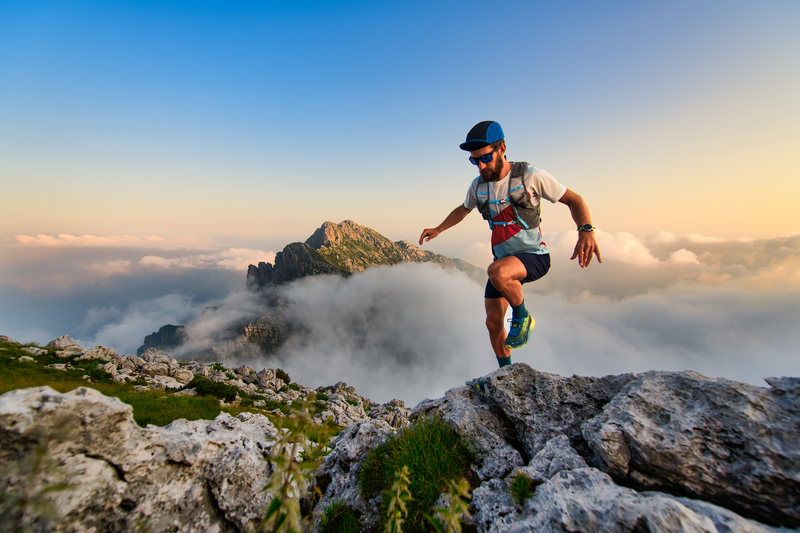
94% of researchers rate our articles as excellent or good
Learn more about the work of our research integrity team to safeguard the quality of each article we publish.
Find out more
ORIGINAL RESEARCH article
Front. Sustain. Food Syst. , 12 August 2024
Sec. Nutrition and Sustainable Diets
Volume 8 - 2024 | https://doi.org/10.3389/fsufs.2024.1417045
This article is part of the Research Topic Nutritional Aspects Of Sustainable Foods View all 8 articles
The study aimed to develop gluten-free Riceberry rice pasta supplemented with cricket powder using a twin-screw extruder, contributing valuable knowledge to increasing the nutritional value of pasta through sustainable practices, nutritional enhancement, and novel ingredients. The D-optimal mixture design, an effective statistical tool for optimizing formulation, was used to determine the optimal proportions of Riceberry rice flour (RF, 75–90%), cricket powder (CP, 5–20%), and xanthan gum (XG, 1–5%). The results showed that all predictive models were statistically significant with satisfactory coefficients of determination (R2 ≥ 0.75), and exhibited a non-significant lack of fit. The contour plot demonstrated that pasta with high RF, XG, and low CP contents had the lowest L* and highest a* values. The incorporation of XG increased firmness, while an increase in CP led to higher protein and fat contents in the pasta. A decrease in RF resulted in reduced total phenolic content (TPC), anthocyanin content (ATC), and 1,1-diphenyl-2-picrylhydrazyl radical (DPPH) scavenging activity (AOA). The sensory evaluation scores of the pasta, in terms of the texture attribute and overall acceptance, decreased as the proportion of XG increased. The optimization analysis showed that the highest desirability was achieved with RF, CP, and XG at 84.10, 14.45, and 1.45%, respectively. The optimally desirable product had the following properties: L* at 22.60, a* at 4.80, b* at 4.78, firmness at 3.41 N, protein content at 12.57%, fat content at 4.68%, TPC at 360.13 mg GAE/100 g dw, ATC at 476.24 mg C3G/100 g dw, AOA at 25.10 mg TE/100 g dw, texture rating at 7.40, and overall acceptance rating at 7.01. This study highlights the potential of cricket powder as a protein source in gluten-free Riceberry rice pasta. It offers insights into the effects of ingredients on pasta quality and facilitates the development of nutritious and appealing gluten-free pasta.
Celiac disease, commonly known as gluten allergy, is caused by gluten found in grains like wheat, barley, and rye, leading to malnutrition and digestive problems due to reduced nutritional absorption in the intestinal wall (O’Shea et al., 2014). Celiac disease affects 0.5–1% of the global population, and epidemiological studies indicate a rise in celiac cases (Gujral et al., 2012). To prevent falling ill, it is important to consume gluten-free foods. Therefore, the market demand for gluten-free products has continuously grown in the past decade. Pasta is a cereal-based product that contains two essential ingredients, including semolina flour and water, which play an important role in product quality (De Noni and Pagani, 2010). Numerous studies have been carried out to develop gluten-free flours by using other cereals and tubers as substitutes for gluten-free foods. However, the absence of gluten in food formulations poses a challenge to the production of gluten-free foods, especially regarding texture. Consequently, earlier researchers investigated several methods to enhance the quality of gluten-free pasta. One of these methods is the use of hydrocolloids such as xanthan gum (Milde et al., 2020), guar gum, and hydroxypropyl methylcellulose (Susanna and Prabhasankar, 2013). The extrusion process is among the most effective technologies for producing and enhancing the qualities of gluten-free pasta. This process integrates gelatinized starch into the protein matrix, resulting in pasta with characteristics similar to wheat-based pasta (Bouasla et al., 2017; Baah et al., 2022). However, pasta products made from wheat and rice are usually low in essential amino acids and bioactive compounds. Presently, consumers are concerned with the nutritional and functional properties of their food choices. Therefore, pasta products should be enriched with bioactive compounds and nutritional value.
Riceberry (Oryza sativa L.) is a unique variety of rice that is indigenous to Thailand. It has a distinct dark purple color, and is rich in phenolic compounds, anthocyanins (cyanidin-3-glucoside and peonidin-3-glucoside), vitamin E complex (tocopherols and tocotrienols), ß-carotene, γ-oryzanol, and folic acid. The bioactive compounds present in Riceberry rice flour display several health benefits, including antioxidant properties, cancer prevention, hyperglycemia improvement, amelioration of hyperlipidemia, and anti-inflammatory properties (Leardkamolkarn et al., 2011; Wiriyawattana et al., 2018; Anuyahong et al., 2020). Previous studies have examined the use of Riceberry rice flour as a gluten-free ingredient to enhance the nutritional value and bioactive compounds of food products such as bread (Thiranusornkij et al., 2019), noodles (Sirichokworrakit et al., 2015; Thongkaew and Singthong, 2020), and extruded snack (Piayura and Itthivadhanapong, 2023). Despite the numerous health benefits of Riceberry rice flour, there is currently no literature available on utilizing it for extruded pasta production.
Food fortification involves adding essential nutrients, such as vitamins and minerals, to improve their nutritional value or to address deficiencies (Zielińsk et al., 2021). Insects have traditionally been part of human diets, and current trends indicate that there has been a significant rise in studies investigating the use of insects in various food products. Insects are a rich source of essential vitamins (A, E, C, niacin, riboflavin, folic acid, and pyridoxine) and minerals (K, P, Ca, Fe, and Zn). Previous studies have explored the incorporation of insects, particularly protein, as an alternative source in various food products, including bread (Kowalczewski et al., 2021), pasta (Jakab et al., 2020), and extruded snacks (Igual et al., 2020). The house cricket (Acheta domestica L.), commonly found in Thailand, is frequently consumed by frying and is also commonly used as a food ingredient due to its high protein content (62%) and moderate fat content (8%) (Kowalski et al., 2022). One of the potential food applications for insects is pasta, a well-recognized and traditional cereal-based meal. However, there has not been much research on using rice-based pasta supplemented with edible insects to increase protein content. While some studies (Duda et al., 2019; Pasini et al., 2022) have used insect powders to add protein to pasta, more information is needed.
The optimization of experiments has the potential to enhance the various components and processing variables. Mixture design is a helpful optimization technique for investigating various combinations of food ingredients. This technique enables the examination of ingredient functions in processed food products, emphasizing the significance of component interactions (Amini Sarteshnizi et al., 2015). The D-optimal mixture experimental design is a statistical methodology used to investigate the different effects and interactions of components in a combination. This methodology is commonly used to determine the most effective combination of composite materials through fitted models in an efficient manner. Researchers often use this statistical methodology to investigate the effects and interactions of components within a combination. Fitting models using this methodology allows for the efficient determination of the most effective combination of composite materials (Mohamad Zen et al., 2015).
Cricket powder is gaining recognition as a sustainable protein source, and Riceberry rice is known for its antioxidants and health benefits. This research attempts to develop gluten-free pasta with high nutritional components that differ from those found in conventional wheat-based pasta. The novel aspect of this study is the innovative use of a mixture of cricket powder and Riceberry rice in the pasta formulation, as well as the addition of xanthan gum, to improve texture quality, which has not been studied before. Therefore, the purpose was to use a D-optimal mixture design to develop gluten-free Riceberry rice pasta supplemented with cricket powder, with independent variables of Riceberry rice flour (RF), cricket powder (CP), and xanthan gum (XG), and to examine their effects on the nutritional and functional characteristics of the pasta, determining the best optimal amounts of RF, CP, and XG. As a result, a gluten-free pasta product with an alternative protein source has been developed.
Organic Riceberry rice flour (RF), cricket powder (CP), and xanthan gum (XG) were used as ingredients in this study. The RF, CP, and XG were purchased from Gracebio Co., Ltd. (Thailand), Lallalit Agri Foods Co., Ltd. (Thailand), and Chemipan Corporation Co., Ltd. (Thailand), respectively. Xanthan gum can enhance dough strength as well as the cooking and sensory qualities of pasta (Milde et al., 2020). Therefore, xanthan gum was chosen to improve the texture quality of rice pasta.
An experimental design was developed using a D-optimal mixture design to determine the optimum proportions of pasta components (factors) on the response variables. The methodology enables the determination of the most optimal composition for a mixture while minimizing the total number of experimental runs. The Design-Expert (13.0) software (Stat-Ease, Minneapolis, United States) was used to determine the optimum proportions of the gluten-free Riceberry rice pasta supplemented with cricket powder formulation. The mixture of three components consisted of X1 (RF: 70–95%), X2 (CP: 5–20%), and X3 (XG: 1–5%); the high and low values of the components in the mixture are shown in Table 1. The component proportions were expressed as the true level (%) of the mixture. In a mixture experiment, the measured response depends only on the relative proportion of components present, usually 100% (Larrosa et al., 2013). The experimental design consisted of 13 experiments, comprising 10 different design points, 3 design points each with two replicates, and 5 lack of fit points (Table 2). The dependent variables were the physicochemical, functional, and sensory properties of the pasta. The 11 responses were determined, including L* (Y1), a* (Y2), b* (Y3), firmness (Y4), protein content (Y5), fat content (Y6), total phenolic content (Y7), anthocyanin content (Y8), DPPH radical scavenging activity (Y9), texture (Y10), and overall acceptance (Y11).
Table 2. Effects of mixtures composition in gluten-free Riceberry rice pasta supplemented with cricket powder on physicochemical, functional, and sensory properties of 13 pasta treatments.
The data were analyzed using the following methods: (1) coefficient of determination (R2), (2) adjusted coefficient of determination (adjusted R2), (3) predicted residual sum of squares (Predicted R2) to measure the variation explained by the components and their interactions, and (4) p-value, (5) F-value, and (6) the lack of fit test to assess the adequacy of the model, providing an independent estimate of experimental error variance derived from repeated experimental runs. Multiple regression analysis was employed to fit the following models (Equations 1–3):
where Y is the predicted dependent variable; β is the equation coefficients; X is the proportions of components (Saeleaw and Schleining, 2010).
Based on the best-fitted model, an equation is created through statistical analysis. The equation shows that positive coefficients affect the response variable positively and negative coefficients negatively (Mohamad Zen et al., 2015). Additionally, surface contour plots were obtained (resulting from the interaction of variables in a model) based on the generated regression models (adjusted R2 ≥ 0.75) for a better explanation.
The optimal formulation of the mixture ratio was carried out by including the components RF, CP, and XG in a range of values, which was intended to provide pasta that exhibits desirable protein content, total phenolic content, anthocyanin content, antioxidant activity measured by DPPH scavenging radical activity, and sensory (texture and overall acceptance) properties. Finally, the optimal mixture ratio with the highest desirability was performed to locate the response surface regions, and the dependent variables were predicted. The graphical optimization was performed by overlaying contour plots to analyze the relationship between composition levels and various responses (Sahu and Patel, 2021).
The pasta was produced using a pilot scale co-rotating intermeshing twin-screw extruder (CTE-D22L32 model, Chareun Tut Co. Ltd., Thailand). The screw had a diameter of 24.70 mm and a length-to-diameter ratio of 32:1. The extruder barrel consists of six jacketed zones. Throughout the experiment, the temperature profile in the first through sixth zones was maintained at 40, 70, 120, 120, 70, and 50°C, respectively, following a previous study report by Detchewa et al. (2016) with some modifications. The extrusion was done with a die diameter of 1.0 mm and a die temperature of 50°C. The mixed flour was fed into the extrusion barrel at the initial zone of the barrel at an average rate of 8 kg/h, with a feed moisture content of 28%. The extruder drove a 3.7 kW motor driving at 120 rpm. The extruded pasta was cut into 25 cm lengths and dried in a tray dryer at 50°C for 90 min until the moisture content was less than 13%. All samples were stored in aluminum foil pouches at ambient temperature until further analysis.
The color parameters (L*, a*, and b* values) were measured with a Datacolor colorimeter (200 M model, Datacolor, NJ, United States). The samples were randomly arranged into groups and placed upright to fill a glass measurement container. Color readings were taken from three different points. Each sample was determined in triplicates.
The samples were cooked for 10 min in boiling distilled water. Firmness (N) was measured by a TA7 blade from a general probe kit using a texture analyzer (CT3™, AMETEK Brookfield, United States). The test speed was 1 mm/s, with 80% of the initial thickness and a load cell of 5 kg.
The protein and fat contents of the pasta were determined according to the AOAC method (AOAC, Official Methods of Analysis, 2002). Nitrogen content was analyzed using the Kjeldahl method, and protein content was estimated using a nitrogen-protein conversion factor of 6.25 (method 954.01). The fat content was determined by a hexane extraction using the Soxhlet method (method 920.39).
Total phenolic content (TPC), anthocyanin content (ATC), and antioxidant activity (AOA) measured by 1,1-diphenyl-2-picrylhydrazyl radical (DPPH) scavenging assay, were described by Mala et al. (2024) with some modifications. Two g of ground pasta was extracted with 20 mL of 70% methanol, stirred for 3 h, and then centrifuged at 3,000 xg for 10 min. The supernatant was filtered using a syringe filter (0.45 μm). The extracts were measured for TPC, ATC, and AOA using a double-beam spectrometer (Libra S70, Biochrom, United Kingdom). The results of TPC, ATC, and AOA were expressed as milligram of gallic acid equivalent per 100 g dry weight of sample (mg GAE/100 g dw), milligram of cyanidin-3-glucoside equivalent per 100 g dry weight of sample (mg C3G/100 g dw), and milligram of Trolox equivalent per 100 g dry weight of sample (mg TE/100 g dw), respectively.
The samples were arranged in Balanced Incomplete Block Design (BIB) experiments (t = 13, k = 4, r = 4, b = 13, and λ = 1). This means there were 13 different pasta treatments (t = 13), each panelist tested 4 treatments per block (k = 4), each treatment was replicated 4 times (r = 4), there were 13 different groups of panelists (b = 13), and each pair of treatments appeared together in the same block exactly once (λ = 1). Each panelist tested only 4 of the 13 treatments to ensure a balanced and manageable evaluation process. This design helps to minimize bias and fatigue, ensuring reliable feedback on each pasta treatment. The 13 pasta treatments were tested by 104 untrained panelists with a 9-point hedonic scale. The panelists were instructed to evaluate sensory attributes, including texture and overall acceptance. Ethical approval for human subjects was granted by the Sakon Nakhon Rajabhat University Research Ethics Committee (COA No. 006/2566, IEC No. HE 64–060) on June 16, 2021.
All statistical analysis, mixture design, generation of response surfaces, desirability functional analysis, and optimization were accomplished using Design-Expert (13.0) software (Stat-Ease, Minneapolis, United States), significant at p < 0.05.
The results of physicochemical, functional, and sensory properties for all 13 treatments are shown in Table 2. The responses to the developed regression models were statistically significant, with a high coefficient of determination for the models. The data for the dependent variables were simultaneously fitted to linear, quadratic, and special cubic models (Table 3). ANOVA was used to find the significant terms of the response variable, which are shown in Table 3. According to ANOVA summary statistics, the fitted model for each response was statistically significant, including the sequential p-value, coefficient of determination (R2), adjusted R2, predicted R2, p-value, F-value, and lack of fit. The statistics suggested that the linear models were the best fit for b* value, firmness, protein content, TPC, ATC, AOA, texture, and overall acceptance, whereas the quadratic models for L* value, fat content, and special cubic model for a* value. Also, the statistical analysis created an equation based on the best-fitted model, as shown in Table 4. Based on the results, all variables showed a non-significant lack of fit, indicating a good correspondence between the measured data and the second-order polynomial models.
Table 3. Model fitting for each analytical response (physicochemical, functional, and sensory properties) and the obtained statistical data when applying ANOVA of lack of fit and regression of the selected models.
Table 4. Models of predictive regression of the physiochemical, functional, and sensory properties of the pasta.
The L*, a*, and b* values of the 13 pasta treatments ranged from 20.03–28.65, 3.22–7.69, and 4.23–7.92, respectively, as shown in Table 2. The natural anthocyanin pigments in Riceberry rice imparted the characteristic dark purple hue, resulting in a darker and more intense red color in pasta formulations with a higher proportion of RF. The contour plot showed that pasta with high RF, high XG, and low CP contents had low L* values (Figure 1A) and high a* values (Figure 1B). The anthocyanin content in RF imparted a purple-red color, while XG likely maintained color stability during processing. In contrast, when CP added in lower amounts, its impact on color was limited compared to RF and XG, resulting in the observed color characteristics. Pasta formulations with low RF and high CP and XG contents exhibited a brighter color due to the pale-yellow hue of CP. Several factors influenced the color of pasta, including the type of flour used and adding other ingredients. Moreover, the complex interactions between these ingredients significantly affected the final color of the product. Previous studies reported that adding XG to pasta formulations resulted in a brighter color (Milde et al., 2020). In contrast, incorporating hydrocolloids such as sodium carboxymethyl cellulose (0.5 and 1.0%) into wheat noodle formulations decreased L*, a*, and b* values (Choy et al., 2012). As shown in Figure 1C, the increase in RF and the decrease in CP led to a reduction in the b* value, emphasizing the significant influence of RF in providing a blue hue due to its anthocyanin content. Meanwhile, the pale-yellow color of CP decreased at lower concentrations.
Figure 1. The contour diagram shows the relationship between three variables, including RF, CP, and XG, on the color of pasta: (A) L*, (B) a*, and (C) b* values. The red dots on the contour plot and response surface represent the location of experimental runs in the experimental design space.
In addition, the ANOVA results for L*, a*, and b* values, presented in Table 3, showed model F-values of 0.16, 0.83, and 1.54, respectively. These values suggested the models were statistically significant (p ≤ 0.05) and did not exhibit a significant lack of fit. The statistical significance confirmed that the models could validly predict the color attributes of the pasta. Moreover, the high coefficients of determination (R2) for L* (0.91), a* (0.98), and b* (0.82) confirmed the accuracy of these models. The adjusted R2 values for L*, a*, and b* were higher than 0.75, demonstrating a best fit between the model terms and the observed data. These high R2 values indicated that the models explained a substantial proportion of the variability in the color measurements. The predictive regression equations for L*, a*, and b*, which are shown in Table 4, provided the mathematical relationships between the color values and the proportions of RF, CP, and XG. The equations were helpful in determining the effect of component differences on pasta color characteristics. Overall, the statistical analysis confirmed that the models were both significant and reliable, providing valuable insights into the effects of RF, CP, and XG on the color attributes of gluten-free rice pasta.
Firmness values observed for all treatments varied within the range of 2.31–9.93 N (Table 2). The treatment 13 exhibited the highest level of firmness, which contained 5.0% of XG. Generally, gluten-free pasta products without wheat flour necessitate the addition of other ingredients to improve texture. XG is a hydrocolloid with gelling abilities, a combination of XG and CP that may create covalent bonds with high gel strength (Hua et al., 2003). However, more XG additions caused an increase in firmness, as demonstrated by the contour plot depicted in Figure 2. This finding supports the hypothesis that XG can produce a structure that imitates the elastic texture of gluten, thereby enhancing the firmness of the pasta. Larrosa et al. (2013) reported that gluten-free pasta without XG in the combination caused the pasta to break during boiling because the dough lacks the cohesive structure provided by gluten and needs binding agents to form a gel-like substance when mixed with water. Similar results were observed for gluten-free cassava and corn flour-based pasta; increasing amounts of XG increased firmness and cutting force values (Milde et al., 2020). Furthermore, the high temperature of the extrusion process could increase the pasta firmness, resulting in a strong starch network and higher fracture strength values than low-temperature extrusion. The texture of the pasta produced by the extrusion process also depends on the raw materials in the mixture (Igual et al., 2020).
Figure 2. The contour diagram shows the relationship between three variables, including RF, CP, and XG, on the firmness of pasta. The red dots on the contour plot and response surface represent the location of experimental runs in the experimental design space.
In addition, RF and CP did not exhibit the same effects on firmness. RF, derived from a non-glutenous variety of rice, lacks the gluten proteins necessary to create the strong, elastic structure typical of traditional pasta, resulting in a less firm texture (Bouasla and Wójtowicz, 2021). While CP contributes to the nutritional composition with a higher protein content, it lacks the ability to combine effectively with other components of the pasta dough to enhance firmness. The protein structure of CP differed from gluten, and its water absorption and retention capabilities were not as effective in improving textural properties. Therefore, although RF and CP offer functional and nutritional properties, their potential to increase pasta firmness is limited.
The ANOVA analysis showed a significant F-value of 0.86 (p ≤ 0.0001) for the firmness model, which demonstrated its statistical significance. The model showed a high coefficient of determination (R2 = 0.97), indicating that the linear model effectively fit the data under the studied conditions. The high R2 value showed that the model explained 97% of the variability in firmness measurements, proving its validity. Additionally, the high adjusted R2 value of 0.96 showed strong agreement between the predicted and actual experimental firmness data, further validating the accuracy of the model predictions. The non-significant lack of fit confirmed that the model adequately described the data without significant error. The linear regression equation for firmness, shown in Table 4, demonstrated the mathematical relationship between the independent variables and the firmness of pasta.
Protein and fat contents of 13 pasta treatments ranged from 10.18–14.57% and 4.06–6.85%, respectively, as shown in Table 2. Figures 3A,B shows the relationship between nutritional compositions and the component variables. As expected, increasing the amount of CP increased the protein. In particular, treatment 5, which contained 20% CP, exhibited the highest protein content among all the treatments. This is consistent with earlier research (Duda et al., 2019) that has shown that adding alternative protein sources, such as insect proteins, can improve the nutritional composition of food products. According to Kowalski et al. (2022), CP comprises 2.46% moisture, 62.51% protein, 3.39% ash, 8.37% fat, and 9.48% dietary fiber. These components significantly enhance the nutritional value of pasta by providing essential nutrients and improving its functional properties. Another study by Kurdi et al. (2021) reported that CP has a high protein content of approximately 60 g/100 g, indicating that the protein content of pasta increases as the CP content rises. Moreover, previous research has demonstrated that insect flour significantly increases the protein content of extruded corn snacks (Igual et al., 2020) and wheat bread (Kowalski et al., 2022). Similarly, Duda et al. (2019) found that adding 15% CP to durum wheat pasta improved the product properties and increased the amount of protein and fat from 9.96–16.92% and 1.31–4.73%, respectively.
Figure 3. The contour diagram shows the relationship between three variables, including RF, CP, and XG, on the protein content (A) and fat content (B) of pasta. The red dots on the contour plot and response surface represent the location of experimental runs in the experimental design space.
In this study, the results also show that an increase in CP also contributed to a higher fat content in the pasta. Crickets and other edible insects are known for their relatively high fat content, primarily consisting of beneficial unsaturated fatty acids (Payne et al., 2016). Thus, the addition of CP not only increased protein content but also enhanced lipids, potentially offering additional health benefits. Moreover, the plots (Figures 3A,B) demonstrated that mixtures with higher RF content have lower protein and fat content in pasta compared to those with higher CP content. This is because RF is primarily carbohydrate-based with low protein and fat content, whereas insects are rich in these nutrients. Thiranusornkij et al. (2019) reported that RF contains 7.64% protein and 3.02% fat. As the proportion of rice flour increased, it diluted the protein and fat contributed by the insects, resulting in a lower overall protein and fat content. XG had less effect on protein and fat content because it was used in small amounts (1–5%) in the experiment. Generally, XG primarily acts as a stabilizer and thickener, enhancing the consistency and mouthfeel of food products (Yang and Xiang, 2022).
The statistical analysis of protein and fat contents, as presented in Table 3, indicated that the models were highly adequate. The F-values of 1.87 for protein content and 5.93 for fat content demonstrated high significance (p ≤ 0.001), indicating that the models were statistically significant. Additionally, the models showed no significant lack of fit, which further confirms their reliability. The high R2 values also indicated the best fit of the models to the observed data. The equations accurately predicted protein and fat contents, delivering satisfactory R2 values. The high R2 values showed that the models explained a substantial proportion of the variability in protein and fat contents, demonstrating their effectiveness in predicting these nutritional parameters (Table 4). These results revealed the reliability of the statistical models used in this study, ensuring accurate predictions of protein and fat contents in the pasta formulations. The significant findings validated the models and enhanced the importance of using reliable statistical methods.
According to the findings, the TPC, ATC, and AOA in pasta products can vary depending on the amount of RF used in the formulation. The values of the TPC, ATC, and AOA of all pastas ranged from 261.80–450.11 mg GAE/100 g dw, 321.12–585.60 mg C3G/100 g dw, and 21.66–28.19 mg TE/100 g dw, as shown in Table 2. The contour diagrams in Figures 4A–C show that increasing the proportion of RF increased TPC, ATC, and AOA in pasta. This trend likely resulted from the high anthocyanin and phenolic content intrinsic to Riceberry rice, which are well-known for their antioxidant properties (Ghasemzadeh et al., 2018; Kongthitilerd et al., 2020). The incorporation of higher amounts of RF enhanced the nutritional composition of the pasta and contributed to its functional properties by increasing its antioxidant capacity, which was beneficial for reducing oxidative stress and promoting overall health (Settapramote et al., 2021; Muangchan et al., 2022). According to Piayura and Itthivadhanapong (2023), reducing the amount of RF in the extruded snack formulation resulted in a reduction in TPC and ATC. Thus, the proportion of RF highly influences the TPC, ATC, and AOA of the pasta, as found in this study.
Figure 4. The contour diagram shows the relationship between three variables, including RF, CP, and XG, on the (A) TPC, (B) ATC, and (C) AOA of pasta. The red dots on the contour plot and response surface represent the location of experimental runs in the experimental design space.
Additionally, CP positively influences the bioactive compounds and antioxidant activity of pasta products. Several studies indicated that CP significantly enhanced antioxidant properties due to its high content of bioactive compounds. The incorporation of CP into food matrices significantly enhanced TPC and antioxidant capacity (Osimani et al., 2018; Pauter et al., 2018; Gumul et al., 2023; Suga et al., 2023). XG did not affect the TPC, ATC, and AOA because it is primarily a thickening agent with no significant bioactive compounds responsible for functional properties.
However, this study did not investigate extrusion temperature on the pasta properties, but previous studies reported that the extrusion process affects the functional properties of the product. Under extrusion temperature processing at 100°C or above significantly reduced TPC, ATC, and AOA in black rice (Ti et al., 2015) and brown rice (Zhang et al., 2018) flour-based products. In this study, the third and fourth zones of the extruder maintained a temperature of 120°C in the pasta production, which may cause a reduction in AOA due to the TPC and ATC deteriorating at high temperatures, which decreased scavenging-free radicals. A previous study on brown rice extrudates showed significantly lower antioxidant activity than unextruded brown rice flour, attributed to the significantly reduced free radicals caused by the high temperature of the extrusion process (Chalermchaiwat et al., 2015). The extrusion temperature plays an important role in the extrusion process. Further studies could explore the impact of varying extrusion temperatures within the range used in this study (e.g., 100°C to 120°C) on the functional properties of pasta.
The statistical analysis results for TPC, ATC, and AOA indicated that these responses best fitted a linear model. The regression data revealed F-values of 0.64, 2.77, and 3.42 for TPC, ATC, and AOA, respectively, suggesting that the linear model was the most suitable with a significance level of p ≤ 0.0001. Notably, there was no significant lack of fit, indicating that the model accurately described the data. Additionally, the R2 were notably high, with values of 0.85, 0.96, and 0.91 for TPC, ATC, and AOA, respectively, as presented in Table 3. These high R2 values demonstrated the strong predictive capability of the model and its ability to explain a substantial proportion of the variability in the data. The linear regression models derived from the best-fitted model for the TPC, ATC, and AOA equations are shown in Table 4, emphasizing the strength and reliability of the linear models in predicting these responses.
The recommended daily intake of anthocyanin extract may differ based on gender, age, weight, and health status (Yamuangmorn and Prom-U-Thai, 2021). Consuming natural food sources rich in anthocyanins offers health benefits, and these foods are generally safe and inexpensive compared to anthocyanin extracts. Therefore, the results indicated that Riceberry rice pasta, with high levels of polyphenolic compounds and anthocyanins demonstrating significant antioxidant activity, becomes an advantageous choice for anthocyanin intake. This is particularly notable when used to produce Riceberry rice pasta for many consumers worldwide.
According to the results shown in Table 2, the pasta formulated with Treatment 5 containing a proportion of 79% RF, 20% CP, and 1% XG had the highest scores for texture. Treatment 4, containing a proportion of 90% RF, 9% CP, and 1% XG, had the highest scores for overall acceptance. The contour plots in Figures 5A,B demonstrate that the sensory evaluation scores of pasta in terms of the texture attribute and overall acceptance decreased as the proportion of XG increased. Additionally, the findings suggest that RF and CP had a small impact on texture and overall acceptance, potentially due to the dominant effect of XG. As previously discussed, XG primarily acts as a stabilizer and thickener, improving the texture and structural integrity of the final product. RF, consisting mainly of carbohydrates, and CP, which contains fat and protein, play a role in nutrition and structural integrity. However, XG had a greater impact on texture and overall acceptability.
Figure 5. The contour diagram shows the relationship between three variables, including RF, CP, and XG, on the (A) texture attribute and (B) overall acceptance of pasta. The red dots on the contour plot and response surface represent the location of experimental runs in the experimental design space.
A previous study investigated the effect of XG on the sensory properties of glutinous rice-based noodles and reported that the addition of XG (5%) increased overall acceptance scores due to the improvement of hardness and chewiness (Cai et al., 2016). Moreover, a study investigating the effect of varying amounts of XG (1, 2, and 3%) on the sensory properties of horse chestnut flour-based noodles found that the inclusion of 3% XG was acceptable to the panelists (Rafiq et al., 2016). As a result, the critical factor influencing the sensory evaluation scores was the XG content. Therefore, it is important to use XG or other hydrocolloids in the proper amount to achieve the desired texture and stability without compromising the sensory quality of the final product.
The ANOVA analysis for texture and overall acceptance scores provided F-values of 1.22 and 3.19, respectively, both of which indicated statistical significance (p ≤ 0.001). This significance suggested that a linear model was appropriate for describing the relationships. The R2 for overall acceptance was 0.86, which was considered adequate for prediction purposes (Table 3). The linear regression models derived from the best-fitted model for the texture and overall acceptance equations are presented in Table 4, emphasizing the strength and reliability of these linear models in predicting the sensory evaluation responses.
In the formulation of equations to predict the content of RF, CP, and XG in pasta, the values of the factors were derived from the coefficient analysis of the regression equation for the physicochemical, functional, and sensory properties of the pasta products. The R2 was used to quantify the percentage change in the dependent variable that the independent variable could explain in the regression equation. It was considered to assess the appropriateness of the equation, with a preference for values where p ≤ 0.05 indicated statistical significance. The predictive regression models and contour plots were used to investigate the relationships between the RF, CP, and XG proportions of the pasta. Finally, optimization was performed to locate the response surface regions with the highest desirability (Venkatesan et al., 2019).
The overlay plot in Figure 6 illustrates the range within which the optimum conditions for a desirable-quality pasta product were located. The results indicated values at the central point of the mixture model design, and the shaded area in yellow signified the range of ingredients where the product exhibited the most desirable characteristics. The model was considered an acceptable predictor for the mixture of the pasta product. Specifically, it was observed that a mixture of 84.10% RF, 14.45% CP, and 1.45% XG resulted in optimized product desirability. The respective optimized response values of L*, a*, b*, firmness, protein, fat, TPC, ATC, AOA, texture, and overall acceptance were 22.60, 4.80, 4.78, 3.41 N, 12.57, 4.68%, 360.13 mg GAE/100 g dw, 476.24 mg C3G/100 g dw, 25.10 mg TE/100 g dw, 7.40, and 7.01, respectively (Table 5).
Figure 6. Optimized region obtained of contour plots for the effect of RF, CP, and XG on physicochemical, functional, and sensory properties of the pasta. The red dots on the contour plot and response surface represent the location of experimental runs in the experimental design space.
Table 5. Optimization criteria and formulation for the gluten-free Riceberry rice pasta supplemented with cricket powder.
The results demonstrate that the D-optimal mixture design was a successful and effective tool for optimizing gluten-free Riceberry rice pasta products enriched with a protein source from cricket powder. The experimental results indicated a significant influence of the pasta components on their physicochemical, functional, and sensory properties. The addition of CP increased both protein and fat contents. Additionally, higher levels of RF resulted in significant increases in TPC, ATC, and AOA. However, the sensory evaluation score of the pasta decreased with an increase in the proportion of XG. The D-optimal mixture design revealed optimal RF, CP, and XG values at 84.10, 14.45, and 1.45%, respectively. Thus, optimizing the production of gluten-free pasta by varying RF, CP, and XG using the D-optimal mixture design not only enhances its nutritional and functional properties but also maintains satisfactory sensory attributes. This study provides valuable knowledge for developing gluten-free pasta products, guiding the innovative use of cricket powder as an alternative protein source. Future research could explore the scalability of this formulation and its acceptance in consumer markets.
The original contributions presented in the study are included in the article/supplementary material, further inquiries can be directed to the corresponding author/s.
The studies involving humans were approved by the Sakon Nakhon Rajabhat University Research Ethics Committee, COA No. 006/2566, IEC No. HE 64–060, granted ethical approval for the use of human subjects in this study on June 16, 2021. The studies were conducted in accordance with the local legislation and institutional requirements. The participants provided their written informed consent to participate in this study.
JM: Conceptualization, Formal analysis, Data curation, Methodology, Validation, Writing – original draft, Writing – review & editing. CK: Conceptualization, Formal analysis, Data curation, Methodology, Validation, Writing – original draft, Writing – review & editing. PI: Conceptualization, Data curation, Methodology, Project administration, Supervision, Validation, Visualization, Writing – original draft, Writing – review & editing. TM: Conceptualization, Formal analysis, Data curation, Methodology, Writing – original draft. PH: Conceptualization, Data curation, Methodology, Validation, Writing – original draft. SP: Conceptualization, Formal analysis, Funding acquisition, Methodology, Project administration, Supervision, Validation, Visualization, Writing – original draft, Writing – review & editing, Data curation, Resources.
The author(s) declare that financial support was received for the research, authorship, and/or publication of this article. This study was supported by Research and Development Institute (RDI), Sakon Nakhon Rajabhat University, under grant number 808/256.
The authors thank to Department of Food Technology Laboratory, Sakon Nakhon Rajabhat University for the use of their facility and equipment for the preparation of samples and experiments.
The authors declare that the research was conducted in the absence of any commercial or financial relationships that could be construed as a potential conflict of interest.
All claims expressed in this article are solely those of the authors and do not necessarily represent those of their affiliated organizations, or those of the publisher, the editors and the reviewers. Any product that may be evaluated in this article, or claim that may be made by its manufacturer, is not guaranteed or endorsed by the publisher.
Amini Sarteshnizi, R., Hosseini, H., Bondarianzadeh, D., Colmenero, F. J., and Khaksar, R. (2015). Optimization of prebiotic sausage formulation: effect of using β-glucan and resistant starch by D-optimal mixture design approach. LWT 62, 704–710. doi: 10.1016/j.lwt.2014.05.014
Anuyahong, T., Chusak, C., and Adisakwattana, S. (2020). Incorporation of anthocyanin-rich riceberry rice in yogurts: effect on physicochemical properties, antioxidant activity and in vitro gastrointestinal digestion. LWT 129:109571. doi: 10.1016/j.lwt.2020.109571
AOAC, Official Methods of Analysis (2002). Association of Official Analytical Chemists, vol. 2. 17th Edn. Gaithersburg, MD: AOAC, Official Methods of Analysis.
Baah, R. O., Duodu, K. G., and Emmambux, M. N. (2022). Cooking quality, nutritional and antioxidant properties of gluten-free maize-orange-fleshed sweet potato pasta produced by extrusion. LWT 162:113415. doi: 10.1016/j.lwt.2022.113415
Bouasla, A., and Wójtowicz, A. (2021). Gluten-free rice instant pasta: effect of extrusion-cooking parameters on selected quality attributes and microstructure. PRO 9:693. doi: 10.3390/pr9040693
Bouasla, A., Wójtowicz, A., and Zidoune, M. N. (2017). Gluten-free precooked rice pasta enriched with legumes flours: physical properties, texture, sensory attributes and microstructure. LWT 75, 569–577. doi: 10.1016/j.lwt.2016.10.005
Cai, J., Chiang, C. H., Tan, M. Y. P., Saw, L. K., Xu, Y., and Ngan-Loong, M. N. (2016). Physicochemical properties of hydrothermally treated glutinous rice flour and xanthan gum mixture and its application in gluten-free noodles. J. Food Eng. 186, 1–9. doi: 10.1016/j.jfoodeng.2016.03.033
Chalermchaiwat, P., Jangchud, K., Jangchud, A., Charunuch, A., and Prinyawiwatkul, W. (2015). Antioxidant activity, free gamma-aminobutyric acid content, selected physical properties and consumer acceptance of germinated brown rice extrudates as affected by extrusion process. LWT 64, 490–496. doi: 10.1016/j.lwt.2015.04.066
Choy, A. L., May, B. K., and Small, D. M. (2012). The effects of acetylated potato starch and sodium carboxymethyl cellulose on the quality of instant fried noodles. Food Hydrocoll. 26, 2–8. doi: 10.1016/j.foodhyd.2011.02.001
De Noni, I., and Pagani, M. A. (2010). Cooking properties and heat damage of dried pasta as influenced by raw material characteristics and processing conditions. Crit. Rev. Food Sci. Nutr. 50, 465–472. doi: 10.1080/10408390802437154
Detchewa, P., Thongngam, M., Jane, J., and Naivikul, O. (2016). Preparation of gluten-free rice spaghetti with soy protein isolate using twin-screw extrusion. J. Food Sci. Technol. 3, 3485–3494. doi: 10.1007/s13197-016-2323-8
Duda, A., Adamczak, J., Chełmińska, P., Juszkiewicz, J., and Kowalczewski, P. (2019). Quality and nutritional/textural properties of durum wheat pasta enriched with cricket powder. Food Secur. 8, 46–56. doi: 10.3390/foods8020046
Ghasemzadeh, A., Karbalaii, M. T., Jaafar, H. Z. E., and Rahmat, A. (2018). Phytochemical constituents, antioxidant activity, and antiproliferative properties of black, red, and brown rice bran. Chem. Cent. J. 12:17. doi: 10.1186/s13065-018-0382-9
Gujral, N., Freeman, H. J., and Thomson, A. B. R. (2012). Celiac disease: prevalence, diagnosis, pathogenesis and treatment. World J. Gastroenterol. 18, 6036–6059. doi: 10.3748/wjg.v18.i42.6036
Gumul, D., Oracz, J., Kowalski, S., Mikulec, A., Skotnicka, M., Karwowska, K., et al. (2023). Bioactive compounds and antioxidant composition of nut bars with addition of various edible insect flours. Molecules 28:3556. doi: 10.3390/molecules28083556
Hua, Y., Cui, S. W., and Wang, Q. (2003). Gelling property of soy protein-gum mixtures. Food Hydrocoll. 17, 889–894. doi: 10.1016/S0268-005X(03)00110-3
Igual, M., Segovia, P. G., and Monzo, J. M. (2020). Effect of Acheta domesticus (house cricket) addition on protein content, colour, texture, and extrusion parameters of extruded products. J. Food Eng. 282:110032. doi: 10.1016/j.jfoodeng.2020.110032
Jakab, I., Tormási, J., Dhaygude, V., Mednyánszky, Z., Sipos, L., and Szedljak, I. (2020). Cricket flour-laden millet flour blends’ physical and chemical composition and adaptation in dried pasta products. Acta Aliment. 49, 4–12. doi: 10.1556/066.2020.49.1.2
Kongthitilerd, P., Suantawee, T., Cheng, H., Thilavech, T., Marnpae, M., and Adisakwattana, S. (2020). Anthocyanin-enriched Riceberry rice extract inhibits cell proliferation and adipogenesis in 3T3-L1 preadipocytes by downregulating adipogenic transcription factors and their targeting tenes. Nutrients 12:2480. doi: 10.3390/nu12082480
Kowalczewski, P. L., Gumienna, M., Rybicka, I., Górna, B., Sarbak, P., Dziedzic, K., et al. (2021). Nutritional value and biological activity of gluten-free bread enriched with cricket powder. Molecules 26:1184. doi: 10.3390/molecules26041184
Kowalski, S., Mikulec, A., Mickowska, B., Skotnicka, M., and Mazurek, A. (2022). Wheat bread supplementation with various edible insect flours. Influence of chemical composition on nutritional and technological aspects. LWT 159:113220. doi: 10.1016/j.lwt.2022.113220
Kurdi, P., Chaowiwat, P., and Hansawasdi, C. (2021). Studies on microbial quality, protein yield, and antioxidant properties of some frozen edible insects. Int. J. Food Sci. 2021:5580976. doi: 10.1155/2021/5580976
Larrosa, V., Lorenzo, G., Zaritzky, N., and Califano, A. (2013). Optimization of rheological properties of gluten-free pasta dough using mixture design. J. Cereal Sci. 53, 520–526. doi: 10.1016/j.jcs.2013.03.003
Leardkamolkarn, V., Thongthep, W., Suttiarporn, P., Kongkachuichai, R., Wongpornchai, S., and Wanavijitr, A. (2011). Chemopreventive properties of the bran extracted from a newly-developed Thai rice: the Riceberry. Food Chem. 125, 985–978. doi: 10.1016/j.foodchem.2010.09.093
Mala, T., Piayura, S., and Itthivadhanapong, P. (2024). Characterization of dried pineapple (Ananas comosus L.) peel powder and its application as a novel functional food ingredient in cracker product. Future Foods 9:100322. doi: 10.1016/j.fufo.2024.100322
Milde, L. B., Chigal, P. S., Olivera, J. E., and González, K. G. (2020). Incorporation of xanthan gum to gluten-free pasta with cassava starch. Physical, textural and sensory attributes. LWT 131:109674. doi: 10.1016/j.lwt.2020.109674
Mohamad Zen, N., Abd Gani, S. S., Shamsudin, R., and Fard Masoumi, H. R. (2015). The use of D-optimal mixture design in optimizing development of Okara tablet formulation as a dietary supplement. Sci. World J. 2015:684319. doi: 10.1155/2015/684319
Muangchan, N., Khiewvan, B., Chatree, S., Pongwattanapakin, K., Kunlaket, N., Dokmai, T., et al. (2022). Riceberry rice (Oryza sativa L.) slows gastric emptying and improves the postprandial glycaemic response. Br. J. Nutr. 128, 424–432. doi: 10.1017/s0007114521003494
O’Shea, N., Arendt, E., and Gallagher, E. (2014). State of the art in gluten-free research. J. Food Sci. 79, 1067–1076. doi: 10.1111/1750-3841.12479
Osimani, A., Milanović, V., Cardinali, F., Roncolini, A., Garofalo, C., Clementi, C., et al. (2018). Bread enriched with cricket powder (Acheta domesticus): a technological, microbiological and nutritional evaluation. Innov. Food Sci. Emerg. Technol. 48, 150–163. doi: 10.1016/j.ifset.2018.06.007
Pasini, G., Cullere, M., Vegro, M., Simonato, B., and Dalle Zotte, A. (2022). Potentiality of protein fractions from the house cricket (Acheta domesticus) and yellow mealworm (Tenebrio molitor) for pasta formulation. LWT 164:113220. doi: 10.1016/j.lwt.2022.113638
Pauter, P., Różańska, M., Wiza, P., Dworczak, S., Grobelna, N., Sarbak, P., et al. (2018). Effects of the replacement of wheat flour with cricket powder on the characteristics of muffins. Acta Sci. Pol. Technol. Aliment. 17, 227–233. doi: 10.17306/j.Afs.0570
Payne, C. L. R., Scarborough, P., Rayner, M., and Nonaka, K. (2016). A systematic review of nutrient composition data available for twelve commercially available edible insects, and comparison with reference values. Trends Food Sci. Technol. 47, 69–77. doi: 10.1016/j.tifs.2015.10.012
Piayura, S., and Itthivadhanapong, P. (2023). The effects of feed moisture and dried coconut meal content on the physicochemical, functional, and sensory properties of gluten-free Riceberry rice flour-based extruded snacks. Front. Sustain. Food Syst. 7:1194594. doi: 10.3389/fsufs.2023.1194594
Rafiq, S. I., Rafiq, S. M., and Saxena, D. C. (2016). Effect of hydrocolloids on the quality evaluation of flour based noodles from horse chestnut. MATEC Web Conf. 57:04005. doi: 10.1051/matecconf/20165704005
Saeleaw, M., and Schleining, G. (2010). Effect of blending cassava starch, rice, waxy rice and wheat flour on physico-chemical properties of flour mixtures and mechanical and sound emission properties of cassava crackers. J. Food Eng. 100, 12–24. doi: 10.1016/j.jfoodeng.2010.03.020
Sahu, C., and Patel, S. (2021). Optimization of maize–millet based soy fortified composite flour for preparation of RTE extruded products using D-optimal mixture design. J. Food Sci. Technol. 58, 2651–2660. doi: 10.1007/s13197-020-04771-1
Settapramote, N., Utama-Ang, N., Petiwathayakorn, T., Settakorn, K., Svasti, S., Srichairatanakool, S., et al. (2021). Antioxidant effects of anthocyanin-rich Riceberry™ rice flour prepared using dielectric barrier discharge plasma technology on iron-induced oxidative stress in mice. Molecules 26:4978. doi: 10.3390/molecules26164978
Sirichokworrakit, S., Phetkhuta, J., and Khommoona, A. (2015). Effect of partial substitution of wheat flour with riceberry flour on quality of noodles. Procedia. Soc. Behav. Sci. 197, 1006–1012. doi: 10.1016/j.sbspro.2015.07.294
Suga, N., Tsumura, E., Naito, Y., Hamaguchi, I., Matsuda, S., Kawabata, K., et al. (2023). Thermal stability of cricket powder and its effects on antioxidant activity, physical, and sensory properties of rice crackers. LWT 186:115267. doi: 10.1016/j.lwt.2023.115267
Susanna, S., and Prabhasankar, P. (2013). A study on development of gluten-free pasta and its biochemical and immunological validation. LWT 50, 613–621. doi: 10.1016/j.lwt.2012.07.040
Thiranusornkij, L., Thamnarathip, P., Chandrachai, A., Kuakpetoon, D., and Adisakwattana, S. (2019). Comparative studies on physicochemical properties, starch hydrolysis, predicted glycemic index of Hom Mali rice and Riceberry rice flour and their applications in bread. Food Chem. 283, 224–231. doi: 10.1016/j.foodchem.2019.01.048
Thongkaew, C., and Singthong, J. (2020). Effect of partial substitution of riceberry rice flour on rice noodles quality. Food Res. 4, 9–16. doi: 10.26656/fr.2017.4(S4).002
Ti, H., Zhang, R., Zhang, M., Wei, Z., Chi, J., Deng, Y., et al. (2015). Effect of extrusion on phytochemical profiles in milled fractions of black rice. Food Chem. 178, 186–194. doi: 10.1016/j.foodchem.2015.01.087
Venkatesan, M., Zaib, Q., Shah, I. H., and Park, H. S. (2019). Optimum utilization of waste foundry sand and fly ash for geopolymer concrete synthesis using D-optimal mixture design of experiments. Resour. Conserv. Recycl. 148, 114–123. doi: 10.1016/j.resconrec.2019.05.008
Wiriyawattana, P., Suwonsichon, S., and Suwonsichon, T. (2018). Effects of drum drying on physical and antioxidant properties of riceberry flour. Agric. Nat. Resour. 52, 445–450. doi: 10.1016/j.anres.2018.11.008
Yamuangmorn, S., and Prom-U-Thai, C. (2021). The potential of high-anthocyanin purple rice as a functional ingredient in human health. Antioxidants 24:833. doi: 10.3390/antiox10060833
Yang, Y., and Xiang, D. (2022). Effect of coconut protein and xanthan gum, soybean polysaccharide and gelatin interactions in oil-water interface. Molecules 27:2879. doi: 10.3390/molecules27092879
Zhang, R., Khan, S. A., Chi, J., Wei, Z., Zhang, Y., Deng, Y., et al. (2018). Different effects of extrusion on the phenolic profiles and antioxidant activity in milled fractions of brown rice. LWT 88, 64–70. doi: 10.1016/j.lwt.2017.09.042
Keywords: gluten-free pasta, Riceberry rice flour, cricket powder, insect protein, antioxidants, food fortification, D-optimal mixture design, extrusion
Citation: Musika J, Kapcum C, Itthivadhanapong P, Musika T, Hanmontree P and Piayura S (2024) Enhancing nutritional and functional properties of gluten-free Riceberry rice pasta supplemented with cricket powder using D-optimal mixture design. Front. Sustain. Food Syst. 8:1417045. doi: 10.3389/fsufs.2024.1417045
Received: 13 April 2024; Accepted: 31 July 2024;
Published: 12 August 2024.
Edited by:
Konstantina Argyri, University of Peloponnese, GreeceReviewed by:
Sunil C. K., National Institute of Food Technology, Entrepreneurship and Management, Thanjavur (NIFTEM-T), IndiaCopyright © 2024 Musika, Kapcum, Itthivadhanapong, Musika, Hanmontree and Piayura. This is an open-access article distributed under the terms of the Creative Commons Attribution License (CC BY). The use, distribution or reproduction in other forums is permitted, provided the original author(s) and the copyright owner(s) are credited and that the original publication in this journal is cited, in accordance with accepted academic practice. No use, distribution or reproduction is permitted which does not comply with these terms.
*Correspondence: Sumeth Piayura, spiayura@snru.ac.th; Pimchada Itthivadhanapong, pimchada.i@mail.rmutk.ac.th
Disclaimer: All claims expressed in this article are solely those of the authors and do not necessarily represent those of their affiliated organizations, or those of the publisher, the editors and the reviewers. Any product that may be evaluated in this article or claim that may be made by its manufacturer is not guaranteed or endorsed by the publisher.
Research integrity at Frontiers
Learn more about the work of our research integrity team to safeguard the quality of each article we publish.