- 1College of Economics and Management, Huzhou College, Huzhou, China
- 2School of Economics and Management, Huzhou University, Huzhou, China
- 3School of Public Management and Law, Anhui University of Technology, Ma'anshan, China
Background: The adoption of new agricultural technologies is one of the key factors for achieving sustainable agricultural development. With the rapid development of China’s economy and the widening gap between urban and rural areas, it has become the norm for farmers to seek employment outside their home villages. This phenomenon not only changes the lifestyle of farmers but may also affect their attitudes toward and adoption behavior of new agricultural technologies. However, existing research in this field is not yet sufficient. This paper aims to assess the overall impact, heterogeneous effects, and mechanisms of action of migrant work experience on farmers’ willingness to adopt new agricultural technologies.
Methods: Based on the 8,391 sample data from 243 counties in the 2018 China Labor Force Dynamic Survey (CLDS2018), this paper uses the Linear Probability Model (LPM), Two Stage Least Square (2SLS), Conditional Mixed Process (CMP) methods, and omitted variable test to estimate the impact of migrant work experience on farmers’ willingness to adopt new agricultural technologies. Simultaneously, it explored the heterogeneity in the relationship between migrant work experience and the willingness to adopt new agricultural technologies from the perspectives of gender, age, and educational level. Lastly, the Karlson-Holm-Breen (KHB) model was utilized to analyze the mechanisms through which migrant work experience affects farmers’ willingness to adopt new agricultural technologies.
Results: Migrant work experience significantly enhances farmers’ willingness to adopt new agricultural technologies, with the probability of willingness among farmers with migrant work experience being 26.65% higher than that of farmers without such experience. At the same time, compared to female farmers, those born before 1980, and those with primary education or less, this enhancing effect is more pronounced among male farmers, those born after 1980, and those with more than a primary education. Furthermore, it was found that migrant work experience not only directly enhances farmers’ willingness to adopt new agricultural technologies but also indirectly promotes it by increasing capital accumulation, enhancing risk awareness, expanding social networks, and strengthening agricultural cognition, with risk awareness and agricultural cognition playing a larger indirect role.
Conclusion: Based on the empirical results, this paper suggests actively guiding farmers with migrant work experience to adopt new agricultural technologies, and encouraging return migrant labor with the intention of resettling in rural areas to engage in agriculture again, to further promote the adoption of new agricultural technologies.
1 Introduction
With the intensification of agricultural non-point source pollution and the continuous rise in labor costs, traditional agriculture, reliant on intensive input, has become a significant burden on the environment and economically unsustainable due to increased labor costs. Therefore, it is particularly crucial to achieve the transformation and upgrading of the agricultural industry through the adoption of new agricultural technologies. The introduction of new agricultural technologies can not only optimize the input structure of agricultural production factors but also compensate for the deficiencies of a single technology through the integrated application of various technologies, thereby enhancing the overall efficiency and sustainability of agricultural production (Barnes et al., 2019). Moreover, the promotion of new agricultural technologies is crucial for changing the status of inefficient resource utilization and narrowing the gap in agricultural production efficiency between developing and developed countries (Chen, 2020). These technologies can not only enhance the adaptability of small-scale farmers to climate change but also help improve the efficiency of agricultural production factor allocation, creating higher economic benefits and better quality of life for farmers (Makate et al., 2019).
However, the promotion and adoption of new agricultural technologies have always been core issues in agricultural development research. Particularly in developing countries, the development and application of modern agricultural technologies have become important means to promote agricultural production efficiency and sustainable growth. These technologies, including precision agriculture, biotechnology, the development of drought-resistant and pest-resistant crop varieties, and the application of information and communication technology in agricultural management, have the potential to significantly enhance the efficiency and sustainability of agricultural production (Reddy, 2015). However, despite the significant potential advantages of these advanced agricultural technologies, their adoption rate in many developing countries is often lower than expected. Over the past few decades, the adoption of agricultural technologies in some countries and regions of Africa has been lagging, especially in Sub-Saharan Africa, where the adoption and development of agricultural technologies have been almost stagnant (Duflo et al., 2011). This phenomenon contrasts sharply with the rapid growth of agricultural productivity in developed countries and many middle-income countries. According to data from the World Bank, from 1961 to 2014, grain production in Sub-Saharan Africa increased by only 80%, while in the United States, it grew by 203% during the same period (World Bank, 2014). Furthermore, comparing data from 1961 and 2014, agricultural productivity in the United States was 273% higher than in Sub-Saharan Africa, and by 2014, this gap had widened to 473% (Magruder, 2018). Additionally, the “2024 China Agricultural and Rural Development Trend Report” shows that in 2023, the comprehensive mechanization rate of China’s crops exceeded 73%, and the contribution rate of agricultural technological progress exceeded 63%, which still remains at a lower level compared to developed countries (over 90%). Why is there such a large gap between developed and developing countries in the adoption of new agricultural technologies? Why are some profitable new agricultural technologies still underadopted?
Currently, academic discussions on the factors influencing farmers’ adoption of new agricultural technologies mainly include the following aspects: Firstly, economic factors. When deciding whether to adopt new agricultural technologies, farmers weigh the expected costs and benefits (Feder et al., 1985). A lack of sufficient funds and financing channels limits farmers’ investment capacity in new agricultural technologies, thereby affecting their willingness to adopt (Lemecha, 2023; Rayhan et al., 2023). Secondly, social and cultural factors. Social networks and peer effects can significantly influence farmers’ perceptions and attitudes toward new agricultural technologies (Dearing and Cox, 2018). Traditional agricultural practices and cultural values also affect farmers’ acceptance of new technologies. Deep-rooted traditional agricultural practices and resistance to new things may hinder the adoption of new agricultural technologies (Stone, 2007). Thirdly, biophysical and environmental factors. Farmers’ adoption of new agricultural technologies is influenced not only by economic and social factors but also by natural conditions, soil fertility, water resources, and climate change (Amankwah, 2023; Asante et al., 2024). Fourthly, psychological factors. Farmers’ risk preferences and expectations for the future also affect their attitudes toward new agricultural technologies. Farmers with a high tolerance for risk are more likely to try adopting new agricultural technologies (Dercon and Christiaensen, 2011; Musyoki et al., 2022). Fifthly, policy and institutional environmental factors. Government policy support can reduce the costs and risks associated with adopting new agricultural technologies, thus encouraging farmers to adopt new technologies (Abate et al., 2016). Additionally, research has found that farmers’ characteristics, including gender, age, education level, health status, and income, are also key factors influencing their adoption of new agricultural technologies (Djibo and Maman, 2019; Hirpa Tufa et al., 2022; Kafando et al., 2023).
With the lowering of the barriers to migrant work and the increase in non-agricultural employment opportunities, the phenomenon of rural residents in China migrating for work has become increasingly common. At the same time, there has been a significant return migration. This shift not only affects the economic conditions of farming households but may also change farmers’ attitudes toward and adoption of new technologies. Although existing studies have explored various factors influencing farmers’ adoption of agricultural technologies, research on how migrant work experience affects farmers’ willingness to adopt new agricultural technologies remains relatively limited. Therefore, based on CLDS2018 data, this paper attempts to analyze the key factors and mechanisms influencing farmers’ willingness to adopt new agricultural technologies from the perspective of migrant work experience. This study is not only significant for understanding the socio-economic factors in the promotion of agricultural technologies but also provides empirical evidence for the formulation of relevant policies. Compared to existing research, the marginal contributions of this paper are: First, from the perspective of non-agricultural employment, it systematically examines the promoting effect of migrant work experience on farmers’ willingness to adopt new agricultural technologies, thereby extending the related research on this topic. Second, based on the evaluation of the basic effects, this paper deeply analyzes the mechanisms by which migrant work experience influences farmers’ willingness to adopt new agricultural technologies from the perspectives of capital accumulation, risk awareness, social networks, and agricultural cognition. Additionally, it examines the heterogeneity in the influence of migrant work experience on farmers’ willingness to adopt new agricultural technologies. This helps to more comprehensively grasp the causal relationships between the core variables.
2 Theoretical analysis
In modern agriculture, technological innovation is regarded as the key to enhancing productivity and achieving sustainable development. As rational economic agents, farmers weigh the adoption of new agricultural technologies based on the principle of maximizing economic benefits (Feder et al., 1985). Migrant work experience, as wealth and social capital accumulated through practice, significantly impacts farmers’ behavior in adopting new agricultural technologies. This experience not only provides farmers with opportunities to acquire new knowledge, experience, and skills but may also change their fundamental attitudes towards agricultural production and lifestyle (De Janvry and Sadoulet, 2001). Against the backdrop of counter-urbanization, farmers who have worked away from home choosing to return to engage in agricultural production has become a rational career choice. Compared to traditional farmers, those with migrant work experience show greater enthusiasm in adopting and investing in new agricultural technologies (De Janvry and Sadoulet, 2001). Thus, the impact of migrant work experience on farmers’ decisions to adopt new agricultural technologies may be a combination of direct and indirect effects. Migrant work experience also indirectly enhances farmers’ willingness to adopt new agricultural technologies by increasing capital accumulation, enhancing risk awareness, expanding social networks, and strengthening agricultural cognition. This paper constructs the analytical framework as shown in Figure 1.
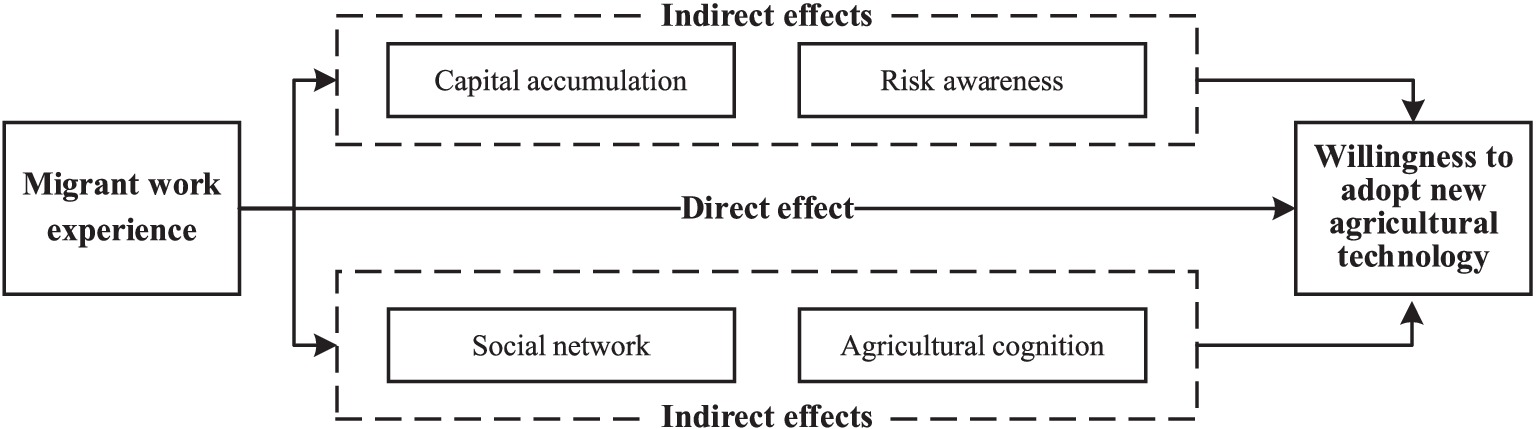
Figure 1. The impact path of migrant work experience on farmers’ willingness to adopt new agricultural technology.
Firstly, income from migrant work is one of the primary sources of income and wealth accumulation for rural households (Zhao and Jiang, 2022). Compared to traditional farming, income from migrant work far exceeds that from traditional farming, thus forming an accumulation of economic capital (Zhao, 1999; Frankelius et al., 2019). At the same time, migrant work, by diversifying sources of income, increases the possibility of accessing formal credit, thereby alleviating liquidity constraints on the adoption of new agricultural technologies (Stark and Bloom, 1985; Phan, 2012). Therefore, migrant work experience indirectly increases farmers’ willingness to adopt new agricultural technologies through the accumulation of economic capital.
Secondly, migrant work experience is also beneficial in enhancing farmers’ risk awareness and ability. The adoption of new agricultural technologies carries both risks and economic benefits; improper use of new technologies can result in the risk of reduced agricultural production and income (Sunding and Zilberman, 2001). Faced with intense market competition and operational risks, migrant work experience is more advantageous for farmers to accumulate extensive market expansion experience and form scientific development concepts (Yu et al., 2017). Considering costs and long-term benefits, as rational economic agents, to maximize the mitigation of agricultural operational risks, the adventurous spirit and innovation consciousness accumulated during migrant work may encourage farmers to adopt new agricultural technologies more actively (Knight and Gunatilaka, 2010). Therefore, migrant work experience indirectly increases farmers’ willingness to adopt new agricultural technologies by enhancing risk awareness.
Thirdly, migrant work can expand the radius of farmers’ social interactions, break through inherent geographical and kinship social networks, and construct entirely new networks based on profession and interest. Extensive networks play a crucial role in farmers obtaining technical information and resources (Amuedo-Dorantes and Mundra, 2007). In the process of adopting new agricultural technologies, farmers will apply the social network relationships acquired during migrant work to agricultural production as much as possible, in order to maximize economic returns (Ramirez, 2013; Varshney et al., 2022). Networks based primarily on profession and interest help broaden funding sources, enhance the level of private lending, and alleviate farmers’ financial pressures (Maertens and Barrett, 2013). Therefore, migrant work experience indirectly enhances farmers’ willingness to adopt new agricultural technologies through the expansion of social networks.
Lastly, the premise of adopting production technology is the cognition of the agricultural technology; the higher the farmers’ level of cognition of agricultural production technology, the more accurately they will recognize its role in agriculture, and the greater the likelihood of adopting the technology (Chen and Chen, 2017; Sui and Gao, 2023). Compared with traditional farmers, migrant work experience not only can broaden farmers’ horizons and expand their knowledge but also can promote changes in farmers’ knowledge structure and enhance their self-learning ability, thereby increasing their enthusiasm for adopting agricultural technologies (Li et al., 2023). Therefore, migrant work experience indirectly increases farmers’ willingness to adopt new agricultural technologies by enhancing agricultural cognition.
3 Methodology
3.1 Data source
This paper uses data from the 2018 China Labor-force Dynamics Survey organized by Sun Yat-sen University. The use of this data is primarily due to the following considerations: First, the survey covers 28 provincial-level administrative units in mainland China and employs a random stratified sampling method, including data on individuals, households, and villages, combining large sample size and scientific rigor. Second, the CLDS focuses on the current status and changes of China’s labor force, with particular attention to rural household agricultural production, as well as the education and migration histories of workers, providing foundational data for this study. Third, CLDS2018 is the latest available data, providing empirical evidence for evaluating farmers’ willingness to adopt new agricultural technologies. Considering the questionnaire design and research needs, only samples aged 18 and above with agricultural household registration were retained. After excluding samples with missing key variables, a total of 8,391 valid samples were obtained.
3.2 Variables selection
The dependent variable is the willingness to adopt new agricultural technologies. Referring to existing studies on the measurement of the tendency and behavioral intention to adopt new agricultural technologies (Bonokwane and Ololade, 2022; Zhou et al., 2022), this paper measures farmers’ willingness to adopt new agricultural technologies through the item in the CLDS 2018 questionnaire: “Do you agree that compared to other farmers, you are more proactive in adopting newly promoted agricultural technologies?” The respondents’ answers include “strongly disagree,” “disagree,” “neutral,” “agree,” and “strongly agree,” which are assigned values of 1, 2, 3, 4, and 5, respectively.
The key explanatory variable is the experience of working away from home. According to the “2023 Migrant Worker Monitoring Survey Report” published by the National Bureau of Statistics of China, migrant workers are defined as those who are registered in rural areas but engage in non-agricultural industries outside their registered areas or work away from their registered areas for 6 months or more within a year. This means that working away from home is defined as leaving one’s registered area for 6 months or more. Therefore, this paper measures farmers’ experience of working away from home through the survey question, “Have you ever worked away from home for more than 6 months (cross-county migration)?” The respondents’ answers include “Yes” and “No,” which are coded as 1 and 0, respectively.
The instrumental variable is natural disasters. Considering potential endogeneity issues, this paper uses the instrumental variable method for testing. An effective instrumental variable should meet the following two criteria: first, relevance, meaning the instrumental variable should be related to the key explanatory variable, which is the experience of working away from home. Second, exogeneity, meaning the instrumental variable should not directly affect the dependent variable, which is the willingness to adopt new agricultural technologies. Referring to the approach used in relevant literature (Munshi, 2003; Chen, 2012), this paper selects whether the respondent’s village experienced severe natural disasters before 1978 as the instrumental variable. On one hand, severe natural disasters may cause damage to local agricultural production, thereby forcing farmers to seek off-farm employment opportunities to supplement their household income. On the other hand, natural disasters are often considered to be random events, and severe natural disasters that occurred in the past generally do not directly affect the current willingness of farmers to adopt new agricultural technologies. Therefore, this study selects whether the village experienced severe natural disasters before 1978 as the instrumental variable.
Meanwhile, capital accumulation, risk awareness, social networks, and agricultural cognition are regarded as mechanisms through which off-farm work experience influences farmers’ willingness to adopt new agricultural technologies. Capital accumulation refers to the process by which farmers increase their economic resources through various means and is a key factor affecting their behavior in adopting new agricultural technologies. Drawing on the approach of existing research (Dercon and Christiaensen, 2011), this paper measures capital accumulation using the survey item “total non-agricultural income of the household in the past year.” Considering the large income differences among households, this study log-transforms the total non-agricultural income to reduce heteroscedasticity. Risk awareness refers to an individual’s level of cognition and understanding of potential risks, as well as their ability to consider and respond to risks in decision-making (Conley and Udry, 2010). This paper measures farmers’ risk awareness using the survey item “whether you would take a bank loan to buy a car,” with responses coded as 1 for “yes” and 0 for “no.” Social networks refer to relatively stable relationship systems formed among social members through interaction. The main measurement methods are the name-generator method and the position-generator method (Wellman, 1979). This paper measures farmers’ social networks using the name-generator method with the survey item “whether you have local friends with whom you can discuss important issues,” coding responses as 1 for “yes” and 0 for “no.” Agricultural cognition mainly refers to farmers’ understanding and knowledge of various aspects of agricultural production, including techniques, resources, and management methods. Drawing on relevant research (Barham et al., 2018), this paper measures agricultural cognition using the survey item “whether you agree that agricultural production is becoming increasingly important for your family,” with responses coded as 1 for “yes” and 0 for “no.”
Additionally, drawing on existing research (Mao et al., 2021; Lemecha, 2023), this study also controlled for various potential confounding factors that may simultaneously affect farmers’ off-farm work experience and willingness to adopt new agricultural technologies. These factors include individual characteristics such as gender, age, marital status, health status, education level, political affiliation, agricultural production experience, and professional skills training, as well as family characteristics such as the number of agricultural workers in the family, agricultural planting area, agricultural operating income, agricultural operating costs, and agricultural cultivation methods. Additionally, considering the differences in the socio-economic development levels of different regions (county-level administrative units), this study also controlled for regional effects in the form of dummy variables.
3.3 Empirical strategies
First, the econometric model for the impact of off-farm work experience on farmers’ willingness to adopt new agricultural technologies is specified as follows:
As shown in Equation (1), represents the ith farmer’s willingness to adopt new agricultural technologies, represents the ith farmer’s migrant work experience, are control variables, and is a dummy variable for the county where farmers are located, thereby controlling for the impact of differences in the socio-economic development level of different counties on farmers’ willingness to adopt new agricultural technologies. , , , and are the parameters to be estimated, and is the random error term.
Second, it is important to note that the above baseline regression may still have potential endogeneity. On the one hand, the adoption of new agricultural technologies can, to some extent, reduce the demand for household labor, thus providing farmers with more opportunities to seek migrant work. In this case, the willingness to adopt new agricultural technologies might be a cause of migrant work experience among farmers, not merely a result, leading to reverse causality issues. On the other hand, the factors influencing farmers’ decisions to adopt new agricultural technologies are complex and not easily fully controlled in the model. There may be other unobservable factors that simultaneously affect both migrant work experience and the willingness to adopt new agricultural technologies, leading to omitted variable issues. The instrumental variable method is a conventional means of addressing endogeneity issues, which requires constructing a regression equation for migrant work experience and its instrumental variable before estimating Equation (1):
In Equation (2), is the instrumental variable, , , and are parameters to be estimated, and is the error term. Then, the 2SLS model is employed to correct for the potential endogeneity bias in the baseline regression process. At the same time, considering that the potentially endogenous variable of migrant work experience is a binary variable, the use of the 2SLS model to some extent overlooks the categorical nature of migrant work experience, failing to fully utilize the information, thus resulting in a loss of estimation efficiency. For this reason, the CMP estimation method is introduced, which can fit a series of multi-equation, multilevel, and conditionally recursive mixed processes, allowing for the re-estimation of instrumental variables within a unified CMP framework (Roodman, 2011). CMP is based on seemingly unrelated regression, constructing a system of recursive equations based on maximum likelihood estimation, requiring the simultaneous estimation of Equation (1) and Equation (2). The correlation coefficient atanhrho of the error terms of the two equations can be used to determine whether the migrant work experience variable is endogenous. If the atanhrho value is significantly different from 0, the CMP estimation results are superior to the baseline regression results.
Third, changing the analytical approach. If, after including as many reasonable control variables as possible in the analysis, the coefficients of the core explanatory variables remain stable, this means that even the presence of omitted variables is unlikely to “overturn” the core conclusions of this paper (Lacetera et al., 2012). This paper uses the method proposed by Oster to test for potential omitted variables and their impact on regression results (Oster, 2019). Specifically, when the regression model contains some unobservable omitted variables, an approximation of the consistent estimate of the impact of migrant work experience on farmers’ willingness to adopt new agricultural technologies, , can be obtained through calculation:
In Equation (3), represents the impact of migrant work experience on farmers’ willingness to adopt new agricultural technologies, and respectively represent the estimated parameter and goodness-of-fit for migrant work experience when constrained control variables are included. and respectively represent the estimated parameter and goodness-of-fit for migrant work experience when all observable variables are included as control variables. represents the ratio of the explanatory power of observable variables to unobservable variables on farmers’ willingness to adopt new agricultural technologies. represents the maximum goodness-of-fit of the regression equation when all omitted variables are included in the model.
Following Oster’s suggestion (Oster, 2019), two identification strategies are employed to test the impact of omitted variables: First, assuming is 1.3, 1.5, and 1.7 times the current regression equation’s goodness-of-fit, and =1, if falls within the 95% confidence interval of the estimated parameters, then the coefficient estimate passes the robustness test. Second, also assuming is 1.3, 1.5, and 1.7 times the current regression equation’s goodness-of-fit, but =0, if the value of is greater than 1, it indicates that omitted variables do not change the impact of explanatory variables on the dependent variable.
In addition, to further validate the empirical results, robustness checks were conducted through four methods: adjusting the dependent variable, independent variables, regional effects, and econometric model. Firstly, the assignment of the dependent variable was adjusted, converting the five-category variable of willingness to adopt agricultural new technologies into a binary variable, with “somewhat agree” and “strongly agree” assigned a value of 1, and all other cases assigned a value of 0. Secondly, a variable highly correlated with the explanatory variables was used for robustness testing. The experience of working continuously outside the township for more than 6 months was measured by the questionnaire item “Have you ever worked continuously outside your township for more than 6 months?” with “yes” assigned a value of 1 and “no” assigned a value of 0. Thirdly, the county-level dummy variables in the regression model were replaced with city-level dummy variables to capture the differences between different cities. Finally, since the variable for adopting agricultural new technologies is an ordered categorical variable, the econometric model was changed to an ordered Probit model for re-estimation.
4 Results and discussion
4.1 Descriptive statistics
The basic characteristics of the sample are shown in Table 1. The average value of farmers’ willingness to adopt new agricultural technologies is 3.4827, which is between “neutral” and “agree,” indicating that farmers’ willingness to adopt new agricultural technologies is high. Meanwhile, 21.53% of farmers have experience working outside their hometowns, with males accounting for 51.42%. The average age is 49.54 years, and 89.23% of the farmers are married. The average years of education are 7.1, and 68.47% of farmers have experience in agricultural production, while only 5.21% have participated in professional skills training. In addition, the average number of people involved in family agricultural production is 1.29, and the proportion of agricultural mechanization is 36.84%.
4.2 Baseline regression results
This paper estimates Equation (1) using a linear probability model, with the results shown in Table 2. To delineate the influence of different factors on the willingness to adopt agricultural new technologies, a stepwise regression approach was adopted: (1) controlling only for core explanatory variables, (2) adding individual characteristic control variables, (3) adding household characteristic control variables, and (4) adding county-level dummy variables. From the estimation results in Table 2, whether controlling only for the core explanatory variables, adding individual characteristic control variables, urban feature control variables, or incorporating county-level dummy variables, the impact of migrant work experience on farmers’ willingness to adopt new agricultural technologies is significant at the 1% statistical level, and the regression coefficient is positive, indicating robust estimation results. From the results in column (4), compared to farmers without migrant work experience, those with such experience have a 10.54% higher probability of willingness to adopt new agricultural technologies. The results above indicate that migrant work experience can effectively enhance farmers’ willingness to adopt new agricultural technologies.
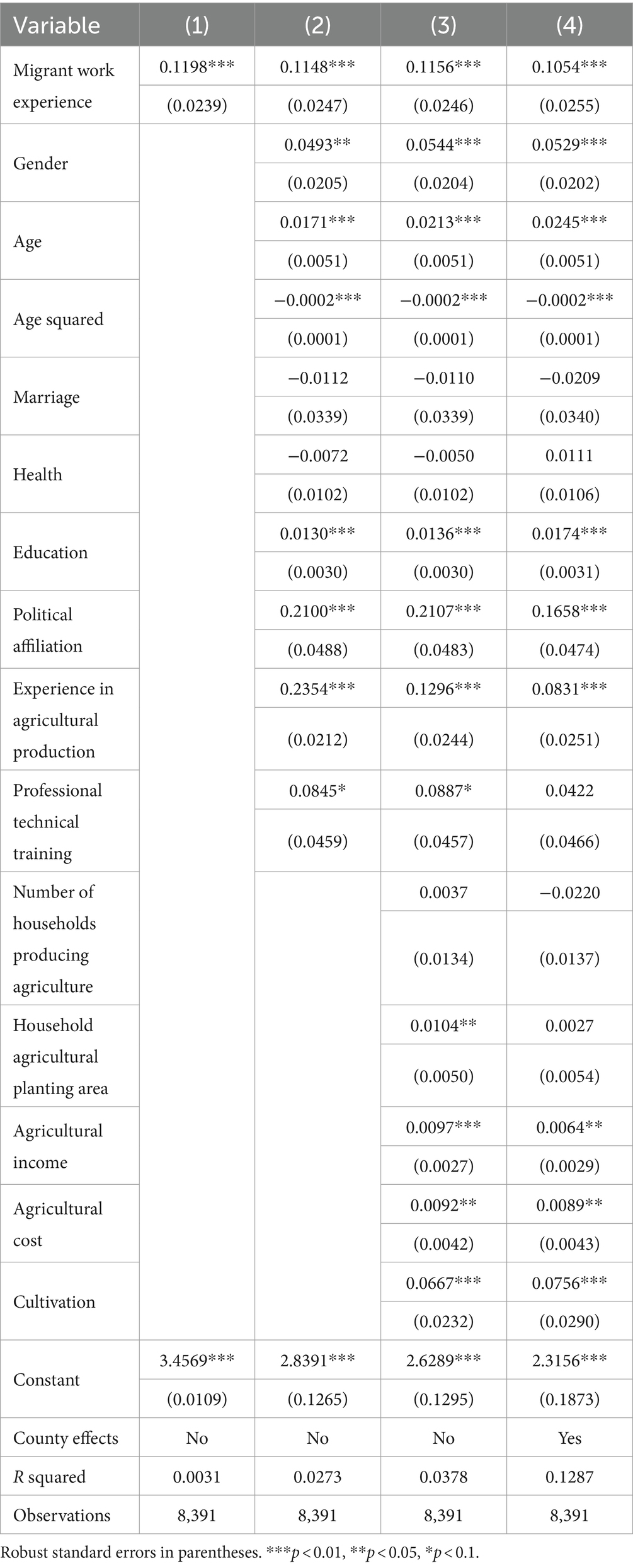
Table 2. Baseline regression results: the overall impact of migrant work experience on farmers’ adoption of agricultural technology.
Additionally, the estimation results of individual characteristic control variables in column (4) show that gender is significant, with a positive coefficient, indicating that male farmers have a stronger willingness to adopt agricultural new technologies compared to female farmers. This is consistent with the conclusions of most studies, suggesting that male farmers may have more resources and opportunities to acquire information and knowledge related to new technologies, and social and cultural factors may also give males greater autonomy in the decision-making process of adopting new technologies (Peterman et al., 2014). There is a nonlinear relationship (inverted U-shaped) between age and willingness to adopt agricultural new technologies. As age increases, farmers’ willingness to adopt new agricultural technologies gradually increases, but after reaching a certain age threshold (around 43 years old), this willingness begins to decline. This finding is similar to existing research results, which also observe a decrease in farmers’ enthusiasm for adopting new technologies after a certain age point (Yue et al., 2023). Older farmers tend to be more conservative, relying more on existing agricultural practices, and becoming more cautious about adopting new agricultural technologies that may bring changes (Neway and Zegeye, 2022). The higher the level of education, the stronger the willingness of farmers to adopt agricultural new technologies. Similar results have been found in related studies, indicating that education can enhance farmers’ receptivity to new information, improve their understanding of agricultural new technologies, and thus increase the likelihood of adopting them (Conley and Udry, 2010). Additionally, Communist Party members and farmers with agricultural production experience have a significantly stronger willingness to adopt agricultural new technologies. Communist Party members are representatives of rural areas who are more likely to accept advanced technologies and ideas, with better knowledge reserves and stronger abilities to accept new things. They are more inclined to adopt and promote agricultural new technologies that can improve agricultural production efficiency and sustainability (Kung and Liu, 1997; Luo et al., 2023). Farmers with agricultural production experience may have a deeper understanding of the practical needs of agricultural production and the potential value of technological improvements, thus being more willing to adopt new technologies (Hu et al., 2022). The higher the agricultural operating income, the stronger the willingness of farmers to adopt agricultural new technologies. Existing literature generally agrees that farmers’ income level is an important factor influencing their willingness and ability to adopt new technologies. Higher income not only provides more resources for investing in new technologies but also increases the ability to try new technologies and reduces the risk of failure in adopting new technologies (Dercon and Christiaensen, 2011).
Moreover, the higher the agricultural operating cost, the stronger the willingness of farmers to adopt agricultural new technologies. This finding contrasts with the conclusions of some existing literature. The traditional view is that lower agricultural operating costs enable farmers to invest more in new technologies (Feder et al., 1985). However, recent studies have begun to focus on the complex motivations behind farmers’ adoption of new agricultural technologies, including using them to cope with increasing production costs (Kinuthia and Mabaya, 2017). Previous research has found that when faced with higher production costs, farmers in Uganda are more inclined to adopt improved seeds and more efficient cultivation methods (Kabunga et al., 2012). Farmers using mechanized cultivation have a stronger willingness to adopt agricultural new technologies. Mechanization can act as a catalyst for technological innovation, encouraging farmers to try and adopt other new agricultural technologies (Beaman et al., 2021).
4.3 Instrumental variable checks
Although the baseline regression analysis controlled for variables that may affect the relationship between migration experience and the adoption of new agricultural technologies, potential endogeneity issues such as reverse causality and omitted variable bias may still exist in the empirical analysis. To address this, further analysis was conducted using the 2SLS model, and Table 3 reports the estimation results of the 2SLS model. From the first stage estimation results of the 2SLS model in column (1), the instrumental variable has a significant positive impact on migrant work experience, indicating that the instrumental variable meets the relevance condition. The robust F-statistic is 18.4757, greater than the commonly used critical value of 10, suggesting that there is no weak instrumental variable. At the same time, the endogeneity test parameter for migrant work experience is significant at the 1% statistical level, indicating that migrant work experience is indeed endogenous, making the 2SLS model’s estimation results more credible compared to the CMP model. From the second stage estimation results of the 2SLS model in column (2), the coefficient for migrant work experience is 2.5307 and significant, larger than the coefficient in Table 2, with a larger robust standard error, indicating that the baseline regression underestimates the enhancing effect of migrant work experience on farmers’ willingness to adopt new agricultural technologies.
Moreover, considering that the endogenous variable of migrant work experience is binary, the 2SLS model, to some extent, overlooks the binary nature of migrant work experience, failing to fully utilize the information and thus leading to a loss in estimation efficiency. For this reason, the CMP method was further utilized for re-estimation. From the first stage regression results of the CMP method in column (3), the instrumental variable has a significant positive impact on migrant work experience, which also verifies that the instrumental variable meets the relevance requirement. The second stage regression results of the CMP method in column (4) show that migrant work experience has a significant positive impact on farmers’ willingness to adopt new agricultural technologies, with a coefficient of 0.2665. Compared to the coefficient in column (2), it is smaller and the standard error is reduced, indicating that there is indeed an issue of effectiveness loss in the 2SLS model estimation. From the endogeneity test results, the atanhrho_12 value is significant at the 1% statistical level, rejecting the null hypothesis that migrant work experience is an exogenous variable. After addressing endogeneity, the estimation results of the CMP method and the 2SLS model, as well as the direction and significance of effects in the LPM model, are consistent, fully demonstrating that the positive impact of migrant work experience on farmers’ willingness to adopt new agricultural technologies is robust.
4.4 Omitted variables checks
Table 4 reports the results of the omitted variable test based on the Oster method. It can be seen that when =0, regardless of whether is set to 1.3 times, 1.5 times, or 1.7 times, the actual calculation results of the migration experience variable all fall within the 95% confidence interval of , and the δ values are all greater than 1, indicating that the coefficient of the migration experience variable’s impact on farmers’ willingness to adopt new agricultural technologies is relatively stable. Therefore, it can be considered that even if there are omitted variables, the judgment regarding the relationship between migration experience and the adoption of new agricultural technologies remains robust, that is, migration experience significantly enhances farmers’ willingness to adopt new agricultural technologies.
4.5 Robustness checks
Table 5 reports the estimation results of the robustness tests. From column (1), which adjusts the assignment method of the dependent variable for re-regression, it can be seen that migration experience remains highly significant and the coefficient is positive. The results of adjusting the explanatory variables for regression are shown in column (2). The results indicate that even with different measures of migration experience, the conclusions of Table 2 remain unchanged. From column (3), where the county-level dummy variables in the regression model are replaced with city-level dummy variables for re-regression, it can be seen that migration experience is significant at the 1% statistical level, and the coefficient is positive. From column (4), where the econometric model is replaced with an ordered Probit model for re-estimation, it can be seen that migration experience remains highly significant and the coefficient is positive. The above four robustness test results indicate that migration experience significantly enhances farmers’ willingness to adopt new agricultural technologies, further confirming the robustness of the core conclusion.
4.6 Heterogeneous effects
The previous analysis has concluded that migration experience significantly enhances farmers’ willingness to adopt new agricultural technologies. However, it is important to note that this is only the average effect at the whole-sample level and does not consider the heterogeneity of this impact. To obtain more detailed research conclusions, heterogeneity analysis will be conducted by grouping based on gender, age, and education level. The specific estimation results are shown in Table 6.
Columns (1) and (2) report the differences between genders. The results show that, overall, both male and female farmers’ willingness to adopt new agricultural technologies is significantly positively influenced by migration experience. However, in terms of the magnitude of the effect, migration experience has a greater impact on male farmers’ willingness to adopt new agricultural technologies compared to female farmers. Due to social and cultural factors and gender differences in resource access, male farmers often have more opportunities to access new technologies and related training, making them more likely to adopt new agricultural technologies (Peterman et al., 2014).
Columns (3) and (4) report the differences between generations. The results show that, overall, the willingness to adopt new agricultural technologies is significantly positively influenced by migration experience for both farmers born before 1980 and those born after 1980. However, in terms of the magnitude of the effect, migration experience has a greater impact on the willingness to adopt new agricultural technologies for farmers born after 1980. This is mainly because younger farmers generally have higher education levels, stronger learning abilities, and broader social networks, which facilitate the reception and adoption of new agricultural technologies (Feder et al., 1985).
Columns (5) and (6) report the differences between education levels. The results show that, overall, migration experience significantly positively influences the willingness to adopt new agricultural technologies for both farmers with elementary education or below and those with more than elementary education. However, in terms of the magnitude of the effect, migration experience has a greater impact on the willingness to adopt new agricultural technologies for farmers with higher than elementary education. Previous research has shown that education level is one of the key factors affecting farmers’ adoption of new agricultural technologies. Farmers with higher education levels are more likely to access information about new agricultural technologies and have better understanding and implementation capabilities (Dearing and Cox, 2018).
4.7 Analysis of mediating effect
The analysis above shows that migrant work experience significantly enhances farmers’ willingness to adopt new agricultural technologies. So, how can the mechanism of this effect be explained? To test the mechanisms through which migrant work experience affects farmers’ willingness to adopt new agricultural technologies, this paper, integrating previous analysis and data availability, uses the KHB decomposition method proposed by Karlson et al. to examine the mechanisms of the enhancing effect of migrant work experience from four aspects: capital accumulation, risk awareness, social networks, and agricultural cognition, aiming to deeply reveal the relationship mechanism between migrant work experience and farmers’ willingness to adopt new agricultural technologies (Karlson et al., 2012).
Table 7 reports the estimation results based on the KHB method. From the results in column (1), it can be seen that the indirect effect of capital accumulation is significantly positive at the 10% statistical level, indicating that migration experience indirectly increases farmers’ willingness to adopt new agricultural technologies through increased capital accumulation. Similarly, columns (2), (3), and (4) show that the indirect effects of risk awareness and social networks are significantly positive at the 10% statistical level, and the indirect effect of agricultural cognition is significantly positive at the 5% statistical level. This implies that migration experience indirectly enhances farmers’ willingness to adopt new agricultural technologies by increasing risk awareness, expanding social networks, and enhancing agricultural cognition. Further analysis shows that the indirect effects of capital accumulation, risk awareness, social networks, and agricultural cognition account for 1.43, 4.91, 2.29, and 3.87% of the total effect of migration experience on farmers’ willingness to adopt new agricultural technologies, respectively. Therefore, migration experience not only directly increases farmers’ willingness to adopt new agricultural technologies but also indirectly enhances it by increasing capital accumulation, improving risk awareness, expanding social networks, and enhancing agricultural cognition. Among these, risk awareness and agricultural cognition have the larger indirect effects.
Migration experience for farmers is not only a source of household income but also an important means of capital accumulation. Higher income and capital accumulation provide farmers with the economic resources needed to adopt new technologies, thereby reducing the economic risk of adopting agricultural innovations (Zhao, 1999; Dercon and Christiaensen, 2011). This suggests that migration experience not only directly increases farmers’ income but also potentially enhances their economic status, thereby boosting their confidence and ability to adopt new agricultural technologies. Migration experience for farmers is not just an economic activity but also a process that enhances risk awareness. This increased risk awareness helps to improve farmers’ willingness to adopt new agricultural technologies. Existing literature generally considers risk awareness and risk management capabilities as important factors influencing farmers’ adoption of new agricultural technologies (Feder et al., 1985; Knight and Gunatilaka, 2010). Compared to existing studies, this research provides direct evidence that migration experience promotes technology adoption by enhancing risk awareness. Additionally, previous studies have shown that social networks are important channels for the dissemination and adoption of agricultural technologies. They facilitate the acceptance and application of new agricultural technologies through information sharing, experience exchange, and mutual learning (Bandiera and Rasul, 2006; Conley and Udry, 2010). Further emphasizes the role of migration experience in promoting the expansion of social networks, indicating that migration not only brings economic benefits to farmers but also increases their connections with the outside world, thereby broadening the channels for obtaining and exchanging information on new technologies. It is also found that migration experience for farmers is not just an economic activity; more importantly, it helps to enhance farmers’ understanding of agriculture, thereby increasing their willingness to adopt new agricultural technologies. Previous studies have already pointed out that education and knowledge levels are crucial for farmers to adopt new technologies (Dearing and Cox, 2018). However, there are relatively few studies on how migration experience, as a form of informal education, influences technology adoption by enhancing agricultural cognition. This study emphasizes the role of migration experience in promoting agricultural cognition, revealing that such experience allows farmers to be exposed to new agricultural practices, technologies, and concepts, thereby increasing their willingness to adopt new agricultural technologies. This finding extends the understanding of the mechanisms through which migration experience affects technology adoption, indicating that such experience influences technology adoption not only through economic channels but also through knowledge and cognitive channels.
5 Conclusion and policy implications
5.1 Conclusion
Smallholders are the main operators in the agriculture of most developing countries, which determines that promoting the development of new agricultural technologies needs to focus on the role of smallholders as the main practitioners of these technologies. China is the largest developing country in the world, and farmers, who account for over 98% of the micro-level agricultural operators nationwide, hold the key to agricultural development through the adoption of new agricultural technologies. However, in terms of the adoption of new agricultural technologies, the current adoption rate among farmers is generally low, with the vast majority still using extensive farming methods (World Bank, 2014; Chen, 2020). Existing studies have mainly explained farmers’ adoption of new agricultural technologies from the perspectives of farmer characteristics, transaction costs, technological risks, and diffusion channels. Additionally, migration for work, as an important form of non-agricultural employment, has gradually become a new entry point for scholars studying farmers’ adoption of new agricultural technologies, but the conclusions of existing literature are not yet consistent. Based on data from 8,391 samples across 243 counties in the CLDS2018, this paper systematically evaluates the impact of migration experience on farmers’ willingness to adopt new agricultural technologies. It deeply analyzes the mechanisms through which migration experience influences farmers’ willingness from the perspectives of capital accumulation, risk awareness, social networks, and agricultural cognition. Furthermore, it explores the significance of demographic characteristics in influencing farmers’ willingness to adopt new technologies, providing a new perspective for the promotion of new agricultural technologies.
Firstly, the study found that migration experience significantly increases the likelihood of farmers adopting new agricultural technologies. Farmers with migration experience are 26.65% more likely to adopt new agricultural technologies than those without such experience. This positive effect persists even after controlling for potential endogeneity bias and conducting a series of robustness tests. This study not only helps to reassess the value of farmers’ migration experience in promoting the dissemination of new agricultural technologies but also provides a deeper understanding of the development process of farmers’ adoption and application of new agricultural technologies. It offers factual support for further promoting the diffusion of new agricultural technologies and sustainable agricultural development.
Secondly, considering the heterogeneity in gender, age, and education level, the enhancement effect may vary. The heterogeneity results indicate that the impact of migration experience on farmers’ willingness to adopt new agricultural technologies differs by gender, age, and education level. This enhancement effect is more pronounced among male farmers, those born after 1980, and those with more than a primary school education. Therefore, in the process of promoting new agricultural technologies, training should be tailored to the attributes of farmers’ gender, age, and education level to select the most compatible and effective target groups.
Finally, it was found that migration experience not only has a direct effect on farmers’ willingness to adopt new agricultural technologies but also indirectly enhances this willingness through increasing capital accumulation, raising risk awareness, expanding social networks, and enhancing agricultural cognition. The results of this study confirm that capital accumulation, risk awareness, social networks, and agricultural cognition are important mechanisms through which migration experience influences farmers’ willingness to adopt new agricultural technologies.
5.2 Limitations
Despite using large national survey data organized by Sun Yat-sen University and employing various empirical analysis methods, this study still has limitations. Analyzing cross-sectional data makes it impossible to use fixed-effects models to control for individual differences that do not change over time. Additionally, due to limitations in the questionnaire information, it is not possible to further capture the impact of the duration of migration on farmers’ willingness to adopt new agricultural technologies, which is undoubtedly a significant regret of this study.
5.3 Policy implications
The conclusions of this study have important policy implications. First, for farmers with migration experience, they should be guided to combine the new skills and knowledge acquired through migration with modern agricultural production. This can be achieved through education, professional training, and technical guidance to improve their management quality and ultimately increase their adoption of new agricultural technologies. Second, when formulating policies, the agricultural sector should consider the differences in the adoption of new agricultural technologies among different groups (such as gender, age, and educational background). Customized support measures should be designed for different groups to ensure broad coverage and effectiveness of the policies. Finally, financial support and risk management training should be provided, and an information feedback mechanism should be established to enhance farmers’ preferences for agricultural production technologies, stimulate their enthusiasm for adopting new agricultural technologies, and thus promote the modernization and sustainable development of agricultural production.
Data availability statement
The original contributions presented in the study are included in the article/supplementary material, further inquiries can be directed to the corresponding author.
Ethics statement
Ethical review and approval was not required for the study on human participants in accordance with the local legislation and institutional requirements. Written informed consent from the [patients/ participants OR patients/participants legal guardian/next of kin] was not required to participate in this study in accordance with the national legislation and the institutional requirements.
Author contributions
QZ: Conceptualization, Data curation, Formal analysis, Methodology, Project administration, Software, Visualization, Writing – original draft, Writing – review & editing. MY: Funding acquisition, Supervision, Writing – review & editing. RS: Data curation, Writing – review & editing. RG: Formal analysis, Writing – review & editing.
Funding
The author(s) declare that financial support was received for the research, authorship, and/or publication of this article. This study was supported by the Soft Science Project of Ministry of Agriculture and Rural Affairs of China (Grant no. RKX2024028), the Key Project in Philosophy and Social Sciences of Zhejiang Province (Grant no. 24NDJC03Z), the Anhui University of Technology Young Faculty Research Fund Project (Grant no. QS202302), and Anhui University of Technology Introduced Talents Research Start-up Fund Project (Grant no. QD202375).
Conflict of interest
The authors declare that the research was conducted in the absence of any commercial or financial relationships that could be construed as a potential conflict of interest.
Publisher’s note
All claims expressed in this article are solely those of the authors and do not necessarily represent those of their affiliated organizations, or those of the publisher, the editors and the reviewers. Any product that may be evaluated in this article, or claim that may be made by its manufacturer, is not guaranteed or endorsed by the publisher.
References
Abate, G. T., Rashid, S., Borzaga, C., and Getnet, K. (2016). Rural finance and agricultural technology adoption in Ethiopia: does the institutional Design of Lending Organizations Matter? World Dev. 84, 235–253. doi: 10.1016/j.worlddev.2016.03.003
Amankwah, A. (2023). Climate variability, agricultural technologies adoption, and productivity in rural Nigeria: a plot-level analysis. Agric. Food Secur. 12:7. doi: 10.1186/s40066-023-00411-x
Amuedo-Dorantes, C., and Mundra, K. (2007). Social networks and their impact on the earnings of Mexican migrants. Demography 44, 849–863. doi: 10.1353/dem.2007.0039
Asante, B. O., Ma, W., Prah, S., and Temoso, O. (2024). Promoting the adoption of climate-smart agricultural technologies among maize farmers in Ghana: using digital advisory services. Mitig. Adapt. Strateg. Glob. Change 29:19. doi: 10.1007/s11027-024-10116-6
Bandiera, O., and Rasul, I. (2006). Social networks and technology adoption in northern Mozambique. Econ. J. 116, 869–902. doi: 10.1111/j.1468-0297.2006.01115.x
Barham, B. L., Chavas, J.-P., Fitz, D., and Schechter, L. (2018). Receptiveness to advice, cognitive ability, and technology adoption. J. Econ. Behav. Organ. 149, 239–268. doi: 10.1016/j.jebo.2017.12.025
Barnes, A. P., Soto, I., Eory, V., Beck, B., Balafoutis, A., Sánchez, B., et al. (2019). Exploring the adoption of precision agricultural technologies: a cross regional study of EU farmers. Land Use Policy 80, 163–174. doi: 10.1016/j.landusepol.2018.10.004
Beaman, L., BenYishay, A., Magruder, J., and Mobarak, A. M. (2021). Can network theory-based targeting increase technology adoption? Am. Econ. Rev. 111, 1918–1943. doi: 10.1257/aer.20200295
Bonokwane, L. P., and Ololade, O. O. (2022). Socio-economic factors affecting smallholder farmers’ willingness to adopt biodigester technology in South Africa. J. Energy South. Afr. 33, 10–20. doi: 10.17159/2413-3051/2022/v33i1a8860
Chen, C. (2020). Technology adoption, capital deepening, and international productivity differences. J. Dev. Econ. 143:102388. doi: 10.1016/j.jdeveco.2019.102388
Chen, Y. (2012). Do networks pay off among internal migrants in China?: an instrumental variable analysis. Chin. Sociol. Rev. 45, 28–54. doi: 10.2753/CSA2162-0555450102
Chen, Z., and Chen, G.-H. (2017). The influence of green technology cognition in adoption behavior: on the consideration of green innovation policy perception’s moderating effect. J. Discret. Math. Sci. Cryptogr. 20, 1551–1559. doi: 10.1080/09720529.2017.1390839
Conley, T. G., and Udry, C. R. (2010). Learning about a new technology: pineapple in Ghana. Am. Econ. Rev. 100, 35–69. doi: 10.1257/aer.100.1.35
Janvry, A.De, and Sadoulet, E. (2001). Income strategies among rural households in Mexico: the role of off-farm activities. World Dev. 29, 467–480, doi: 10.1016/S0305-750X(00)00113-3
Dearing, J. W., and Cox, J. G. (2018). Diffusion of innovations theory, principles and Practice. Health Aff. 37, 183–190. doi: 10.1377/hlthaff.2017.1104
Dercon, S., and Christiaensen, L. (2011). Consumption risk, technology adoption and poverty traps: evidence from Ethiopia. J. Dev. Econ. 96, 159–173. doi: 10.1016/j.jdeveco.2010.08.003
Djibo, O., and Maman, N. M. (2019). Determinants of agricultural technology adoption: farm households evidence from Niger. J. Dev. Agric. Econ. 11, 15–23. doi: 10.5897/JDAE2018.0998
Duflo, E., Kremer, M., and Robinson, J. (2011). Nudging farmers to use fertilizer: theory and experimental evidence from Kenya. Am. Econ. Rev. 101, 2350–2390. doi: 10.1257/aer.101.6.2350
Feder, G., Just, R. E., and Zilberman, D. (1985). Adoption of agricultural innovations in developing countries: a survey. Econ. Dev. Cult. Change 33, 255–298. doi: 10.1086/451461
Frankelius, P., Norrman, C., and Johansen, K. (2019). Agricultural innovation and the role of institutions: lessons from the game of drones. J. Agric. Environ. Ethics 32, 681–707. doi: 10.1007/s10806-017-9703-6
Hirpa Tufa, A., Alene, A. D., Cole, S. M., Manda, J., Feleke, S., Abdoulaye, T., et al. (2022). Gender differences in technology adoption and agricultural productivity: evidence from Malawi. World Dev. 159:106027. doi: 10.1016/j.worlddev.2022.106027
Hu, Y., Li, B., Zhang, Z., and Wang, J. (2022). Farm size and agricultural technology progress: evidence from China. J. Rural. Stud. 93, 417–429. doi: 10.1016/j.jrurstud.2019.01.009
Kabunga, N. S., Dubois, T., and Qaim, M. (2012). Yield effects of tissue culture bananas in Kenya: accounting for selection Bias and the role of complementary inputs. J. Agric. Econ. 63, 444–464. doi: 10.1111/j.1477-9552.2012.00337.x
Kafando, B., Pelenguei, E., and Gnedeka, K. T. (2023). Education and agricultural technology adoption: evidence from rural India. Emerg. Agric. Hortic. 17, 1–9. doi: 10.23958/eah/vol2023/17
Karlson, K. B., Holm, A., and Breen, R. (2012). Comparing regression coefficients between same-sample nested models using logit and Probit: a new method. Sociol. Methodol. 42, 286–313. doi: 10.1177/0081175012444861
Kinuthia, B. K., and Mabaya, E. (2017). The impact of agriculture technology adoption on farmers’ welfare in Uganda and Tanzania. Available at:https://www.academia.edu/download/77131754/download.pdf. Accessed on April 9, 2024).
Knight, J., and Gunatilaka, R. (2010). The rural–urban divide in China: income but not happiness? J. Dev. Stud. 46, 506–534. doi: 10.1080/00220380903012763
Kung, J. K., and Liu, S. (1997). Farmers’ preferences regarding ownership and land tenure in post-Mao China: unexpected evidence from eight counties. China J. 38, 33–63. doi: 10.2307/2950334
Lacetera, N., Pope, D. G., and Sydnor, J. R. (2012). Heuristic thinking and limited attention in the Car market. Am. Econ. Rev. 102, 2206–2236. doi: 10.1257/aer.102.5.2206
Lemecha, M. E. (2023). Credit constraint and agricultural technology adoptions: evidence from Ethiopia. Agric. Finance Rev. 83, 395–415. doi: 10.1108/AFR-06-2022-0075
Li, C., Li, X., and Jia, W. (2023). Non-farm employment experience, risk preferences, and low-carbon agricultural technology adoption: evidence from 1843 grain farmers in 14 provinces in China. Agriculture 13:24. doi: 10.3390/agriculture13010024
Luo, L., Luo, C., Liu, Y., and Fu, X. (2023). Study on green production technology adoption behavior of farmers from the perspective of heterogeneity: based on survey data of 836 citrus farmers in Sichuan Province. Chin. J. Agric. Resour. Reg. Plan. 44, 172–183. doi: 10.7621/cjarrp.1005-9121.20230417
Maertens, A., and Barrett, C. B. (2013). Measuring social networks’ effects on agricultural technology adoption. Am. J. Agric. Econ. 95, 353–359. doi: 10.1093/ajae/aas049
Magruder, J. R. (2018). An assessment of experimental evidence on agricultural technology adoption in developing countries. Annu. Rev. Resour. Econ. 10, 299–316. doi: 10.1146/annurev-resource-100517-023202
Makate, C., Makate, M., Mango, N., and Siziba, S. (2019). Increasing resilience of smallholder farmers to climate change through multiple adoption of proven climate-smart agriculture innovations. Lessons from southern Africa. J. Environ. Manag. 231, 858–868. doi: 10.1016/j.jenvman.2018.10.069
Mao, H., Zhou, L., Ying, R., and Pan, D. (2021). Time preferences and green agricultural technology adoption: field evidence from rice farmers in China. Land Use Policy 109:105627. doi: 10.1016/j.landusepol.2021.105627
Munshi, K. (2003). Networks in the modern economy: Mexican migrants in the U. S. Labor market*. Q. J. Econ. 118, 549–599. doi: 10.1162/003355303321675455
Musyoki, M. E., Busienei, J. R., Gathiaka, J. K., and Karuku, G. N. (2022). Linking farmers’ risk attitudes, livelihood diversification and adoption of climate smart agriculture technologies in the Nyando basin, South-Western Kenya. Heliyon 8:e09305. doi: 10.1016/j.heliyon.2022.e09305
Neway, M. M., and Zegeye, M. B. (2022). Gender differences in the adoption of agricultural technology in north Shewa zone, Amhara regional state, Ethiopia. Cogent Soc. Sci. 8:2069209. doi: 10.1080/23311886.2022.2069209
Oster, E. (2019). Unobservable selection and coefficient stability: theory and evidence. J. Bus. Econ. Stat. 37, 187–204. doi: 10.1080/07350015.2016.1227711
Peterman, A., Behrman, J. A., and Quisumbing, A. R. (2014). “A review of empirical evidence on gender differences in nonland agricultural inputs, technology, and services in developing countries” in Gender in agriculture: Closing the knowledge gap. eds. A. R. Quisumbing, R. Meinzen-Dick, T. L. Raney, A. Croppenstedt, J. A. Behrman, and A. Peterman (Dordrecht: Springer Netherlands), 145–186.
Phan, D. (2012). Migration and credit constraints: theory and evidence from Vietnam. Rev. Dev. Econ. 16, 31–44. doi: 10.1111/j.1467-9361.2011.00644.x
Ramirez, A. (2013). The influence of social networks on agricultural technology adoption. Procedia Soc. Behav. Sci. 79, 101–116. doi: 10.1016/j.sbspro.2013.05.059
Rayhan, S. J., Rahman, M. S., and Lyu, K. (2023). The role of rural credit in agricultural technology adoption: the case of Boro Rice farming in Bangladesh. Agriculture 13:2179. doi: 10.3390/agriculture13122179
Roodman, D. (2011). Fitting fully observed recursive mixed-process models with cmp. Stata J. 11, 159–206. doi: 10.1177/1536867X1101100202
Stark, O., and Bloom, D. E. (1985). The new economics of labor migration. Am. Econ. Rev. 75, 173–178.
Stone, G. D. (2007). Agricultural deskilling and the spread of genetically modified cotton in Warangal. Curr. Anthropol. 48, 67–103. doi: 10.1086/508689
Sui, Y., and Gao, Q. (2023). Farmers’ endowments, technology perception and green production technology adoption behavior. Sustain. For. 15:7385. doi: 10.3390/su15097385
Sunding, D., and Zilberman, D. (2001). “Chapter 4 the agricultural innovation process: research and technology adoption in a changing agricultural sector” in Handbook of agricultural economics (Oxford, UK: North-Holland: Elsevier), 207–261.
Varshney, D., Mishra, A. K., Joshi, P. K., and Roy, D. (2022). Social networks, heterogeneity, and adoption of technologies: evidence from India. Food Policy 112:102360. doi: 10.1016/j.foodpol.2022.102360
Wellman, B. (1979). The community question: the intimate networks of east Yorkers. Am. J. Sociol. 84, 1201–1231. doi: 10.1086/226906
World Bank (2014). World development report 2015: mind, society, and behavior. Washington, D.C.: World Bank. 2015. doi: 10.1596/978-1-4648-0342-0
Yu, L., Yin, X., Zheng, X., and Li, W. (2017). Lose to win: entrepreneurship of returned migrants in China. Ann. Reg. Sci. 58, 341–374. doi: 10.1007/s00168-016-0787-0
Yue, M., Li, W. J., Jin, S., Chen, J., Chang, Q., Glyn, J., et al. (2023). Farmers’ precision pesticide technology adoption and its influencing factors: evidence from apple production areas in China. J. Integr. Agric. 22, 292–305. doi: 10.1016/j.jia.2022.11.002
Zhao, S., and Jiang, Y. (2022). Heterogeneous effects of rural–urban migration and migrant earnings on land efficiency: empirical evidence from China. Land Use Policy 115:106003. doi: 10.1016/j.landusepol.2022.106003
Zhao, Y. (1999). Labor migration and earnings differences: the case of rural China. Econ. Dev. Cult. Change 47, 767–782. doi: 10.1086/452431
Keywords: migrant work experience, adopt new agricultural technology, willingness, farmer, China
Citation: Zhao Q, Yu M, Shi R and Gong R (2024) The impact of migrant work experience on farmers’ willingness to adopt new agricultural technology: insights from China. Front. Sustain. Food Syst. 8:1415489. doi: 10.3389/fsufs.2024.1415489
Edited by:
Ruishi Si, Xi'an University of Architecture and Technology, ChinaReviewed by:
Alain Ndoli, International Union for Conservation of Nature, BangladeshTabussam Tufail, University of Lahore, Pakistan
Copyright © 2024 Zhao, Yu, Shi and Gong. This is an open-access article distributed under the terms of the Creative Commons Attribution License (CC BY). The use, distribution or reproduction in other forums is permitted, provided the original author(s) and the copyright owner(s) are credited and that the original publication in this journal is cited, in accordance with accepted academic practice. No use, distribution or reproduction is permitted which does not comply with these terms.
*Correspondence: Minjie Yu, yuminjie@zjhu.edu.cn