- College of Management, Sichuan Agricultural University, Chengdu, China
Introduction: Global climate change (CC) significantly impacts sustainable food systems and the agricultural sector, primarily through increasing adverse weather events. This study aims to explore the adaptation strategies farmers use to address these challenges and evaluate the effectiveness of climate-smart agricultural (CSA) practices on food performance.
Methods: We collected data from 720 crop farmers located in three provinces of Pakistan using a random sampling method. To address potential biases, this study employed the endogenous switching regression (ESR) model. This model effectively addresses endogeneity and selection bias by considering both observable and unobservable characteristics.
Results: Study findings indicate that CSA practices substantially enhance net farm returns, reduce volatility, and mitigate downside risks. The analysis also highlights key features affecting the acceptance of CSA practices, including higher education, age, climate information, and availability of agricultural extension services.
Discussion: These insights are essential for policymakers, offering a framework for informed decision-making to tackle CC’s effects on food production, improve living standards, and enhance global food security.
1 Introduction
About 80% of the world’s poorest people live in rural areas and depend on agriculture for their livelihoods and food security. This sector is the primary source of income for about 70% of the global poor and employs around 1.3 billion smallholder farmers (Food and Agriculture Organization, 2017; Agbenyo et al., 2022). However, with the world population expected to reach 9.7 billion by 2050 and surpass 10 billion by the century’s end, agriculture will play a crucial role in meeting the demands of this growing population (Godfray et al., 2010). As well, global warming trend, with average temperatures expected to rise by 0.2°C over the next three decades. The agricultural sector and its related activities make a sustainable contribution to the rising greenhouse atmosphere (IPCC, 2007; Arora et al., 2019). Climate change (CC) forces agriculture to transition (Branca et al., 2011; Agbenyo et al., 2022). Farmers need to improve their capacity and stability in order to adapt effectively to the environment. It is important to note that while CC impacts all regions, its often-imperceptible influence on food production and agriculture poses a particularly severe threat to developing nations, especially in Asia and Africa. These areas have been characterized by high temperatures and slow development and are vulnerable to their impact (Hossain et al., 2019; Hussain et al., 2020). Shifting temperatures and rainfall patterns pose a threat to agricultural development (Yildiz, 2019). These aforementioned changes are expected to have both instant and long-standing consequences for worldwide food stuff safety (Praveen and Sharma, 2019). Crop failures, reduced yields, and soil degradation may exacerbate the challenges faced by local economies, worldwide product markets, and food security (Bitterman et al., 2019).
However, CC is a critical issue for global food sustainability (Mase et al., 2017). Extreme events related to CC have a devastating impact on the most impoverished populations, especially small farmers who are particularly vulnerable due to limited land holdings, financial resources, and lack of market access (Paris and Rola-Rubzen, 2018). According to Anuga et al. (2019), farmers in developing countries are more vulnerable to CC impacts. Nonetheless, agricultural systems are becoming increasingly vulnerable to the impacts of CC, demanding adaptations and alterations to present agricultural methods. For example, the food and agriculture organization has proposed the implementation of climate smart agricultural (CSA) methods. These techniques have the potential to mitigate the negative consequences of severe weather events connected with CC while also improving household well-being and agricultural production in emerging nations. This intensity is amplified when combined with the use of digital CC mitigation devices. Several prior lessons have highlighted the enormous effect of CC on farmers’ income (Huang et al., 2015). Furthermore, only a few experts have examined how adaptation to severe weather events affects hazard exposure and household welfare among climate-vulnerable rural farmers (Bouwer, 2019). According to research conducted by Ali and Erenstein (2017), CC has a negative influence on farming in Pakistan, but adjusting to it has beneficial implications. Some of the adaptation strategies, such as smart practices, frequently applied by small-scale growers in emerging economies comprise shifting the cropping calendar, changing the input mix, changing crop rotation, crop diversification, conserving water and soil, diversifying seed varieties, increasing irrigation efficiency, using improved seed, and income diversification (Abdulai, 2018).
According to the Shahzad and Abdulai (2020), severe weather events including excessive temperature, erratic perception, and climate-associated stressors (diseases of crops, dust cyclones, vegetation, and floods) had a substantial impact on Pakistan’s agricultural systems. The usual world temperature has improved by 0.6 to 0.8°C over the past 10 years and is expected to keep rising quickly (The World Bank, 2012). Pakistan’s rainfall has become incredibly unpredictable and heavier over shorter periods. Consequently, farmers occasionally experience water shortages and even drought (Khan, 2015). These climatic changes increase the risk associated with farming operations, have a detrimental impact on geographic areas, and cause floods and a shortage of water (Shannon and Motha, 2015). Additionally, it appears that agricultural yields are decreasing due to these severe weather events (Bocchiola et al., 2019). The worldwide financial systems, farmers’ well-being, food supply, and availability of water are all pointedly impacted by these fluctuations (Field, 2012). According to CC forecasts, a local climatic hazard is still a problem (Planning Commission Government of Pakistan, 2010). While social and economic factors and agronomic inputs contribute to increasing agricultural yields, temperature and rainfall are crucial since they account for one-third of the adaptability in crop production (Ray et al., 2015). Depending on the crop type, extreme weather events tend to have an adverse effect on farming yield, with the range of crop yield variation being between 18 and 43% (Vogel et al., 2019). Specifying these details, it is necessary to do an economic study of agricultural performance during situations of severe weather that have the potential to degrade agricultural output, lower farm net returns, and ultimately impact the welfare of families in rural Pakistan.
According to Eckstein et al. (2019), Pakistan is the number 7th most adversely affected nation globally by environmental degradation. Based on the Economic Survey of Pakistan (GoP, 2014), unfavorable climatic events cost the nation approximately 365 billion Pakistani rupees (PKR) annually due to insufficient water supply degraded soil, pollution, and deforestation. According to a study by Anache et al. (2018) hazards associated with extreme weather also affect soil deterioration, soil-water interaction, waterlogging, humidity, and soil nutrients. Therefore, managing farming fields via adaptation to harsh weather is a choice that growers exposed to hazard requirements make. Because agriculture makes up a more significant portion of the gross domestic product, it has a higher impact on diminished revenue in emerging nations including Pakistan. Agricultural productivity decline is producing ongoing poverty in farming communities and an overall insufficient food supply (Ali and Erenstein, 2017), in addition, environmental hazard management could play an important job in alleviating poverty in rural areas (Hansen et al., 2019). An economic analysis of risk vulnerability due to severe weather conditions reveals their significant impact on farmers’ well-being in the country. Implementing adaptation techniques in response to CC has been shown to increase agricultural output and revenue, based on insights from farming-level datasets (Arslan et al., 2015). For instance, an investigation conducted by Iqbal et al. (2015) indicated that adaptation techniques had a favorable influence on net revenue, whereas an investigation by Abid et al. (2016) displayed that adaptation to CC has a valuable effect on grain production and net agricultural profits. Another survey indicated that producers who employ CC adaptation measures are more food sheltered and have low poverty levels (Ali and Erenstein, 2017). Considering the significance of adaptation to severe weather in terms of growers’ production and net profits that improve farm family well-being, assessing the adapting effect to adverse climate events on products like farm yield and hazard events of farm net returns can provide esteemed information on farming outcomes that may be utilized to design strategies for agricultural development.
Numerous lessons on coping with severe weather events have been highlighted by Nagy et al. (1802), focusing on farmers’ experiences with climate variability, adaptation approaches, and the factors that drive or hinder adoption. While comparing production and well-being indicators between users and non-users can yield valuable insights, such analyzes often overlook confounding variables, including observable family and farm attributes, as well as unobserved factors. Another research employing econometric techniques, like propensity score matching, similarly fails to adequately address for these unobserved variables (Ali and Erenstein, 2017). These hidden characteristics can influence both adoption decisions and farm net revenue, leading to potential biases in estimating farm outcomes. Self-selection may affect these estimates, as farmers with stronger fundamental skills may be more likely to adopt new practices. Ignoring these variables can lead to conflicts regarding the true nature of farmers’ adoption decisions, which are influenced by both observed and unobserved variables (Ma and Abdulai, 2016). To address these challenges, our study employs an Econometric Selection Regression (ESR) approach that accounts for both observable and unobservable heterogeneity, enabling more accurate estimation of choice and outcome models. Additionally, this research examines a variety of crops, including wheat and maize, and analyzes growers’ cultivation techniques, unlike many previous studies that focus on a single crop. Thus, the primary objective of this study is to investigate how the adoption of climate-smart agricultural practices affects food production during adverse weather events.
The rest of this paper is organized as follows. Section 2 presents materials and methods. Section 3 presents results. Section 4 presents discussions, while conclusions, policy implication, limitations and future directions are presented in section 5.
2 Methodology
2.1 Description of study area
Punjab, Khyber Pakhtunkhwa (KP), and Balochistan are the three provinces of Pakistan, each with its own distinct topography and characteristics. The study areas were selected for several key reasons: (1) The three provinces represent a variety of agricultural systems, enabling a comprehensive analysis of CSA adaptations. (2) These regions are particularly affected by CC, experiencing extreme weather events like floods and droughts, making them ideal for studying CSA effectiveness. (3) The provinces significantly contribute to Pakistan’s agricultural output, making it essential to understand how farmers adapt to climate challenges for national food security. (4) The provinces vary in access to agricultural extension services and CC information, providing insight into factors influencing CSA adoption. (5) These regions have engaged farming communities and stakeholders, facilitating effective data collection and relevant findings for policy development. By focusing on these provinces, the study aims to provide valuable insights into farmers’ adaptation strategies in response to CC, informing policymakers and agricultural practitioners. Punjab is situated in the eastern part of the country and is known to be a key agricultural region. It accounts for 56% of Pakistan’s total arable land and accounts for 74% of the country’s total cereal production. KP, formerly known as the North-West Frontier Province, is located in northwestern Pakistan. The province is characterized by hilly terrain, with the Hindu Kush mountain range occupying the western part of the province. While KP is known for its breathtaking landscapes, it is also recognized as an important agricultural region (Figure 1).
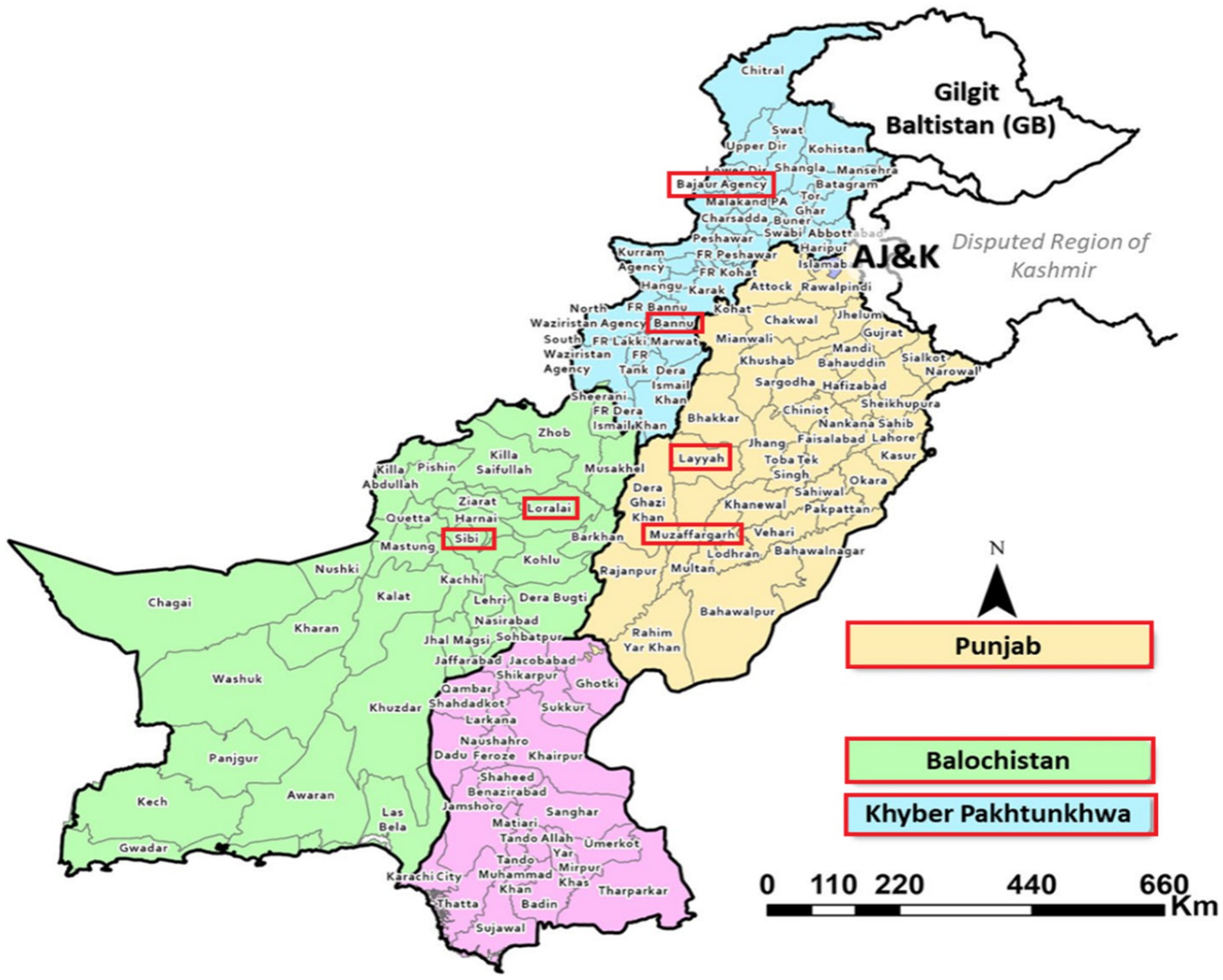
Figure 1. Map of the study areas. The selected three provinces and six district’s boundaries are shown in rad color.
The province contributes a noteworthy share to the country’s total cultivated area and plays a significant role in cereal production. In contrast, Balochistan is positioned in the southwestern part of Pakistan. It stands as the largest province in terms of land area but is sparsely populated. Balochistan is endowed with diverse landscapes, unique natural resources, and rich cultural diversity. The province is picturesque, fertile and plays an important role in tourism and agriculture. Furthermore, Balochistan’s strategic location along the border areas also adds to its importance. Overall, Punjab, Khyber, and Balochistan are integral provinces of Pakistan and each province in its own way contributes to the country’s agricultural sector, tourism and strategic importance.
2.2 Data collection
The current study used data from three provinces of Pakistan. In order to ensure that the sample is representative, a extensive sampling frame was developed by comprehensively considering various factors such as planting patterns, agricultural activities, land area, and climatic conditions in different regions. The selected regions exhibit diverse climatic conditions and cropping patterns, expanding the breadth of the study. Employing a multi-stage random sampling strategy, three provinces were initially chosen based on their climatic and agro-ecological characteristics. Subsequently, two districts were randomly selected from each province, followed by the random selection of two tehsils and three villages from each district. Finally, twenty crop growers were sampled from each village, following in a total of 720 growers participating in interviews for data collection (Figure 2). An organized questionnaire, pre-tested for reliability, was employed to conduct face-to-face interviews and gather farmers’ characteristics and information on farm level. The questionnaire covered various aspects including farmers’ socioeconomic factors, CSA practices, and other pertinent variables. The primary gathered data underwent through coding and editing utilizing Stata 14 software to ensure homogeneity, accuracy, completeness, and coherence before further analysis.
2.3 Adaptation to adverse weather events selection
To establish an adoption criterion based on selection equation model discussed by Di Falco and Veronesi (2014) and Antle (1983), we denote as the variance between projected net returns of farm utility when adopting and when not adopting . In Equation 1a, grower i decides to adopt adverse weather events is greater than zero. As can not be directly observed due to its latent variable, we specify it as an observable components function within the following latent variable model:
The selection equation is then given by:
In Equation 1b, the variable yi signifies whether household i adopts a particular practice (yi = 1) or not (yi = 0). It serves as a binary indicator for adoption status. Meanwhile, Zi encompasses a multitude of factors potentially shaping adoption decisions. These factors include environmental variables, such as extreme weather conditions (e.g., climate associated shocks, rainfall anomaly, and temperature anomaly), as well as long term climate patterns (e.g., rainfall and average temperature). Additionally, socio-economic factors, like farmers’ personal attributes (e.g., education, age, and household size), farm related attributes (e.g., soil types, and farm size), and regional dynamics (e.g., ago-climatic regions like wheat, and maize), play a crucial role. Asset ownership, including number of animals, farm machinery, and infrastructure, also influences adoption choices. Furthermore, institutional and financial considerations, such as extension services, credit availability, and information on CC, are important determinants (Table 1). Lastly, individuals’ perceptions of extreme weather conditions, reflecting subjective assessments of risk and adaptation needs, contribute to adoption behaviors. The parameters βiare estimated to understand the relationship between these explanatory variables and adoption behavior, with the error term presumed to follow a normal distribution with a mean of zero and constant variance, capturing unobserved factors influencing adoption decisions.
2.4 Effects of adapting to severe weather events
In assessing the impact of adjustments to adverse weather, this study employs a moment-based approach inspired by Di Falco and Veronesi (2014) and Antle (1983), focusing on maximizing predictable utility for farm net returns . The analysis prioritizes optimizing relevant moments within the risk exposure distribution (e) based on input utilization. To quantify the risk associated with farm net returns, this study calculate residuals from the net returns function to derive key moments such as kurtosis, variance, and skewness for each farmer. These moments serve as critical risk indicators for farm net returns and grower welfare. Variance reflects the dispersion of net returns around the mean and serves as a measure of volatility. Skewness captures downside risk exposure, indicating the uncertainty of returns falling below expectations. Additionally, kurtosis identifies extreme deviations in returns, thereby highlighting increased risk exposure for farm households. To assess the influence of adopting CSA practices on net returns and associated risks in the context of adverse weather, we assume a linear relationship between these variables, expressed as follows:
In Equation 2, Vi represents a vector of various outcome variables, including farm net returns, downside risk exposure, volatility, and kurtosis. Similarly, Xi denotes another vector that comprises explanatory variables such as soil types (clay soil and loamy soil), farmer characteristics (education, age, family size, farm-related factors), and financial and institutional variables (access to credit and extension services). Additionally, is a binary dummy variable indicating the acceptance of CSA practices. The parameters α and ψ need to be estimated, while denotes the error term in the model.
2.5 Calculation and identification
Given our reliance on study data and the non-random nature of selection into adaptation, it is imperative to employ a method addressing selection bias. Consequently, we used the ESR method to balance selection bias emerging from both observable and un-observable heterogeneity within the sample, as discussed by Wu (2022). The ESR model is structured in two phases: first phase addresses the choice to use, as stated in selection (Equation 1b), we formulate separate equations for users and not users as outcome as outcome equations, as detailed below:
In this context, and represent outcomes (e.g., kurtosis, net returns of farm, downside risk exposure, and volatility) for users and not users, correspondingly (Equation 4). is a vector of explanatory variables supposed to affect these outcomes, with & as the parameters to be assessed. Ci denotes error term linked with outcome variables.
In assessment process, consideration is given to the potential overlap between in selection and outcome formulas. Through, for proper model identification, it is vital that at minimum one variable present in does not appear in . In this research, CC data and individuals’ perceptions of extreme weather conditions are used as instrumental variables to establish the model’s identification, with a focus on their exclusive impact on the adoption aspect without affecting the outcome variables.
ESR method addresses selection bias caused by unobservable factors, often referred to as the omitted variable issue. To mitigate this bias, two important components are introduced in Equations 3a, 3b: the inverse Mills ratio (denoted as ) and the two covariance terms = cov ( ) and = cov ( ).
In this model, and represent error terms with conditional zero mean. Employing the full information maximum likelihood (FIML) technique outlined via Ojo and Baiyegunhi (2020), we measure both the selection and outcome equations concurrently. After applying the ESR model, correlation coefficients (labelled and ) were calculated to evaluate the covariance between the selection and outcome equations. Statistical significance of ( or ) indicates the presence of selection bias due to unobservable factors. When ( ), a positive selection bias occurs; when ( ), a negative selection bias occurs. If the signs of ( and ) are opposite, it indicates that the decision is influenced by comparative advantage. Instead, similar signs point to “hierarchical sorting,” whereby adopters achieve better outcomes than non-adopters regardless of acceptance decision.
2.5.1 Average treatment effects on the treated (ATT)
The ESR model estimates enable the calculation of the ATT. Using Equation 5, we can express the ATT as follows:
The selection equation highlights the possible endogeneity of variables for instance extension services and credit restrictions. The involvement of extension officers in providing information on extreme weather conditions can significantly affect growers’ choices to accept specific practices of CSA, thereby impacting farm production outcomes. Similarly, credit constraints, which may limit farmers’ ability to invest in adaptation measures, can be influenced by changes in farm productivity and income resulting from adaptation to dangerous weather situations. This interconnections suggests the possible endogeneity of extension services and credit constraints. Given the binary nature of these variables, this research utilizes the control function method, following Wooldridge (2015) approach, to address endogeneity concerns associated with extension services and credit restrictions. In this study, model endogenous variables solely based on other variables in the selection equation. Additionally, instrumental variables are introduced into the first-step assessment process to mitigate endogeneity concerns and enhance the robustness of analysis. These instrumental variables are carefully selected to ensure their relevance and validity in capturing the exogenous variation in extension contact and credit constraints, thereby improving the reliability of results.
In this model, Gi denotes potential endogenous variables, while Ii represents instrumental variables and ζi accounts for random error in (Equation 6). To ensure proper identification, we have integrated the distance to the extension department as an instrumental variable. This variable influences access to services of extension without directly influencing adaptation to extreme weather conditions. To address potential endogeneity arising from credit constraints, this research utilized the presence of a personal relationship in a credit institution as an instrumental variable. It is crucial to note that instrumental variables would not associate with others utilized for ESR method identification. To effectively tackle endogeneity, we integrated observed standards of possible endogenous variables and assessed residuals into the selection equation as follows:
Within this framework, Ri signifies the residuals vector derived from Equation 7, which pertains to the endogenous variables. These residuals play a crucial role as a regulatory tool in the subsequent estimation stage, ensuring a dependable assessment of the endogenous variables. Utilizing this approach enables us to perform a rigorous regression-based Hausman test to assess the exogeneity of variables that might be endogenous, and consistent with the prescribed framework (Wooldridge, 2015).
3 Results
3.1 Descriptive statistics of weather-related agricultural practices
Results in Table 2, show that nearly 46% of farmers in the region have adopted CSA practices. This reflects a growing awareness of sustainable farming methods among the agricultural community. Over the past 35 years, the region has experienced an average daily temperature of 27 degrees Celsius, accompanied by average daily precipitation of approximately 1 mm. Notably, the mean rainfall anomaly indicates positive rainfall shocks, suggesting periods of increased precipitation that could benefit crop production. Conversely, the mean temperature anomaly reveals negative temperature shocks, which may pose challenges for agricultural sustainability. Additionally, the average farm size in the region is 7.42 acres, indicating a predominance of small-scale farming households. These households typically comprise 6–8 individuals, underscoring the social and economic dynamics that influence farming practices. Agricultural extension agents have engaged with approximately 55.11% of the farms, highlighting the role of extension services in promoting CSA adoption and supporting farmers. Despite these positive indicators, about 25% of farmers report facing financial resource limitations, which can hinder their ability to invest in CSA practices. This financial barrier is critical, as it may affect the long-term viability of sustainable agricultural methods in the region. The findings indicate a significant portion of farmers actively embracing CSA practices, which is encouraging given the increasing climate variability. The positive rainfall shocks observed could be instrumental in facilitating this adoption, as they may provide the necessary moisture for crops. However, the negative temperature shocks present a substantial challenge, as they can lead to heat stress on plants and reduce yields.
Table 3 presents the different characteristics between individuals who have adopted CSA practices and those who have not. Particularly, adopters tend to possess greater affluence and manage larger-scale farms compared to their non-adopter counterparts. The adopters also exhibit higher livestock holdings and a propensity to invest in agricultural machinery, indicative of their economic prosperity. Furthermore, they benefit from enhanced access to CC knowledge, maintain closer ties with extension workers, and are more attuned to dangerous weather events. However, in terms of climate-related attributes, there is minimal divergence between adopters and non-adopters. The subsequent section reveals disparities in alteration, kurtosis, skewness, and net returns. Notably, adopters farmers report significantly greater net returns in compare to non-adopters. While risk measures such as alteration and kurtosis of farmhouse net returns do not exhibit substantial differences between the two groups. It is essential to emphasize that these statistics only capture average distinctions and do not consider other variables or farmer-specific attributes. Therefore, to mitigate potential selection bias and comprehend the diverse influence of adopters and non-adopters, the current study employed an ESR technique to model the process of adaptation to challenging weather conditions.
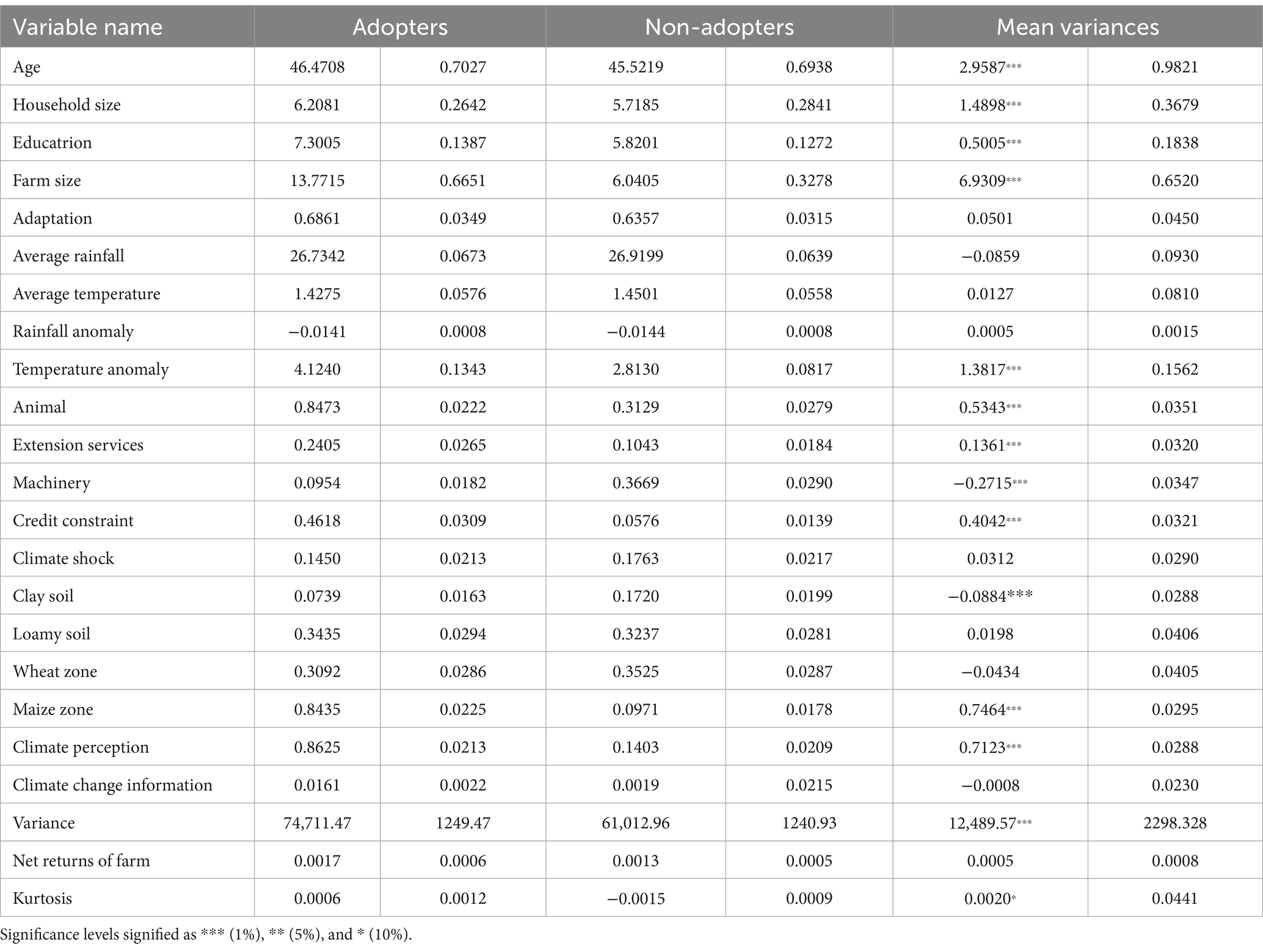
Table 3. Analyzing adopters and non-adopters: a comparative examination with descriptive statistics and mean–variance analysis.
3.2 Factors influencing adaptation to severe weather events
In Tables 4–7, the consistent use of identical variables provides a clear insight into the research findings. The temperature anomaly coefficient stands out prominently, recording a positive value of 74.670, indicating a strong correlation with CSA practices adoption. This underscores the importance of temperature anomalies in driving farmers to embrace adaptive measures in response to extreme weather variations. Conversely, the rainfall anomaly coefficient presents an intriguing observation, displaying a negative value of −0.506. This suggests that heavy rainfall may hinder CSA practices’ adoption, potentially diminishing the perceived necessity for adaptive measures among farmers. Furthermore, the age of farmers emerges as a significant factor positively associated with CSA practices adoption. This aligns with the notion that education and experience influence farmers’ willingness to adopt innovative practices. The education further reinforces this trend, highlighting the crucial role of knowledge in fostering adaptation efforts. Agricultural extension services are found to play a pivotal role in serving CSA practices adoption, as evidenced via consistently positive coefficients across all specifications. Extension agents significantly facilitate adaptive measure adoption among farmers. Previous change connected shocks increase the likelihood of CSA practice acceptance, reflecting firsthand experience’s impact on adaptive behaviors. Location, especially in crop-growing regions, positively influences adaptation, suggesting better coping mechanisms for adverse weather.
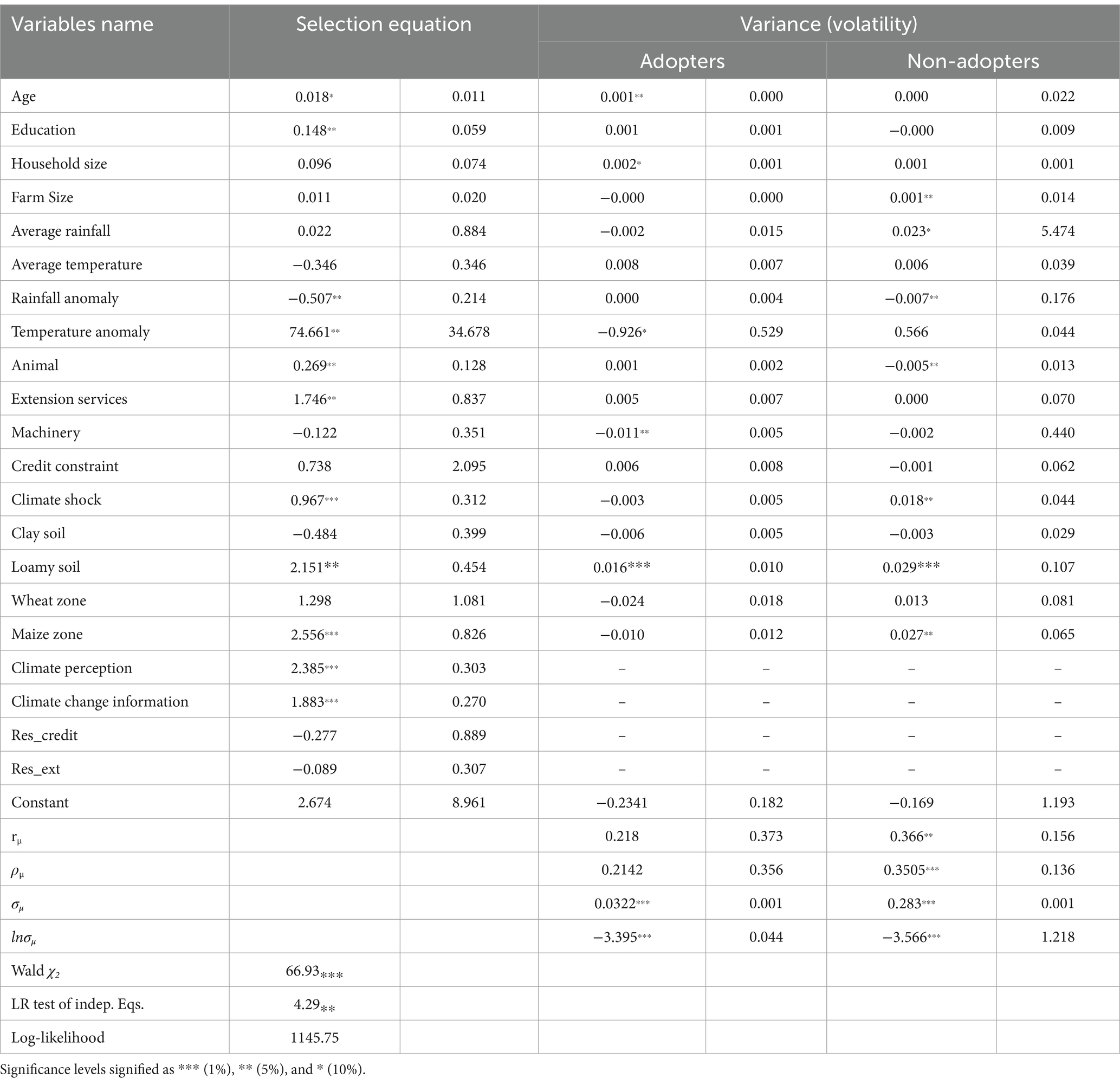
Table 4. Factors influencing adaptation to adverse weather events and their effects on net return of farm volatility.
3.3 Net returns of farms’ volatility
Table 4, the estimated values for farm net return volatility shed light on the influence of various factors on income stability in agriculture. Notably, for non-adopters, the coefficient associated with average rainfall stands out at 0.023, signifying that average rainfall inclines to raise income volatility among this group, leading to greater uncertainty in earnings. Conversely, adopters display a different pattern, with an average rainfall coefficient of 0.002, signifying a diminishing in the net returns of farm volatility. However, this reduction lacks statistical significance, indicate that the effect of average rainfall on volatility for adopters may be less pronounced compared to non-adopters. Moreover, the temperature anomaly variable shows a highly significant negative coefficient of −0.926, indicating that adopters achieve greater income stability by adapting to extreme temperature fluctuations, thereby enhancing resilience to climate-related risks. Additionally, for non-adopters, the coefficient for precipitation incongruity is adverse −0.007 and substantial, indicating a decrease in farm net return volatility in response to rainfall anomalies. This suggests potential predictability and income shock mitigation within this group. Furthermore, the farm size variable exhibits a positive coefficient of 0.001, suggesting that larger farms tend to experience higher net return volatility. This positive association between farm size and income instability may be attributed to operational and logistical challenges.
3.4 Exploring downside risks exposure and kurtosis
Table 5 illuminates the influence of adapting to severe weather events on the skewness of net returns of farms, providing invaluable insights into the dynamics of downside risk in agriculture. While average rainfall and temperature show minimal impact on skewness, anomalies in both variables significantly affect skewness levels. Temperature anomalies emerge as pivotal factors shaping the risk profiles of both adopters and non-adopters. Adopters experience an optimistic effect of temperature anomalies 0.488, indicating reduced risk of crop failure in extreme temperature conditions. Conversely, non-adopters face adverse effects −0.550, suggesting increased vulnerability to crop failure due to temperature extremes. This highlights the critical role of adaptation strategies in managing risks associated with extreme temperatures. Furthermore, rainfall skewness demonstrates a significant correlation with skewness for non-adopters 0.004, underscoring the impact of excessive rainfall on downside risk. Additionally, farm size negatively affects skewness for non-adopters 0.001, likely due to operational efficiencies leading to a higher likelihood of crop failure on larger farms. Soil type also plays a significant role, with sandy loam soil benefiting adopters but posing risks for non-adopters −0.005, particularly in wheat and maize zones. Additionally, non-adopters in crop zones exhibit higher exposure to downside risk equated to those in other cropping zones, emphasizing the advantages of inter-crop growing in reducing downside risk and promoting diversified agricultural practices. These conclusions underline the requirement for tailored adaptation strategies to effectively manage downside risk in varying agricultural contexts.
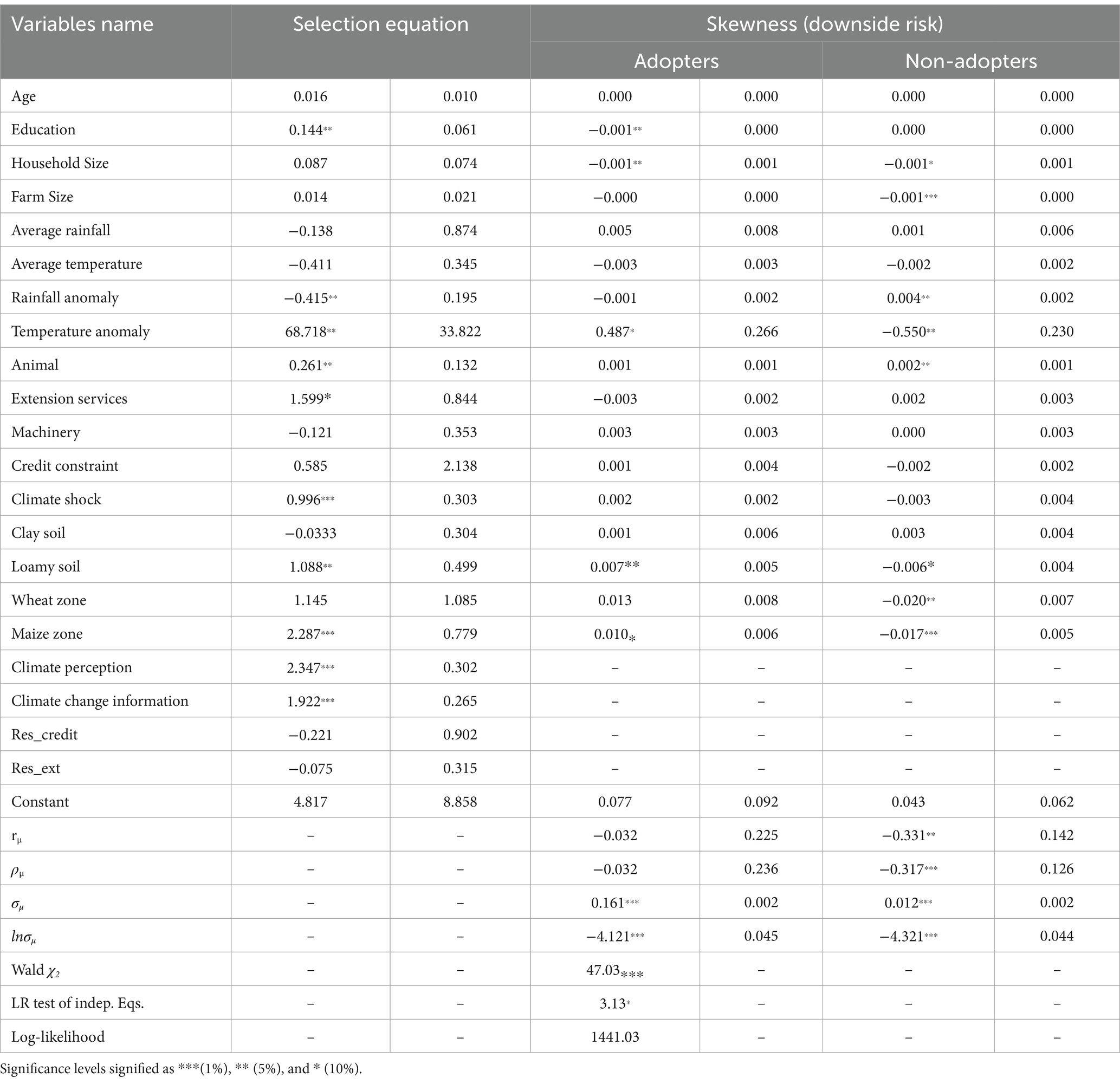
Table 5. Factors affecting adaptation to adverse weather events and their effect on downside risk exposure.
The investigation in this study expanded specifically focusing on growers’ responses to adverse weather events to derive the kurtosis function coefficients, as documented in Table 6. For adopters, the temperature anomaly exhibits a noteworthy negative coefficient of −0.277, and outcome is statistically substantial at the 5% level. This finding aligns with the observations on variance and implies that temperature anomalies serve to reduce the kurtosis of adopters’ farm net returns. In essence, it indicates that adaptation measures taken by adopters help mitigate the extremeness of their net benefit distribution, resulting in a smoother and less risky distribution. In contrast, the temperature anomaly coefficient for non-adopters is significant at 0.051, indicating that, while the effect is not as pronounced as with adopters, temperature anomalies do contribute to an elevation in the kurtosis of non-adopters. This suggests that non-adopters are more susceptible to experiencing extreme variations in their farm net returns due to temperature anomalies. Additionally, an intriguing observation is made concerning farming technology ownership. It significantly decreases the adopters’ kurtosis. This implies that having access to farming equipment has dampening impact on the tails of the distribution of net returns for adopters, making their income distribution more stable and less subject to extreme values. This may reflect the efficiency and productivity gains associated with the mechanization of farming processes.
3.5 Farm net returns
Table 7 provides a revealing comparison of the effects of temperature anomalies on adopters and non-adopters. Adopters experience a marked advantage, with a temperature anomaly coefficient of 8.161%, compared to non-adopters at −8.031%, both statistically significant. This indicates that embracing measures to tackle extreme temperatures leads to higher net returns of farms for users. Conversely, not all users seem to benefit from rainfall anomalies, as evidenced by the positive coefficient for rainfall variance of 0.044%. This suggests that surplus rainfall helps sustain income for non-adopters grappling with water scarcity challenges. Education emerges as a pivotal factor influencing net returns of farms for both users and not users amidst adverse weather events. Substantial coefficients highlight the role of knowledge in maximizing the benefits of CSA practices. However, farm size exhibits negative coefficients for both groups, implying that larger farms tend to yield lower net returns of farms compared to smaller ones. Conversely, a higher number of animals within a household shows positive coefficients, suggesting that animal husbandry is allied with increased returns for users and not users. Sandy load soil presents negative coefficients, but extension facilities play a significant role for both. This underscores the positive impact of agri-extension facilities on farm net benefits for both groups. Furthermore, prior climate-related shocks significantly reduce returns for not users, highlighting the adverse effect of such shocks on net returns of farms and emphasizing the need for improved coping mechanism to alleviate the impact of adverse weather events.
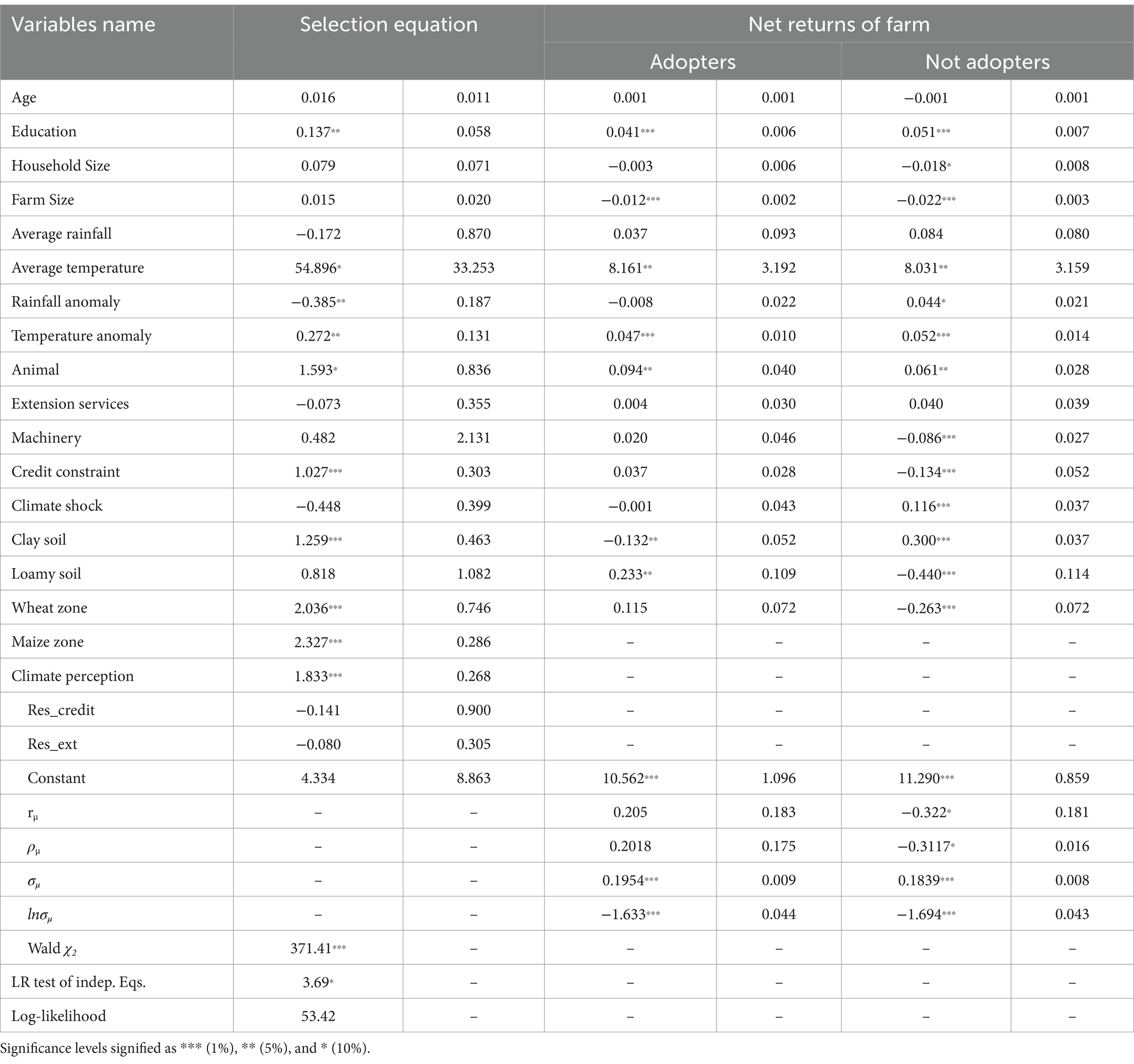
Table 7. Factors that affect the adaption to adverse weather events and its effect on the net returns of a farm.
3.6 Effect of smart practices adoption on-farm risk and net profit
Table 8 presents the ATT, which addresses selection bias and evaluates disparities between adopters and non-adopters of smart practices. The ATT for farm net returns is significantly positive at 0.225, indicating a 2% increase in net returns attributable to the adoption of smart practices in response to challenging weather conditions. This adaptation not only reduces production risks substantially but also enhances overall farm profitability. The counterfactual analysis shows a notable negative ATT of −0.009 for the variance in farm net returns, suggesting a significant 33% reduction in volatility. This leads to a remarkable 126% decrease in downside risk exposure and crop loss. The mean skewness further illustrates this transformation, shifting from −0.005 to 0.001. Additionally, the ATT for kurtosis is also negative at 0.0001, maintaining high significance at the 1% level, which reflects a 5.90% reduction in kurtosis. This reduction indicates that adapting to severe weather conditions decreases the likelihood of extreme outcomes, thereby contributing to greater stability in farm net returns among adopters. Overall, these findings highlight the positive impact of adopting smart practices on farm net revenues, volatility reduction, and the mitigation of downside risk exposure during adverse weather events. This underscores the importance of proactive adaptation strategies in promoting agricultural productivity and resilience.
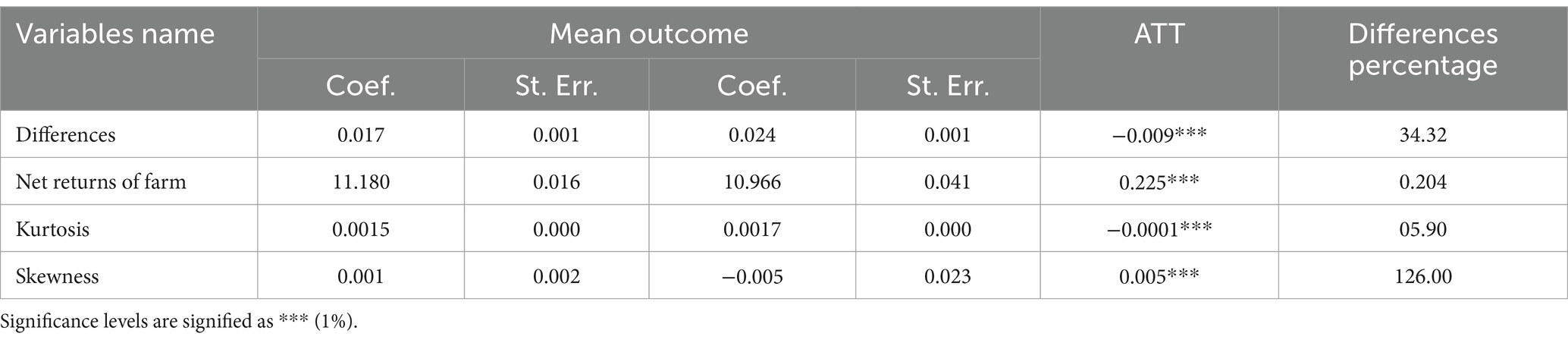
Table 8. The effects of adaptability to adverse weather events on profits of net farm volatility and risk of downside exposures.
4 Discussion
Severe weather events, for instance, high temperatures and heavy rainfall, along with climate-related fluctuations, pose a significant risk to crop production. To mitigate these challenges, risk-averse farm households in Pakistan employ CSA practices. These practices involve various strategies, including the use of drought-resistant and genetically modified seed varieties, as well as high-yielding regional varieties tailored to specific farm environments. The adoption of CSA practices offers promising technical solutions to alleviate climate-related risks in Pakistan. It not only diminishes the exposure to hazards but also enhances farm net returns, ultimately improving the farmers’ welfare. Though, implementing emerging technologies like conservation farming, which incorporates CSA practices, presents several challenges due to complex, interconnected issues. Access to proper guidance and instruction on these techniques is limited, particularly in impoverished nations, hindering their adoption.
While CSA practices can stabilize farmhouse net returns and mitigate the impact of adverse weather and climate variability to some extent, they may not fully protect against extreme weather events like floods and droughts. In such cases, an index-based policy, as suggested by Lybbert and Carter (2015), could be employed to provide compensation for the severity of these extreme conditions. Another strategy to mitigate drought impact is to supply farmers with drought-resistant seed varieties. This study findings underscore the efficiency of CSA performs in managing severe weather events and climate hazards. Growers’ choices to accept or not accept CSA practices are affected via self-selection and are subject to substantial selection bias. Factors such as climate-associated conditions, socioeconomic variables, and farm-specific attributes significantly influence adoption rates. Current study analysis reveals that the implementation of CSA practices reduces the farm net returns volatility, stabilizing farm output and improving the well-being of farm households in Pakistan.
Furthermore, this research demonstrates that CSA practice adopters adjust their crop schedule to mitigate temperature unpredictability, which reduces the risk for non-adopters. Additionally, increased rainfall lowers irrigation costs and benefits water-intensive crops. Adopters also alter crop planting schedules to avoid temperature spikes, reducing uncommon fluctuations in farm net returns. It is essential to acknowledge that the choice to accept CSA practices is not random but influenced by self-selection, leading to selection bias. To address this issue, present research employ the ESR method, which simultaneously guesstimates outcome and selection equations using the FIML technique. This approach accounts for unobservable features affecting adaptation to adverse climate events. However, it is worth noting that the ESR model has limitations, as it aggregates unobservable heterogeneity, which can vary among individuals.
5 Conclusions and policy implications
5.1 Conclusion
This study explores the CSA practices adoption and its influence on food crop production in Pakistan, focusing on factors influencing growers’ decisions during severe weather events. The analysis of questionnaire data from 720 randomly selected farmers in three provinces reveals substantial variances between CSA practices adopters and non-adopters in terms of farm net returns and hazard-associated variables. Employing an ESR model, the research findings indicate that CSA practices adoption reduces risk exposure and enhances the stability of farm net returns. Specifically, it leads to a 34% reduction in farm net return volatility, a 126% decrease in downside risk exposure, and a 5.90% decline in kurtosis. Preparing for extreme weather events results in a 2% boost in agricultural net returns. These outcomes highlight the potential of CSA practices adoption to improve the well-being of rural households, reduce poverty, and enhance farm-household production in Pakistan. The study identifies education, temperature anomalies, livestock ownership, access to agricultural extension services, and prior climate-associated experiences as significant factors positively influencing CSA practices adoption. Education also positively impacts weather adaptability, emphasizing the importance of education accessibility in rural areas. Livestock ownership increases the possibility of adopting CSA practices in unfavorable climate circumstances, and extension services play a favorable and significant role in both adoption and farmhouse revenues.
5.2 Policy implications
The study suggests potential benefits of CSA practices adoption in increasing farmhouse revenues and reducing downside hazard exposure. Policymakers are encouraged to implement supportive policies, such as subsidies or grants, to assist agricultural families in adopting CSA practices. Financial assistance can offset initial investment costs and incentive more farmers to adopt these practices. Strengthening agricultural extension services is crucial for disseminating knowledge about CSA practices, and policymakers should invest in these services to provide farmers with accurate information. Additionally, policymakers should prioritize developing and implementing risk management strategies to enhance climate resilience among agricultural households, including improving weather forecasting capabilities, promoting crop diversification, encouraging the use of climate-resilient seeds and breeds, and providing access to tailored insurance schemes.
5.3 Limitations and future directions
While the study provides valuable insights, limitations and future research directions should be considered. The exclusive focus on agricultural families limits the broader applicability of findings. Future research should explore CSA practices adoption in diverse agricultural contexts worldwide. The study’s use of cross-sectional data and an ESR model does not establish causation, and future investigations could employ experimental designs to establish causal links. Exploring alternative measures or frameworks for understanding factors influencing CSA practices adoption is recommended. Longitudinal data would enhance comprehension of the long-term effects of adoption, and investigating external factors like government policies, market conditions, and technological advancements can offer valuable insights. A deeper exploration of contextual factors specific to the Pakistani agricultural sector can reveal barriers and facilitators of adoption, providing a more nuanced understanding. Addressing these limitations and pursuing these research directions can advance the understanding of CSA practices’ adoption implications for agricultural communities worldwide.
Data availability statement
The raw data supporting the conclusions of this article will be made available by the authors, without undue reservation.
Author contributions
RT: Conceptualization, Data curation, Formal analysis, Investigation, Methodology, Project administration, Software, Supervision, Validation, Writing – original draft, Writing – review & editing. DL: Conceptualization, Funding acquisition, Investigation, Project administration, Resources, Supervision, Validation, Visualization, Writing – review & editing. NK: Conceptualization, Formal analysis, Methodology, Writing – review & editing.
Funding
The author(s) declare that no financial support was received for the research, authorship, and/or publication of this article. This work was supported by National Social Science Fund of China (Grant Number: 22BJY161).
Conflict of interest
The authors declare that the research was conducted in the absence of any commercial or financial relationships that could be construed as a potential conflict of interest.
Publisher’s note
All claims expressed in this article are solely those of the authors and do not necessarily represent those of their affiliated organizations, or those of the publisher, the editors and the reviewers. Any product that may be evaluated in this article, or claim that may be made by its manufacturer, is not guaranteed or endorsed by the publisher.
References
Abdulai, A. (2018). Simon brand memorial address: the challenges and adaptation to climate change by farmers in sub-Saharan Africa. Agrekon 57, 28–39. doi: 10.1080/03031853.2018.1440246
Abid, M., Schneider, U. A., and Scheffran, J. (2016). Adaptation to climate change and its impacts on food productivity and crop income: perspectives of farmers in rural Pakistan. J. Rural. Stud. 47, 254–266. doi: 10.1016/j.jrurstud.2016.08.005
Agbenyo, W., Jiang, Y., Jia, X., Wang, J., Ntim-Amo, G., Dunya, R., et al. (2022). Does the adoption of climate-smart agricultural practices impact farmers’ income? Evidence from Ghana. Int. J. Environ. Res. Public Health 19:3804. doi: 10.3390/ijerph19073804
Ali, A., and Erenstein, O. (2017). Assessing farmer use of climate change adaptation practices and impacts on food security and poverty in Pakistan. Clim. Risk Manag. 16, 183–194. doi: 10.1016/j.crm.2016.12.001
Anache, J. A., Flanagan, D. C., Srivastava, A., and Wendland, E. C. (2018). Land use and climate change impacts on runoff and soil erosion at the hillslope scale in the Brazilian Cerrado. Sci. Total Environ. 622, 140–151. doi: 10.1016/j.scitotenv.2017.11.257
Antle, J. M. (1983). Incorporating risk in production analysis. Am. J. Agric. Econ. 65, 1099–1106. doi: 10.2307/1240428
Anuga, S. W., Gordon, C., Boon, E., and Surugu, J. M.-I. (2019). Determinants of climate smart agriculture (CSA) adoption among smallholder food crop farmers in the Techiman municipality, Ghana. Ghana J. Geogr. 11, 124–139. doi: 10.4314/gjg.v11i1.8
Arora, B., Wainwright, H. M., Dwivedi, D., Vaughn, L. J., Curtis, J. B., Torn, M. S., et al. (2019). Evaluating temporal controls on greenhouse gas (GHG) fluxes in an Arctic tundra environment: an entropy-based approach. Sci. Total Environ. 649, 284–299. doi: 10.1016/j.scitotenv.2018.08.251
Arslan, A., McCarthy, N., Lipper, L., Asfaw, S., Cattaneo, A., and Kokwe, M. (2015). Climate smart agriculture? Assessing the adaptation implications in Zambia. J. Agric. Econ. 66, 753–780. doi: 10.1111/1477-9552.12107
Bitterman, P., Bennett, D. A., and Secchi, S. (2019). Constraints on farmer adaptability in the Iowa-Cedar River basin. Environ. Sci. Pol. 92, 9–16. doi: 10.1016/j.envsci.2018.11.004
Bocchiola, D., Brunetti, L., Soncini, A., Polinelli, F., and Gianinetto, M. (2019). Impact of climate change on agricultural productivity and food security in the Himalayas: a case study in Nepal. Agric. Syst. 171, 113–125. doi: 10.1016/j.agsy.2019.01.008
Bouwer, L. M. (2019). “Observed and projected impacts from extreme weather events: implications for loss and damage” in Loss and damage from climate change: Concepts, methods and policy options. eds. R. Mechler, L. Bouwer, T. Schinko, S. Surminski, and J. Linnerooth-Bayer (Cham: Springer), 63–82.
Branca, G., McCarthy, N., Lipper, L., and Jolejole, M. C. (2011). Climate-smart agriculture: A synthesis of empirical evidence of food security and mitigation benefits from improved cropland management. Rome: Food and Agriculture Organization of the United Nations (FAO).
Di Falco, S., and Veronesi, M. (2014). Managing environmental risk in presence of climate change: the role of adaptation in the Nile Basin of Ethiopia. Environ. Resour. Econ. 57, 553–577. doi: 10.1007/s10640-013-9696-1
Eckstein, D., Künzel, V., and Schäfer, L. (2019). Global climate risk index 2019: who suffers most from extreme weather events. Weather-related loss events in 2017. Berlin: Germanwatch.
Field, C. B. (2012). Managing the risks of extreme events and disasters to advance climate change adaptation: Special report of the intergovernmental panel on climate change. Cambridge: Cambridge University Press.
Food and Agriculture Organization (2017). Food and agriculture: Driving action across the 2030 agenda for sustainable development. Rome: FAO.
Godfray, H. C. J., Beddington, J. R., Crute, I. R., Haddad, L., Lawrence, D., Muir, J. F., et al. (2010). Food security: the challenge of feeding 9 billion people. Science 327, 812–818. doi: 10.1126/science.1185383
GoP (2014). Economic survey of Pakistan 2013-14. Economic Advisor’s wing. Islamabad: Ministry of Finance, government of Pakistan.
Hansen, J., Hellin, J., Rosenstock, T., Fisher, E., Cairns, J., Stirling, C., et al. (2019). Climate risk management and rural poverty reduction. Agric. Syst. 172, 28–46. doi: 10.1016/j.agsy.2018.01.019
Hossain, M. S., Arshad, M., Qian, L., Zhao, M., Mehmood, Y., and Kächele, H. (2019). Economic impact of climate change on crop farming in Bangladesh: an application of Ricardian method. Ecol. Econ. 164:106354. doi: 10.1016/j.ecolecon.2019.106354
Huang, J., Wang, Y., and Wang, J. (2015). Farmers' adaptation to extreme weather events through farm management and its impacts on the mean and risk of rice yield in China. Am. J. Agric. Econ. 97, 602–617. doi: 10.1093/ajae/aav005
Hussain, M., Butt, A. R., Uzma, F., Ahmed, R., Irshad, S., Rehman, A., et al. (2020). A comprehensive review of climate change impacts, adaptation, and mitigation on environmental and natural calamities in Pakistan. Environ. Monit. Assess. 192:48. doi: 10.1007/s10661-019-7956-4
IPCC (2007). The physical science basis. Contribution of working group I to the fourth assessment report of the intergovernmental panel on climate change. Cambridge: Cambridge University Press.
Iqbal, M., Ahmad, M., and Mustafa, G. (2015). Impact of farm households’ adaptation on agricultural productivity: Evidence from different agro-ecologies of Pakistan. London: MPRA.
Khan, M. A. (2015). “Climate change risk and reduction approaches in Pakistan” in Disaster risk reduction approaches in Pakistan. eds. R. Atta-Ur, A. N. Khan, and R. Shaw (Tokyo: Springer).
Lybbert, T. J., and Carter, M. R. (2015). “Bundling drought tolerance and index insurance to reduce rural household vulnerability to drought” in Sustainable economic development. ed. S. Asefa (Amsterdam: Elsevier).
Ma, W., and Abdulai, A. (2016). Linking apple farmers to markets: determinants and impacts of marketing contracts in China. China Agric. Econ. Rev. 8, 2–21. doi: 10.1108/CAER-04-2015-0035
Mase, A. S., Gramig, B. M., and Prokopy, L. S. (2017). Climate change beliefs, risk perceptions, and adaptation behavior among Midwestern US crop farmers. Clim. Risk Manag. 15, 8–17. doi: 10.1016/j.crm.2016.11.004
Nagy, G. J., Filho, W. L., Azeiteiro, U. M., Heimfarth, J., Verocai, J. E., and Li, C. (1802). An assessment of the relationships between extreme weather events, vulnerability, and the impacts on human wellbeing in Latin America. Int. J. Environ. Res. Public Health 15:15. doi: 10.3390/ijerph15091802
Ojo, T., and Baiyegunhi, L. (2020). Determinants of climate change adaptation strategies and its impact on the net farm income of rice farmers in south-West Nigeria. Land Use Policy 95:103946. doi: 10.1016/j.landusepol.2019.04.007
Paris, T., and Rola-Rubzen, M. F. (2018). Gender dimension of climate change research in agriculture: Case studies in Southeast Asia. Los Baños: Southeast Asian Regional Center for Graduate Study and Research in Agriculture.
Planning Commission Government of Pakistan (2010). Task force on climate change. Final report. Islamabad: Planning Commission Government of Pakistan.
Praveen, B., and Sharma, P. (2019). A review of literature on climate change and its impacts on agriculture productivity. J. Public Aff. 19:e1960. doi: 10.1002/pa.1960
Ray, D. K., Gerber, J. S., MacDonald, G. K., and West, P. C. (2015). Climate variation explains a third of global crop yield variability. Nat. Commun. 6:5989. doi: 10.1038/ncomms6989
Shahzad, M. F., and Abdulai, A. (2020). Adaptation to extreme weather conditions and farm performance in rural Pakistan. Agric. Syst. 180:102772. doi: 10.1016/j.agsy.2019.102772
Shannon, H. D., and Motha, R. P. (2015). Managing weather and climate risks to agriculture in North America, Central America and the Caribbean. Weather Clim. Extrem. 10, 50–56. doi: 10.1016/j.wace.2015.10.006
Vogel, E., Donat, M. G., Alexander, L. V., Meinshausen, M., Ray, D. K., Karoly, D., et al. (2019). The effects of climate extremes on global agricultural yields. Environ. Res. Lett. 14:054010. doi: 10.1088/1748-9326/ab154b
Wooldridge, J. M. (2015). Control function methods in applied econometrics. J. Hum. Resour. 50, 420–445. doi: 10.3368/jhr.50.2.420
Wu, F. (2022). Adoption and income effects of new agricultural technology on family farms in China. PLoS One 17:e0267101. doi: 10.1371/journal.pone.0267101
Keywords: adoption, food security, climate change, crop challenges, endogenous switching regression
Citation: Tunio RA, Li D and Khan N (2024) Maximizing farm resilience: the effect of climate smart agricultural adoption practices on food performance amid adverse weather events. Front. Sustain. Food Syst. 8:1423702. doi: 10.3389/fsufs.2024.1423702
Edited by:
Siyabusa Mkuhlani, International Institute of Tropical Agriculture (IITA), KenyaReviewed by:
Farirai Rusere, University of the Witwatersrand, South AfricaMichele Moretti, University of Pisa, Italy
Copyright © 2024 Tunio, Li and Khan. This is an open-access article distributed under the terms of the Creative Commons Attribution License (CC BY). The use, distribution or reproduction in other forums is permitted, provided the original author(s) and the copyright owner(s) are credited and that the original publication in this journal is cited, in accordance with accepted academic practice. No use, distribution or reproduction is permitted which does not comply with these terms.
*Correspondence: Dongmei Li, Y25kbGRtQDE2My5jb20=