFP by optimizing agricultural productive services, such as providing timely market information, efficient supply chain management, and high-quality policy advisory services, thereby enhancing the quality and accessibility of services. Therefore, digital village construction enhances AGTFP by improving agricultural productive services. It verifies hypothesis 4.
To arrange agricultural output in the most efficient way possible, guarantee food security, and provide an adequate quantity of necessary agricultural products, the Ministry of Agriculture and Rural Development of China issued the National Cultivation Structure Adjustment Plan (2016–2020), which divides 31 provinces into 13 major grain-producing areas and 18 non-major grain-producing areas, considering the specificities of different regions in terms of food consumption and production. Differences in agricultural policies, planting structures, and production methods in various functional areas can impact the AGTFP. Therefore, according to the functional attributes of agriculture, the sample is divided into two areas to conduct regressions separately to examine the impact of digital village construction on AGTFP in areas with different functional attributes. According to columns (1) and (2) of Table 8, the effect of digital village construction on AGTFP is significantly positive at the 5% level in both areas. The difference is that the regression coefficient of digital village construction is more significant than that of non-major grain-producing areas in major grain-producing areas, indicating that digital village construction can promote AGTFP in major grain-producing areas. Slightly different from the division adopted in this paper, Guo and Liu (2023) subdivided non-major grain-producing areas into food marketing and balance areas. However, their study demonstrates that digital village construction significantly affects AGTFP in major grain-producing areas.
The possible reason for this is that the major grain-producing areas, due to their rich agricultural production resources and strong policy support, tend to be the pioneer zones of agricultural modernization and agricultural technology innovation, which means that the major grain-producing areas not only possess progressive agricultural technology but also show significant advantages in agricultural management and operation. In addition, because of their large-scale agricultural production activities, major grain-producing areas are more likely to attract new agricultural management bodies, which adopt advanced technologies and foster a green revolution in agriculture, thus enhancing the effectiveness of digital village construction in major grain-producing areas.
At present, the problem of unbalanced regional progress in China still exists. There is a particular gap in each region’s economic development level, which also influences agricultural production. A division into economically developed and underdeveloped areas comprises 31 provinces determined by the per capita GDP division standard. The influence of digital village construction on AGTFP under different stages of economic growth is then empirically examined to determine whether there is any variability. Digital village construction favors AGTFP in developed and underdeveloped areas, as seen by Table 8’s columns (3) and (4). The influence of digital village construction is insignificant in the developed areas.
Conversely, at the 1% level, the impact is higher and more substantial in underdeveloped areas. The potential cause is relatively limited infrastructure construction in underdeveloped areas. Promoting digital village construction can rapidly improve infrastructure conditions, such as network coverage and informationisation in these regions, thus directly promoting the green development of local agriculture. In addition, digital village construction can also encourage the transformation of traditional agriculture to modern agriculture through the adoption of high-end technologies and intelligent equipment, improve the efficiency of resource allocation, and promote the enhancement of AGTFP in underdeveloped areas. Furthermore, areas with prosperous economies tend to focus more on environmental concerns and employ various tactics to lessen the damaging effects of agriculture on ecosystems.
Moreover, the infrastructure and agricultural technology in economically developed areas are already more perfect, so digital village construction can only play a role in optimization and enhancement, with relatively little impact on the greening of agriculture. The role of digital village construction on AGTFP is more significant in economically underdeveloped areas.
In tandem with the growth of the regional economy in China, new challenges and problems have gradually emerged, among which the tendency toward uneven economic growth is becoming more noticeable. The center of gravity of economic activities has continued to tilt toward the South, causing the developmental differences between the North and the South to become a focus of attention gradually. The Qinling-Huaihe line is an essential demarcation between northern and southern China, and it has a significant distinguishing role in many aspects, including nature, culture, and agriculture. Therefore, following the traditional division, using the Qinling-Huaihe River as the boundary, the 31 provinces are separated into two sub-samples, the South and the North, and regression analyses are conducted separately. As depicted in columns (5) and (6) of Table 8, the influence of digital village construction on AGTFP is significantly positive in both regions, indicating that digital village construction has boosted AGTFP in both regions. However, the regression coefficients show that digital village construction contributes more significantly to the green development of agriculture in the North and has the second highest impact on agriculture in the South. The possible reason is that, on the one hand, the terrain in the North is dominated by plains and plateaus, which are conducive to mechanized farming. In addition to increasing agricultural productivity efficiency, it also establishes the groundwork for greening agriculture.
On the other hand, as the core region of China’s grain production, the North, especially the Northeast, has a large scale and standardization of agricultural output, which helps promote green agricultural technologies and management practices. The northern region’s topography and degree of agricultural scale give a solid basis for developing digital villages, which helps foster the greening of the region’s agriculture. Therefore, its positive effects are more significant.
This section incorporates environmental regulation as a threshold factor to investigate further the function of digital village construction on AGTFP. The following is the model.
In Equation 6, is the threshold variable. is the indicator function. The condition in the brackets is assigned a value of 1 if it is accurate and 0 otherwise. represents the estimated threshold. Since Equation 6 defines a single threshold, the multi-threshold model can be developed in line with it. Table 9 exhibits the outcome of a 300-time sample for the threshold effect test conducted using the self-help sampling approach. The findings of the threshold test show that the p value corresponding to the threshold variable is significant only under the single-threshold model. At the same time, the model has only a single-threshold effect, with a threshold value of 0.0410, as the double-threshold fails the significance test.
Table 10 exhibits the findings of the threshold regression. If environmental regulation is below 0.0410, the coefficient is 3.234. Above 0.0410, it is 2.155. The significance test is passed in both phases, suggesting a consistent positive impact of digital village construction on AGTFP. There is a single threshold effect based on the intensity of environmental regulation. Hypothesis 5 is verified. Notably, once the regulatory level exceeds the threshold, there is a tendency for the facilitating role of digital village construction on AGTFP to weaken.
Digital village construction can significantly contribute to AGTFP when environmental regulation is low. During this period, environmental regulation pressure has not yet reached a critical point, and the agricultural sector exhibits greater flexibility and enthusiasm in adopting and innovating green technologies. Specifically, moderate environmental regulation can stimulate agricultural technology innovation by agricultural technicians, encourage producers to adopt environmentally friendly production technologies and methods and motivate the service industry to promote green agricultural practices. This combination of moderate environmental regulation and digital village construction effectively enhances AGTFP. However, if the intensity of environmental regulation exceeds the threshold, the impact of digital village construction on agricultural greening weakens. The reasons for this can be summarized as follows: first, under high-intensity environmental regulation, agricultural technological innovations may focus too much on environmental adaptability, neglecting productivity and economic efficiency. Second, meeting stricter environmental standards requires agricultural producers to invest more in environmental management. These additional costs create financial pressure for agricultural producers, hindering their continued investment in green technologies.
Drawing on the balanced dataset encompassing 31 provinces in China from 2011 to 2022, this paper first constructs an evaluation index system of digital village construction and AGTFP. Then, the fixed effects model was leveraged to evaluate the role of digital village construction in enhancing AGTFP. Second, by constructing a mediation model, we explored the fundamental pathways by which digital village construction impacts AGTFP. Furthermore, we scrutinize the uniformity of digital village construction’s effects on AGTFP across various scenarios. Finally, the paper employs a threshold model to identify whether environmental regulation is a threshold factor in the link between digital village construction and AGTFP. The paper’s principal results are: (1) China’s AGTFP has been trending upward in recent years. (2) Digital village construction significantly increases AGTFP, a conclusion supported by numerous robustness and endogeneity tests. This finding highlights the positive economic consequences of digital village construction and identifies critical drivers for greening agriculture. (3) Digital village construction can promote agricultural technology innovation, enhance agricultural human capital, and improve agricultural productive services, thereby promoting AGTFP. This paper further enriches the theoretical framework of the field. (4) The favorable outcome of digital village construction on AGTFP is more significant in major grain-producing areas, economically underdeveloped areas, and northern region. (5) The influence of digital village construction on AGTFP is subject to a single threshold effect of environmental regulations. This finding provides policymakers with a reference for formulating environmental regulations. It opens new research paths and perspectives for future studies.
As an emerging research field, digital village construction is showing vigorous development. Future research can explore the impact of digital village construction on the agricultural industry, rural communities, and individual farmers. Additionally, future studies can use micro-level databases to more accurately and carefully assess the impact of digital village construction on agriculture.
1. Strengthening digital infrastructure in rural areas is essential to address agricultural deficiencies. Firstly, the government should allocate more funds to build digital infrastructure for agriculture, expand network coverage in rural areas, and reduce the digital divide between urban and rural areas. Secondly, the digitalization of traditional rural infrastructure should be reinforced to enhance its service capacity and efficiency. Additionally, to expand funding channels and improve financing efficiency, it is crucial to support innovation in the rural digital infrastructure investment and financing system and attract social capital for this initiative.
2. Village talent should be nurtured to bridge the digital divide. The government should increase rural education, training funding, and subsidies to enhance farmers’ knowledge. Simultaneously, training programs should be designed to meet the actual demands for occupational skills that farmers require. Additionally, the innovation and promotion of green technologies in agriculture are inseparable from high-quality talent. Robust social security systems, enhanced public services, and well-designed talent development programs are essential for rural communities to create an environment conducive to attracting, developing, and retaining talent.
3. Regions should be encouraged to innovate and formulate digital village strategies tailored to their local characteristics. Firstly, regions with remarkable results should summarize and share their outstanding experiences to facilitate mutual learning and progress. Secondly, each area should assess its geographic position, economic foundation, and social and cultural background to develop region-specific digital village strategies and models for green agricultural growth. Social organizations, enterprises, institutions of higher learning, and research institutions should be encouraged to engage in the process, brainstorming new paths for digital village construction.
4. The balance between environmental protection and economic development should be controlled, and the intensity of environmental regulation should be flexibly adjusted. Firstly, environmental regulation policies should take a long-term perspective, considering their long-term impact and overall benefits for sustainable agricultural development. Secondly, the participation of stakeholders, including farmers, environmental organizations, and scientific research institutes, should be promoted in the policy formulation process to enhance transparency and public participation. Additionally, the effectiveness of environmental regulation should be regularly reviewed and updated based on agricultural development, technological progress, and social change. Adequate environmental regulation is fundamental to advancing sustainable and green farming practices. By reasonably regulating the strength of the regulation, farmers can be effectively incentivized to adopt green technologies and elevate productivity and product excellence in farming, thus achieving both farming efficiency and environmental safeguards.
The raw data supporting the conclusions of this article will be made available by the authors, without undue reservation.
QC: Conceptualization, Project administration, Validation, Writing – review & editing. XH: Data curation, Formal analysis, Methodology, Software, Visualization, Writing – original draft, Writing – review & editing.
The author(s) declare that financial support was received for the research, authorship, and/or publication of this article. This work was supported by Soft Science Research Program Project of Henan Province (No. 242400411098); the Plan for Key Research Projects of Henan Province Higher Education Institutions (No. 24B790026).
The authors declare that the research was conducted in the absence of any commercial or financial relationships that could be construed as a potential conflict of interest.
All claims expressed in this article are solely those of the authors and do not necessarily represent those of their affiliated organizations, or those of the publisher, the editors and the reviewers. Any product that may be evaluated in this article, or claim that may be made by its manufacturer, is not guaranteed or endorsed by the publisher.
Adamowicz, M., and Zwolińska-Ligaj, M. (2020). The “Smart Village” as a way to achieve sustainable development in rural areas of Poland. Sustain. For. 12:6503. doi: 10.3390/su12166503
Akzar, R., and Amandaria, R. (2021). "Climate change effects on agricultural productivity and its implication for food security", in: IOP conference series: Earth and environmental science: IOP Publishing), 012059.
Arts, K., Ioris, A. A., Macleod, C. J., Han, X., Sripada, S. G., Braga, J. R., et al. (2016). Environmental communication in the information age: institutional barriers and opportunities in the provision of river data to the general public. Environ. Sci. Pol. 55, 47–53. doi: 10.1016/j.envsci.2015.08.011
Audretsch, D. B., Heger, D., and Veith, T. (2015). Infrastructure and entrepreneurship. Small Bus. Econ. 44, 219–230. doi: 10.1007/s11187-014-9600-6
Bai, Z., Wang, T., Xu, J., and Li, C. (2023). Can agricultural productive services inhibit carbon emissions? Evidence from China. Land 12:1313. doi: 10.3390/land12071313
Baležentis, T., Blancard, S., Shen, Z., and Štreimikienė, D. (2021). Analysis of environmental total factor productivity evolution in European agricultural sector. Decis. Sci. 52, 483–511. doi: 10.1111/deci.12421
Barbera, A. J., and McConnell, V. D. (1990). The impact of environmental regulations on industry productivity: direct and indirect effects. J. Environ. Econ. Manag. 18, 50–65. doi: 10.1016/0095-0696(90)90051-Y
Bartik, T. J. (2009). How do the effects of local growth on employment rates vary with initial labor market conditions? Upjohn Working Papers & Journal Articles.
Cai, Z., Li, S., and Cheng, D. (2023). Has Digital Village construction improved rural family resilience in China? Evidence based on China household finance survey. Sustain. For. 15:8704. doi: 10.3390/su15118704
Chen, Z., and Chu, Y. (2023). The impact of digital transformation on Chinese commercial banks’ risk-taking. J. World Econ. 3, 85–88. doi: 10.56397/JWE.2024.06.09
Chen, C., Wang, J., Wang, X., Duan, W., and Xie, C. (2024). Does rural industrial integration promote the green development of agriculture?–based on data from 30 provinces in China from 2010 to 2021. Pol. J. Environ. Stud. 33, 1569–1583. doi: 10.15244/pjoes/174479
Chen, W., Wang, Q., and Zhou, H. (2022). Digital rural construction and farmers’ income growth: theoretical mechanism and micro experience based on data from China. Sustain. For. 14:11679. doi: 10.3390/su141811679
Dai, X., Chen, Y., Zhang, C., He, Y., and Li, J. (2023). Technological revolution in the field: green development of Chinese agriculture driven by digital information technology (DIT). Agriculture 13:199. doi: 10.3390/agriculture13010199
Dou, Y., Deng, Y., and Chen, S. (2016). China's agricultural environment pollution present situation and the technologicai innovation path. Sci. Manag. Res. 34, 76–79. doi: 10.19445/j.cnki.15-1103/g3.2016.04.020
Du, J., Zhang, Y., Liu, B., and Dong, R. (2023). Impact of digital village construction on agricultural green total factor productivity and its mechanisms. China Popul. Resour. Environ. 33, 165–175. doi: 10.12062/cpre.20221013
Du, F., Zhao, X., Zheng, J., and Zhang, M. (2023). Can digital literacy promote green production for farmers?—Based on CLES data. World Agric. 10, 97–109. doi: 10.13856/j.cn11-1097/s.2023.10.009
Dubey, A., and Lal, R. (2009). Carbon footprint and sustainability of agricultural production systems in Punjab, India, and Ohio, USA. J. Crop Improv. 23, 332–350. doi: 10.1080/15427520902969906
Fang, L., Hu, R., Mao, H., and Chen, S. (2021). How crop insurance influences agricultural green total factor productivity: evidence from Chinese farmers. J. Clean. Prod. 321:128977. doi: 10.1016/j.jclepro.2021.128977
Fang, Z., Li, G., Yin, Y., and Liu, P. (2024). Measurement of rural digital economy development level and its impact on agricultural productivity growth. J. China Agric. Univ. 29, 252–268. doi: 10.11841/j.issn.1007-4333.2024.05.23
Gao, Q., Cheng, C., Sun, G., and Li, J. (2022). The impact of digital inclusive finance on agricultural green total factor productivity: evidence from China. Front. Ecol. Evol. 10:905644. doi: 10.3389/fevo.2022.905644
Granell, C., Havlik, D., Schade, S., Sabeur, Z., Delaney, C., Pielorz, J., et al. (2016). Future internet technologies for environmental applications. Environ. Model Softw. 78, 1–15. doi: 10.1016/j.envsoft.2015.12.015
Guo, H., and Liu, X. (2023). Can digital rural development promote agricultural green Total factor productivity by reducing resource element mismatch? Ningxia Soc. Sci. 5, 107–117. doi: 10.3969/j.issn.1002-0292.2023.05.013
Hao, A., Hou, Y., and Tan, J. (2022). How does digital village construction influences carbon emission? The case of China. Plos one 17:e0278533. doi: 10.1371/journal.pone.0278533
Ji, X., Xu, J., and Zhang, H. (2023). Environmental effects of rural e-commerce: a case study of chemical fertilizer reduction in China. J. Environ. Manag. 326:116713. doi: 10.1016/j.jenvman.2022.116713
Jiang, T. (2022). Mediating effects and moderating effects in causal inference. China Ind. Econ. 5, 100–120. doi: 10.3969/j.issn.1006-480X.2022.05.007
Jorgenson, D. W., and Gollop, F. M. (1992). Productivity growth in US agriculture: a postwar perspective. Am. J. Agric. Econ. 74, 745–750. doi: 10.2307/1242588
Koiry, S., and Huang, W. (2023). Do ecological protection approaches affect total factor productivity change of cropland production in Sweden? Ecol. Econ. 209:107829. doi: 10.1016/j.ecolecon.2023.107829
Li, B., Zhang, J. B., and Li, H. (2011). Research on spatial-temporal characteristics and affecting factors decomposition of agricultural carbon emission in China. China Popul. Resour. Environ. 21, 80–86. doi: 10.3969/j.issn.1002-2104.2011.08.013
Lin, Y., and Li, C. (2023). Towards sustainable development: research on the green growth effect of digital agriculture in China. Environ. Sci. Pollut. Res., 1–14. doi: 10.1007/s11356-023-28367-2
Liu, Y., Ji, D., Zhang, L., An, J., and Sun, W. (2021). Rural financial development impacts on agricultural technology innovation: evidence from China. Int. J. Environ. Res. Public Health 18:1110. doi: 10.3390/ijerph18031110
Liu, Y., Lu, C., and Chen, X. (2023). Dynamic analysis of agricultural green development efficiency in China: spatiotemporal evolution and influencing factors. J. Arid. Land 15, 127–144. doi: 10.1007/s40333-023-0007-6
Liu, S., Zhu, S., Hou, Z., and Li, C. (2023). Digital village construction, human capital and the development of the rural older adult care service industry. Front. Public Health 11:1190757. doi: 10.3389/fpubh.2023.1190757
Luo, J., Huang, M., Hu, M., and Bai, Y. (2023). How does agricultural production agglomeration affect green total factor productivity? Empirical evidence from China. Environ. Sci. Pollut. Res. 30, 67865–67879. doi: 10.1007/s11356-023-27106-x
Lv, C., Shao, C., and Lee, C.-C. (2021). Green technology innovation and financial development: do environmental regulation and innovation output matter? Energy Econ. 98:105237. doi: 10.1016/j.eneco.2021.105237
Ma, W., and Han, W. (2023). The internal logic and realization path of digital economy enabling rural revitalizatior. Tianjin Soc. Sci. 3, 91–98. doi: 10.16240/j.cnki.1002-3976.2023.03.009
Mei, Y., Miao, J., and Lu, Y. (2022). Digital villages construction accelerates high-quality economic development in rural China through promoting digital entrepreneurship. Sustain. For. 14:14224. doi: 10.3390/su142114224
Myeki, L. W., Matthews, N., and Bahta, Y. T. (2023). Decomposition of green agriculture productivity for policy in Africa: an application of global Malmquist–Luenberger index. Sustain. For. 15:1645. doi: 10.3390/su15021645
Oskam, A. (1991). Productivity measurement, incorporating environmental effects of agricultural production. In K. Burger, M. GrootDe, J. Post, and V. Zachariasse, eds., Agricultural Economics and Policy: International Challenges for the Nineties, Elsevier, Amsterdam, 186–204
Porter, M. E., and Linde, C. V. D. (1995). Toward a new conception of the environment-competitiveness relationship. J. Econ. Perspect. 9, 97–118. doi: 10.1257/jep.9.4.97
Ren, J., Lei, H., and Ren, H. (2022). Livelihood capital, ecological cognition, and farmers’ green production behavior. Sustain. For. 14:16671. doi: 10.3390/su142416671
Rockström, J., Williams, J., Daily, G., Noble, A., Matthews, N., Gordon, L., et al. (2017). Sustainable intensification of agriculture for human prosperity and global sustainability. Ambio 46, 4–17. doi: 10.1007/s13280-016-0793-6
Shen, F., and Ye, W. (2021). Digital rural construction: a strategic choice to realize the revitalization of high-quality rural areas. J. Nanjing Agric. Univ. (Soc. Sci. Edit.) 21, 41–53. doi: 10.19714/j.cnki.1671-7465.2021.0071
Shin, D.-H., and Choi, M. J. (2015). Ecological views of big data: perspectives and issues. Telematics Inform. 32, 311–320. doi: 10.1016/j.tele.2014.09.006
Sims, B., and Kienzle, J. (2017). Sustainable agricultural mechanization for smallholders: what is it and how can we implement it? Agriculture 7:50. doi: 10.3390/agriculture7060050
Sun, Y. (2022). Environmental regulation, agricultural green technology innovation, and agricultural green total factor productivity. Front. Environ. Sci. 10:955954. doi: 10.3389/fenvs.2022.955954
Tang, Y., and Chen, M. (2022). The impact mechanism and spillover effect of digital rural construction on the efficiency of green transformation for cultivated land use in China. Int. J. Environ. Res. Public Health 19:16159. doi: 10.3390/ijerph192316159
Tang, W., Zhou, F., Peng, L., and Xiao, M. (2023). Does agricultural productive service promote agro-ecological efficiency? Evidence from China. Therm. Sci. 27, 2109–2118. doi: 10.2298/TSCI2303109L
Tone, K. (2001). A slacks-based measure of efficiency in data envelopment analysis. Eur. J. Oper. Res. 130, 498–509. doi: 10.1016/S0377-2217(99)00407-5
Tone, K. (2002). A slacks-based measure of super-efficiency in data envelopment analysis. Eur. J. Oper. Res. 143, 32–41. doi: 10.1016/S0377-2217(01)00324-1
Wang, H., Cui, H., and Zhao, Q. (2021). Effect of green technology innovation on green total factor productivity in China: evidence from spatial durbin model analysis. J. Clean. Prod. 288:125624. doi: 10.1016/j.jclepro.2020.125624
Wang, F., Du, L., and Tian, M. (2022). Does agricultural credit input promote agricultural green total factor productivity? Evidence from spatial panel data of 30 provinces in China. Int. J. Environ. Res. Public Health 20:529. doi: 10.3390/ijerph20010529
Wang, P., Li, C., and Huang, C. (2023). The impact of digital village construction on county-level economic growth and its driving mechanisms: evidence from China. Agriculture 13:1917. doi: 10.3390/agriculture13101917
Wang, S., Yu, N., and Fu, R. (2021). Digital rural construction: action mechanism, realistic challenge andimplementation strategy. Reform 4, 45–59.
Wang, Y., Zhao, Z., Xu, M., Tan, Z., Han, J., Zhang, L., et al. (2023). Agriculture–tourism Integration’s impact on agricultural green productivity in China. Agriculture 13:1941. doi: 10.3390/agriculture13101941
Wei, Z., Qi, L., and Wang, R. (2022). The relationship between farm size and fertilizer use efficiency: evidence from China. J. Integr. Agric. 21, 273–281. doi: 10.1016/S2095-3119(21)63724-3
West, T. O., and Marland, G. (2002). A synthesis of carbon sequestration, carbon emissions, and net carbon flux in agriculture: comparing tillage practices in the United States. Agric. Ecosyst. Environ. 91, 217–232. doi: 10.1016/S0167-8809(01)00233-X
Xu, Q., Zhu, P., and Tang, L. (2022). Agricultural services: another way of farmland utilization and its effect on agricultural green total factor productivity in China. Land 11:1170. doi: 10.3390/land11081170
Yang, J., Huang, Z., Zhang, X., and Reardon, T. (2013). The rapid rise of cross-regional agricultural mechanization services in China. Am. J. Agric. Econ. 95, 1245–1251. doi: 10.1093/ajae/aat027
Yu, D., Liu, L., Gao, S., Yuan, S., Shen, Q., and Chen, H. (2022). Impact of carbon trading on agricultural green total factor productivity in China. J. Clean. Prod. 367:132789. doi: 10.1016/j.jclepro.2022.132789
Zanello, G. (2012). Mobile phones and radios: effects on transactions costs and market participation for households in northern Ghana. J. Agric. Econ. 63, 694–714. doi: 10.1111/j.1477-9552.2012.00352.x
Zhang, L., Bai, Y., Sun, M., Xu, X., and He, J. (2021). Views on agricultural green production from the perspective of system science. Issues Agric. Econ. 10, 42–50. doi: 10.13246/j.cnki.iae.2021.10.004
Zhang, F., Wang, F., Hao, R., and Wu, L. (2022). Agricultural science and technology innovation, spatial spillover and agricultural green development—taking 30 provinces in China as the research object. Appl. Sci. 12:845. doi: 10.3390/app12020845
Zhang, X., and Zhang, Z. (2020). How do smart villages become a way to achieve sustainable development in rural areas? Smart village planning and practices in China. Sustain. For. 12:10510. doi: 10.3390/su122410510
Zhang, R., and Zhong, C. (2023). Digital Village construction and county ecological environment quality empirical evidence from comprehensive demonstration policiesfor e-commerce going to rural areas. Contemp. Econ. Manage. 45, 54–65. doi: 10.13253/j.cnki.ddjjgl.2023.02.008
Zhao, X., and Zhao, R. (2024). The impact and mechanism of digital villages on agricultural resilience in ecologically fragile ethnic areas: evidence from China’s provinces. Agriculture 14:221. doi: 10.3390/agriculture14020221
Zhu, Y., Deng, J., Wang, M., Tan, Y., Yao, W., and Zhang, Y. (2022a). Can agricultural productive services promote agricultural environmental efficiency in China? Int. J. Environ. Res. Public Health 19:9339. doi: 10.3390/ijerph19159339
Keywords: digital village construction, agricultural green total factor productivity, agricultural technology innovation, agricultural human capital, agricultural productive services, environmental regulation
Citation: Cai Q and Han X (2024) Impacts and mechanisms of digital village construction on agricultural green total factor productivity. Front. Sustain. Food Syst. 8:1431294. doi: 10.3389/fsufs.2024.1431294
Received: 11 May 2024; Accepted: 12 September 2024;
Published: 25 September 2024.
Edited by:
Isabelle Piot-Lepetit, INRAE Occitanie Montpellier, FranceReviewed by:
Hao Aimin, Zhengzhou University of Aeronautics, ChinaCopyright © 2024 Cai and Han. This is an open-access article distributed under the terms of the Creative Commons Attribution License (CC BY). The use, distribution or reproduction in other forums is permitted, provided the original author(s) and the copyright owner(s) are credited and that the original publication in this journal is cited, in accordance with accepted academic practice. No use, distribution or reproduction is permitted which does not comply with these terms.
*Correspondence: Xinyu Han, aGFueGlueGluMDkyNUAxNjMuY29t
Disclaimer: All claims expressed in this article are solely those of the authors and do not necessarily represent those of their affiliated organizations, or those of the publisher, the editors and the reviewers. Any product that may be evaluated in this article or claim that may be made by its manufacturer is not guaranteed or endorsed by the publisher.
Research integrity at Frontiers
Learn more about the work of our research integrity team to safeguard the quality of each article we publish.