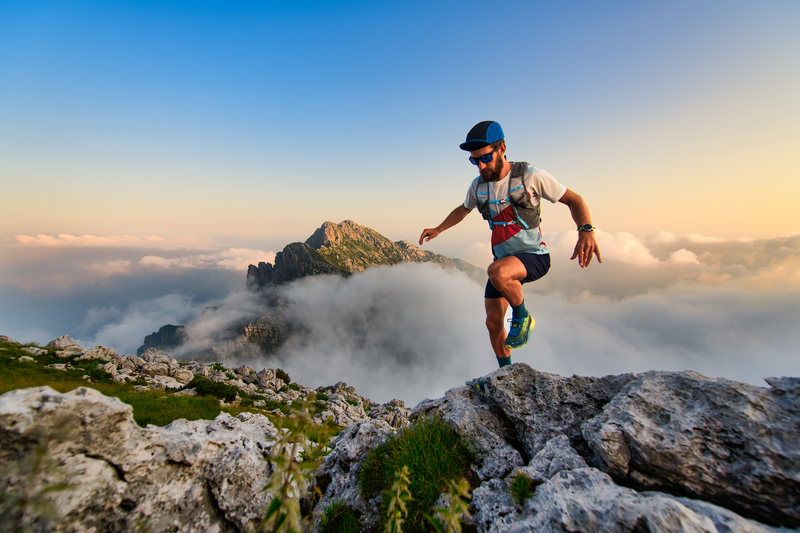
94% of researchers rate our articles as excellent or good
Learn more about the work of our research integrity team to safeguard the quality of each article we publish.
Find out more
ORIGINAL RESEARCH article
Front. Sustain. Food Syst. , 06 November 2024
Sec. Land, Livelihoods and Food Security
Volume 8 - 2024 | https://doi.org/10.3389/fsufs.2024.1420700
This article is part of the Research Topic Land Management and Food/Nutrition (In)Security In Mixed Farming Systems View all 24 articles
Analysis of grain production performance can provide reference information to explore multiple cropping options and further improve the resource use efficiency of farming methods. This study investigated the spatiotemporal dynamics of grain production performance and efficiency of major crop production systems (CPS) in the Ethiopia’s Blue Nile Basin. The results show that only 39% of the basin is currently cultivated, although a significant cropland expansion (10%) was recorded between 1985 and 2020. The study identified 11 major CPS, mostly practiced in the basin. Of these, single cropping based on the main rainy season (Meher-Only) covers the largest area (26%), followed by Meher-Residual-Intermittent (12%) and Meher-Belg-Dependable (11%). Extended-Meher, Meher-Residual-Dependable, Meher-Residual-Intermittent, and Meher-Belg-Dependable are the four more powerful CPS with higher efficiency. Comparatively, CPS practiced in Wet-Woyna-Dega and Wet-Dega have better overall performance. Findings confirm that agricultural space management (land) and green-water (rainfall) utilization are the most influential factors, followed by land use planning and land use systems (CPS) invention. As landscape suitability for grain production governs future performance, in the low elevation and flood plains parts of the basin, the possibility of creating additional space into the food system is very high. In mountainous and high-altitude regions, the efficiency of grain production will decrease because incorporating additional arable land into the food system is trivial. In the last three decades, in BNB, only 10% of arable land (equivalent to 30 million quintals of food) has been added to the good system, which can support approximately 6 million people. Compared to the population growth of the basin (12 million 1985–2020), its contribution to the food system was less than 50%. This confirms that multiple cropping systems, such as Residual moisture-based CPS, have played a significant role in boosting the food system in the basin. Therefore, improving grain production performance/efficiency requires targeted investments, including the invention of more adaptable crop varieties, efficient cropping practices, and the introduction of advanced agricultural space and water management technologies. The results of the study will help identify important policy gaps and suggest possible options to enhance residual farming and other multiple cropping systems.
As a developing agricultural country, Ethiopia relies heavily on rainfed agriculture for almost 95% of its food production, making its development strategies a global topic of discussion (Ahadu, 2019). With the population expected to double in 20 years (CSA, 2020), Ethiopia must develop a strategy to double food production accordingly (Endalew et al., 2015). In 1990, the food requirement for 45 million people was 350 million quintals per year (Taddese, 2001; CSA, 2014). By 2020, this need increased to almost 1 billion quintals for over 120 million people (CSA, 2020; Tekeste, 2021). Increasing food shortages have resulted in increasing food insecurity (Wani et al., 2009; Rahmato, 2003; Zerssa et al., 2021; CSA, 2020). Opinions differ on the reasons for Ethiopia’s increased grain production: some attribute this to land management and inputs, while others point to the expansion of cultivated areas. The literature shows that total grain produced from the area under cereal crops has increased significantly, from 60 to 80 million quintals in 1980/85 to 316–350 million quintals in 2020/23—an overall increase of 300% (CSA, 2020). The CSA attributes this growth primarily to productivity improvements. Non-spatial data show that the cultivated area of the country increased from 5 to 6 million hectares in 1980–85 to 14–17 million hectares in 2020–23, with productivity increasing from 10 to 12 quintals per hectare to 26 to 28 quintals per hectare (CSA, 2020; Teshome, 2014). Overall, existing data on grain production are inconsistent and do not provide a clear picture of spatiotemporal trends, potentially leading to misjudgment of the development trajectories of the country.
The main data source for agricultural production in Ethiopia is the Central Statistical Agency (CSA) (Teshome, 2014). Although CSA national census data are detailed and spatially comprehensive, they have limitations in temporal coverage and accessibility. Annual agricultural surveys provide more consistent temporal data but lack detail and representativeness. Regional and seasonal variations in crop production systems (CPS) create inconsistencies in the literature and make it difficult to understand trends in cropland and grain production at larger scales (Teshome, 2014). Although case study data can provide greater accuracy, its inconsistencies and terminological differences hinder scalability to the national level. Therefore, conducting spatially explicit analyses of spatiotemporal trends in grain production and productivity is challenging. In addition, outdated and poorly managed information makes it difficult to integrate and update, further hindering effective planning and evidence-based decision-making. In summary, there exist about four main limitations that lead to data inconsistencies in grain production: (i) methodological issues, (ii) different definitions and meanings, (iii) lack of thematic detail and scope, and (iv) spatial and temporal limitations with (v) data management issues. To address these root causes, the authors have identified four key research gaps: (1) the need for spatially explicit and multi-temporal data, (2) limited local knowledge about the potential and challenges of crop production systems (CPS), (3) financial and methodological challenges in regularly assessing land use or cultivation practices, and (4) technical limitations.
Data inconsistencies are mainly caused by differences in definition, meaning, and approach and lead to incorrect assumptions (Kassawmar, et al., 2018b). Many studies typically attribute changes in grain volume to (a) changes in arable land (Teshome, 2014) and (b) changes in inputs (Silva, et al., 2021). However, other particularly overlooked factors include land allocation strategies and land use shifts as well as changes in crop production systems (CPS) (Korbu, et al., 2020). The former deals with land use and land cover changes (LULC), while the latter refers to changes in land use systems (LUS). The spatiotemporal dynamics of grain production reflect the diverse types and forms of land use systems (LUS) and crop production systems (CPS) across the globe, influenced by local variations in climate, soil, economic factors, social structures, and historical contexts (Yu et al., 2021; Lesur-Dumoulin et al., 2018; Panigrahy et al., 2011; Wu et al., 2015). The nature of CPS in Ethiopia is extremely complex as they are conditioned by unpredictable spatiotemporal dynamics of the two governing factors: agricultural space/land and water coupled with farmers’ knowledge (Lairez et al., 2023; Abera, 2017). Spatiotemporal changes in grain production are primarily driven by two key farmers’ decisions: (i) shifts in land use or reallocation and (ii) the introduction of new CPS. As water and land are limited resources, increasing grain production should focus on efficiency, rather than unlimited expansion of inputs such as space and water (Nasrallah et al., 2020; Biswas et al., 2006; Zhao et al., 2021). Instead, land use decisions and CPS should focus on continuously improving grain production levels through effective land allocation and reallocation as well as enhancing the land utilization performance of farming systems (Liu J. et al., 2020; Berhanu et al., 2021). This can be achieved by co-designing appropriate land use plans and co-inventing efficient CPS, and making evidence-based land use decisions (Panigrahy et al., 2011). To realize a food-secure society in the basin, developing effective land use plans and high-performance CPS, effectively managing agricultural spaces and green water is crucial. These strategies require spatially explicit evidence of natural capital and proper understanding of the various CPS. Integration of such data can improve planning and decision-making through detailed assessments of land use performance (LUP) and land use efficiency (LUE). However, there is a lack of comprehensive studies assessing cultivated land dynamics and CPS at the required scale and level of detail. The first important move is generating multi-temporal cropland data, map and characterize existing CPS, and conduct in-depth assessments of rainfed grain production performance and its impacts on economic development, food security, and water security at the catchment scale.
Previous LUE studies have been performed from input–output perspective (Nasrallah et al., 2020; Silva et al., 2021). However, as grain production efficiency is largely governed by the inherent potential of the natural capital (space, water, climate, etc.) and the performance of the land use practice or efficacy of a particular CPS in response to inputs, studying the performance of CPS and cropping practices has paramount importance (Lairez et al., 2023; Sun et al., 2021; Biswas et al., 2006). Very recently, grain production research tended to focus on large-scale and multidimensional approaches to spatial econometric analysis, which allows analysis of the level of grain production, spatial distribution, and contribution of each production factor at the regional scale from an economic standpoint (Liu J. et al., 2020) or analysis of regional food security by measuring food production capacity and potential (Nkwasa et al., 2023). However, a common research gap is overlooking the incorporation of the space and time dimensions in the assessment, which, in turn, hinders a synoptic perspective of grain production dynamics.
Existing studies on LUE have two focuses: (i) single-use and (ii) combined-use system-oriented assessments. While the former ignores the synergy and trade-off that exist between several possible LUS, it emphasizes only on single and specific land use systems, for example, the cultivated land use system (Liu J. et al., 2020). Indeed, from a specific category of LUSs, various sub-categories can exist; for example, within a cultivated landscape, varying crop Production/cropping systems (CPS/CRS) exist. In that case, the performance assessment may require to single out specific practices, as residual soil moisture farming can be selected and assessed. However, still the former category of LUS performance and efficiency assessment approach disregards the interplay between CPS in a given landscape. The second category of performance assessment gives an equal focus for all existing land use systems, such as grain production, timber production, pasture production, and urban and settlement, which fulfills multidimensionality. It helps evaluate the performances of different LUS from the perspectives of resource utilization and strives to explore the relationship between the LUE of varying LUS and its link with socioeconomic development (Wang et al., 2022). According to Liu J. et al. (2020), such an approach allows to evaluation of the synergy and trade-off, while evaluating the performance and efficiency of LUS and/or CPSs. Nonetheless, previous studies mainly focused on the comparison of regional grain production differences but ignored the spatial interaction and impacts among land use sub-category performances/efficiencies and the vast variances in crop production within the same area. This necessitates the need to undertake a synoptic assessment of cropland change and CPS applying a multidimensional land use performance/efficiency assessment framework (Liu J. et al., 2020).
Recognizing the need to better understand the spatiotemporal trends in grain production, this study was conducted with the following specific objectives: (1) to map and assess the spatiotemporal dynamics of grain-producing landscapes of the UBNB; (2) to map and characterize major CPS targeting the spatiotemporal variation in grain production; and (3) to assess the performance and efficiency of rainfed-based CPS with special focus on Residual soil moisture Crop production System (RCS).
The study area is the Upper Blue Nile Basin (UBNB) commonly known as the Abbay River basin (Nkwasa et al., 2023; Roth et al., 2018). UBNB is part of the greater Nile River, covering only the Ethiopian part. It is located in the Northwestern part of Ethiopia’s highlands covering approximately 200,000 km2. The geographical location, extent, and basic spatial data about UBNB are presented in Figure 1. UBNB hosts approximately 79.6 million people (Teshome, 2014). It is the frontier area of Ethiopia’s water and agricultural development basin, with a national land area of 1.12% carrying a population of 5.78% and a total economic output of 10.22% due to its superior geographical location and mild climate. Nevertheless, the area also suffers from severe resource shortage, with a per capita arable land area of only 0.75 ha/hh, which is below the national (0.78 ha/HH). With rapid economic and social development, regional water development has a significant crowding effect on agriculture and ecological space (Wani et al., 2009). As with other basins in Ethiopia, UBNB has also faced development constraints such as intensified conflicts in water utilization and space utilization for competing land uses, degradation and reduction in cultivated land resources, environmental damage, loss of biodiversity, and widening development gaps between regions. More importantly, as a typical economically less developed area in Ethiopia, the UBNB basin serves as an excellent example of regional development for other areas in Eastern and Horn of Africa developing countries (Hurni et al., 2013). Particularly, it presents a typical case study for assessing land use sub-category efficiencies and coordinating conflicts based on food production, economic development, and ecological protection.
Figure 1. Geographic location of the study area (UBNB) with basic topographic and climatic information.
Several socioeconomic and biophysical data, with spatial and non-spatial nature, were collected from multi-sources and applying different data collection methods, such as (I) field visit/ground survey, (II) socioeconomic survey, (III) remote sensing, and (IV) other secondary sources.
Applying FGD and KII tools, basic socioeconomic data, such as total cropped land, productivity, production systems, cropping systems, and cropping calendar, were collected at the village/Kebele level. However, for some specific assessments, data collected at the village level remain scarce to obtain representative facts. Thus, for detailed assessments such as yield gap and food demand, comprehensive data were collected from systematically selected zones and districts found in the UBNB (CSA, 2020).
Fifteen-day composite NDVI (Normalized Difference Vegetation Index) products of two sensors, Sentinel 2A and MODIS satellite sensors, were important inputs for the analysis. Using Google Earth Engine (GEE), the bimonthly NDVI data, for the year 2015–2022, were systematically collected from October to May (Lebrini et al., 2021). The reasons to use only these periods are (i) due to cloud cover problems in the rest of the months of the year and (ii) the targeted CRSs, i.e., RSM and BRF cropping systems, are practiced only during these months of the year. Multitemporal NDVI data-based spectral profile was generated for each CPS taking representative sites in terms of AEZ, crop types, cropping practices, etc. (Liu L. et al., 2020; Peng, 2012). Using a fifth-order polynomial fitted model, the beginning and end dates of cropping cycles were computed, which were used to identify the spectral emergence and spectral maturity date (Cheng et al., 2023; Panigrahy et al., 2005). In order to compute the total duration (di) of a cropping system, 10 days was added at the beginning (15 days for rice crop) and 10 days at the end of each crop-growing period (Qiu et al., 2023). These days account for the duration of field preparation, the gap between sowing and spectral emergence, and the gap between spectral maturity and the harvest of the crop (Panigrahy et al., 2005).
Three categories of secondary-sourced geospatial data were used: (1) bio-climate; (2) edaphic, and (3) detailed LULC maps (Kassawmar et al., 2018a). In the former case, World Bio-Climatic data, containing approximately 19 determinant factors/variables, were used to identify CPS and cropping systems and further explore the link between RSM-based CPS and determinant factors and further predict the spatial distribution of the practice. Bioclimatic variables are sourced from the freely available WorldClim 1.4 database for scientific research purposes, as indicated in Supplementary Figure 1. Historical climate data, essential for assessing the impact of climate change, will be acquired from the Intergovernmental Panel on Climate Change Fifth Assessment Report (IPCC5 or CMIP5) at a spatial resolution of 30 s (1 km)1 from 1900 to 1990.
In the later case, detailed LULC data, produced by the lead-author was used and available at ethiogis-mapserver.org, https://www.ethiogis-mapserver.org. Detailed inforamtion is available at Kassawmar et al, 2018a and 2018b.
These datasets are presented as Supplementary Figure 2.
Various spatial and non-spatial first-hand data were collected through recursive field visits and extensive ground surveys on systematically selected villages. Data were collected from the ground, and secondary data were obtained from pertinent governmental offices at different levels. Eventually, a holistic database comprising various information about the biophysical and socioeconomic aspects of the different CPS and cropping practices was developed.
Mapping cropped pixels and clustering of CPS/CRS zones over large and complex landscapes characteristically depict smallholder farming, which is not trivial (Reckling et al., 2016; Liu J. et al., 2020; Yu et al., 2021; Kassawmar et al., 2018b). From clustering and a classification point of view, two categories of datasets were used: non-spectral and spectral datasets (Reckling et al., 2016). The present study used both multi-source and multi-scale spatial datasets to produce crop production systems zones (CPSZ), thereby mapping and characterizing cropping practices.
Specialists in the field suggest that applying non-spectral solutions out of image analysis techniques (Kassawmar et al., 2018a; Reckling et al., 2016) can help a lot to minimize the challenges. Non-spectral cluster analysis can be strategized based on several premises (Kassawmar et al., 2018a), one of which is stratifying the landscapes based on major characterizing features such as cropping system similarities (Kassawmar et al., 2018a). Given the characteristics of different CPS can be explained and described using non-spectral information that exists in various determinate factors, the world bioclimatic datasets, containing 19 layers, were used as an important dataset for stratification. Before using the World Bioclimatic datasets for clustering, spatial dependency and redundancy were tested by applying PCA. From the PCA result, five datasets, with a varying number of principal component images, were generated. From these generated PC images, it is evident that most of the information has been concentrated in the first three PC images as shown in Supplementary Figure 1.
This study used multi-temporal raw satellite imageries, transformed indices, and produced CPSZ and cropping practices (Kassawmar et al., 2018a). Unlike images containing raw reflectance values, multi-temporal but transformed images are effective in identifying and map complex CPS (Aziz et al., 2023; Cheng et al., 2023). Thus, to gain a couple of benefits, composite multi-temporal indices were produced: (1) simplification of the classification process, (2) enhancing the inherent potential of multi-temporal and multi-spectral imageries (Cheng et al., 2023). Therefore, appropriate MODIS image data collection was done in Google Earth engine and required MODIS data were composite NDVI images as an important input to create HCPZ and further identify and map cropping systems. The creation of multi-temporal and multi-spectral NDVI datasets was designed to accomplish three major objectives of the study: (1) producing HCPZ and (2) identifying, mapping, and assessing major cropping systems and practices (3) undertaking reliable land use and cropping system performance assessment. In the former case, important factors that govern CPS such as growing seasons, LGP and AEZ were considered while creating a composite NDVI map. In the latter case, the phenology of major crop types and cropping calendar for major cropping systems were considered. While downloading and producing composite NDVI data, phenology of crops was assumed to be the same in 10–15 time (Peng, 2012). From the available multi-temporal images within the defined 15 15-day phenology period, first, a maximum NDVI value was selected and one NDVI raster dataset was created. Each NDVI raster dataset represents one phonological stage of the dominant crop, for instance, planting or seeding. Then, a series of NDVI maps representing the full range of the crop phenology over a defined growing period (Crop calendar) were created. Later, the intra-annual multi-temporal NDVI raster dataset was staked and a composite NDVI raster dataset with two bands in a month and six bands in 3 months was created (Lebourgeois et al., 2017). This allowed us to capture the intra and inter-annual phenology dynamics, which can be used to classify farm fields managed under different CPSs (Rose and Adiku, 2001; Griffiths et al., 2019).
Mapping and delineating of CPS demand the identification of major crops and accordingly delineation of their growing regions/boundary through an iterative clustering process by which the Homogeneous Crop Production zones (HCPZ) can be created (Nath et al., 2022).
Proper delineation of CPS requires explicitly defining a specific type of CPS and practices targeted to map and characterize. The present study targeted to map and characterize only rainfed-based CPS and CEP.
A hybridized and multi-stage clustering approach was implemented. For this purpose, at the pre-processing stage, two categories of geodatabases containing non-spectral and spectral layers were separately created. Some of the datasets, included under the non-spectral category are World Bio-Climatic data, AEZ maps, administrative boundary-based crop production data, and other auxiliary spatial layers. On the other hand, LULC maps, cropped pixels, crop phenology, and vegetation indices are some of the datasets included under the spectral category. Eventually, one raster layer, containing about 25-factor maps or layers, was produced. Depending on the level of dependency, redundancy, and importance of the non-spectral factors, about five composite datasets, with varying bands (19, 17, 15, 10, and 5), were created using layers included under the non-spectral geodatabase.
Using the five composite datasets, random homogeneous spatial units (HSU) were separately generated by applying the ISODATA clustering algorithm. The generated HSUs were iteratively checked with our primary data collected to identify and describe the different types of CPS. Using this reference information and HSU generated by a specific composite dataset, the effect of inclusion or exclusion of a particular factor/layer in delineating CPS was evaluated. The generated smaller HSU polygons helped us know the appropriateness of a particular factor/layer. By cross-checking the boundary of the HSU with multi-temporal spectral behaviors of surface vegetation cover (such information available from the spectral datasets), generalization and grouping of smaller HSU maps helped us to cluster areas with similar growing seasons. A major growing season zone map was eventually produced by integrating several secondary geospatial data, such as AEZ, farming systems, livelihood zones, and land use systems zone maps (Vintrou et al., 2012; Grytnes and Vetaas, 2002).
Integrating various socioeconomic primary data, such as Village/Kebele level crop production data (polygonal level training sample dataset), FGD, KII, as well as ground-based physical observation data (point level training sample datasets), training datasets were developed. Accordingly, a sufficient, representative, and accurate training sample dataset was produced. Approximately 459 sample points, on average 30 samples from each growing season zone map, were systematically developed. Using the training sample dataset, spectral signatures for each GS and CPS were iteratively generated and checked. Approximately 30% of them were used for validation and the remaining 70% were used to train the clustering algorithms.
Through iterative execution of the above steps, the actual clustering of HCPZ was performed by producing a refined composite dataset developed by selectively taking important layers from both categories of the geodatabase (spectral and non-spectral). Two clustering approaches were tested; unsupervised and supervised. For the former case, we chose Random Forest and Support Vector Machine algorithms, and for the latter case, we took ISODATA and K-Means algorithms. Recursive evaluation of these clustering algorithms was performed at different scales; local, meso, and basin scales. The evaluation results confirmed that the ISODATA algorithm gave a better accuracy and good quality boundary of growing seasons and varying CPS zones. Thus, a final HCPS zone map was produced by applying an iterative unsupervised clustering approach on the multi-source composite dataset (spectral and non-spectral) by applying the ISODATA clustering algorithm. Finally, approximately 45 HCPS clusters were generated that could represent the complex rainfed CPS of the UBNB. Involving eight experts in the field, expert knowledge, coupled with the comprehensive ground survey, GPS, quantitative and qualitative socioeconomic survey as well as other secondary data, the ground reality representation of each cluster was systematically checked and verified. The HCPSZ map is provided as Supplementary Figure 3.
Using multi-source data, pertinent indicators useful for the identification, mapping, and performance assessment of cropping practices in each CPS were produced (Supplementary Table S1 and Figures 2,3). For simplification and generalization purposes, continuous quantitative values in each index were further classified and reclassified into qualitative values as such; very high, high, medium, low, and very low. Detailed information and maps of the input layers used for the assessment are presented in Figure 2.
Figure 2. Actual values of variables/indices used to assess the land utilization and production performance/efficiency of different CPS: CDI (A), AGDI (B), Natural capital for crop production (C); CLUI (D), MCI (E), AGDI (F), CII (G), RYI (H) and GGSI (I).
Figure 3. Actual values of variables/indices used to assess the implications of land utilization and production performance/efficiency of different CPS on food systems: food supply index (A), food demand (B), food gap (C), and food needy population (D).
As far as rainfed-based multiple (CPS/CRS) potential and performance assessment is concerned, available pertinent studies on land use systems efficiency have two main focuses: (i) single-use and (ii) combined use system-oriented assessments. While the former ignores the synergy and trade-offs exist between several possible LUS, it emphasizes only on single and specific land use systems, for example, the cultivated land use system (Lin and Hülsbergen, 2017; Yerseitova et al., 2018) and industrial land (Xie et al., 2018). Indeed, from a specific category of LUSs, various sub-categories can exist, for example, within a cultivated landscape, varying (CPS/CRS) exist. In that case, the performance assessment may require to single out specific practices, like RSM and/or BRF can be selected and assessed. However, still the former category of LUS performance and efficiency assessment approach disregards the interplay between CPS in a given landscape. The second category of performance assessment gives equal focus to all existing land use systems such as grain production, timber production, pasture production, and urban and settlement from a multidimensional perspective such as sustainability. The second category helps evaluate the performances of different LUS from the perspectives of resource utilization and strives to explore the relationship between the LUE of varying LUS and its link with socioeconomic development (Masini et al., 2018), urban growth (Halleux et al., 2012; John et al., 2019), environmental constraints (Saikku et al., 2017; Searchinger et al., 2020), and economic transformation (Guastella et al., 2017; Lu et al., 2018).
To evaluate the performances and efficiency of different CPSs, we applied multidimensional land use performance and efficiency evaluation frameworks widely used for similar purposes (Liu J. et al., 2020). According to Liu J. et al. (2020), a multidimensional land use and crop production performance/efficiency analysis has three major dimensions, namely: (1) food production, (2) economic development, and (3) ecological maintenance. After thoroughly assessing the assessment framework, we customized the framework because (i) Liu J. et al. (2020) demonstrated the framework by taking all types of land use systems and taking three important sustainability dimensions. Although they demonstrated the framework for general LUS evaluations, authors considered only crop production as important in the evaluation of the land use system element. Moreover, contextually adapting the framework, the present assessment was performed after systematically identifying seven evaluation categories/dimensions: (1) natural land capacity and Technical feasibility measures (it compares the natural capacity of the landscape and environmental/ecological suitability of the landscape for a particular LUS/CPS); (2) land utilization performance measures (it evaluates the performance of CPS/CRS in terms of utilizing the land resources or the natural capital/land quality to produce grain); (3) production performance measures (it evaluates the performance of a particular CPS/CRS in terms of the production of grain per unit of inputs mainly land, water and time against the inherent the inherent capacity); (4) economic development measures (it evaluates the economic development contribution of a particular LUS/CPS in a particular landscape in terms of economic value of the produced grain over a given period of time); and (5) ecological maintenance measures (it measures the balance between production service against the regulatory and supportive functions); and (6) implication measures (it measures the overall grain production performance of a particular LUS/CPS in reference to food security. (ii) Given the framework is very young, as it stands, obtaining the required data for all indicators used in all three dimensions is difficult, specifically, regional expectation, overhead room, land use efficiency, and unused potential evaluation, we focused only on the performance and efficiency evaluation parts of the framework. (iii) As presented in Liu J. et al. (2020), the framework is demonstrated using dimension by dimension and composite or overall performance and efficiency indicators. While testing the framework, authors found that the dimension-by-dimension approach of the framework could hide some important facts that could unveil the reasons for some land use trade-offs. Thus, in addition to dimension-by-dimension (combined/composite) evaluation, authors needed to implement the framework applying discrete/individual and categorical indicators level assessment. Contextually adapting the implementation procedure of the framework, the performance assessment was performed at three stages/levels: individual, categorical, and composite levels. The third stage/level performance assessment requires combination of all individual indices or categorical indices and create one new composite index. However, in order to avoid double counting, the evaluation process was performed categorically.
Before the evaluation process, the present study identified 15 pertinent indices widely used to evaluate the performance and effectiveness of 13 CPSs. Continuous pixel values of each indicator were produced using data generated from multi-sources. The continuous quantitative values in each index were further classified and reclassified into qualitative values, such as very high, high, medium, low, and very low. While creating the nominal indices, rescaling and reclassification were done by applying natural and geometric breaks algorithms, available in ESRI ArcGIS software. Depending on the context, manual breaks techniques were also applied. The assessment was performed in two modalities: categorical modality, i.e., dimension-by-dimension evaluation and overall modality, where the evaluation is performed by aggregating all the indicators in all dimensions. The former modality is useful as it allows us to easily link the evaluation or assessment indicator values with possible factors that determine the performance/efficiency of CPS. The assessment was performed in two phases: (I) actual grain production evaluation and (II) attainable or possible grain production.
where E_LUPdi denotes the existing grain production performance of a particular landscape managed under a specific CPS in the dith dimension; Iidi denotes specific indices used to measure the performance of the landscape in the dith dimension; WIidi denotes the weight given to the Iith indicator in the dith dimension; and n represents the number of dimensions considered in the evaluation.
where OE_LUPdi denotes the overall existing/actual grain production performance of a landscape managed under a specific CPS estimated considering all indicators in all dimensions, E_LUPdi denotes the existing land use performance of the landscape in the dith dimension (Equation 1), and Wdi is the weight given to the dith dimension.
where A_LUEdi denotes attainable grain production efficiency of a particular landscape managed under a specific CPS estimated considering a particular dith dimension. It reflects the degree to which an attainable cultivated land (space) and other natural capitals (soil and climate) are efficiently utilized to produce grain in the corresponding dith dimension; Iidi denotes specific indices used to measure the performance of the landscape; n represents the number of dimensions considered in the evaluation.
where OA_LUE denotes the overall attainable grain production efficiency of a particular landscape managed under a specific CPS estimated considering all dimensions. It reflects the overall degree to which an attainable cultivated land (space) and other natural capitals (soil, water, and climate) are efficiently utilized to produce grain; WIdi denotes the weight given to dith dimension.
Given several factors determine the performance of different CPS (Cano et al., 2023); applying a multidimensional performance assessment framework was critically important (Liu J. et al., 2020). To clearly identify the major bottleneck of cropping intensifications, individual measures/indices-based CPS performance assessment has paramount importance. However, discrete-level performance evaluation has shortfalls as some indices overestimate and others underestimate performances. To overcome these limitations and obtain the benefits of individual indicators-based performance assessment, contextually modifying the multidimensional assessment framework was needed. Keeping the concept of a multidimensional assessment framework, the performance assessment was performed at three stages with three levels: individual, categorical, and composite. The third stage/level performance assessment requires us to combine all individual indices or categorical indices and create one new composite index. This was done by applying spatial overlay techniques. The multidimensional performance approach was adopted from Liu J. et al. (2020). To create workable indices, usable at all stages, the original indices values were converted into common nominal values (1–5). While creating the nominal indices, rescaling and reclassification were done by applying natural and geometric breaks algorithms, available in ESRI ArcGIS software, were used. Depending on the context, manual breaks techniques were also applied.
To generalize the performance/efficiency level of the different CPS, a composite index approach was implemented (Abdollahzadeh et al., 2023), two generalization assumptions and/or options do exist (De Montis et al., 2021): (i) each of the selected factors has the same level of influence and (ii) each of the considered evaluation factors has different levels of influence. In the former case, linear arithmetic mean rule can be applied whereas a weighted average rule can be applied for the latter case. For the latter case, involving eight experts in the field, an assignment of weight to each factor was given applying AHP.
The performance of CPS has a profound linkage with ecosystem services (Bommarco et al., 2013). Studying CPS and assessing their performance has a vital role in understanding the implication on food systems (Amin et al., 2022). This study made a brief implication assessment of water and food ecosystem services although CPS implications go beyond these two ecosystem services. The overall research methodology and workflow is presented in (see the maps and descriptions presented in section 3.5) Figure 4.
The entire UBN basin (100%) is served by rainfed farming systems, but currently, only 39% is used for grain production, meaning the remaining 69% does not produce grain. Grains/food are produced using different systems practiced in the basin however, the present study identified and mapped about 12 major CPS. Of the major CPS identified and mapped, Meher-Only (3) accounts for approximately 26%, Meher-Residual-Dependable (7) represents (12%), Meher-Residual-Dependable (8) covers 12%, and Meher-Residual-Dependable (8) covers approximately 26%. Belg Synergy (6) covers approximately 10% of the basin area.
Figure 5A shows the distribution of the main CRSs that are mostly practiced in the UBNB. Details about the types of major crops grown in each CPS and their proportional coverage is presented in Figure 6 and more information also provided as supplementary figure (Supplementary Figure 2). According to the statistical summary of the coverage of major crops in each CPS, important cropping systems, such as.… Maize, Teff and Sorghum(20%), Finger Millet, Teff, and Wheat(11%) and Potato, Beans and Barely(10%), cover the majority of the landmass of the catchment area.
Figure 5. Major Crop Production Systems (CPS, A) and Cropping Systems (CRS, B) widely practiced in the basin and the respective statistical facts.
Figure 6. Multi-temporal cropped pixels map overlaid with major crop production systems (CPS) at different years: 1986 (A), 2000 (B), 2020 (C), and major cropland changes (D).
As shown in Figure 6 and Table 1, the cultivated landscape of the UBNB covers only 39% of the catchment area and the remaining 61% of the catchment area provides various ecosystem services managed under different LUSs. As shown in Table 1 and Figure 6, the cultural landscape of the UBNB is managed by various rainfed CPS, of which Meher-Only (3) represents the larger part of the basin (Table 1. Multi-temporal area coverage of cropped pixels in each CPSZ).
As shown in Figure 6 and Table 1, the basin experienced an average increase in grain area of 10% over the past four decades. However, the dynamics of cultural landscapes vary considerably over time and space. Between 1985 and 2005 the increase was modest at only 2%, while the period from 2005 to 2020 observed a significant increase in acreage (Supplementary Figure 2 and Supplementary Table 2). Figure 6 shows that there have been both gains and losses across grain production landscapes. Long-cultivated areas experienced slight losses, while recently plowed landscapes, particularly in flood plains and lower elevations, experienced significant increases in grain production area. Detailed multi-temporal information about various information on various LULC types is provided as supplementary figure (Supplementary Figure 3).
The analysis results show that accurate mapping of crop areas, cropping systems, (Figure 5, Supplementary Figure 2, crop types Supplementary Figure 2 and Supplementary Table 3) and relevant spatial factors related to grain production improves the understanding and characterization of the spatio-temporal variations in the performance of rainfed crop production systems (CPS) at the local scale. Although approximately 10 indices were used, for simplicity reason, outputs from individual indicators are not presented. Figures 7A–D show the results of this dimensional and overall performance evaluations outputs, respectively.
Figure 7. Categorical spatial variation in CPS performance: land utilization performance (A); production performance (B), economic development performance (C), and overall performance (D).
Figure 8. Temporal aspect of the overall grain production performance of CPS: overall grain production performance in 1985 (A); overall grain production performance in 2020 (C); overall change in grain production performance between 1985 and 2020 (top B); statistical summary for overall grain production performance (D).
As shown in Figure 7A (top left), about half of the cultural landscape of UBNB has moderate land use performance, while almost a third has low performance. Currently, approximately 25% of the catchment area has very good cultivated land use performance. When ranking crop production systems (CPS) by land use performance, Meher-Belg-Dependable (1) and Meher-Residual-Dependable (7) are at the top, with approximately 58 and 45% of their cultivated areas showing high and very high performance, respectively. Extended-Meher (3), Meher-Residual-Belg Synergy, and Meher-Belg Intermittent also perform well in the use of their cultural landscapes.
According to Figure 7B and Table 2, approximately 50% of the UBNB cultural landscape has moderate productivity and medium grain yield, while approximately 37% has high performance (above the national average). However, over 10% of the landscape is below the national average. When ranking crop production systems (CPS) by productivity and yield, Meher-Residual-Dependable (7) and Extended-Meher (3) perform excellently, with approximately 90 and 80% of their areas having high and very high performance, respectively. Other systems such as Meher-Belg-Dependable (1), Meher-Residual-Belg Synergy, and Meher-Only also show good productivity. As shown in Figure 7B, areas of high productivity are concentrated in the central, southern, and southwestern parts of the basin, particularly in the Lake Tana floodplain, central mid-elevation highlands (East and West Gojam), and the south upper Dedessa and lower Dhabus subbasins. In contrast, despite the possibility of double cropping, the eastern region struggles with low productivity during the Meher-Residual-Belg season, mainly due to soil acidity problems.
The economic performance of various crop production systems (CPS) is shown in Figure 2I and Supplementary Figure 4 with the average annual gross grain supply index (GGSI) for the basin estimated at approximately 10 billion quintals on 55,000–75,000 km2 of arable land. Based on average grain prices, this equates to approximately 1.2 trillion Birr in annual sales. The top performing CPS by grain volume are Meher-Only (23%), Meher-Residual-Dependable (21%), and Extended-Meher (16%). However, Meher-Residual-Dependable (27%), Meher-Only (19%), and Extended-Meher (15%) generate the highest revenue. This discrepancy arises from factors such as greater plant diversity (AADI) and the cultivation of more valuable crops in systems such as Meher-Residual (Figure 2, Supplementary Table 4, and Supplementary Figure 4). Economically, the central, southern, and southeastern parts of the basin perform better, while the northwestern, western, and eastern regions lag behind.
Single or category-based performance assessments do not capture the full picture of the reality on the ground and require an overall assessment that combines all indicators. Figure 7 shows the results of this comprehensive performance evaluation. The composite score, reflecting existing performances, highlights, highlights Meher-Residual-Dependable (7), Extended-Meher (3), and Meher-Residual-Belg-Synergy (6) as the best-performing CPS at 50, 33% and 31% respectively of their landscapes each have higher overall performance values. In contrast, the Short-Meher (4) CPS has poor overall performance. In areas with multiple CPS, such as Residual and Meher-Residual, overall performance values are above average due to higher values of the Cropping Land Utilization Index (CLUI) and the Cropping Intensity Index (CII).
CPS performance assessments often do not demonstrate the gap between existing and potential production levels for each system. Land use system efficiency (LUS) reflects this gap by comparing achievable versus actual performance. Figure 9 shows both categorical and overall efficiency for large CPS and shows that none exceeds 60% efficiency. This suggests that most of the CPS are not utilizing even half of its rainfed grain production potential. Although the individual performance metrics for RCS are higher than other CPSs, the efficiency metrics show minimal differences compared to them, indicating significant room for improvement across systems.
Figure 9. Individual and Categorical spatial variation in CPS efficiency: Land Utilization Efficiency (A); Production Efficiency (B), Economic development efficiency (C) and Overall efficiency (D).
The low individual and categorical efficiency scores in areas practicing RCS or MCS indicate a significant gap between potential and actual performance. This suggests that current management practices are inadequate and are preventing farmers from achieving their maximum production potential. In addition, crucial indicators such as regulatory services and ecological maintenance are missing from the overall efficiency assessment. The lower efficiency scores for RCS may be attributed to this weak evaluation approach as important factors such as soil health and the regulatory roles of each CPS were overlooked, impacting the perceived efficiency of RCS compared to others.
The production performance and efficiency indicators better reflect the impact of RSM and other MCS on grain production. As shown in Figures 9A–C, the land use efficiency indicators revealed little difference between different CPS, while the economic development indicators show different efficiency values. This discrepancy arises from two main factors: (1) economic indicators were generated by combining government data with our primary data and (2) the market values of crops grown under different CPS vary significantly (e.g., Grasspea vs. Teff). Furthermore, the weighting used in the assessment did not adequately take these important differences into account.
The overall efficiency of grain production was assessed using both the linear arithmetic mean and weighted average approaches, as shown in Figure 9D. The results show that the most efficient CPS are Meher-Belg-Dependable (1), Meher-Residual-Dependable (7), and Extended-Meher (3), with 45, 43, and 41% of their respective landscapes achieving overall efficiency scores above 40. In contrast, Short-Meher (4) showed poor efficiency across all time periods. The moderate overall efficiency of all CPS indicates significant untapped potential, suggesting that grain production could be increased by 40%.
The differences in efficiency between different CPS are obvious. To illustrate the spatiotemporal changes in overall effectiveness, overall efficiency maps for two points in time (1985 and 2020) were compared, as shown in Figure 10. The results show that no CPS achieved overall efficiency scores above 60.
In several areas of the basin where multiple CPS, such as Residual, Meher-Residual, and Meher-Residual-Belg, are practiced, overall efficiency values were below 30 in 1985 but rose above 50 by 2020. This indicates that the introduction and widespread implementation of RCS significantly improved the overall grain performance in the basin.
The spatiotemporal dynamics of CPS performance are evaluated using the overall gain and loss of cropped areas in each CPS zone. Figure 8 illustrates this variation, showing significant negative changes (green pixels) in the eastern and central high mountain regions, while positive changes (red pixels) are concentrated in the central and western flatlands and floodplains. There was a notable shift in grain production from the eastern and central parts of the basin to the southern and southwestern regions, with a trend of shifting production from higher to lower elevations. Among the CPS, the Meher-Residual-Dependable, Meher-Residual-Intermittent, Meher-Residual-Belg, and Meher-Belg systems have shown the greatest improvements.
Analyzing the food gap serves as a key indicator for evaluating the grain production performance and efficiency of CPS. Figure 11 and Table 3 show the relevant indicators used for this analysis at the grid level. According to the GGI, surplus grain production is rare. Given that the eastern, central, and southern parts of the UBNB are highly populated, directly comparing CPS with food supply and demand can be misleading (Figure 11).
Figure 11. Temporal aspect of the overall grain production efficiency of CPS: Overall grain production efficiency in 1985 (A); Overall grain production Efficiency in 2020 (C); Overall change in grain production efficiency between 1985 and2020 (top B); Statistical summary for overall grain production efficiency (D).
The assessment findings indicate that approximately 10% of the non-agrarian and 45% of the agrarian population in the UBNB meet their food demand through the current rainfed-based CPS. This means that approximately 60% of the total population—90% of non-agrarian and 55% of agrarian households—fulfill their subsistence food needs from other sources, such as livestock production and various agricultural and non-agricultural activities (Table 3).
In regions where smallholder farmers primarily practice double cropping, such as Meher-Residual, the percentage of food-insecure individuals is relatively lower. However, interpreting the data requires a deeper analysis due to the complex factors at play. For instance, long-term crop cultivation on hilly terrain in the eastern basin has led to significant unproductivity, with many areas abandoned for grain production. Additionally, this region is home to a large population that exceeds its natural capacity. Conversely, frequent crop failures in areas reliant on Belg and Residual-Only CPS lead farmers to abandon the main production season due to risks like frost, floods, and pests, prompting them to pursue alternative livelihoods. Overall, linking grain production directly to food insecurity necessitates detailed data and thorough investigation. In areas where Belg-only, Meher-Only, Residual-Belg, and Residual-only CPS are prevalent, there is a high level of food deficit and a significant number of food-insecure individuals. In contrast, Meher-Residual and Meher-Residual-Belg CPS demonstrate relatively low food deficits due to their superior production performance, resulting in surplus grain in areas practicing Meher-Residual. CPS that synergize with RSM show enhanced food production and security. The performance of these CPS improves with greater synergy between Meher and RSM, highlighting the substantial contribution of RSM-based CPS to the food system, in both gross grain supply and crop diversity.
Rainfed agriculture system of Ethiopia faces numerous challenges despite its significant potential (Oweis et al., 2007). Smallholder grain production is constrained by various biophysical and socioeconomic factors, including poorly designed policies (Wani et al., 2009; Ahadu, 2019). Rainfed farming often serves as a testing ground for unproven strategies (Ahadu, 2019; Pretty, 1999).
Official data in Ethiopia are limited to zone-level information, making it difficult to assess smallholder grain production systems. While agricultural areas have generally increased, including in UBNB, the trend is irregular (Muluneh, 2010). The annual increase in cropped area is minimal compared to the rapidly growing population (Hurni et al., 2005; Wondie et al., 2016; CSA, 2019). Available data, including government sources, have poor spatial and temporal coverage and problems with accuracy. This highlights the need for in-depth research to better understand rainfed farming systems (Asfaw et al., 2021; Pretty, 1999). This study achieved three key milestones: (1) producing accurate cropland data at the 30-m pixel level for the years 1985, 2005, and 2020; (2) identified and mapped complex, dynamic cropping systems; and (3) evaluates the performance and efficiency of these (Equations 1–4). The assessment found that the entire UBNB is theoretically suitable for rainfed farming systems, with an average annual minimum rainfall of over 800 mm (Samy et al., 2019), sufficient for various crop production systems (Hurni, 1998). However, only 39% of the UBNB is currently used for grain production, while 61% is used for other purposes. Several studies show that Ethiopia’s cereal crop productivity has grown significantly (Quddus et al., 2022; CSA, 2020; Belachew et al., 2022), although yields have varied over time and space. While some attribute this increase to expanded farmland (Belachew et al., 2022), others cite improved varieties, enhanced extension services, and increased fertilizer (Berhanu, et al., 2021). However, none of the previous studies provide spatial evidence to validate their findings. Inconsistencies in available data on grain production are mainly due to incorrect assumptions about arable land and yield (Berhanu, et al., 2021 and Silva et al., 2021). The authors emphasize the need to examine the causes of these inconsistencies—such as definitions, approaches, scope, and methods—in order to address the problems. A major argument against previous analysis is the misattribution of changes in food production area to grain volume mainly emanated from the lack of spatially related evidence. The undeniable fact is that changes in grain volume, apart from changes in arable land or production input, are due to two main causes: (i) land allocation strategies and land use shifts and (ii) changes in crop production system (CPS) or cropping systems. The former explains land use and land cover change (LULC) and the latter explains land use system (LUS) and land management changes (Korbu et al., 2020 and Reckling et al., 2016).
The study found that in some areas, despite a trivial change in net-cropped area (1985–2020, Tables 1 and 3), grain volume increased significantly (Figure 12 and Supplementary Table 2). This shows that grain production can rise due to farmers’ intensification strategies or changes in land allocation and use, even without expanding cropped areas (CSA, 2014, 2020). For instance, as land becomes depleted, farmers may shift grain production from less productive areas such as hillsides to more fertile areas such as floodplains (Table 3). In some regions, the amount of grain increased due to changes in land use systems (LUS). Even with the same farmland size, LUS or management changes increased production. As population pressure and land scarcity become more critical, more efficient farming systems are emerging (Table 3). The authors have investigated that two prominent transformations on LUS are responsible for such processes: (i) the flourishing of multiple cropping systems and (ii) the shift in farming systems from livestock to grain production (conversion of pasture to cropland, Figure 6 and Supplementary Table 2). A key example of both transformations is the residual soil moisture-based crop production system (RCS). It converts waterlogged areas into arable land and enables farmers to grow grain two times a year on black cotton soils, which was uncommon a few decades ago (Supplementary Table 2). While RCS currently plays a major role in grain production, its socio-economic and ecological impact has not been fully studied.
Figure 12. Implications of grain production performance/efficiency of CPS: existing food gap (A); existing food gap (B); existing food needy population at grid level (C) and long year food needy population at district level (D).
Such crop production systems (CPS) are not included in the Annual Agricultural Sample Survey (CSA, 2019), which only considers crops grown in the main rainy season (Meher). Without detailed data on (i) land use shifts and allocations and (ii) the types of CPS used by smallholder farmers, it is impossible to understand trends in grain production and link its impact on food security. To make evidence-based decisions to address food shortages, the authors suggest three categories of spatiotemporal information on grain production; (i) natural capital, population distribution; (ii) cropped area and productivity; and (iii) description of crop production systems (CPS) including the land use and crop types. LUS changes, such as some aspects, like crop selection and multiple cropping, have contributed more to grain production than to the expansion of arable land. However, the impact of land allocation and CPS changes varies over time and by region.
This study identified 11 major crop production systems (CPS), with Meher-Only single cropping covering the largest share (26%) of the UBNB, followed by Meher-Residual-Dependable (12%), Meher-Residual-Intermittent (12%), and Extended-Meher (10%). Short-Meher-Only accounts for a smaller portion (3%). Between 1985 and 2020, the spatiotemporal LULC change assessment revealed a 10% increase in cropland added to the food system. Attributing the 10% increase in cropland directly to grain volume in the UBNB is misleading. While a 10% cropped area increase over four decades (1985–2023) is minor compared to the doubling population every 20 years, it still has a significant overall impact on grain volume. Between 2005 and 2020, the cultivated area in the UBNB increased from 58,506 km2 (30%) to 75,679 km2 (38%). Recent years (after 2005) show larger temporal and spatial fluctuations in grain production than earlier periods (1985-2005) (CSA, 2020). Changes in the total cropped area vary by crop production system (CPS), with the Meher-Shifting cropping zone showing the largest increase, followed by the Meher-Residual-Intermittent and Meher-Residual-Dependable zones. The conversion of extensive black cotton soils in flood plains, such as those around Lake Tana, contributed significantly to this increase (Abera, 2017; Korbu et al., 2020; Debele and Deressa, 2016; Elias et al., 2022). Specifically, landscapes managed under Meher-Residual-Dependable CPS increased from 5% in 1985 to 8% in 2020 (Figure 10 and Table 3). In contrast, MCS showed a declining trend in high-elevation regions with minimal net change in Meher-Belg. The performance of different crop production systems (CPS) varies significantly in time and space Figure 10–Figure 13. An increase in the area under grain cultivation directly increases the efficiency of specific CPS. There have been significant improvements in the use of cultivated land, particularly in low-lying areas such as the Lake Tana floodplain. Conversely, some high-elevation regions, particularly in the eastern and central areas, experienced a decline in grain production landscapes, as seen in CPS in the Wet and Dry-Dega ecoregions such as Meher-Belg. The analysis results suggest that overall CPS in these high-altitude areas have revealed a poor performance (Figure 10).
Several factors influence the spatiotemporal dynamics of grain production, including location, altitude, population distribution, technology, and land management (Zerssa et al., 2021; Abdollahzadeh et al., 2023). Geographically, there has been a significant shift in grain production from the eastern and central parts of the basin to the southern and southwestern regions. Altitudinally, production has moved from high and mid-altitudes to lower ones. Unproductive landscapes, like the Lake Tana floodplain, have been transformed into surplus grain producers, while other areas, such as the Awi Zone, have become less productive (Desta et al., 2021). In contrast, high-altitude landscapes, which have significant potential for double cropping and improvement, are deteriorating over time (Zerssa et al., 2021). Furthermore, poorly designed strategies, such as providing food aid to rehabilitate land, have undermined the development of adaptive solutions to these challenges (Figure 10).
In the eastern and central high mountain areas, particularly in the Meher-Belg and Belg CPS, significant losses in grain production efficiency were observed (indicated by deep green pixels in Figure 9). Elevation comparisons show that although high and mid-elevation landscapes lost overall grain efficiency, lower-elevation and mid-elevation floodplain landscapes showed an improvement in production efficiency. Significant improvements in grain production efficiency occurred in the central and western parts of the basin, particularly in flat areas and floodplains (represented by deep red pixels in Figure 9). This transformation is largely due to the shift from no-cropping and Meher-Only systems to Meher-Residual and Meher-Belg CPS. Among the CPS, the most notable improvements were observed in the Meher-Residual-Dependable, Meher-Residual-Intermittent, Meher-Residual-Belg, and Meher-Belg systems (Figures 9–11).
The findings from the spatiotemporal dynamics assessment can inform planners and decision makers about the existing CPS and its both direct and indirect implications for water and food security (Zhao et al., 2018). Assuming a direct relationship between the expansion of cultivated area and the amount of food, the 10% increase in cultivated area contributed significantly to grain production. Since 1985, the total gross grain volume of the basin has increased by an average of 153 million quintals per year (Figure 13 and Table 3). While agricultural area increased by approximately 10% over the last four decades, the total amount of grains added to the food system is estimated at 17% (30 million quintals) (Supplementary Figure 4).
This estimate aligns closely with the National Agricultural Sample Survey (CSA, 2019), which reported an annual grain production of approximately 173 million quintals for all zones within the basin (CSA, 2019). However, such assessments can be misleading because they focus solely on yield differences over time and not on sustainable grain supplies, which directly reflect levels of food security. A better indicator is the maximum number of people supported by the additional acreage. Between 1985 and 2015, the population of the basin grew by approximately 12 million (from 6 to 19 million) (Teshome, 2014), suggesting that the new farmland could support approximately 6 million people. This raises the question of how to meet the food needs of the remaining population. One can speculate that other food sources such as multiple cropping, intensive agriculture, improved productivity, and food aid probably helped meet the food needs of the rest of the population.
The potential of the catchment for multiple cropping has steadily increased, but productivity faces challenges due to various biophysical and socioeconomic constraints, including climate change, pests and diseases, and lack of government support (e.g., credit, improved seeds, and pesticides) (Tekeste, 2021; Mekonen and Berlie, 2021). Efforts to realize the potential of double cropping in areas where RCS is used have been limited in addressing existing constraints. Given continued population growth and inadequate land management, opportunities for multiple cropping will continue to remain untapped (Nkwasa et al., 2023). Researchers emphasize that improving grain production requires targeted investments, including developing adaptive crop varieties, adopting advanced agricultural technologies, and implementing effective land management practices (Liu J. et al., 2020). In addition, the government should introduce various incentive mechanisms, such as improving road network, market infrastructure, value chains, input subsidies, and capacity building to encourage smallholder farmers to focus on double cropping systems (such as RCS), particularly Meher-Residual, Meher-Belg, and Meher-Residual-Belg CPS. In summary, the CPS assessment approach demonstrated in this study can be extended to national assessments. The results can help identify the challenges in grain production and enable planners and decision-makers to find effective and high-performing CPS at different levels. This will strengthen efforts to address food security challenges as strategies to improve the grain production system are based on accurate evidence (Korbu et al., 2020).
Agricultural growth strategy of Ethiopia faces significant challenges, with food security a major concern due to its large population. Despite the potential for efficiency of agricultural area of the UBNB, grain production performance is average or below average. Rapid population growth and competing land uses necessitate careful assessment of land use systems (LUS) and crop production systems (CPS). Evaluating spatiotemporal variations in grain production across different CPS is crucial for a country reliant on rainfed agriculture. To improve the performance and efficiency of crop production systems (CPS), four input-oriented strategies are commonly recommended: Space, natural capital, chemicals, and institutional and technology. Space Input: While adding more land for grain production is an option, the study found that suitable slopping and high-altitude areas are largely exhausted forintensive grain production. Natural Capital Inputs: Despite rich natural resources of Ethiopia, existing CPS performance is poor. Enhancing how grain is produced on current landscapes is crucial, along with maintaining and improving land quality to boost efficiency. Chemical Input: The limited use of agricultural inputs due to lack of finance, such fertilizers and pest control, hampers smallholder farmers’ productivity. However, without detailed data on land quality and crop needs, applying these inputs may not yield positive results. Further research is needed in this area. Institutional and Technological Inputs: Traditional CPS suffer from inadequate agricultural infrastructure and low technological adoption. Lack of attention on multiple cropping, absence of required institutional or fragile setup hindered to enhance grain production. To foster innovative CPS, the sector requires better financing and advanced facilities.
In an effort to realize the above strategies, four categories of contributions from the present study can be identified, namely:
1) Methodological contributions (context-assessment approach): Proper and comprehensive grain production assessment demands not only detailed and accurate spatial data but also a holistic and multidimensional assessment approach. The present study has demonstrated a multidimensional and holistic assessment approach to evaluate the grain production performance/efficiency of rainfed CPS practiced in UBNB (Equations 1–4). The assessment approach presented in this study could help to boost existing research dimensions and further promote the efficient utilization of agricultural space, green water, and land resources, in addition to providing a basis for improving food production efficiency.
2) Spatial and multi-temporal data contributions: The success of such comprehensive assessments depends on the generation of detailed input data at the required spatial, temporal, and thematic scales, including cropland area, crop types, cropping systems, and production determinants. This study successfully created several spatial datasets, creating a database for future research. However, due to limited resources, the authors failed to include some important indicators from the analysis such as yield of each crop at different years.
3) Scientific evidence and knowledge generation: Previous studies often lacked comprehensive national or basin-level performance assessments due to data limitations, limiting insight into crop production systems (CPS). Many attempts focused on single-factor analyses such as sown area or yield rather than comprehensive assessments. The analysis presented here not only uncovers current CPS characteristics and spatial variations in grain production but also improves understanding of CPS and their performance and efficiency. Furthermore, this framework provides a foundation for future research, with the data generated serving as valuable input for future studies.
4) Policy: The results of this study will benefit policymakers in four ways: (i) refining the basin-level agriculture-oriented economic development strategies, (ii) assisting planners and decision-makers in understanding rain-based crop production systems (CPS), (iii) identifying effective solutions to improve grain production efficiency for smallholder farmers who still practice traditional rainfed CPS, and (iv) informing food security policies and optimizing resource allocation in the agricultural sector.
The raw data supporting the conclusions of this article will be made available by the authors, without undue reservation.
TK: Conceptualization, Data curation, Formal analysis, Investigation, Methodology, Project administration, Resources, Software, Supervision, Validation, Visualization, Writing – original draft, Writing – review & editing. ET: Data curation, Investigation, Methodology, Supervision, Validation, Visualization, Writing – review & editing. ST: Data curation, Formal analysis, Methodology, Software, Writing – review & editing. WB: Investigation, Project administration, Supervision, Validation, Writing – review & editing. GZ: Funding acquisition, Investigation, Project administration, Resources, Supervision, Visualization, Writing – review & editing. LA: Data curation, Formal analysis, Investigation, Methodology, Software, Writing – review & editing. CW: Funding acquisition, Investigation, Project administration, Resources, Supervision, Validation, Visualization, Writing – review & editing. GO’D: Investigation, Project administration, Resources, Software, Supervision, Validation, Visualization, Writing – review & editing.
The author(s) declare that financial support was received for the research, authorship, and/or publication of this article. This research was supported by the Water Security and Sustainable Development Hub, which was funded by the UK Research and Innovation’s Global Challenges Research Fund (GCRF) (grant no. ES/S008179/1).
The authors express their sincere gratitude to the Water and Land Resource Centre of Addis Abeba University (WLRC-AAU) for the facilitation of the data collection and provision of other required resources to undertake this research.
The authors declare that the research was conducted in the absence of any commercial or financial relationships that could be construed as a potential conflict of interest.
All claims expressed in this article are solely those of the authors and do not necessarily represent those of their affiliated organizations, or those of the publisher, the editors and the reviewers. Any product that may be evaluated in this article, or claim that may be made by its manufacturer, is not guaranteed or endorsed by the publisher.
The Supplementary material for this article can be found online at: https://www.frontiersin.org/articles/10.3389/fsufs.2024.1420700/full#supplementary-material
Abdollahzadeh, G., Sharifzadeh, M. S., Sklenička, P., and Azadi, H. (2023). Adaptive capacity of farming systems to climate change in Iran: application of composite index approach. Agric. Syst. 204:103537. doi: 10.1016/j.agsy.2022.103537
Abera, M. (2017). Agriculture in the Lake Tana Sub-basin of Ethiopia. in Social and Ecological System Dynamics. AESS Interdisciplinary Environmental Studies and Sciences Series. Eds. Stave, K., Goshu, G., Aynalem, S. Springer, Cham.
Amin, E., Belda, S., Pipia, L., Szantoi, Z., El Baroudy, A., Moreno, J., et al. (2022). Multi-season phenology mapping of Nile Delta croplands using time series of Sentinel-2 and Landsat 8 green LAI. Remote Sens. 14:1812. doi: 10.3390/rs14081812
Asfaw, A., Bantider, A., Simane, B., and Hassen, A. (2021). Smallholder farmers’ livelihood vulnerability to climate change-induced hazards: agroecology-based comparative analysis in northcentral Ethiopia (Woleka Sub-Basin). Heliyon 7:e06761. doi: 10.1016/j.heliyon.2021.e06761
Aziz, M. A., Haldar, D., Danodia, A., and Chauhan, P. (2023). Use of time series Sentinel-1 and Sentinel-2 image for Rice crop inventory in parts of Bangladesh. Appl. Geomat. 15, 407–420. doi: 10.1007/s12518-023-00501-2
Belachew, K. Y., Maina, N. H., Dersseh, W. M., Zeleke, B., and Stoddard, F. L. (2022). Yield gaps of major cereal and grain legume crops in Ethiopia: a review. Agronomy 12:1–22. doi: 10.3390/agronomy12102528
Berhanu, Y., Angassa, A., and Aune, J. B. (2021). A system analysis to assess the effect of low-cost agricultural technologies on productivity, income and GHG emissions in mixed farming systems in southern Ethiopia. Agric. Syst. 187:102988. doi: 10.1016/j.agsy.2020.102988
Biswas, B., Ghosh, D. C., Dasgupta, M. K., Trivedi, N., Timsina, J., and Dobermann, A. (2006). Integrated assessment of cropping Systems in the Eastern Indo-Gangetic Plain. Field Crop Res. 99, 35–47. doi: 10.1016/j.fcr.2006.03.002
Bommarco, R., Kleijn, D., and Potts, S. G. (2013). Ecological intensification: harnessing ecosystem Services for Food Security. Trends Ecol. Evol. 28, 230–238. doi: 10.1016/j.tree.2012.10.012
Cano, P. B., Cabrini, S. M., Peper, A. M., and Poggio, S. L. (2023). Multi-criteria assessment of cropping Systems for the Sustainable Intensification in the pampas. Agric. Syst. 210:103723. doi: 10.1016/j.agsy.2023.103723
Cheng, G., Ding, H., Yang, J., and Cheng, Y. (2023). Crop type classification with combined spectral, texture, and radar features of time-series Sentinel-1 and Sentinel-2 data. Int. J. Remote Sens. 44, 1215–1237. doi: 10.1080/01431161.2023.2176723
CSA (2014). The federal democratic republic of Ethiopia central statistical report agency statistical. Eur. J. Clin. Pharmacol. 73:1–121.
CSA (2019). The Federal Democratic Republic of Ethiopia central statistical Agency agricultural sample survey. Rep Area Prod Major Crops 1:1–102.
CSA (2020). The Federal Democratic Republic of Ethiopia central statistical Agency volume vii report on (private peasant holdings, Meher season). Most 10:1–97.
De Montis, A., Serra, V., Calia, G., Trogu, D., and Ledda, A. (2021). To weight or not to weight, that is the question: the Design of a Composite Indicator of landscape fragmentation. Appl. Sci. 11:1–27. doi: 10.3390/app11073208
Debele, T., and Deressa, H. (2016). Integrated Management of Vertisols for crop production in Ethiopia: a review. J. Biol. 6:1–36.
Desta, G., Kassawmar, T., Tadesse, M., and Zeleke, G. (2021). Extent and distribution of surface soil acidity in the rainfed areas of Ethiopia. Land Degrad. Dev. 32, 5348–5359. doi: 10.1002/ldr.4113
Elias, E., Biratu, G. K., and Smaling, E. M. A. (2022). Vertisols in the Ethiopian highlands: interaction between land use systems, soil properties, and different types of fertilizer applied to Teff and wheat. Sustainability 14:1–13. doi: 10.3390/su14127370
Endalew, B., Muche, M., and Tadesse, S. (2015). Assessment of food security situation in Ethiopia: a review. Asian J. Agric. Res. 9:1–7. doi: 10.3923/ajar.2015.55.68
Griffiths, P., Nendel, C., and Hostert, P. (2019). Intra-annual reflectance composites from Sentinel-2 and Landsat for National-Scale Crop and land cover mapping. Remote Sens. Environ. 220, 135–151. doi: 10.1016/j.rse.2018.10.031
Grytnes, J. A., and Vetaas, O. R. (2002). Species richness and altitude: a comparison between null models vand interpolated plant species richness along the Himalayan altitudinal gradient, Nepal. Am. Nat. 159, 294–304. doi: 10.2307/3079080
Guastella, G., Pareglio, S., and Sckokai, P. (2017). “A spatial econometric analysis of land use efficiency in large and small municipalities.” Land Use Policy. 63:288–297.
Halleux, J-M., Szymon, M., and van der Krabben, E.. (2012). “The adaptive efficiency of land use planning measured by the control of urban sprawl. The cases of the Netherlands, Belgium and Poland.” Land Use Policy, 29: 887–898.
Hurni, H. (1998). “Agroecologial belts of Ethiopia: explanatory notes on three maps at a scale of 1:1,000,000” in Research Report, Soil Conservaton Research Program (Addis Ababa).
Hurni, H., Debele, B., Lemann, T., Roth, V., and Zeleke, G.. (2013). Impacts of watershed management on runoff in the eastern Nile tributaries. In New Nile Perspectives Conference. 213–217.
Hurni, H., Tato, K., and Zeleke, G. (2005). The implications of changes in population, land use, and land Management for Surface Runoff in the upper Nile Basin area of Ethiopia. Mt. Res. Dev. 25, 147–154. doi: 10.1659/0276-4741(2005)025[0147:TIOCIP]2.0.CO;2
John, B., Christopher, L., Lang, D. J., and von Wehrden, H. (2019). “Toward sustainable urban metabolisms. From system understanding to system transformation.” Ecological economics, 157: 402–414.
Kassawmar, T., Eckert, S., Hurni, K., Zeleke, G., and Hurni, H. (2018a). Reducing landscape heterogeneity for improved land use and land cover (LULC) classification across the large and complex Ethiopian highlands. Geocarto Int. 33, 53–69. doi: 10.1080/10106049.2016.1222637
Kassawmar, T., Zeleke, G., Bantider, A., Gessesse, G. D., and Abraha, L. (2018b). A synoptic land change assessment of Ethiopia’s Rainfed agricultural area for evidence-based agricultural ecosystem management. Heliyon 4:e00914. doi: 10.1016/j.heliyon.2018.e00914
Korbu, L., Tafes, B., Kassa, G., Mola, T., and Fikre, A. (2020). Unlocking the genetic potential of chickpea through improved crop management practices in Ethiopia: a review. Agron. Sustain. Dev. 40:1–15. doi: 10.1007/s13593-020-00618-3
Lairez, J., Affholder, F., Scopel, E., Leudpanhane, B., and Wery, J. (2023). Sustainability assessment of cropping systems: a field-based approach on family farms. Application to maize cultivation in Southeast Asia. Eur. J. Agron. 143:126716. doi: 10.1016/j.eja.2022.126716
Lebourgeois, V., Dupuy, S., Vintrou, É., Ameline, M., Butler, S., and Bégué, A. (2017). A combined random Forest and OBIA classification scheme for mapping smallholder agriculture at different nomenclature levels using multisource data (simulated Sentinel-2 time series, VHRS and DEM). Remote Sens. 9:259. doi: 10.3390/rs9030259
Lebrini, Y., Boudhar, A., Laamrani, A., Htitiou, A., Lionboui, H., Salhi, A., et al. (2021). Mapping and characterization of phenological changes over various farming systems in an arid and semi-arid region using multitemporal moderate spatial resolution data. Remote Sens. 13:578. doi: 10.3390/rs13040578
Lesur-Dumoulin, C., Laurent, A., Reau, R., Guichard, L., Ballot, R., Jeuffroy, M. H., et al. (2018). Co-design and ex ante assessment of cropping system prototypes including energy crops in eastern France. Biomass Bioenergy 116, 205–215. doi: 10.1016/j.biombioe.2018.06.013
Lin, H-C, and Hülsbergen, K.-J. (2017). “A new method for analyzing agricultural land-use efficiency, and its application in organic and conventional farming systems in southern Germany”. European Journal of Agronomy, 83, 15–27.
Lu,, Xin-hai,, and Shan-gan Ke,. (2018). “Evaluating the effectiveness of sustainable urban land use in China from the perspective of sustainable urbanization”. Habitat International, 77: 90–98.
Liu, J., Jin, X., Weiyi, X., Zhengming, G., Yang, X., Ren, J., et al. (2020). A new framework of land use efficiency for the coordination among food, economy and ecology in regional development. Sci. Total Environ. 710:135670. doi: 10.1016/j.scitotenv.2019.135670
Liu, L., Xiao, X., Qin, Y., Wang, J., Xinliang, X., Yueming, H., et al. (2020). Mapping cropping intensity in China using time series Landsat and Sentinel-2 images and Google earth engine. Remote Sens. Environ. 239:111624. doi: 10.1016/j.rse.2019.111624
Masini,, Emanuela,, Anna Barbati,, Massimiliano Bencardino,, Margherita Carlucci,, Piermaria Corona,, et al. (2018). “Paths to change: bio-economic factors, geographical gradients and the land-use structure of Italy”. Environmental management. 61: 116–131.
Mekonen, A. A., and Berlie, A. B. (2021). Rural households’ livelihood vulnerability to climate variability and extremes: a livelihood zone-based approach in the northeastern highlands of Ethiopia. Ecol. Process. 10:1–23. doi: 10.1186/s13717-021-00313-5
Muluneh, A. (2010). Synthesis of research on land use and land cover : UNU-Land Restoration Training Programme. 1–39.
Nasrallah, A., Belhouchette, H., Baghdadi, N., Mhawej, M., Darwish, T., Darwich, S., et al. (2020). Performance of wheat-based cropping systems and economic risk of low relative productivity assessment in a sub-dry Mediterranean environment. Eur. J. Agron. 113:125968. doi: 10.1016/j.eja.2019.125968
Nath, B., Hossain, M., and Majumder, S. C. (2022). Agricultural crop pattern mapping and change analysis at a Sub-District level using Landsat satellite data: evidence from south-eastern region, Bangladesh. J. Agric. Environ. Int. Dev. 116, 5–38. doi: 10.36253/jaeid-11961
Nkwasa, A., Waha, K., and van Griensven, A. (2023). Can the cropping Systems of the Nile Basin be Adapted to climate change? Reg. Environ. Chang. 23:9. doi: 10.1007/s10113-022-02008-9
Oweis, T. Y., Wani, S., Bruggeman, A., Farahani, J., Karlberg, L., and Zhu, Q. (2007). Unlocking the potential of rainfed agriculture: managing water in rainfed agriculture IWMI Part 4, Chapter 8–16.
Panigrahy, S., Manjunath, K. R., and Ray, S. S. (2005). Deriving cropping system performance indices using remote sensing data and GIS. Int. J. Remote Sens. 26, 2595–2606. doi: 10.1080/01431160500114698
Panigrahy, S., Ray, S. S., Manjunath, K. R., Pandey, P. S., Sharma, S. K., Sood, A., et al. (2011). A spatial database of cropping system and its characteristics to aid climate change impact assessment studies. J. Indian Soc. Remote Sens. 39, 355–364. doi: 10.1007/s12524-011-0093-3
Peng, D. (2012). Crop monitoring using a multiple cropping index based on multi-temporal MODIS data. Afr. J. Agric. Res. 7:3828–3838. doi: 10.5897/ajar11.2455
Pretty, J. (1999). Can sustainable agriculture feed Africa? New evidence on progress, processes and impacts. Environ. Dev. Sustain. 1, 3–4. doi: 10.1023/a:1010039224868
Qiu, B., Xiang, H., Yang, P., Tang, Z., Wenbin, W., and Li, Z. (2023). A robust approach for large-scale cropping intensity mapping in smallholder farms from vegetation, brownness indices and SAR time series. ISPRS J. Photogramm. Remote Sens. 203, 328–344. doi: 10.1016/j.isprsjprs.2023.08.007
Quddus, M., Abdul, M., Siddiky, A., Sarker, K. K., Rahman, M., Khan, M. A. H., et al. (2022). The assessment of four crop-based cropping system productivity, nutrient uptake and soil fertility with existing cropping systems. J. Agric. Sci. 14:206. doi: 10.5539/jas.v14n6p206
Rahmato, D. (2003). Resettlement in Ethiopia. The tragedy of population relocation in the 1980s. Forum Soc Stud 22:66–75.
Reckling, M., Hecker, J. M., Bergkvist, G., Watson, C. A., Zander, P., Schläfke, N., et al. (2016). A cropping system assessment framework—evaluating effects of introducing legumes into crop rotations. Eur. J. Agron. 76, 186–197. doi: 10.1016/j.eja.2015.11.005
Rose, C. W., and Adiku, S. (2001). Conceptual methodologies in agro-environmental systems. Soil Tillage Res. 58, 141–149. doi: 10.1016/S0167-1987(00)00164-1
Roth, V., Lemann, T., Zeleke, G., Subhatu, A. T., Nigussie, T. K., and Hurni, H. (2018). Effects of climate change on water resources in the upper Blue Nile Basin of Ethiopia. Heliyon 4:e00771. doi: 10.1016/j.heliyon.2018.e00771
Saikku, L., Laura,, and Mattila, T. J. (2017). “Drivers of land use efficiency and trade embodied biomass use of Finland 2000–2010.” Ecological Indicators, 77: 348–356.
Searchinger, T. D., Chris Malins,, Patrice Dumas,, Baldock,, Joe Glauber,, Thomas Jayne,, et al. (2020). “Revising public agricultural support to mitigate climate change ”.
Samy, A., Ibrahim, M. G., Mahmod, W. E., Fujii, M., Eltawil, A., and Daoud, W. (2019). Statistical assessment of rainfall characteristics in upper Blue Nile Basin over the period from 1953 to 2014. Water 11:1–17. doi: 10.3390/w11030468
Silva, J. V., Reidsma, P., Baudron, F., Jaleta, M., Tesfaye, K., and van Ittersum, M. K. (2021). Wheat yield gaps across smallholder farming systems in Ethiopia. Agron. Sustain. Dev. 41:1–16. doi: 10.1007/s13593-020-00654-z
Sun, T., Zhao, C., Feng, X., Yin, W., Gou, Z., Lal, R., et al. (2021). Maize-based intercropping systems achieve higher productivity and profitability with lesser environmental footprint in a water-scarce region of Northwest China. Food Energy Secur 10. doi: 10.1002/fes3.260
Taddese, G. (2001). Land degradation: a challenge to Ethiopia. Environ. Manag. 27, 815–824. doi: 10.1007/s002670010190
Tekeste, K. (2021). Climate-smart agricultural (CSA) practices and its implications to food security in Siyadebrina Wayu District, Ethiopia. Afr. J. Agric. Res. 17, 92–103. doi: 10.5897/ajar2020.15100
Teshome, M. (2014). Population growth and cultivated land in rural Ethiopia: land use dynamics, access, farm size, and fragmentation. Resourc. Environ. 4:148–161. doi: 10.5923/j.re.20140403.03
Vintrou, E., Desbrosse, A., Bégué, A., Traoré, S., Baron, C., and Seen, D. L. (2012). Crop area mapping in West Africa using landscape stratification of MODIS time series and comparison with existing global land products. Int. J. Appl. Earth Obs. Geoinf. 14, 83–93. doi: 10.1016/j.jag.2011.06.010
Wang, Y., Jin, C., Peng, Q., Liu, J., and Xiaohang, W. (2022). Systematic measurement and evolution situation of coupling coordination level between intensive cultivated land utilization and new-type urbanization. Sustainability 14:1–26. doi: 10.3390/su141811716
Wani, S. P., Rockström, J., and Oweis, T. (2009). Rainfed agriculture: unlocking the potential. Rainf. Agric. doi: 10.1079/9781845933890.0000
Wondie, M., Schneider, W., Katzensteiner, K., Mansberger, R., and Teketay, D. (2016). Modelling the dynamics of landscape transformations and population growth in the highlands of Ethiopia using remote-sensing data. Int. J. Remote Sens. 37, 5647–5667. doi: 10.1080/01431161.2016.1246773
Wu, B., Gommes, R., Zhang, M., Zeng, H., Yan, N., Zou, W., et al. (2015). Global crop monitoring: a satellite-based hierarchical approach. Remote Sens. 7, 3907–3933. doi: 10.3390/rs70403907
Xie, H., Qianru, C., Fucai, L., Qing, W., and Wei, W. (2018). “Spatial-temporal disparities, saving potential and influential factors of industrial land use efficiency: A case study in urban agglomeration in the middle reaches of the Yangtze River”. Land use Policy, 75: 518–529.
Yerseitova, A., Sara, I., Leila, J., Almarа, N., and Altynay, M. (2018). “Efficiency of using agricultural land in Kazakhstan”. Entrepreneurship and Sustainability, no. 2, 558–576.
Yu, Q., Xiang, M., Sun, Z., and Wenbin, W. (2021). The complexity of measuring cropland use intensity: an empirical study. Agric. Syst. 192:103180. doi: 10.1016/j.agsy.2021.103180
Zerssa, G., Feyssa, D., Kim, D. G., and Eichler-Löbermann, B. (2021). Challenges of smallholder farming in Ethiopia and opportunities by adopting climate-smart agriculture. Agriculture 11:1–25. doi: 10.3390/agriculture11030192
Zhao, Y., Feng, J., Luo, L., Bai, L., Wan, H., and Ren, H. (2021). Monitoring cropping intensity dynamics across the North China plain from 1982 to 2018 using glass Lai products. Remote Sens. 13:3911. doi: 10.3390/rs13193911
Keywords: residual farming, grain production, Blue Nile, water security, food security, soil moisture, Ethiopia, land use efficiency
Citation: Kassawmar T, Teferi E, Tsegaye S, Bewket W, Zeleke G, Abraha L, Walsh CL and O’Donnell G (2024) Spatiotemporal variation in grain production performance and efficiency of the cultivated landscapes in Upper Blue Nile Basin of Ethiopia: the impact of residual moisture-based farming on water and food security. Front. Sustain. Food Syst. 8:1420700. doi: 10.3389/fsufs.2024.1420700
Received: 20 April 2024; Accepted: 23 September 2024;
Published: 06 November 2024.
Edited by:
Tendai Polite Chibarabada, Zimbabwe Sugar Association Experiment Station, ZimbabweReviewed by:
Francis Oloo, Technical University of Kenya, KenyaCopyright © 2024 Kassawmar, Teferi, Tsegaye, Bewket, Zeleke, Abraha, Walsh and O’Donnell. This is an open-access article distributed under the terms of the Creative Commons Attribution License (CC BY). The use, distribution or reproduction in other forums is permitted, provided the original author(s) and the copyright owner(s) are credited and that the original publication in this journal is cited, in accordance with accepted academic practice. No use, distribution or reproduction is permitted which does not comply with these terms.
*Correspondence: Tibebu Kassawmar, dGliZWJ1LmtAd2xyYy1ldGgub3Jn; Claire L. Walsh, Y2xhaXJlLndhbHNoQG5jbC5hYy51aw==
Disclaimer: All claims expressed in this article are solely those of the authors and do not necessarily represent those of their affiliated organizations, or those of the publisher, the editors and the reviewers. Any product that may be evaluated in this article or claim that may be made by its manufacturer is not guaranteed or endorsed by the publisher.
Research integrity at Frontiers
Learn more about the work of our research integrity team to safeguard the quality of each article we publish.