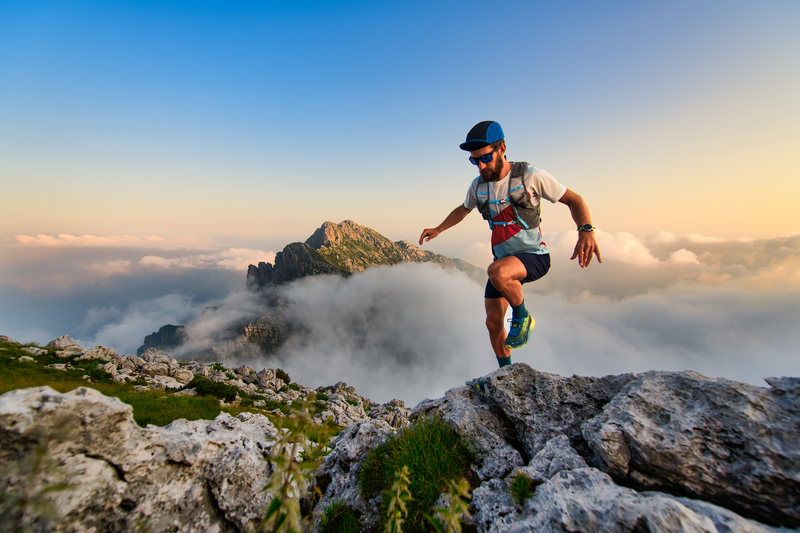
95% of researchers rate our articles as excellent or good
Learn more about the work of our research integrity team to safeguard the quality of each article we publish.
Find out more
ORIGINAL RESEARCH article
Front. Sustain. Food Syst. , 16 December 2024
Sec. Agricultural and Food Economics
Volume 8 - 2024 | https://doi.org/10.3389/fsufs.2024.1430670
This article is part of the Research Topic Economic visions to mitigate Climate Change View all 4 articles
Introduction: Pollutants from farming and rural life have a serious negative impact on water and soil. The advantages of green finance in financial support and credit management can guide the green transformation of agricultural production, thus protecting the ecological environment.
Methods: Based on panel data from 30 provinces in China from 2005 to 2021, the study used inventory analysis, entropy method and two-way fixed effect model to analyze the impact of green finance on agricultural non-point source pollution (ANSP) and the mediating mechanism.
Results: ANSP has experienced an inverted U-shaped trend of first rising and then falling, and the rural ecological environment is improving. At the time level, the ANSP produced by agricultural production in China experienced an inverted U-shaped evolution trend of first increasing and then decreasing during the sample period. At the spatial level, ANSP has a significant low regional differentiation. The ANSP of the central region and the coastal economic development area is higher than that of the western region. The results of linear regression show that green finance has a negative effect on ANSP. Promoting green finance in rural areas can effectively guide the green transformation of agriculture and reduce the dependence of crop cultivation on fertilizers and pesticides. This result held even after the elimination of endogeneity and after various tests. Land transfer and environmental supervision are important intermediary mechanisms. Under the influence of these two economic variables, the role of green finance in pollution reduction can be fully brought into play. However, there is regional heterogeneity in the negative effects of green finance on ANSPs. Its effect on pollution reduction is stronger in major grain-producing areas and regions with well-developed digital financial infrastructure.
Discussion: This study confirms once again that green finance has a negative effect on ANSP, and the findings help to decouple the development of the agricultural economy from emissions. The outstanding contribution of this study is the discovery of the role of land transfer and government environmental regulation. I order to give full play to the role of green finance in pollution reduction, this study provides relevant policy measures. These measures are intended to improve the green finance system and business model in agriculture and rural areas.
A good ecological environment is the most universal welfare for people's livelihoods. Green agricultural development is not only an important component of ecological civilization construction, but also an inevitable choice for comprehensively promoting rural revitalization strategy. China is a big agricultural country. The rapid development of agricultural production has played a prominent role in ensuring the supply of agricultural products, providing food and nutrition to prevent hunger, and stabilizing social development (Du and Hu, 2023). By the end of 2023, China's grain output had been stable at more than 1.3 trillion kilograms for nine consecutive years, with a per capita grain share of 493 kg, far higher than the internationally recognized food security line. However, the rapid growth of agricultural economy has produced agricultural environmental problems that cannot be ignored. Under the background of China's extensive agricultural economic model, the problem of non-point source pollution caused by the market players' one-sided pursuit of intensive and large-scale agricultural development with high input and high output is very serious. According to the data of the Second National Pollution Sources Census Bulletin released in 2020, the chemical oxygen demand, total nitrogen, and total phosphorus in the emissions of water pollutants from agricultural sources in China are 10.6713, 1.414900, and 21.2 million tons respectively, accounting for 49.8%, 46.5%, and 67.2% of the national water pollutant emissions, respectively. In 2021, the single acre net use of nitrogen, phosphorus, potassium, and compound fertilizers for China's three major grain crops was 8.39, 0.44, 0.56, and 15.54 kg, respectively. However, the utilization rates of fertilizers and pesticides were only 41.3 and 41.8%, respectively, causing high-intensity and overloaded pollution to soil and water quality. Agricultural non-point source (ANSP) is the main source of regional pollutants (Zhao et al., 2022).
Accelerating the green development of agriculture not only faces the constraints of soil environment, water environment and other resources, but also faces many difficulties such as the lack of green technology, the lack of green production capacity and production power of producers. The vulnerability of sustainable agriculture includes not only the risks brought by factors such as location, soil and climate, but also the conflicts between environmental requirements and production efficiency faced by green agriculture in production links such as irrigation, weeding and fertilization. The development of green agriculture is difficult to become a conscious action of agricultural producers, and it is necessary to correct the market failure caused by positive externalities and vulnerabilities of green agriculture through policy support and economic incentives. In the current context of resource scarcity and severe environmental pollution, green finance (GF) is particularly important. Through instruments like green credit, green bonds, and other financial mechanisms, GF channels funds toward low-carbon, clean energy, and environmental protection projects. This facilitates the transition of capital from high-pollution industries to low-pollution sectors, enhances returns on investments in green industries, improves fund availability, and mitigates pollution emissions (Tian et al., 2022; Ge and Zhu, 2022). The 20th National Congress of the Communist Party of China (CPC) underscored the vital role of financial backing for green development. It explicitly advocated for the rational allocation of resources, promotion of resource transfer to green and low-carbon projects, and facilitation of the transition of traditional industries toward ecological practices and the development of new green industries. In this context, agricultural nonpoint source pollution (ANSP), as a significant driver of systemic environmental pollution, plays a crucial role in achieving sustainable agricultural development and ensuring human health and safety (Fan et al., 2024).
However, due to the characteristics of ANSP, despite China's robust policy and financial support for controlling such pollution, administratively-driven fiscal policies have encountered a “dysfunctional” dilemma, making ANSP control a key and challenging aspect of environmental governance. The dispersion, hidden nature, and delayed characteristics of ANSP exacerbate the situation, leading to a yearly increase in government financial support. Urgent expansion and supplementation of the tools for managing ANSP are necessary. In this regard, GF should serve as a means to manage ANSP effectively and play its crucial role. The inclusion of “green financial standardization construction” as a key project during the “13th Five-Year Plan” period, along with the formulation of guiding opinions by the CPC Central Committee and the State Council to comprehensively strengthen ecological environmental protection and fight pollution, signifies the steady advancement of GF standardization in China. With a positive trend in financial market development, financial support has become a primary driver in promoting the management of ANSP and the development of ecological civilization.
Financial support is the most important policy tool besides government fiscal means. The realization of the coordinated development of finance and green agriculture can not only guarantee the interests of financial institutions, but also maximize the demand for financial products and financial services in the green development of agriculture (Yan et al., 2012). While GF yields positive effects in managing ANSP, it faces numerous bottlenecks and challenges, including the imbalance and inadequacy of China's financial development. Hence, in this context, it is essential to examine the impact of GF on the governance of ANSP in China. Does it effectively support pollution management? What mechanisms does GF employ in managing ANSP? This paper aims to address these questions by exploring scientific issues, developing an assessment of the impact mechanism of GF on managing ANSP, providing decision-making guidance for the government to promote ecological construction, and offering reference significance for other developing countries' pollution management efforts. This research aims to contribute to the improvement of the green financial system and achieve dual benefits in financial and environmental domains.
The research framework of this study is as follows: First section is the introduction. This part mainly states the research background and the importance of green finance for agricultural pollution control. The second section is literature review. This part is mainly to collate the efforts and contributions of the published literature under the topic of this study. On the basis of combing them, the advantages and disadvantages of them are reviewed, and the potential literature contributions of this study are given. The third section is the research hypothesis. In this section, the potential influence of GF on ANSP is analyzed and the corresponding research hypothesis is given. The fourth section is model setting and data source. It mainly introduces the empirical model and variable setting of this study. The fifth and sixth sections are empirical analysis. They correspond to the direct and indirect effects of GF on ANSP. The seventh section is discussion.
The concept of GF, originating from global concerns for environmental protection and sustainable development, represents a novel paradigm in financial theory and practice. It is also referred to as environmental finance or sustainability finance in existing literature. GF primarily restructures the operational concepts, management policies, and business processes of the financial industry through an environmental protection lens, aiming to achieve sustainable development (Zhang et al., 2019). In recent years, the Chinese government has actively promoted the establishment of a green financial system through various policy measures, including the introduction of green credit, green bonds, and support for the development and implementation of green projects (Li et al., 2023a). China's GF policy not only addresses urban environmental issues but also encompasses the agricultural sector, particularly focusing on the challenge of agricultural surface source pollution.
Agricultural surface source pollution refers to the contamination of the ecological environment due to excessive chemical inputs in the planting industry and improper treatment of crop straws and livestock manure in the farming industry. It is driven by factors such as rainfall, topography, and a variety of influencing factors, making its monitoring challenging. With the rapid development of agriculture since the 21st century, China's major lakes and rivers have been increasingly affected by surface pollution, leading to the dangerous problem of eutrophication (Li Q. et al., 2023). Similarly, foreign countries also face agricultural surface source pollution due to extensive chemical fertilizer use and intensified modern agricultural practices (Shortle et al., 2012).
There are two main methods for measuring agricultural surface source pollution. The experimental method involves selecting representative farms to test and measure pollutants discharged using modeling and monitoring methods (Shen et al., 2020). The source strength estimation method, used for macro calculations, estimates pollutant loads per unit area from farmland fertilizers, livestock and poultry farming, farmland solid waste, and rural life (Ding et al., 2023). Zou et al. (2020) used chemical oxygen demand (COD), total nitrogen (TN) and total phosphorus (TP) to measure the pollution load of agricultural non-point sources, and found that agricultural non-point source pollution was closely related to agricultural conditions. Xu et al. (2022) used NH3N as an indicator to characterize agricultural non-point source pollution, and believed that agricultural non-point source pollution had a long-term impact on water quality pollution. Wang et al. (2022) applied a statistical data mining technique boosted regression tree model to quantify the contribution of eight influencing factors (soil type, slope, elevation, RUSLE LS factor, RUSLE K factor, runoff, fertilizer application rate and land use) on two types of CSA (TN-CSA and TP-CSA), as well as the marginal effects and potential thresholds of influencing factors on the occurrence of CSA.
As the financial system continues to evolve, market-led green financial support has become an important tool for managing ANSP. Financial institutions can influence the environmental protection capacity of the production sector by incorporating environmental values into their financial products or services, thereby directing social funds to participate in pollution management, particularly in less developed areas (Jiang et al., 2019). Measures such as promoting organic fertilizers, improving livestock and poultry management, and supporting eco-agriculture not only reduce pollution but also enhance agricultural production efficiency, achieving economic and environmental benefits (Bah et al., 2020; Wen et al., 2024). However, the application of GF in agriculture still faces numerous challenges. Scholtens (2017) suggest that the imbalance in financial resource allocation can hinder efforts to combat ANSP. Factors such as capital scarcity, technological limitations, and the immaturity of financial products and services may inhibit farmers' adoption of ecological practices for soil and water conservation (Spearing et al., 2022; Muthukannan et al., 2020). Lv et al. (2023) showed through empirical research that green finance has a significant inhibitory effect on agricultural non-point source pollution, and green finance policies should be formulated according to local conditions to achieve green agriculture. Geng et al. (2024) conducted a study in Chinese provinces, and the results show that green finance has an inhibitory effect on agricultural non-point source pollution, and environmental regulation and land transfer have a mechanism effect. Cao and Gao (2024) believe that green finance can not only curb non-point source pollution but also reduce carbon emissions.
Overall, research on GF and ANSP appears to be both systematic and comprehensive, yielding significant findings across various dimensions. Nonetheless, as investigations advance, certain limitations in existing studies have become evident. In response, this paper utilizes panel data from 30 mainland Chinese provinces between 2005 and 2021. It constructs a GF index employing the entropy value method and assesses emissions from seven categories of agricultural non-point source pollutants (agricultural fertilizers, livestock and poultry farming, aquaculture, crops, rural life, pesticides, and agricultural films) using the inventory method. Moreover, the paper performs empirical analyses of the pollution control and emission reduction impacts of GF by developing a mediation effect model. The research contribution of this article mainly includes three aspects.
Firstly, innovatively identify the causal relationship between GF and ANSP. Previous studies have mainly focused on the relationship between single GF and ANSP and other influencing factors. At the same time, this article also delves into the role of government environmental regulation and the role of land transfer in this causal relationship. This article not only reveals the interactions between these elements, but also provides empirical evidence for optimizing the development of the green finance system, which will help strengthen the control of agricultural non-point source pollution.
Secondly, this study expanded the scope of ANSP measurement based on the inventory method. Existing research on the use of inventory methods mostly only selects limited indicators, such as the amount of fertilizer and pesticide usage, for calculation, and the selection of indicators is not comprehensive enough. This article selects more than 10 indicators from five aspects, including fertilizers, pesticides, and crops, for calculation. The calculation results are more accurate and can provide a more comprehensive evaluation of the relationship between GF and ANSP.
Finally, the study examined the heterogeneity of the effect of GF on reducing ANSP. The degree of economic development and geographical location are often used as sample classification criteria for heterogeneity test. However, these two criteria have not been able to add some surprising new findings to the existing research. According to the natural endowments and the degree of fintech development in each region, the study selected major grain-producing areas and digital financial inclusion as the sample classification criteria. According to the results of heterogeneity analysis, we found interesting conclusions regarding different natural and human conditions.
Overall, compared to previous studies, this study provides a more scientific and comprehensive insight into improving the effectiveness of GF in pollution control and emission reduction. Meanwhile, it provides a reference for more effective management and prevention of ANSP.
Blue sky, clear water and green grass is a good ecological environment and a typical public good. Their provision and maintenance are characterized by strong externalities. Marshall's externality theory holds that it is difficult to rely on the market mechanism to satisfy the public goods such as the ecological environment, and the government needs to play the role of product supplier. In order to solve the problem of externality of public environment, economists have put forward different methods, among which the more typical schemes are the theory of property rights and Pigovian tax. The theory of property rights shows that as long as the ownership of goods is clear and there are relevant laws and regulations to achieve effective protection of property rights, in the case of a perfect market, the efficiency loss caused by externalities can be improved by the market itself. Under the guidance of the property rights theory, the rights and interests trading represented by emission rights, carbon emission rights and sulfur dioxide emission rights are derived, and the environmental externalities of enterprise production are transformed into internal costs. In the case of negative environmental externalities, it is necessary for the government to formulate a tax mechanism and take measures to levy pollutant discharge tax on polluters so that the production cost of polluters is equal to the social cost. When there are positive environmental externalities, the government reduces the production cost of enterprises by means of fiscal subsidies or tax incentives, so as to solve the externalities problem to a certain extent. The environmental tax, resource tax and carbon tax already implemented in some countries and regions reflect Pigou's thought of cost internalization. The existing theories reflect that to deal with environmental or climate problems, the cost of pollution control should be internalized into the production cost of enterprises, and the government should adopt the behaviors of regulation, confirmation of rights and supervision (Shen and Zhang, 2022; Ma et al., 2024). The logic of the collective action problem is to provide “selective incentives” to overcome natural obstacles to cooperation, and the task of formulating and implementing measures is necessarily undertaken by the government agency. The same thinking applies to environmental issues, where internalizing externalities requires government intervention. In the dual body composed of the government and individual producers, if the government simply relies on the internalization cost through taxation or defining property rights, it will bring great emission reduction costs to the producers and the government, so it needs the financial support of financial institutions.
GF can make farmers make good environmental and social decisions by internalizing the external costs in the agricultural production process. GF, an emerging financial mechanism aimed at promoting environmentally friendly investments and projects, significantly influences the prevalence of ANSP (Liu and Zhang, 2024). This impact is evidenced in several ways.
Firstly, GF fosters the sustainability of agricultural production by reallocating resources, thereby mitigating the adverse environmental effects of agricultural activities at their source. For instance, green credit, China's primary green financial product, imposes stringent environmental criteria for loan recipients and emphasizes rigorous credit assessment processes, indirectly raising financing costs for enterprises with high pollution and energy consumption levels. The pollution emission behavior of agriculture-related enterprises is regarded as the assessment condition of credit by financial institutions. Through resource reallocation, green credit reduces credit allocation to such enterprises, strictly prohibits support for restricted or newly established projects, increases the risk of exit for these high-polluting enterprises, sends market selection signals, and creates barriers for potential entrants. The credit conditions of green finance can help financial institutions screen out qualified investors with sufficient funds and focus on long-term returns, avoid speculation that only focuses on short-term interests, and then ensure a steady flow of capital into the green agricultural industry to provide necessary funds for the green development of agriculture.
Secondly, GF can also share risks for the development of green agriculture, thereby improving the enthusiasm of agricultural operators. Green financial products include green credit, green insurance, green bonds, green futures, etc. Different green financial products play a different degree of risk sharing role in the development of green agriculture. In the process of agricultural production, promoting farmers to buy green insurance can effectively reduce risks. The transition from traditional agriculture to green agriculture is faced with great risks. The participation of GF can effectively share some risks, help alleviate the resistance of agricultural operators to the green transformation of traditional agriculture, guide farmers to actively participate in the green transformation process, and promote the development of green agriculture.
Finally, GF provides financial support for the research and development of cutting-edge agricultural technologies and the promotion of advanced agricultural equipment, which helps to promote the development of green agriculture. GF facilitates the management of ANSP through technological advancements. It promotes the adoption of eco-friendly agricultural technologies such as soil testing, precision fertilization, commercial organic fertilizers, and integrated water and fertilizer management. By supporting innovative research and development initiatives of enterprises, GF elevates the technological prowess of the agricultural sector. A typical example is that newly developed agricultural machinery and equipment as a new thing, due to its own uncertainty, easy to produce a greater risk to green agricultural production, it is difficult to obtain the trust and widespread use of new agricultural operators, limiting the promotion of agricultural machinery and equipment. GF promotes the promotion of new agricultural machinery and equipment by subsidizing the purchase of green agricultural machinery to reduce the purchase cost of new agricultural operators and extending the warranty period of new green agricultural machinery and equipment, which is conducive to the intensification and cleanliness of agricultural production. Therefore, the funds provided by GF can effectively alleviate the pressure on the development of green agriculture, promote the expansion of green production scale in agriculture, realize the acceleration of technology research and development and promotion, and promote the green process of agricultural development. This shift enhances the efficiency and quality of agricultural development, promotes sustainability, and reduces the burden of ANSP.
Based on the above analysis of the relationship between GF and green agricultural development, this study proposes the first research hypothesis:
Hypothesis 1 (H1): GF helps to curb ANSP.
Wang M. et al. (2023) believe that the Current agricultural non-point source pollution is serious, and it is necessary to reduce agricultural non-point source pollution through environmental regulation, technological upgrading and resource utilization. Environmental regulation (ER) is an important means to prevent and control agricultural non-point source pollution, and verified through empirical study that digital environmental regulation is an important factor to curb ANSP (Zhang et al., 2023). Existing research indicates that intensifying government ER may initially reduce resource utilization efficiency (Boyd and McClelland, 1999), yet it is crucial to recognize that such regulation is instrumental in enhancing the effectiveness of environmental pollution control (Bu et al., 2022). This is evident in two key aspects:
First, ER creates cost-based comparative advantages across various agricultural sectors, thereby facilitating control and reduction of non-point source pollution. Specifically, sectors with high pollution levels incur greater “environmental taxes,” leading to increased production costs. These sectors often lack the research and development capacity to innovate technologically in the short term, thus eroding their comparative advantage. Conversely, green agriculture, with its inherent green competitive advantages, can mitigate environmental costs through optimized resource allocation and accelerated technological progress (Czyżewski et al., 2020).
Second, ER spurs technological innovation and fosters the green transformation of the agricultural industry. As a result, agricultural enterprises enhance the quality of factor inputs, simultaneously phasing out obsolete production capacities and fostering emerging technological leaders. This process facilitates the diffusion of new knowledge, industries, and technologies, thereby advancing the green development of regional agriculture.
Based on these considerations, this paper proposes the following hypothesis:
Hypothesis 2 (H2): GF inhibits the development of ANSP through ER.
The decentralization of agricultural land management and the reasonable proportion of factors have always been regarded as the main obstacle to agricultural progress. As a key measure to alleviate the problems of agriculture and rural development, land transfer policy has become an important management means to optimize the allocation of rural land resources and improve the efficiency of agricultural production (Feng et al., 2022). Compared with household contract management, land circulation can realize the intensive use of land and the optimal allocation of agricultural capital, labor force, and technology. To a certain extent, the circulation of agricultural land in the market is conducive to integrating the three rural industries: energy conservation and emission reduction and high-quality development (Cao and Jin, 2024). It is foreseeable that the expansion of land circulation area will help overcome the practical challenges of land fragmentation, high cost depletion and low economic benefits faced by China's agricultural production field for a long time (Wang and Wang, 2023). Under the influence of land market operation, agriculture will tend to large-scale and intensive development of production mode (Chen and Wang, 2022). The use of pesticides, fertilizers, agricultural film and diesel will be gradually reduced in large-scale operations and replaced by cleaner production.
GF has the potential to curtail the advancement of ANSP through land transfer, and its transmission mechanism can be delineated through the following three dimensions:
(1) Optimization of land resource allocation. GF, bolstered by financial backing, encourages agricultural stakeholders to embrace more efficient farming techniques. Consequently, this reduces the demand for land and other ecosystem resources, leading to diminished usage of ineffective inputs like chemical fertilizers and pesticides. This optimization effectively mitigates the negative externalities associated with agricultural production, fostering pollution control and emission reduction endeavors.
(2) Economic incentives for land transfer. GF incentivizes land transfer by providing economic inducements to agricultural producers who adopt sustainable agricultural practices. Through lowered financing costs and more lenient loan conditions, it facilitates financial support for agricultural producers, thereby facilitating the transition toward environmentally friendly agricultural production (Zang et al., 2022).
(3) Investment in agri-environmental technologies. Green financial support encompasses investment in environmentally friendly agricultural technologies. This implies that agricultural producers can secure funds to procure environmentally friendly agricultural tools such as conservation irrigation systems and eco-fertilizers. The application of these technologies aids in alleviating ecological pressures on land.
Therefore, based on these observations, this paper posits the following hypothesis:
Hypothesis (H3): GF inhibits the development of ANSP through the degree of land transfer.
The core purpose of this section is to test whether the effect of GF on ANSP is as expected. Based on the published literature (Jochmans and Verardi, 2020; Li et al., 2022; Bai et al., 2023; Hu, 2023), the study constructs the following panel data model:
In Equation 1, Controls is the set of control variables; μt is time fixed effects; εit is the random error term; νi is the individual fixed effect; the subscripts i and t represent provincial individuals and times, respectively; a0 is constant term; α1 is the regression coefficient of green finance; α2 is the regression coefficient of the control variable. Equation 1 is a classical fixed effects model, which controls both individual effects and time effects. It has a good advantage in solving the endogenous problems caused by unobservable factors.
Building upon prior analysis, it is posited that GF can influence ANSP both directly and indirectly, through the promotion of ER and LT. In order to verify the role of mediating variables and investigate hypotheses 2 and 3, this study constructs a mediating effect equation based on existing research (Shen and Zhang, 2024a; Liu and Mao, 2019). The specific formulation of the model is presented as follows:
In Equation 2, MV is the intermediate variable, b1 is the regression coefficient of GF, The meanings of the remaining symbols are consistent with Equation 1. The published literature suggests that the two-stage intermediation effect equation has a good advantage in eliminating the endogeneity of economic systems (Jiang, 2022; Giuli and Laux, 2022). Compared with the stepwise regression method used in the three-stage mediating effect model, it focuses on whether the influence of the core explanatory variable on the mediating variable meets the expectation. There are two steps in this method: (1) In the research hypothesis part, the influence of intermediate variables on ANSP is analyzed theoretically. (2) In the empirical part, focus on whether the influence of GF on intermediary variables is in line with expectations, and use finance, environmental economics or management theories to explain why the influence of GF on intermediary variables is valid.
Agricultural production generates various forms of surface source pollution, predominantly consisting of total nitrogen (TN), total phosphorus (TP), chemical oxygen demand (COD), carbon emissions, and residues from pesticides and agricultural films. This pollution impacts the soil environment directly and also reaches water bodies through a combination of precipitation, topography-driven runoff (both surface and subsurface), and plant interception (Sun et al., 2012; Luo et al., 2023). To measure agricultural pollution, this study employs the inventory analysis method based on unit surveys. This method encompasses pollution from both aquaculture and the use of pesticides and agricultural films. It estimates ANSP across seven dimensions: agricultural fertilizers, livestock and poultry farming, aquaculture, crops, rural population, pesticides, and agricultural plastic films. The indicators used for this analysis are detailed in Table 1.
Because agricultural non-point source pollution includes three different sources of pollution, the study calculated its total emissions. The detailed calculation process are as follows:
In Equation 3, the TE is the total emission of ANSP; ETP, ETN, ECOD are the total emission of total nitrogen (TN), total phosphorus (TP) and chemical oxygen demand (COD), respectively. In Equation 4, the EUi is the statistic of the pollution unit ; ρi is the pollution production coefficient of the pollution unit; and θi is the emission coefficient or loss rate. The pollution intensity of various units differs due to distinct influencing factors. For the calculation of emissions from ANSP, the coefficients for the seven pollution units are utilized as follows.
The primary pollutants from agricultural fertilizers include nitrogen, phosphorus, and compound fertilizers. This paper employs the output coefficient method to account for the variances in fertilizer loss rates due to different planting methods. Since the focus is on TN and TP pollution from fertilizer inputs, and the phosphorus fertilizer inputs in statistical yearbooks are indicated as phosphorus pentoxide (P2O5), these inputs are adjusted by multiplying them by 43.66%. Additionally, in line with recent domestic fertilizer practices and prior research findings, the compound fertilizer is converted to TN at 40% and P2O5 at 32%. The fertilizer loss coefficient is derived by averaging the results from different regional samples, based on existing studies (Wang et al., 2019). This specific accounting approach is in accordance with the methods used in the second national pollution source census.
The pollution emissions are calculated as the product of the total quantity of livestock and poultry (either in stock or slaughtered), multiplied by both the pollution discharge coefficient and the wastage coefficient. The discharge coefficients for feces and urine of livestock and poultry are sourced from SEPA data (2022). The formula applied is: livestock and poultry pollution intensity (kg per head per annum) = rearing cycle × fecal (urine) emission factor × fecal (urine) pollutant excretion coefficient. In this study, livestock and poultry statistics encompass cattle, sheep, and pigs. For cattle and sheep, which have a rearing period of more than one year, the total breeding amount is based on the year-end stock. For pigs, due to their rearing period of < 1 year, the total breeding amount is determined by the current year's output.
ANSP primarily arises from bait residues, aquaculture excreta, and chemicals. The extent of this pollution is contingent on the aquaculture type and method. The China Statistical Yearbook classifies aquaculture production into marine and freshwater categories. Given that artificial aquaculture is a significant pollution contributor, this paper exclusively utilizes data from freshwater aquaculture for its analyses. The primary aquaculture species include freshwater fish, crustaceans, shellfish, and other aquatic organisms. The production and discharge coefficients for aquaculture are derived from the First National Pollution Source Census: Handbook of Production and Discharge Coefficients for Pollution Sources in Aquaculture, supplemented by additional literature (Feng et al., 2023).
The primary pollutants from crops include residues, vegetable wastes, and other debris from agricultural production (Norse, 2005). Given the diverse range of crops, this paper focuses on the seven most representative ones for analysis: rice, wheat, maize, beans, potatoes, oilseeds, and vegetables. The estimation of surface source pollution from agricultural solid waste involves calculating the crop residue yield based on the grass to grain ratio and determining the total nitrogen (TN), total phosphorus (TP), and chemical oxygen demand (COD) content from the nutrient composition of the straw. Recognizing the varied straw utilization methods in rural areas, each with different nutrient loss rates, the final emission formula for farmland solid waste pollution is: emissions (tons) = total crop production (tons) × production coefficient × straw utilization structure × straw nutrient loss rate, where the production coefficient equals the grass to grain ratio multiplied by the straw nutrient content (Ma et al., 2019).
Pollution in rural life primarily comprises domestic sewage and human feces. The annual production coefficients per capita for COD, TN, and TP in domestic wastewater are 5.84, 0.584, and 0.146 kg/person, respectively, with an emission factor of 100%. For human feces, the corresponding coefficients are 19.8, 3.06, and 0.64 kg/person, respectively, with an emission factor of 10% (Luo et al., 2019).
Pesticide residues are calculated as the amount of pesticides applied multiplied by a residue factor of 0.5.
The amount of agricultural film residue is determined by multiplying the quantity of agricultural film used by a residue factor of 0.1.
GF primarily aims to adhere to market economy principles while focusing on building an ecological civilization. It employs a range of financial tools, including credit, securities, insurance, and funds, to foster energy conservation, reduce consumption, and achieve a harmonious balance between economic resources and the environment. In 2007, the State Environmental Protection Administration and the People's Bank of China jointly issued the Opinions on Implementing Environmental Protection Policies and Regulations to Prevent Credit Risks, which determined the basic standards and industry norms of green finance in China. Under the guidance of the program, China's green finance has developed rapidly. The financial system has also developed into a form based on green credit, bonds, funds, and insurance. When analyzing the economic effects of green finance, the published literature mainly used two methods: policy evaluation (Daniya and Tang, 2024; Luo et al., 2024; Xie et al., 2024; Chen et al., 2025), and comprehensive index (Ren et al., 2020; Lee et al., 2024; Geng et al., 2024). In 2017, the Chinese government launched the green finance reform Innovation Pilot Zone policy in a small number of cities. Under the guidance of policies, different cities shoulder different financial tasks. However, the pilot cities for the measure are mainly prefecture-level cities and municipalities, while the statistical sample for this study is at the provincial level. The availability of data makes the comprehensive index method more suitable for this study. According to the published literature (Li et al., 2022; Jiang and Wang, 2024; Lu et al., 2024; Xu et al., 2024; Zhang W. et al., 2024), this paper developed indicators in seven domains: green credit, green investment, green insurance, green bonds, green support, green fund, and green rights and interests. These indicators are then integrated using the entropy method to formulate a GF index, which assesses the level of GF development. For this assessment, raw data is initially standardized, followed by the computation of the indicators. The detailed measurement methodology is presented in Table 2. In synthesizing the composite index of green finance, this study mainly uses the entropy method. The method relies on information entropy, which is dependent on data feedback, when setting the weights of each indicator, and is not affected by the subjective preferences of researchers. The weight information obtained by entropy method has excellent objective characteristics.
The selection of the ER variable follows the methodology of Chen et al. (2018). This approach utilizes the frequency of terms related to “environmental protection” in local government work reports compared to the total word count of the report as an indicator. A higher frequency indicates a stronger commitment to environmental governance, thus reflecting the intensity of ER and addressing endogeneity concerns. Relevant terms include ecology, green, low-carbon, pollution, energy consumption, emission reduction, sewage, sulfur dioxide, and carbon dioxide. As local government work reports are typically published early in the year, they predate and thus are not influenced by that year's environmental conditions, further mitigating endogeneity issues.
LT refers to the process in which rural households transfer, lease, or collaborate on contracted farmland within a certain period of time. According to the published literature (Kuang et al., 2021; Zhang G. et al., 2024; Wu and Zhang, 2024), this study determined the specific measurement index of LT as the ratio of the area of household contracted cultivated land transfer to the total area of household contracted cultivated land. The LT rate should consider the area of land transferred in and out (Xiong et al., 2024). However, for a particular area, the land transferred by some farmers is the land transferred by another farmer; that is, the transferred area and the transferred area are equal in quantity. With the development of various new agricultural management subjects, farmers are the only subject of land transfer, but not the only subject of land transfer. The different inflow subjects make it difficult for the land transfer area to reflect the land circulation situation in the market fully. Therefore, this study mainly calculates the land transfer rate from the perspective of land transfer. By observing the value of the land circulation rate, we can judge whether the land resources have been optimized and avoid the practical problem of idle land and abandoned land (Shi, 2024).
Since the control of agricultural non-point source pollution depends on many factors, it is naturally also affected by other economic activities. To avoid the bias of missing important variables, the study selected six control variables based on the published literature (Zhou et al., 2022; Shen et al., 2023a; Xu et al., 2023; Ma, 2024; Wei et al., 2024; Zhu et al., 2024; Abid et al., 2022). The industrial structure (IS) is measured using the share of the value-added of the tertiary industry in the gross domestic product (GDP). Urbanization (UR) is measured by the number of people with urban household registration as a share of the total population. The government macro-control (GMC) is measured by the proportion of general budget expenditure to GDP. Technological innovation (TI) is measured by the number of applications for invention patents. Traditional financial supply (TFS) is measured using the balance of loans per capita. Road accessibility (RA) is measured using highway miles. The degree of agricultural mechanization (DAM) is measured by the proportion of the total power of agricultural machinery to the sown area of crops.
Following the principles of data availability and continuity, the study used panel data for 30 provinces in China from 2005 to 2021 as a statistical sample. Due to the poor availability of data and differences in statistical caliber, the statistical sample does not contain observations from Xizang, Macao, Hong Kong and Taiwan. The data used in the research are mainly from China Statistical Yearbook, China Rural Statistical Yearbook, China Rural Operation and Management Statistical Annual Report and Express Professional Superior (EPS) database. The data of green finance related indicators come from China Environmental Yearbook, Chinese Research Data Services Platform (CNRDS) and China Financial Yearbook. To address any minor data gaps, linear interpolation was employed, and logarithmic transformations were applied to all variables to mitigate heteroskedasticity bias arising from data extremes. Descriptive statistical analysis of all variables is shown in Table 3.
In order to clarify the spatio-temporal evolution of ANSP in China, GIS projection technology is used to analyze it. In this section, the author used the Dysprosium Chart online website to complete the drawing work. The map of the map is provided by Amap, and its review number is GS (2019) −756. The author only colored the map. In the process of mapping, in order to clearly compare the marginalization state of ANSP in different years, we use the data of 2005 as the base, and divide 30 provinces into five categories by natural breakpoint method.
As can be seen from Figure 1A, in 2005, ANSP of most provinces in China was in the first two categories (< 0.17 and 0.17–0.31). There are also a significant number of provinces in the third category (0.31–0.45), and only a few provinces have ANSP values >0.45. This result means that in 2005, China's agro-ecological environment was in a good state, and the related pollution emission was at a low value. The reason for the low emission of agricultural pollution at this stage may be that the agricultural production area is small, and the use of agricultural fertilizers, pesticides and agricultural films is small. Although the Chinese government implemented the agricultural tax reduction policy for some provinces in 2005, the number of regions and farmers covered by the policy is still relatively small, so the enthusiasm of agricultural production is very low. As can be seen from Figure 1B, most provinces are in the second (0.17–0.31), third (0.31–0.45), and fourth (0.45–0.59) categories, while the number of provinces in the first category is significantly reduced. The result means that there is serious environmental pollution in agricultural production. A large amount of COD, TP and TN is discharged from farming and aquaculture, and the water quality of rivers will be seriously affected. In 2006, the Chinese government abolished all agricultural taxes, and farmers no longer pay any taxes to the government. The implementation of this policy has stimulated the enthusiasm of agricultural production. A large amount of wasteland has been reused, and aquaculture and animal husbandry have been well developed. However, the rapid growth of agricultural economy is accompanied by serious environmental pollution. Influenced by natural conditions, technical conditions and traditional habits, China's agricultural production continues to develop in an extensive mode. Its main feature is the extensive use of production factors to increase crop yields. During this period, fertilizer and agriculture were used extensively, and the number of poultry farms also expanded dramatically. As can be seen from Figure 1C, the number of provinces in the first to third categories has increased significantly, with very few provinces having ANSP results >0.45. This result is similar to that of 2005, which means that the rural ecological environment has been significantly improved, and the emission of COD, TP and TN has been reduced. The improvement of ecological environment is closely related to the support of green finance and the guidance of the government. From 2013 to 2021, the Chinese government has fully implemented the zero-growth strategy of fertilizer agriculture in an attempt to reduce potential environmental threats by improving crop seeds and agricultural production techniques. At the same time, the central bank has set preferential policies for financial institutions to issue green credit to rural areas to support the green development of agriculture. It is worth noting that the Figure 1D indicates that China's ANSP is very serious. Compared with 2005, in the following 10 years, pollution emissions from agricultural production continued to increase, causing a serious burden on the ecological environment. In the coming period of time, agricultural growth should focus on ecological and environmental protection, so that green GDP becomes the mainstream.
Figure 1. Space-time evolution of ANSP from 2005 to 2021. (A) ANSP data in 2005. (B) ANSP data in 2013. (C) ANSP data in 2021. (D) Average ANSP for 2005–2021.
Combined with the four figures in Figure 1, it can be found that the provinces with high ANSP values are mainly the central region and Shandong, Jilin, Guangdong, and Sichuan provinces. These places are the main producing areas of China's agricultural products, and agriculture occupies a very important position in the national economy. Henan, Sichuan, and Hubei, for example, are major grain-producing regions with very large agricultural areas. Agricultural pollution is the worst in China, followed by Sichuan, Hebei, and Jilin provinces, and then Shanxi, Shaanxi, and Qinghai provinces. The less polluted provinces differ from other regions in terms of industrial structure and ecological carrying capacity. For example, Shanxi Province is rich in coal resources, its industrial structure is mainly dominated by heavy industry, its economic development depends on resource exploitation, and the proportion of agriculture in the national economy is very low. Because of the fragile ecological environment and high altitude, Qinghai Province is not suitable for the development of traditional planting industry.
As can be seen from the results in Table 4, F statistic is significant at 1% level. The results show that the fixed effect (FE) model is superior to the mixed least square method. According to the results of Hausman test, it can be found that the results of both equations are significant at the 1% level. The result shows that the FE is better than the random effect model. For the multiple regression analysis in this study, the two-way fixed effect (TWFE) model is employed, and the benchmark regression outcomes are presented in Table 4. Analysis of column (1) of Table 4 reveals that the coefficient estimate of GF on ANSP is significantly negative at the 1% significance level when no control variables are incorporated. The results in the second column show that the regression coefficient of GF is −1.059 and significant at 1% level after the inclusion of the control variables. The results of both equations show that GF has a negative effect on ANSP. Therefore, research hypothesis 1 is verified with the support of TWFE. The reasons are as follows: On the one hand, the promotion of green finance can not only promote the improvement of the economic level, but also promote the high-quality development of the economy. Due to its green attribute, the rapid development of the economy can also reduce the generation of pollution (Jiang et al., 2020). On the other hand, the emergence of green finance has promoted the rise in the level of agricultural green technology, prevented pollution in the process of agricultural development from the root, and technologically inhibited the production of agricultural non-point source pollution (Lin et al., 2022).
The results of baseline regression confirm that GF contributes to the reduction of ANSP. To verify the robustness of the results, three methods were used in the study.
Solve the endogenous problem of economic model. The above empirical tests have proved that green finance has a significant inhibitory effect on agricultural non-point source pollution. In order to solve the possible endogenous problems in the model, this paper adopts the method of instrumental variables for regression again, and selects the first-order lag term of green finance and the first-order difference term of green finance as the instrumental variables in this paper (Baiocchi et al., 2014; Abadie et al., 2024). As can be seen from Table 5, the F statistic of the weak instrumental variable test is 19.222, and the results of the unidentifiable test reject the null hypothesis at the significance level of 1%. The results of these two tests show that the instrumental variable constructed in this study are valid. According to the results of Method 1 in Table 5, the regression coefficient of green finance is −1.881 and is significant at the 5% level. This result is consistent with the result of baseline regression, which confirms its robustness.
The econometric model was replaced. Generally speaking, the error term of the panel data model consists of two parts: one is the individual effect that changes with the individual but does not change with time; the second is the time effect which varies with time but not with individual; The third is the unobservable factors that vary with cross-section and time. Introducing individual effect and time effect into panel data model, and using de-mean method to difference out unobstructed factors can reduce the endogeneity problem caused by the correlation between missing variables and explanatory variables. In traditional panel fixed effects, both individual effects and time effects are added to the model. However, in the real economic world, the impact of time dimension may be multidimensional, and the impact of the same kind of impact on different individuals may vary. The traditional fixed-effect model struggles to address the endogenous issues arising from unobservable factors that vary both over time and across individuals. The interaction fixed effect (IFE) model introduces the interaction term of individual and time into the panel data model to reflect the heterogeneity of the influence of common factors on individuals (Bai, 2009). IFE fully considers the multiple shocks of real economic activities and the heterogeneity of the intensity of response of different individuals to the same type of shocks, which can better reflect the economic behavior in the real world (Hagedorn et al., 2015; Yang and Shen, 2023; Shen et al., 2023b). Extending Equation 1 to IFE yields the following equation:
In Equation 5, is interactive fixed effect. It reflects the product of multi-dimensional individual effect and multi-dimensional time effect. Ft is the common factor, λi is the factor load. It can be seen from the results in Table 5 that the regression coefficient of GF is −0.551 and is significant at 1% level. This result still supports the conclusion that GF will reduce ANSP.
The measure method and evaluation system of explained variables are replaced. Although the mechanism of agricultural nonpoint source pollution is complex and uncertain, as long as agricultural supplies such as fertilizers and pesticides are invested in the planting process, it will have a negative impact on water resources and cultivated land resources through rainfall, irrigation and flowing water. As long as there are inputs to agricultural production and agricultural materials, ANSP is inevitable. The study used the United Nations Human Development Index (HDI) methodology to re-measure the ANSP based on published literature (Jiang et al., 2021; Wang B. et al., 2023; Li and Wang, 2024; Liu and Xiao, 2024). The point of this method is to make the weights of each indicator equal. Chemical fertilizers (CF), pesticides (PE), agricultural films (AF) and diesel oil (DO) are often used in agricultural production factors, which inevitably have a negative impact on the ecological environment. Because of the differences in agricultural production conditions and management modes in different provinces, it is not suitable to simply use the average input amount for comparative analysis, so the concept of agricultural non-point source pollution intensity is introduced in this study. The detailed calculation process is as follows:
In equation, SA is the sown area of crops. According to the results in the third column of Table 5, after replacing the explained variables, the regression coefficient of GF is −0.068 and is significant at the 10% level. Compared with the results of the baseline regression model, the significance of GF in Method 3 has decreased, but it is still statistically significant. A significant change from 1% to 10% is not sufficient to disprove the results of the baseline regression. Therefore, the role of GF in reducing ANSP is still proven.
In the mediation effect analysis, ER and LT were selected as the mediating variables of GF and ANSP (Hsiao et al., 2021). The mediating effects of ER and LT are shown in Table 6. Column (1) shows the regression result of GF to ER, and its coefficient is 0.157, which is significant at the significance level of 5%, indicating that ER level increases significantly when GF increases. This suggests that ER is a partial mediator, which validates the hypothesis H2. This indicates that the adoption of environmental regulations will improve the situation of ANSP, and it can be proved that the strengthening of environmental regulations while the development of green finance will improve the ANSP to some extent.
Column (2) in Table 6 is the result of land circulation effect. Model (5) is the regression result of LT to ANSP, and its coefficient is 1.536, which is significant at the 1% significance level, indicating that the degree of land circulation increases with the increase of the degree of land circulation, which proves that LT is also a partial intermediary, and the development of GF can indirectly and positively affect ANSP by increasing the degree of land circulation, thus verifying hypothesis H3. This shows that land transfer can improve land use efficiency, so it is necessary to consider land transfer in the process of green finance development to reduce ANSP.
In this paper, major grain-producing areas and the maturity of digital inclusive finance are included in the regression. In the heterogeneity analysis part of digital financial inclusion, limited to the availability of data, this study used the mean of all provincial samples from 2011 to 2021 as the criterion for sample division. First, the impact of green finance located in major grain-producing areas on ANSP is analyzed, and the results are shown in Table 7. The results show that for the provinces in the main grain-producing areas, the effect of green finance on agricultural non-point source pollution is much greater than that in the provinces not in the main grain-producing areas. This shows that provinces in major grain-producing areas pay more attention to the development of green finance and better improve the generation of agricultural non-point source pollution. Secondly, the impact of green finance on agricultural non-point source pollution in different provinces with different maturity of digital inclusive finance is analyzed. The results show that in provinces with higher maturity of digital inclusive finance, green finance has more obvious inhibition on agricultural non-point source pollution, while provinces with lower maturity have less inhibition. This shows that having a higher maturity of digital inclusive finance can better promote the development of green finance, and then better curb the degree of agricultural non-point source pollution and ensure the sustainable development of agriculture (Wang M. et al., 2023).
This paper examines the significant impact of GF on reducing industrial pollution and explores its direct effects and mechanisms on ANSP at a theoretical level. Utilizing panel data from 30 Chinese provinces from 2005 to 2021, the study applies panel fixed effect and intermediary effect models to assess the impact and influence mechanisms of GF on industrial pollution emissions from a multi-dimensional perspective. The study concluded as follows.
The ANSP produced by agricultural production in China showed an inverted U-shaped trend of first increasing and then decreasing. The ANSP in 2005 was significantly lower than in other years. The ANSP is very high in the central region and some provinces with large agricultural areas. GF is essentially a special financial service to support sustainable development. It mainly guides enterprises and farmers to invest in environmental protection by increasing investment support for corporate green projects. In addition, it will support enterprises and farmers to actively participate in cleaner production projects by developing diversified financial instruments such as green credit, green bonds and green insurance, thereby reducing agricultural pollutant emissions (Mo et al., 2023; Wang and Zhang, 2023). Although theoretically financial services can promote the green development of agriculture, empirical studies do not fully support this conclusion. On the contrary, some empirical studies show that the impact of financial services on the green development of agriculture is not significant, and even has a negative correlation (Chu and Zou, 2023; Shen and Zhang, 2024b). The results of this study confirm that the development and promotion of GF is beneficial to the control of ANSP. Even after considering factors such as economic development and industrial structure, the negative effect of GF on ANSP is still significant. This is somewhat similar to the findings of a previous study which found that green finance can reduce pollution (Cai et al., 2023). After the results of baseline regression were tested using three methods: eliminating endogeneity, replacing econometric model and replacing explained variables, the conclusion that GF would reduce ANSP remained valid.
ER and LT, as mediating variables, partially mediated the effect of GF on ANSP. GF indirectly affects ANSP by reducing ER and increasing land circulation degree. This indicates that ER and LT play a certain mediating role in the influence of GF on ANSP, and ANSP can be further reduced by increasing ER and LT, which is consistent with the study of Li et al. (2021).
When analyzing the role of green finance in the prevention and control of gold exchange pollution, the existing studies are mainly based on the geographical location and economic development level of the subject (Li et al., 2023b; Wang and Du, 2023; Cao and Gao, 2024). However, these heterogeneity tests have not created new literature contributions. Therefore, whether the subject is located in the main grain-producing areas and the degree of development of digital financial inclusion are identified by this study and applied to the heterogeneity test. Heterogeneity analysis results show that in major grain-producing regions and regions with higher maturity of digital inclusive finance, GF has a more significant negative impact on ANSP, indicating that the higher GF development in major grain-producing provinces and provinces with higher maturity of digital inclusive finance, the more ANSP can be reduced, which is also consistent with published literature (Fang et al., 2023).
The support of financial resources is an important force to protect the environment. The results of this study confirm that green finance has good characteristics in the process of reducing agricultural non-point source pollution. The study provides some policy recommendations to improve the precision of agricultural pollution prevention and control.
Government departments and financial institutions should attach great importance to the infrastructure construction of green finance, focusing on improving the rural financial network and basic system. To maximize the impact of GF on addressing ANSP, it is imperative to clearly define the strategic direction and functional roles of financial institutions in supporting rural ecological civilization. Government departments can collaborate with banks and other departments to strengthen the green credit provided by small and medium-sized banks, expand the scope of fiscal subsidies, and increase investment incentives for green credit, especially in the fields of taxation and technological innovation, by issuing policies such as the “Green Finance Evaluation Plan for Banking and Financial Institutions” and the “Green Credit Guidelines.” These measures aim to effectively curb the financial constraints associated with managing ANSP. Additionally, optimizing the policy framework for the growth of agriculture-related GF is essential. This includes offering preferential treatment to green credit products and prioritizing compensation rights for green bonds and other financial instruments. Furthermore, the development and reinforcement of legal and regulatory measures to address financial, credit, and moral risks in rural enterprises are crucial. These measures are intended to mitigate the risks of sunk and opportunity costs arising from ineffective agricultural green financial instruments.
Coordinate the system design and policy system of GF and ER, and promote the classification standard of GF to meet environmental policy requirements. Creating synergies between GF policies and ER is vital for effectively tackling ANSP. Firstly, governments and regulatory bodies should establish and enforce transparent, equitable ERs, including the implementation of environmental administrative agreements. These agreements should recognize the equal standing of governments and entities responsible for ANSP, ensuring clear communication during negotiations to balance economic and environmental benefits. Secondly, enhancing environmental support through initial lenient ER policies encourages innovation that improves the efficiency of ANSP control. Specific measures include offering subsidies, loans, or other financial aid for technological upgrades and industrial transformation to meet new environmental standards. Lastly, financial institutions must innovate green financial products and services in line with the requirements of ER.
Optimizing land transfer policies. To encourage farmer participation in land transfer and facilitate large-scale agricultural land management, government departments must enhance the rural land transfer system and environment. This includes refining the mechanism to incentivize practices that reduce ANSP, implementing and improving the “three-rights partition” system of agricultural land, and establishing an efficient and reliable platform for agricultural land transfer and trading. A focus should be placed on ensuring an orderly transfer of agricultural land through a standardized process and effective legal frameworks. Additionally, increasing awareness and trust among farmers in land transfer policies through enhanced public outreach is crucial. Further improve the utilization rate of land, improve farmers' attention to environmental protection in land use, achieve the popularity of green finance in agriculture, and then reduce agricultural non-point source pollution.
Choose policies suitable for major grain-producing areas and digital financial inclusion: with better technology in major grain-producing areas, the convenience brought by green finance can be better used. Meanwhile, with the popularization and application of digital inclusive finance, better digital finance can make better use of tax incentives and other policies to encourage rural financial institutions, such as agricultural credit cooperatives, to expand loans for green agricultural production. On the one hand, raising awareness and education about green finance in rural areas can increase farmers' awareness and participation in green finance initiatives and promote the growth and interaction of ecological products and green finance. On the other hand, to improve policy-based financial support for agriculture and improve financial literacy, it is also necessary to establish a comprehensive rural financial supervision system. The system should regulate the financial activities of rural financial institutions through laws and regulations, aiming to shift their investments from the non-agricultural sector to the agricultural sector.
With only 7% of agricultural acreage of world, China has managed to provide food for 22% of world population. This study uses China's provincial panel data to analyze the impact and mechanism of green finance on agricultural non-point source pollution. All the research background, sample data and conclusions obtained depend on the economic and social background of China. As the largest developing country in the world, China's agricultural development has its unique management mode and natural geographical factors. For example, due to the influence of monsoon climate and the limited availability of rugged arable land, China's agricultural production model has only achieved scale in some areas, and there are still a large number of provinces that continue to use intensive farming and sporadic production. Affected by the characteristics of natural geographical environment and high population density, China's agricultural management mode is manifested as small-scale peasant economy. The smallholder economy of agricultural production and the East Asian model make it necessary for the rest of the world to be cautious in drawing conclusions. Governments in countries with similar circumstances should consider the study's findings when formulating agricultural policies.
Due to the availability of data, this paper only selects data at the provincial level in China. Overall, the observations from this study are still inadequate. There are obvious differences in the economic structure and even the climatic conditions of each city within each province. In future studies, the authors strongly recommend using samples from prefecture-level cities, counties, and even agricultural enterprises as observations. A large number of research individuals can enhance the reliability and representativeness of the conclusions. In addition, due to the limitation of data, this study mainly adopts the comprehensive index method when measuring green finance, but these indicators lack pertinency in agricultural pollution prevention and control. The authors also call for using the green finance Reform Innovation Pilot Zone policy to evaluate the implementation of agricultural pollution control policies. This method is not only representative in the measurement of sustainable finance, but also has a good causal inference paradigm in economics. With the help of policy evaluation methods, such as difference-in-differences (DID), synthetic control method (SCM) and regression discontinuity design (RDD), it is helpful to clarify the importance of green finance for ecological environmental protection.
This paper only assesses the situation of green finance and agricultural pollution in China in the past decade or so, and lacks predictive analyses of future changes, so there may be some limitations in recommending future policies based on the results of this paper. Future research can combine machine learning and other methods to predict and analyse the changes of the two.
The conclusions obtained by using statistical inference and causal inference have some defects in guiding practical practice. It is clear that the policy recommendations provided by the authors do not have clear operational steps for farmers and agribusinesses. For example, rural commercial banks in Longyou county, Zhejiang province, launched a “green circular loan” project to promote the recycling of livestock and poultry waste. The green financial support provided by the Agricultural Bank of China Zhuhai Branch in the joint development project of the photovoltaic industry and fishery has supported the improvement of rural land use efficiency and the promotion of renewable energy. The authors recommend the use of case analysis, self-ethnography, and grounded theory to perform representative theoretical analysis. The conclusion based on real case study has significant reference and policy reference significance.
The original contributions presented in the study are included in the article/supplementary material, further inquiries can be directed to the corresponding author.
YS: Methodology, Project administration, Software, Supervision, Writing – original draft. XZ: Conceptualization, Funding acquisition, Project administration, Resources, Validation, Visualization, Writing – review & editing.
The author(s) declare financial support was received for the research, authorship, and/or publication of this article. This work was financially supported by the Natural Science Foundation of Fujian Province (grant number: 2022J01320).
The authors declare that the research was conducted in the absence of any commercial or financial relationships that could be construed as a potential conflict of interest.
All claims expressed in this article are solely those of the authors and do not necessarily represent those of their affiliated organizations, or those of the publisher, the editors and the reviewers. Any product that may be evaluated in this article, or claim that may be made by its manufacturer, is not guaranteed or endorsed by the publisher.
Abadie, A., Gu, J., and Shen, S. (2024). Instrumental variable estimation with first-stage heterogeneity. J. Econom. 240:105425. doi: 10.1016/j.jeconom.2023.02.005
Abid, N., Ceci, F., Ahmad, F., and Aftab, J. (2022). Financial development and green innovation, the ultimate solutions to an environmentally sustainable society: evidence from leading economies. J. Clean. Prod. 369:133223. doi: 10.1016/j.jclepro.2022.133223
Bah, H., Zhou, M., Ren, X., Hu, L., Dong, Z., Zhu, B., et al. (2020). Effects of organic amendment applications on nitrogen and phosphorus losses from sloping cropland in the upper Yangtze River. Agric. Ecosyst. Environ. 302:107086. doi: 10.1016/j.agee.2020.107086
Bai, J. (2009). Panel data models with interactive fixed effects. Econometrica 77, 1229–1279. doi: 10.3982/ECTA6135
Bai, T., Qi, Y., Li, Z., and Xu, D. (2023). Digital economy, industrial transformation and upgrading, and spatial transfer of carbon emissions: the paths for low-carbon transformation of Chinese cities. J. Environ. Manage. 344:118528. doi: 10.1016/j.jenvman.2023.118528
Baiocchi, M., Cheng, J., and Small, D. S. (2014). Instrumental variable methods for causal inference. Stat. Med. 33, 2297–2340. doi: 10.1002/sim.6128
Boyd, G. A., and McClelland, J. D. (1999). The impact of environmental constraints on productivity improvement in integrated paper plants. J. Environ. Econ. Manage. 38, 121–142. doi: 10.1006/jeem.1999.1082
Bu, C., Zhang, K., Shi, D., and Wang, S. (2022). Does environmental information disclosure improve energy efficiency? Energy Policy 164:112919. doi: 10.1016/j.enpol.2022.112919
Cai, X., Chen, G., and Wang, F. (2023). How does green finance policy affect the capacity utilization rate of polluting enterprises? Sustainability 15:16927. doi: 10.3390/su152416927
Cao, L., and Gao, J. (2024). The impact of green finance on agricultural pollution and carbon reduction: the case of China. Sustainability 16:5832. doi: 10.3390/su16145832
Cao, X., and Jin, T. (2024). Land transfer, agricultural scale management and agricultural carbon emissions-Quasi-natural experiments based on land transfer policy. J. Huazhong Agric. Univ. 172, 153–163. doi: 10.13300/j.cnki.hnwkxb.2024.04.014
Chen, R., Zhang, Q., and Wang, J. (2025). Impact assessment of green finance reform on low-carbon energy transition: evidence from China's pilot zones. Environ. Impact Assess. Rev. 110:107654. doi: 10.1016/j.eiar.2024.107654
Chen, Y., and Wang, S. (2022). Effect of land transfer Policy on agricultural high-quality development—empirical analysis based on continuous DID method. Contemp. Econ. Manag. 44, 49–57. doi: 10.13253/j.cnki.ddjjgl.2022.02.006
Chen, Z., Kahn, M. E., Liu, Y., and Wang, Z. (2018). The consequences of spatially differentiated water pollution regulation in China. J. Environ. Econ. Manage. 88, 468–485. doi: 10.1016/j.jeem.2018.01.010
Chu, D., and Zou, Y. (2023). Does financial support promote agricultural green development: a meta - analysis. J. Zhengzhou Univ. 56, 11–19.
Czyżewski, B., Trojanek, R., Dzikuć, M., and Czyżewski, A. (2020). Cost-effectiveness of the common agricultural policy and environmental policy in country districts: spatial spillovers of pollution, bio-uniformity and green schemes in Poland. Sci. Total Environ. 726:138254. doi: 10.1016/j.scitotenv.2020.138254
Daniya, G., and Tang, D. (2024). Green finance and industrial low-carbon transition: a case study on green economy policy in Kazakhstan. Sustainability 16:7731. doi: 10.3390/su16177731
Ding, L., Qi, C. C., and Zhang, W. Q. (2023). Distribution characteristics of non-point source pollution of TP and identification of key source areas in Nanyi Lake (China) Basin: based on InVEST model and source list method. Environ. Sci. Pollut. Res. 30, 117464–117484. doi: 10.1007/s11356-023-30405-y
Du, Z., and Hu, L. (2023). The achievements and interpretations of the high-qualityqgricultural development in China since the 18th national congress of the communist party of China. Chinese Rural Econ. 457, 2–17. doi: 10.20077/j.cnki.11-1262/f.2023.01.001
Fan, H., Huang, Z., Feng, C., Wu, Z., Tian, Y., Ma, F., et al. (2024). Functional keystone taxa promote N and P removal of the constructed wetland to mitigate agricultural nonpoint source pollution. Sci. Total Environ. 912:169155. doi: 10.1016/j.scitotenv.2023.169155
Fang, L., Zhao, B., Li, W., Tao, L., He, L., Zhang, J., et al. (2023). Impact of digital finance on industrial green transformation: evidence from the Yangtze River economic belt. Sustainability 15:12799. doi: 10.3390/su151712799
Feng, L., Chen, Z., and Ma, Y. (2022). Study on the influence of land transfer policy on high-quality agricultural development. Stat. Dec. 38, 76–79. doi: 10.13546/j.cnki.tjyjc.2022.19.015
Feng, Z., Zhang, R., Liu, X., Peng, Q., and Wang, L. (2023). Agricultural nonpoint source pollutant loads into water bodies in a typical basin in the middle reach of the Yangtze River. Ecotoxicol. Environ. Saf. 268:115728. doi: 10.1016/j.ecoenv.2023.115728
Ge, Y., and Zhu, Y. (2022). Boosting green recovery: green credit policy in heavily polluted industries and stock price crash risk. Resour. Policy 79:103058. doi: 10.1016/j.resourpol.2022.103058
Geng, G., Shen, Y., and Dong, C. (2024). The impact of green finance on agricultural non-point source pollution: analysis of the role of environmental eegulation and rural land transfer. Land 13:1516. doi: 10.3390/land13091516
Giuli, A. D., and Laux, P. A. (2022). The effect of media-linked directors on financing and external governance. J. Financ. Econ. 145, 103–131. doi: 10.1016/j.jfineco.2021.07.017
Hagedorn, M., Manovskii, I., and Mitman, K. (2015). The impact of unemployment benefit extensions on employment: the 2014 employment miracle? Cambridge, MA: National Bureau of Economic Research. doi: 10.3386/w20884
Hsiao, Y. Y., Kruger, E. S., Lee Van Horn, M., Tofighi, D., MacKinnon, D. P., Witkiewitz, K., et al. (2021). Latent class mediation: a comparison of six approaches. Multivariate Behav. Res. 56, 543–557. doi: 10.1080/00273171.2020.1771674
Hu, J. (2023). Synergistic effect of pollution reduction and carbon emission mitigation in the digital economy. J. Environ. Manage. 337:117755. doi: 10.1016/j.jenvman.2023.117755
Jiang, S., Qiu, S., Zhou, H., and Chen, M. (2019). Can FinTech development curb agricultural nonpoint source pollution?. Int. J. Environ. Res. Public Health 16:4340. doi: 10.3390/ijerph16224340
Jiang, S., Qiu, S., and Zhou, J. (2020). Re-examination of the relationship between agricultural economic growth and non-point source pollution in China: evidence from the threshold model of financial development. Water 12:2609. doi: 10.3390/w12092609
Jiang, S., and Wang, L. (2024). All paths to the same end?—a comparative study on carbon emissions reduction effects of digital finance and green finance. Front. Environ. Sci. 12:1433044. doi: 10.3389/fenvs.2024.1433044
Jiang, S., Zhou, J., and Qiu, S. (2021). Can appropriate scale operation restrain agricultural non-point source pollution?–empirical study based on dynamic threshold panel model. J. Agrotech. Econ. 320, 33–48. doi: 10.13246/j.cnki.jae.2021.07.003
Jiang, T. (2022). Mediating effects and moderating effects in causal inference. China Ind. Econ. 410, 100–120. doi: 10.19581/j.cnki.ciejournal.2022.05.005
Jochmans, K., and Verardi, V. (2020). Fitting exponential regression models with two-way fixed effects. Stata J. 20, 468–480. doi: 10.1177/1536867X20931006
Kuang, Y., Yang, J., and Abate, M. C. (2021). Farmland transfer and agricultural economic growth nexus in China: agricultural TFP intermediary effect perspective. China Agric. Econ. Rev. 14, 184–201. doi: 10.1108/CAER-05-2020-0076
Lee, C., Li, J., and Wang, F. (2024). The role of green finance in the construction of new energy system: evidence from China. Energy Econ. 139:107878. doi: 10.1016/j.eneco.2024.107878
Li, G., Jia, X., Khan, A. A., Khan, S. U., Ali, M. A. S., Luo, J., et al. (2023a). Does green finance promote agricultural green total factor productivity? Considering green credit, green investment, green securities, and carbon finance in China. Environ. Sci. Pollut. Res. 30, 36663–36679. doi: 10.1007/s11356-022-24857-x
Li, G., Wang, L., Li, Z., and Guo, Z. (2023b). Has pilot zones policy for green finance reform and innovations improved the level of green financial development and environmental quality?. Environ. Sci. Pollut. Res. 30, 68667–68676. doi: 10.1007/s11356-023-27243-3
Li, Q., Ouyang, W., Zhu, J., Lin, C., and He, M. (2023). Discharge dynamics of agricultural diffuse pollution under different rainfall patterns in the middle Yangtze river. J. Environ. Manage. 347:119116. doi: 10.1016/j.jenvman.2023.119116
Li, T., and Wang, X. (2024). Study on the spatial spillover effect of environmental tax on agricultural non-point source pollution. Taxation Res. 473, 128–133. doi: 10.19376/j.cnki.cn11-1011/f.2024.05.020
Li, X., Cheng, B., Zhang, J., Wang, L., and Tian, Y. (2021). Impact mechanisms of environmental rules on haze pollution: an examination with the mediation effect. Environ. Eng. Manag. J. 20, 2077–2086. doi: 10.30638/eemj.2021.192
Li, Z., Bai, T., and Tang, C. (2022). How does the low-carbon city pilot policy affect the synergistic governance efficiency of carbon and smog? Quasi-experimental evidence from China. J. Clean. Prod. 373:133809. doi: 10.1016/j.jclepro.2022.133809
Lin, Q., Cheng, Q., Zhong, J., and Lin, W. (2022). Can digital financial inclusion help reduce agricultural non-point source pollution?—an empirical analysis from China. Front. Environ. Sci. 10:1074992. doi: 10.3389/fenvs.2022.1074992
Liu, M., and Xiao, H. (2024). Effect of agricultural factor marketization on agricultural non-point source pollution: the regulatory role of environmental regulation. Chin. J. Eco-Agric. 32, 518–528. doi: 10.12357/cjea.20230240
Liu, X., and Zhang, Y. (2024). Green finance, environmental technology progress bias and cleaner industrial structure. Environ. Dev. Sustain. 26, 8643–8660. doi: 10.1007/s10668-023-03062-x
Liu, Y., and Mao, J. (2019). How do tax incentives affect investment and productivity? Firm-level evidence from China. Am. Econ. J. Econ. Policy 11, 261–291. doi: 10.1257/pol.20170478
Lu, J., Gulzar, F., and Lai, Y. (2024). Exploring the intersection between sustainable finance and achieving carbon neutrality in the transportation sector. Heliyon 10:e34467. doi: 10.1016/j.heliyon.2024.e34467
Luo, C., Lyu, H., Wen, T., Qiang, W., and Lee, H. F. (2024). Green finance and grey air: assessing the impact of green finance pilot zones on company-level pollution in China. Environ. Res. Lett. 19:094010. doi: 10.1088/1748-9326/ad6466
Luo, M., Liu, X., Legesse, N., Liu, Y., Wu, S., Han, F. X., et al. (2023). Evaluation of agricultural non-point source pollution: a review. Water Air Soil Pollut. 234:657. doi: 10.1007/s11270-023-06686-x
Luo, X., Li, Y., Wu, Q., Wei, Z., Li, Q., Wei, L., et al. (2019). Characteristics of internal ammonium loading from long-term polluted sediments by rural domestic wastewater. Int. J. Environ. Res. Public Health 16:4657. doi: 10.3390/ijerph16234657
Lv, W., Zhang, Z., and Zhang, X. (2023). The role of green finance in reducing agricultural non-point source pollution—an empirical analysis from China. Front. Sustain. Food Syst. 7:1199417. doi: 10.3389/fsufs.2023.1199417
Ma, J. (2024). Will agricultural FDI exacerbate agricultural non-point source pollution?—spatial measurement based on China's provincial panel data. Shandong Soc. Sci. 342, 160–168. doi: 10.14112/j.cnki.37-1053/c.2024.02.021
Ma, J., Ding, Y., Cheng, J. C., Jiang, F., and Wan, Z. (2019). A temporal-spatial interpolation and extrapolation method based on geographic Long Short-Term Memory neural network for PM2.5. J. Clean. Prod. 237:117729. doi: 10.1016/j.jclepro.2019.117729
Ma, W., Cao, L., Li, X., and Zhao, X. (2024). Environmental pollution, green finance, and enterprise growth based on the environmental Kuznets curve perspective. Finance Res. Lett. 64:105440. doi: 10.1016/j.frl.2024.105440
Mo, Y., Sun, D., and Zhang, Y. (2023). Green finance assists agricultural sustainable development: evidence from China. Sustainability 15:2056. doi: 10.3390/su15032056
Muthukannan, P., Tan, B., Gozman, D., and Johnson, L. (2020). The emergence of a fintech ecosystem: a case study of the Vizag Fintech Valley in India. Inf. Manag. 57:103385. doi: 10.1016/j.im.2020.103385
Norse, D. (2005). Non-point pollution from crop production: global, regional and national issues. Pedosphere 15, 499–508. doi: 10.1002/jpln.200420493
Ren, X., Shao, Q., and Zhang, R. (2020). Nexus between green finance, non-fossil energy use, and carbon intensity: empirical evidence from China based on a vector error correction model. J. Clean. Prod. 277:122844. doi: 10.1016/j.jclepro.2020.122844
Scholtens, B. (2017). Why finance should care about ecology. Trends Ecol. Evol. 32, 500–505. doi: 10.1016/j.tree.2017.03.013
Shen, W., Zhang, L., Li, S., Zhuang, Y., Liu, H., Pan, J., et al. (2020). A framework for evaluating county-level non-point source pollution: joint use of monitoring and model assessment. Sci. Total Environ. 722:137956. doi: 10.1016/j.scitotenv.2020.137956
Shen, Y., Guo, X., and Zhang, X. (2023a). Digital financial inclusion, land transfer, and agricultural green total factor productivity. Sustainability 15:6436. doi: 10.3390/su15086436
Shen, Y., Yang, Z., and Zhang, X. (2023b). Impact of digital technology on carbon emissions: evidence from Chinese cities. Front. Ecol. Evol. 11:1166376. doi: 10.3389/fevo.2023.1166376
Shen, Y., and Zhang, X. (2022). Study on the impact of environmental tax on industrial green transformation. Int. J. Environ. Res. Public Health 19:16749. doi: 10.3390/ijerph192416749
Shen, Y., and Zhang, X. (2024a). Blue sky protection campaign: assessing the role of dgital technology in reducing air pollution. Systems 12:55. doi: 10.3390/systems12020055
Shen, Y., and Zhang, X. (2024b). Cleaner production: analysis of the role and path of green finance in controlling agricultural nonpoint source pollution. Economics 18:20220118. doi: 10.1515/econ-2022-0118
Shi, C. (2024). Impact of land transfer on high-quality agricultural development: analysis based on the green TFP perspective. J. Nat. Resour. 39, 1418–1433. doi: 10.31497/zrzyxb.20240610
Shortle, J. S., Ribaudo, M., Horan, R. D., and Blandford, D. (2012). Reforming agricultural nonpoint pollution policy in an increasingly budget-constrained environment. Environ. Sci. Technol. 46, 1316–1325. doi: 10.1021/es2020499
Spearing, L. A., Mehendale, P., Albertson, L., Kaminsky, J. A., and Faust, K. M. (2022). What impacts water services in rural Alaska? Identifying vulnerabilities at the intersection of technical, natural, human, and financial systems. J. Clean. Prod. 379:134596. doi: 10.1016/j.jclepro.2022.134596
Sun, B., Zhang, L., Yang, L., Zhang, F., Norse, D., Zhu, Z., et al. (2012). Agricultural non-point source pollution in China: causes and mitigation measures. Ambio 41, 370–379. doi: 10.1007/s13280-012-0249-6
Tian, C., Li, X., Xiao, L., and Zhu, B. (2022). Exploring the impact of green credit policy on green transformation of heavy polluting industries. J. Clean. Prod. 335:130257. doi: 10.1016/j.jclepro.2021.130257
Wang, B., Wang, L., and Li, X. (2023). Can the policy of building high-standard farmland curb agricultural surface source pollution? Resour. Environ. Yangtze Basin 32, 1736–1747. doi: 10.11870/cjlyzyyhj202308016
Wang, B., and Zhang, K. (2023). Impact of green digital finance on green economic recovery and green agricultural development: implications for green environment. Environ. Sci. Pollut. Res. 30, 107611–107623. doi: 10.1007/s11356-023-29599-y
Wang, C., and Wang, L. (2023). Green credit and industrial green total factor productivity: the impact mechanism and threshold effect tests. J. Environ. Manage. 331:117266. doi: 10.1016/j.jenvman.2023.117266
Wang, F., and Du, L. (2023). Does agricultural credit input promote agricultural green total factor productivity? Evidence from spatial panel data of 30 provinces in China. Int. J. Environ. Res. Public Health 20:529. doi: 10.3390/ijerph20010529
Wang, H., He, P., Shen, C., and Wu, Z. (2019). Effect of irrigation amount and fertilization on agriculture non-point source pollution in the paddy field. Environ. Sci. Pollut. Res. 26, 10363–10373. doi: 10.1007/s11356-019-04375-z
Wang, M., Jiang, T., Mao, Y., Wang, F., Yu, J., Zhu, C., et al. (2023). Current situation of agricultural non-point source pollution and its control. Water Air Soil Pollut. 234:471. doi: 10.1007/s11270-023-06462-x
Wang, S., Wang, Y., Wang, Y., and Wang, Z. (2022). Assessment of influencing factors on non-point source pollution critical source areas in an agricultural watershed. Ecol. Indic. 141:109084. doi: 10.1016/j.ecolind.2022.109084
Wei, J., Hu, R., Li, Y., and Shen, Y. (2024). Regional disparities, dynamic evolution, and spatial spillover effects of urban-rural carbon emission inequality in China. Front. Ecol. Evol. 12:1309500. doi: 10.3389/fevo.2024.1309500
Wen, W., Zhuang, Y., Jiang, T., Li, W., Li, H., Cai, W., et al. (2024). “Period-area-source” hierarchical management for agricultural non-point source pollution in typical watershed with integrated planting and breeding. J. Hydrol. 635:131198. doi: 10.1016/j.jhydrol.2024.131198
Wu, Y., and Zhang, W. (2024). The impact of land transfer on sustainable agricultural development from the perspective of green total factor productivity. Sustainability 16:7076. doi: 10.3390/su16167076
Xie, Y., Jiang, J., and Wang, D. (2024). Green finance policy and labor demand: evidence from China. Pac.-Basin Finance J. 86:102434. doi: 10.1016/j.pacfin.2024.102434
Xiong, Q., Guo, X., and Yang, J. (2024). Digital financial inclusion, land circulation and high-quality development of agriculture. Sustainability 16:4775. doi: 10.3390/su16114775
Xu, H., Tan, X., Liang, J., Cui, Y., and Gao, Q. (2022). Impact of agricultural non-point source pollution on river water quality: evidence from China. Front. Ecol. Evol. 10:858822. doi: 10.3389/fevo.2022.858822
Xu, L., Jiang, J., Lu, M., and Du, J. (2023). Spatial-temporal evolution characteristics of agricultural intensive management and its influence on agricultural non-point source pollution in China. Sustainability 15:371. doi: 10.3390/su15010371
Xu, T., Zhu, Z., and Chen, T. (2024). The impact of green finance on promoting industrial structure upgrading: an analysis of Jiangsu province in China. Sustainability 16:7520. doi: 10.3390/su16177520
Yan, L., He, W., and Qiao, C. (2012). Research on policy financial support for green agricultural industrialization. J. Zhongnan Univ. Econ. Law 191, 88–92. doi: 10.3969/j.issn.1003-5230.2012.02.015
Yang, Z., and Shen, Y. (2023). The impact of intelligent manufacturing on industrial green total factor productivity and its multiple mechanisms. Front. Environ. Sci. 10:1058664. doi: 10.3389/fenvs.2022.1058664
Zang, D., Yang, S., and Li, F. (2022). The relationship between land transfer and agricultural green production: a collaborative test based on theory and data. Agriculture 12:1824. doi: 10.3390/agriculture12111824
Zhang, D., Zhang, Z., and Managi, S. (2019). A bibliometric analysis on green finance: current status, development, and future directions. Finance Res. Lett. 29, 425–430. doi: 10.1016/j.frl.2019.02.003
Zhang, G., Leng, L., Yang, S., Lin, X., Chen, S., Li, G., et al. (2024). Impact of land circulation and agricultural socialized service on agricultural total factor productivity. Econ. Geogr. 44, 181–189. doi: 10.15957/j.cnki.jjdl.2024.04.019
Zhang, W., Gao, P., Chen, Z., and Qiu, H. (2023). Preventing agricultural non-point source pollution in China: the effect of environmental regulation with digitization. Int. J. Environ. Res. Public Health 20:4396. doi: 10.3390/ijerph20054396
Zhang, W., Ke, J., Ding, Y., and Chen, S. (2024). Greening through finance: green finance policies and firms' green investment. Energy Econ. 131:107401. doi: 10.1016/j.eneco.2024.107401
Zhao, J., Ji, Y., Liu, Y., Liu, Q., Bai, J., Song, Y., et al. (2022). Current situation, problems and suggestions on agricultural non-point source pollution in the Yangtze river basin. Environ. Protect. 50, 30–32. doi: 10.14026/j.cnki.0253-9705.2022.17.010
Zhou, P., Yang, S., Wu, X., and Shen, Y. (2022). Calculation of regional agricultural production efficiency and empirical analysis of its influencing factors-based on DEA-CCR model and Tobit model. J. Comput. Methods Sci. Eng. 22, 109–122. doi: 10.3233/JCM-215590
Zhu, Y., Qi, Z., Tian, Z., and Wang, X. (2024). Impact of agricultural agglomeration on agricultural non-point source pollution in the main production areas of the Yangtze River basin: an empirical study based on panel threshold modeling. J. China Agric. Univ. 29, 228–240. doi: 10.11841/j.issn.1007-4333.2024.03.20
Keywords: green finance, agricultural non-point source pollution, government environmental regulation, land transfer, environmental pollution, inventory analysis method
Citation: Shen Y and Zhang X (2024) Finance-driven sustainable development: the impact of green finance on agricultural non-point source pollution and its pathways. Front. Sustain. Food Syst. 8:1430670. doi: 10.3389/fsufs.2024.1430670
Received: 10 May 2024; Accepted: 30 November 2024;
Published: 16 December 2024.
Edited by:
Reetu Verma, University of Wollongong, AustraliaReviewed by:
Diby Francois Kassi, Henan University, ChinaCopyright © 2024 Shen and Zhang. This is an open-access article distributed under the terms of the Creative Commons Attribution License (CC BY). The use, distribution or reproduction in other forums is permitted, provided the original author(s) and the copyright owner(s) are credited and that the original publication in this journal is cited, in accordance with accepted academic practice. No use, distribution or reproduction is permitted which does not comply with these terms.
*Correspondence: Xiuwu Zhang, enh3eHo3MTdAaHF1LmVkdS5jbg==
Disclaimer: All claims expressed in this article are solely those of the authors and do not necessarily represent those of their affiliated organizations, or those of the publisher, the editors and the reviewers. Any product that may be evaluated in this article or claim that may be made by its manufacturer is not guaranteed or endorsed by the publisher.
Research integrity at Frontiers
Learn more about the work of our research integrity team to safeguard the quality of each article we publish.