- 1College of Economics and Management, Longdong University, Qingyang, China
- 2Northwest Center for Rural Vitalization Research, Xi’an University of Architecture and Technology, Xi’an, China
To bolster ecological conservation efforts and foster human well-being, the Chinese government has implemented the disaster resettlement program. Rural households encounter various environmental and social challenges regarding disaster resettlement. One of the priorities of disaster resettlement in China is to implement reforms to mitigate disaster losses and improve the livelihoods of households. The research on the livelihood adaptive capacity of households and their research framework provides a new perspective for the livelihood survey of the resettlement population. This article assesses Household Livelihood Adaptive Capacity (HLAC) and further explores how it is impacted by disaster resettlement. Taking Ankang Prefecture in Southern Shaanxi Province as a case, this study investigates the endogeneity and selection bias of resettlement. It employs the Propensity Score Matching method to empirically test the effect of disaster resettlement on household awareness, action, and ability to measure HLAC. The results show that: (1) disaster resettlement significantly reduces HLAC, and (2) poverty alleviation relocation, centralized resettlement, and short-distance relocation have a significant negative impact on HLAC. The Chinese government has tried to use disaster resettlement to address ecological protection and social development problems, and it plays a crucial role in China’s development programs. We provide evidence that disaster resettlement leads to a decrease, rather than an increase, in HLAC. Therefore, we suggest that more follow-up assistance policies should be developed to enhance HLAC.
1 Introduction
The international disaster database (EM-DAT) has reported over 26,000 major disasters globally since 1900 (Ritchie et al., 2023). The total number of global disasters has relatively increased by 214% since 1970 (Asgary et al., 2024). With significant natural hazards, climate change, and environmental degradation around the world, people who reside in impoverished regions in developing countries face enormous survival challenges (Chen et al., 2017). Disaster resettlement solves the problems of communities living in disaster-prone areas, which are often exposed to the dangers posed by riverbanks, coastlines, and mountain slopes, by relocating them to new areas away from such hazards (Pormon et al., 2023). Disaster resettlement plans are adopted as a preventive measure to cope with the increasing risk of disasters (Pormon et al., 2023; Contreras et al., 2013). It is believed that this action constitutes not only a geographical movement but also a change in production, lifestyle, and the reconstruction of social networks for households (Xu et al., 2022). Meanwhile, it is a potential option for households confronted with the need to respond to risks and disasters (Sina et al., 2019a,b). Post-disaster resettlement policies can be considered the lifeblood of post-disaster resettlement projects (Siriwardhana and Kulatunga, 2023). One such policy, the Southern Shaanxi Disaster Resettlement (SSDR) program, is designed for people to move voluntarily from ecologically fragile, steep, remote mountain areas to towns or plains to restore ecosystems and critical ecosystem services, alleviate poverty, and enhance livelihood security. Like other conservation and human development policies worldwide, multiple stakeholders, including local households, are involved in the SSDR. Disaster risk seriously affects the life and property safety and sustainable development of households in disaster areas (Yang et al., 2021; Yang et al., 2023). Relocation, as a solid external shock and policy intervention, poses particular risks to household livelihoods (Xu et al., 2022). Therefore, one of the greatest challenges is to better understand the immediate and potential influences of the SSDR on household well-being and livelihood activities to achieve sustainability goals (Li et al., 2015).
In areas severely affected by climate change, effective adaptation of households is crucial for their survival and development (He et al., 2023). Previous research examining disaster resettlement has mainly focused on population and satisfaction issues (Pormon et al., 2023; Wilmsen and Webber, 2015; Lo and Wang, 2018), the ecological environment, and natural resources problems (Li et al., 2015; Liu and Wu, 2023); however, there are relatively few studies on household sustainable livelihoods and livelihood adaptive capacity within the context of disaster resettlement at the household level. According to Chambers and Conway (1991), livelihood is a family’s ability to earn a living and find a way to make a living based on the ability to own capacity, assets, and activities. Much of the literature on livelihood draws on the Sustainable Livelihoods Framework (SLF) (Wu et al., 2023). In recent years, scholars have increasingly focused on the correlation between disaster resettlement and household livelihoods. For instance, Liu et al. examined rural household livelihood vulnerability under disaster resettlement (Liu et al., 2023). Yang et al. investigated the influencing factors and interrelationships between rural resilient livelihoods and sustainable livelihoods in resettlement communities following significant disasters (Yang et al., 2023). Similarly, Liu et al. (2020a) adopted the framework of livelihood resilience proposed by Speranza et al. (2014), taking the disaster resettlement setting as the research object. The study measured household livelihood resilience and tested how it was impacted by disaster resettlement. Livelihood vulnerability, resilience, and adaptive capacity are pivotal concepts within household livelihood systems that are intricately interconnected and complexly intertwined. Few studies employ quantitative methods to identify and measure rural household livelihood adaptive capacity (Chen et al., 2018; Liu et al., 2022). These existing studies have examined the status of households under disaster resettlement from a livelihood perspective, but there is little research that explores changes in their livelihood adaptive capacity in the context of disaster resettlement from a more detailed angle. From the standpoint of disaster resettlement, this study aimed to conduct a quantitative assessment of rural Chinese household livelihood adaptive capacity (HLAC) to investigate the impact of disaster resettlement on HLAC.
The concept of livelihood adaptive capacity refers to the system’s ability to adjust and modify its characteristics to effectively mitigate damage, capitalize on opportunities, or cope with the impacts of unexpected events (Jones et al., 2010; Thulstrup, 2015; Nyamwanza, 2012). Livelihood adaptive capacity is viewed as a positive property and is critical in fostering sustainable adaptations (Engle, 2011). Household livelihood adaptive capacity (HLAC) refers to the capacity of households to predict and respond to natural or human-induced disturbances, mitigate their impacts, and recover quickly from the consequences (Maldonado and Sanchez, 2014). Some research found that HLAC relies heavily on accessible capital assets, particularly natural capital and biological resources (Chen et al., 2018; Zamasiya et al., 2017; Yin et al., 2019). Many scholars have used the Sustainable Livelihoods Approach Framework (SLA) proposed by the United Kingdom Department for International Development when studying adaptive capacity (Wu et al., 2023; Pagnani et al., 2020), which is a popular integrated approach and pointed out that the framework can describe the complexity of livelihoods at the household level, mainly through human capital, financial capital, social capital, physical capital, and natural capital. While previous studies have detailed the applicability of the method, most studies have yet to address the acquisition of individual abilities. Acosta et al.’s’ framework applies fuzzy logic analysis to combine 12 socioeconomic indicators to generate an adaptive capacity index (Acosta et al., 2013). In the past, the assessment of the HLAC mainly focused on income, poverty, and welfare, which proved challenging in achieving scientific objectivity. Therefore, it is feasible and practical to measure HLAC using the framework applied by Acosta et al., particularly given the analytical framework and indicator quantification. Wu et al. argued that combined capital enhances household adaptability (Wu et al., 2023). The complementary effects of incentivizing livelihood capitals (i.e., material, natural, social, and human capital) can improve HLAC (Wu et al., 2023). The changing dynamics of livelihood capital and the interrelationships among different livelihood capitals influence HLAC (Thulstrup, 2015). Furthermore, the improvement or decline of HLAC is related to the results of micro-individual adaptation, coping with relocation shocks, policy interventions, and climate perceptions (Rogers et al., 2019; Mairura et al., 2021). Its function determines whether households can optimize their livelihood models or fall into the livelihood dilemma (Liu et al., 2020b). Moreover, some studies have combined the vulnerability of household livelihood to analyze and explore changes in HLAC (Chen et al., 2017; Zamasiya et al., 2017; Mekonen and Berlie, 2021). Lower adaptive capacity increases the livelihood vulnerability of households (Mekonen and Berlie, 2021). Among these studies, the evaluation index system constructed by Liu et al., which examined three dimensions—awareness, action, and ability—is conducive to evaluating HLAC (Liu et al., 2022). To dig deeper and provide relevant empirical evidence, based on our previous study, this paper examined whether disaster resettlement can reshape HLAC and the impact of relocation characteristics on HLAC, which is a possible contribution of this paper.
This study is based on survey data relating to 657 households in three counties of Ankang Prefecture, Southern Shaanxi Province, China. Following our previous studies, this article measures HLAC from three dimensions: awareness, action, and ability. It employs Propensity Score Matching (PSM) to examine the influence of disaster resettlement and its characteristics on HLAC. This article may provide helpful information for formulating and implementing policies related to disaster risk management. Compared with previous research, this study makes the following contributions: (1) We assess the level of HLAC under the background of disaster resettlement and supplement relevant studies with cases and evidence; (2) We explore the influence of disaster resettlement and its characteristics on HLAC, which is of great theoretical significance in studying livelihood adaptive capacity at the household level; (3) We study rural households from typical undeveloped areas of the Southern Shaanxi Province, China. The research results could be a reference for developing disaster resettlement prevention systems in other developing regions. As highlighted here, disaster resettlement can alter household livelihood and societal structures, necessitating the transformation of the country’s socio-economic systems into more sustainable states (Chen et al., 2018; Yin et al., 2019). Investigating the effects of disaster resettlement on HLAC holds considerable practical relevance. This study addresses two issues: 1. What is the level of HLAC in the research areas? 2. What is the correlation between HLAC and disaster resettlement? The following sections of this paper are structured as follows: Section 2 outlines the study materials and methods, Section 3 contains the analysis results, and Section 4 presents the discussion and conclusions.
2 Materials and methods
2.1 Study area
This research study was carried out in Ankang Prefecture, one of three prefectures in Southern Shaanxi Province, China, where disaster resettlement programs were implemented (Figure 1; Li et al., 2021). Ankang Prefecture is situated in the central area of the Qinba Mountainous Area, an essential national biodiversity and water conservation ecological area (Liu and Wu, 2023). Rural households have high poverty vulnerability, and many impoverished individuals reside in isolated mountainous regions characterized by severe natural conditions, fragile ecosystems, and inadequate infrastructure development. Relocation in this area affects 220,000 rural households characterized by high levels of livelihood vulnerability, a major livelihood project of concern to the Chinese government, and many of its relocation experiences and practices have been replicated at the national and provincial levels. From 2011 to 2020, the relocation project in Southern Shaanxi Province involved 226,000 rural households in Ankang Prefecture. Moreover, all three surveyed counties are designated as critical national poverty alleviation and development areas with relatively high poverty incidence rates within their jurisdictions. Therefore, the survey area can serve as a representative site for exploring ways to reduce vulnerability, improve HLAC, and improve human well-being.
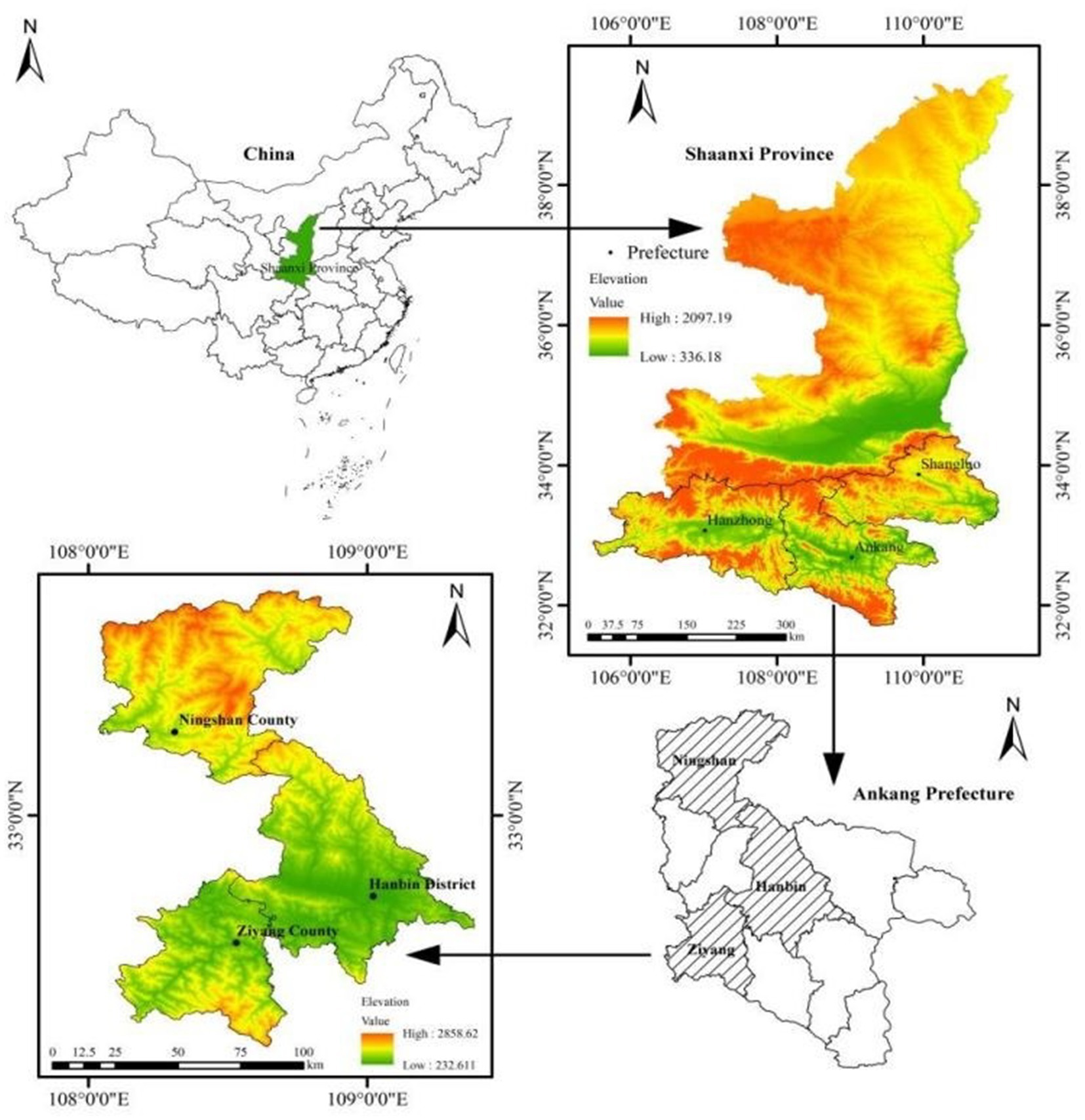
Figure 1. Location of the study area (Liu et al., 2020a).
2.2 Data collection
This study utilizes data from the survey examining rural household livelihood in Ankang Prefecture, located in Southern Shaanxi Province. In the sampling process, three typical concentrated resettlement communities in Ziyang County and eight administrative villages in four towns in Ningshan County and Hanbin District were randomly selected, and questionnaire surveys were conducted among households during the survey period (Liu et al., 2022). Ankang Prefecture is a gathering place for several conservation and development policies, and the case points selected in this survey are representative. Survey data were collected through individual interviews by a trained team of researchers with heads of households or adult family members. During collection, various quality control methods were employed to ensure the accuracy and objectivity of the data collected. A total of 657 valid questionnaires were obtained with a response rate of 98.06%, including 459 respondents who had been relocated and 198 non-relocated households.
2.3 Measuring HLAC
According to the definition of HLAC, we identify HLAC at three levels: awareness, ability, and action. The methodology also provides a practical way to select indicators of HLAC. Households’ awareness of environmental changes and disasters, including whether they are aware that external factors such as climate change and policy shifts may impact their livelihoods, affects their actions. Still, this action depends on the present ability of the person, and different types of situations produce various types of actions or behaviors. Here, the authors suggest selecting HLAC indicators from three levels and six dimensions at the household level. Based on existing literature and experience from past scholars in the context of HLAC, the most relevant indicators are selected (Table 1). In the research framework, awareness is represented by the dimension of experience (Sina et al., 2019b; Alam et al., 2016), which captures how relocated households have responded to previous environmental changes. Households with more extensive experience tend to be more astute in their decision-making when confronted with environmental changes. In this paper, household experience is predominantly represented by work history (Li C. et al., 2017). Ability is reflected across three dimensions: material resources, infrastructure, and technology. Material resources directly reflect the economic capabilities of households, where cultivated land area (Liu and Wu, 2023) and housing type (Li C. et al., 2017) are used. The infrastructure is mainly represented by the distance of the household to the main highway and the products and tools owned by the household; the increase in physical capital helps households shift away from agriculture to non-agriculture and contributes significantly to their income (Shi et al., 2017). Technical skills refer to the household’s skill level and social relations (Li M. et al., 2017), as well as their ability to utilize social networks and resources to cope with changes in the external environment of livelihood systems, including participation in skills training and relationships with village cadre relative (Liu and Wu, 2023). Actions include economic resources and flexibility, characterized by financial assistance, housing value, and agricultural income. Flexibility refers to the ability of households to sustain their livelihoods in the face of external environmental disturbances (Li C. et al., 2017) and how new systems can be reconstructed, including non-agricultural income and household size (Sina et al., 2019b). In summary, based on the quantitative analysis of household surveys, this paper selects 13 indicators to measure HLAC under the background of disaster resettlement.
To further analyze HLAC, this study adopts the method of bias normalization, eliminating the influences of different scales and dimensions and ensuring comparability of the indicators. In addition, questions with multiple-choice answers are specified as specific values on a scale ranging from 0 to 1. For example, housing structures define 1, 0.33, and 0.67 values. The range standardization method is used to standardize each indicator variable, which can eliminate the influence of different dimensions and orders of magnitude of the original data. Thus, we can obtain the HLAC by adding the indicators in Table 1.
2.4 Econometric method
Scholars usually select multiple regression models, plausible irrelevant regression models, and instrumental variables to examine the influencing factors of HLAC. Our study uses Propensity Score Matching (PSM) method to analyze the impact of disaster resettlement on HLAC and to solve the endogeneity and selection bias of disaster resettlement (Li et al., 2015; Ouya et al., 2023). PSM is primarily used to address sample selection bias resulting from non-random assignment, making the treatment and control groups more comparable in terms of covariates. The free choice of whether to participate in disaster resettlement may be endogenous, resulting in non-randomness of the sample and, thus, self-selection problems. The management choices of policymakers regarding project allocation and implementation will also affect disaster resettlement and HLAC. Therefore, these problems will lead to bias in the model estimation results and low reliability. In econometrics, the instrumental variable method, social experiment, and PSM are usually used to solve the above problems. However, the instrumental variable method is highly controversial. Social experiments are challenging to carry out and can be impossible to implement. The PSM method can address issues of bias and low reliability in model estimation results. Moreover, the PSM method is widely applied because of its advantages in reducing the degree of bias of the estimated effect.
For the analysis, considering the sample feature X and the indicator variable T, the propensity score is:
Thus, the average treatment effect of the disaster resettlement policy is:
Further, the average effect of the project ATT can be written as follows:
Among them, T indicates that households participated in relocation, C indicates that they did not participate, N is the sample size of participating households, and W (i, j) is the matching weight. For further details about the PSM method, please refer to Li et al. (2015).
According to the analysis framework of HLAC and the actual situation in the study area, the dependent variable of the PSM model is set as HLAC and its three aspects: awareness, ability, and action. Furthermore, in this paper, we use the expert evaluation method (Sharp, 2003) to determine the weights of each indicator variable, and eliminate the overlap between indicators and subjective assumptions about indicator design as much as possible. Based on the actual situation of previous studies and surveys, the independent variables selected in this paper are the education of household heads, household size, children, livelihood diversity, officers (whether a household member is an official), whether the household participates in the sloping land conservancy program (SLCP), and participation in collective affairs (the number of participations in collective affairs). Xu et al. calculated the degree of livelihood diversification (Xu et al., 2019). Each livelihood activity in which households participate is assigned a value of 1. If a family engages in three livelihood activities (i.e., farming, planting, and migrant work), the livelihood diversification index is 3. The specific definitions and descriptions of each variable are shown in Table 2. In addition, according to the relocation approach, the resettlement is divided into poverty alleviation relocation, disaster avoidance relocation, and ecological restoration relocation. According to the relocation type, it is divided into centralized relocation, non-centralized relocation, long-distance relocation, and short-distance relocation.
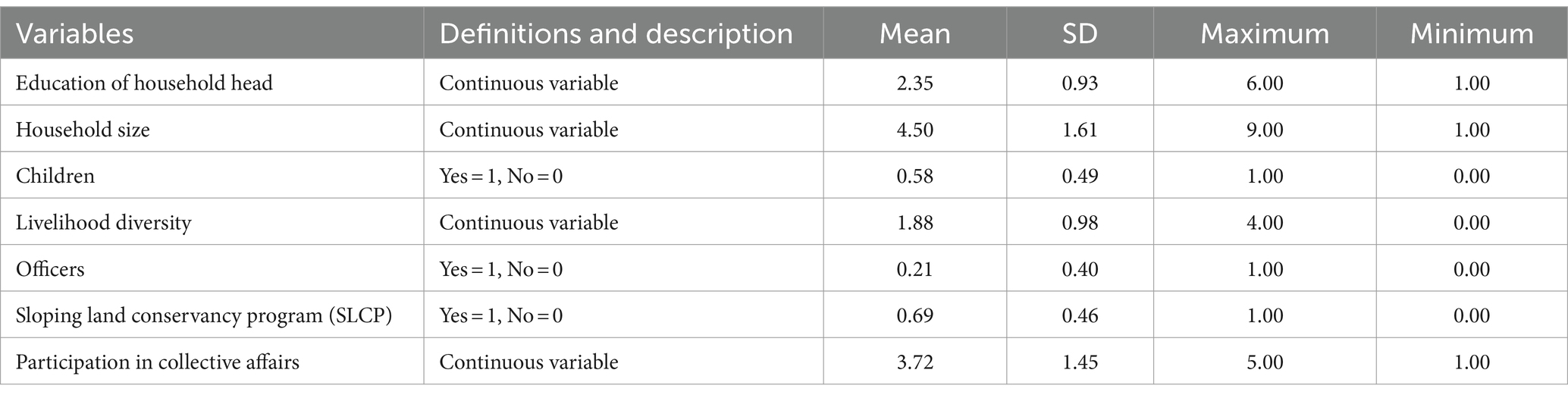
Table 2. Definitions and descriptive information of the determinants of participation in disaster resettlement.
3 Results
3.1 Rural household characteristics in Ankang prefecture
Using field survey data gathered from Ankang Prefecture, this study briefly analyses the sample’s fundamental characteristics. Of the 657 samples, 459 were relocated, and 198 were non-relocated. Among the sample of relocated households, 109 (23.75%) households were relocated under poverty alleviation relocation, 40 (8.71%) under ecological restoration relocation, 64 (13.94%) under project-induced relocation, 201 (43.79%) under disaster avoidance relocation, and 45 (9.80%) for other reasons. Household relocation commonly involves relocating within local communities, local townships, neighboring townships, and other areas. Local communities accounted for the most significant portion of these options, accounting for 54.47%. Following closely behind were local townships at 35.73% and neighboring townships at 4.36%. From the perspective of relocation approaches, 354 households were involved in centralized relocation, 43 in scattered relocations, 52 in self-determined relocations, and 10 households chose other methods. In other words, 77.12% of the households were relocated through centralized relocation, including transferring an entire village to a customized community. According to the length of time since relocation, households were categorized into short-term (less than 3 years), medium-term (between 3 and 5 years), and long-term resettlers (over 5 years). The majority fell into the short-term category (211 households), followed by long-term (141 households) and medium-term resettlement (103 households).
Households choose a variety of livelihoods when faced with disaster resettlement; migrants were divided into four categories: non-farm households, pure farming households, and diversified households, while 45.21% of households chose to work in non-agricultural sectors. 38.66% of households were engaged solely in nonfarming activities, while those involved in pure farming activities accounted for just 16.13%.
3.2 Household livelihood adaptive capacity and resettlement characteristics
In this paper, Stata version 15.1 was used to regress the valid sample of household data. The results of independent variable selection and the estimation results of the probit model are shown in Table 3. Overall, the Pseudo R2 value estimated by the model was 0.12, the chi-square statistic was 92.11, and the log-likelihood value was −352.79, indicating that the overall fitting effect of the model is good. At the same time, the selection of each independent variable met the balance requirements. Table 3 shows the impact of each variable inputted into the model on the household’s participation in disaster resettlement. In the probit model, the education of household heads had a significant negative impact on the involvement in disaster resettlement. This indicates that, with the improvement of the efficiency of target identification by resettlement policies, rural households with low education levels are more likely to be identified as target households and can achieve “relocation” under the guidance of policies. In addition, rural households with children tend to participate in disaster resettlement, and one of the most essential incentives is that rural children are offered the rare opportunity of gaining access to equitable education. The livelihood diversification index for participation in collective affairs, the number of public officials, and the level of involvement in SLCP significantly negatively affected participation in disaster resettlement. The following households did not want to participate in resettlement: households with a higher livelihood diversification index for participation in collective affairs had relatives who were officials or had participated in SLCP. Households with high levels of participation in collective affairs were less likely to participate in disaster resettlement. This may be because, although they had actively participated in collective affairs and moved closer to the local government, certain relocation costs prevented them from moving into the resettlement community. Therefore, the relocation policy has become an effective means to accurately identify vulnerable groups and people living in deep poverty, contributing to the Chinese government’s efforts to address rural poverty issues tangibly.
3.3 The impact of disaster resettlement on HLAC
According to the estimation results of the probit model, the Kernel matching method was selected to calculate the probability that each household would participate in relocation, poverty alleviation relocation, disaster avoidance relocation, ecological restoration relocation, centralized resettlement, non-centralized resettlement, long-distance relocation, short-distance relocation, and non-participation in relocation. This is also termed the ‘propensity score’. To test the matching results of the propensity scores of each sample, Figures 2a–h presents the propensity score density matching graphs for various household samples before and after matching, indicating the disparity between the treatment group and control group, both pre- and post-sample matching.
Based on the propensity score calculated above, this study matched the propensity values of relocated households, different relocation types of rural households, different relocation approaches, and different relocation distances between rural households and non-relocated households. We used the livelihood adaptive capacity of matched non-relocated households as the counterfactual capital of relocated households and other households in the absence of relocation. Table 4 shows the estimated effects on HLAC, awareness, ability, and action based on econometric models of disaster resettlement and relocation types. Disaster resettlement can negatively reduce HLAC and the actions of rural households. Poverty alleviation using relocation can decrease HLAC.
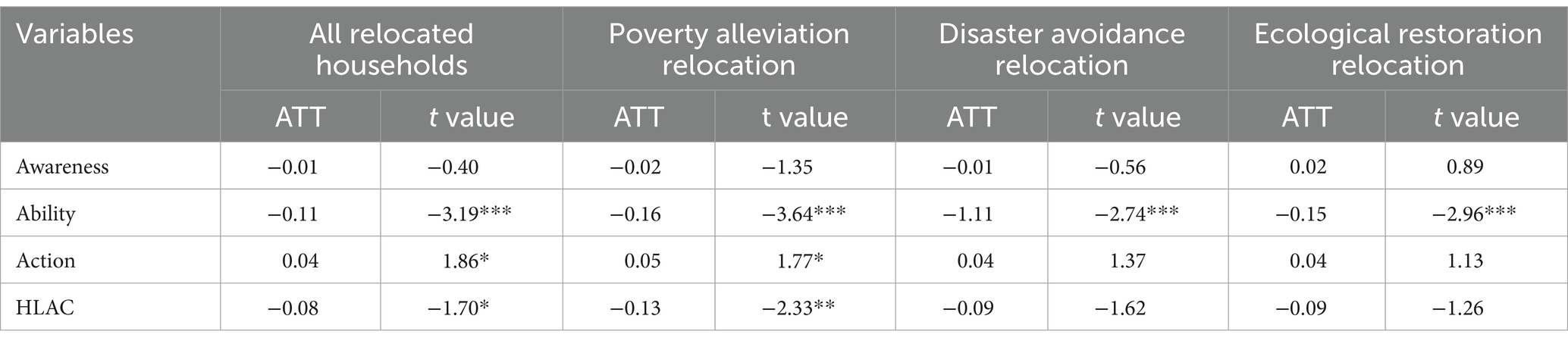
Table 4. Impact of different relocation approaches on livelihood adaptive capacity (HLAC) by Kernel matching.
The influence of disaster relocation approaches on HLAC varied. Centralized resettlement had a significant negative impact on HLAC, as shown in Table 5. This finding can be explained as follows: As a result of household resettlement, households lost the land they had originally occupied, significantly reducing the cultivated land area. At the same time, when the resettlement subsidy for rural households ceased, household channels for accessing funds were restricted. Households were unable to cope with the livelihood pressure associated with disaster resettlement. Therefore, HLAC has declined dramatically. In terms of the relocation distance, Table 5 shows that the impact of short-distance relocation on HLAC was significant. After the short-distance relocation, due to changes in the environment and resources, for example, residents’ living habits have also changed. Indeed, moving to a new area requires people to adapt to new folk customs, production modes, and lifestyles. Therefore, it is unsurprising that HLAC, using this relocation method, has experienced a decline.
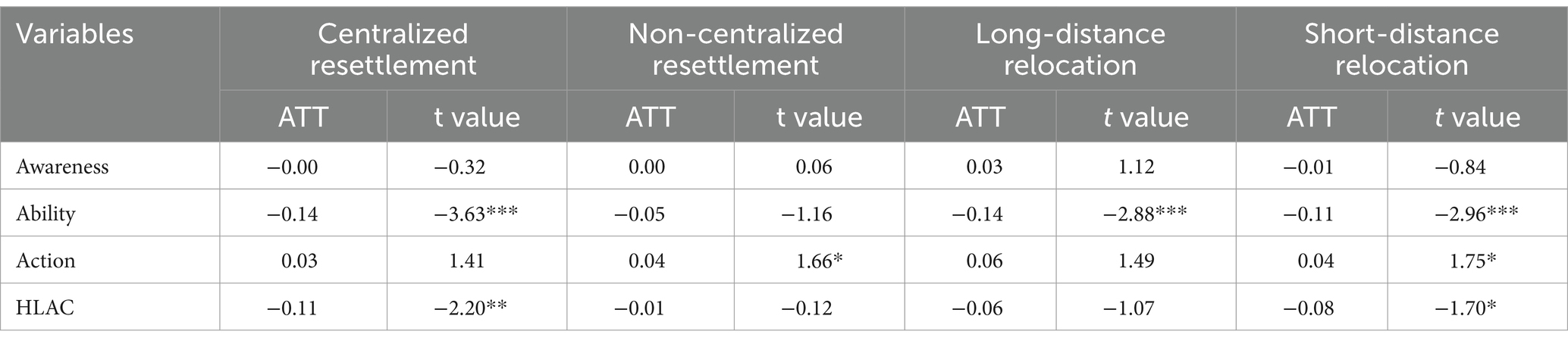
Table 5. Impact of different relocation approaches on livelihood adaptive capacity (HLAC) by Kernel matching.
Under the PSM method, the matching effect must be tested to determine whether the matching result can be used as a counterfactual result. In this study, the reliability of the conclusions in Tables 4, 5 was tested, and the balance test results of the matching of relocated and non-relocated households were determined, as shown in Table 6. We found that the absolute value of the deviation of all variables in the kernel matching was less than 10%. The t-test revealed no significant difference between the treatment group and the control group after all variables were matched. This indicated that the respective variables could no longer provide new information regarding the participation of households in relocation after matching. The matching effect met the test requirements. Therefore, the results of the balance test are satisfactory, and the findings reported in Tables 4, 5 were deemed reliable.
4 Discussion
Disaster resettlement is a vital development strategy to mitigate natural disasters and promote the livelihood transformation of rural households. Our study found that disaster resettlement had a significant negative impact on HLAC, which is similar to the findings of previous studies, which suggested that disaster resettlement increases the vulnerability of resettled households (Chen et al., 2017; Sina et al., 2019a; Galarza-Villamar et al., 2018); indeed, an increase in vulnerability level implies a decrease in HLAC (Liu et al., 2022). According to their research, disaster resettlement indirectly reduces HLAC. Many studies show that households’ recovery and adaptation can take 3–5 years or longer. In the short term, especially within 5 years, their livelihoods will face a slow recovery process. Despite government efforts, challenges in policy implementation often lead to a decline in livelihood adaptation. Continued government support is crucial for long-term development. Therefore, some planned relocation programs may be counterproductive in the short term (Fernando and Jayasinghe, 2023). Furthermore, government-driven, recovery-focused policies may not contribute to the long-term sustainability of rural household livelihoods (Yang et al., 2023). Resettlement has significant positive environmental and social impacts (Liu et al., 2023; Rogers et al., 2019; Li C. et al., 2017; Wang et al., 2018); a significant increase in households’ average and total income and more excellent employment opportunities following disaster resettlement have been reported (Liu et al., 2020a; Rogers et al., 2019; Liu et al., 2020b; Li C. et al., 2017). Resettlement also significantly improves the overall livelihood capital of households, especially physical capital (Liu et al., 2020a). However, the vast costs of resettlement generate a heavy financial burden, which may pose significant challenges for households (Lo and Wang, 2018; Liu et al., 2020a; Lo et al., 2016). As a result, households’ livelihoods change with the various livelihood capitals that they have at their disposal, which simultaneously reshapes HLAC. Therefore, identifying ways to improve HLAC and household livelihoods is complex.
The effects of disaster resettlement characteristics on HLAC differ. Among the reasons for relocation, only poverty alleviation relocation had significant negative impacts on HLAC, whereas disaster avoidance relocation and ecological restoration relocation had no considerable effect on HLAC. The adverse effects of resettlement became evident in the pre-relocation stage (Nikuze et al., 2019). As one of the relocation approaches, poverty alleviation relocation will weaken household livelihood resilience (Liu et al., 2020a). As a result of anti-poverty relocation, the socio-economic conditions surrounding the relocated households change (Nikuze et al., 2019). Changes in livelihood assets and the economy after relocation will impact HLAC. Among relocation types, centralized resettlement had a significant negative effect on HLAC. After centralized resettlement, rural households lost the land they had originally occupied and had less cultivated land. Therefore, households that had once relied on cultivation found themselves in a situation whereby their cash incomes were lost (Li C. et al., 2017). This led to a decline in HLAC. In addition, centralized resettlement households are more inclined to choose expansion strategies and have already begun transitioning faster from predominantly agricultural lifestyles to more diverse and non-agricultural ones (Li C. et al., 2017). Moreover, government follow-up support is lacking (Rogers et al., 2019).
Regarding relocation distance, the current study found that short-distance relocation adversely affected HLAC. According to Lo et al. (2016), long-distance relocation and short-distance relocation can affect resettlement outcomes. However, we found that only short-distance relocation significantly impacted HLAC. Short-distance relocation households enjoy land compensation rights in the resettlement area, particularly in terms of government support. Land compensation rights significantly mitigate the effects of land loss on households. In the long run, most households are overly reliant on land and fail to broaden their livelihood methods, which will still lead to a decline in their HLAC. Furthermore, the proximity to urban centers means that many long-distance relocation households find it easier to secure non-agricultural employment and job positions such as builders, cooks, drivers, and cleaners (Lo and Wang, 2018). Therefore, compared with long-distance relocation, households that are relocated short distances away find it more difficult to adapt in terms of their livelihoods. Thus, the government should provide financial assistance and ensure the livelihoods of households participating in short-distance relocation to promote better adaptation. Finally, factors including the education level of household heads, whether there are children in the household, livelihood diversity, participation in collective affairs, the number of public officials, and participation in SLCP all had significant negative impacts on participation in disaster resettlement.
The study has some limitations. First, it only examined the current impact on HLAC, and there is a need to carry out follow-up studies to explore how HLAC changes over time. Second, selecting HLAC indicators may not be perfect, and each indicator will mean different things in different contexts (Chen et al., 2018). Multiple socio-economic indicators should be included. Third, weights for each indicator were determined using the expert evaluation method. However, to ensure greater data accuracy, comparisons should be performed using different analytical methods. Finally, this paper selected the southern region of Shaanxi Province, China, which limited the scope of the survey, research findings may vary in different localities, so the results may only apply to less developed areas.
5 Conclusion
Under the background of disaster resettlement and sustainable development, the importance of household livelihood has gradually come to the fore (Chen et al., 2017; Sina et al., 2019a; Yang et al., 2021; Peng et al., 2022; Salgueiro-Otero et al., 2022; Yuhan et al., 2021). The current study provides empirical evidence that highlights the impacts of disaster resettlement on HLAC. The results show that disaster resettlement and its characteristics negatively affected HLAC. The main findings of this study are as follows: First, disaster resettlement significantly reduced HLAC. Second, relocation under a poverty alleviation program undermined HLAC. Third, centralized resettlement and short-distance relocation led to a dramatic decline in HLAC.
Disaster resettlement can significantly reduce HLAC. Adaptation is a complex process, and anticipative or designed adaptation is not always effective. Indeed, public policy and adaptation strategies have not always been successful. There is a pressing need to evaluate the implementation of adaptative guidance and resilience strategies. Future research that considers adaptation strategies, adaptive outcome, and livelihood risks in the context of disaster resettlement are needed. Relocated households, especially those with children, are more eager to receive a good education than non-relocated households. This study advocates that the government should implement preferential educational policies in the resettlement areas to meet the desire of relocated households to improve their children’s education. Follow-up support should consider more influential indicators such as school district placement, skills training, employment assistance, and public facilities to improve the HLAC of relocated households. It is essential to assist relocated households in rebuilding and adjusting their livelihoods for sustainable development. Poverty alleviation relocation, centralized resettlement, and short-distance relocation can lead to a pronounced reduction in HLAC. Therefore, in formulating subsequent assistance policies, there should be a certain degree of bias toward poverty alleviation relocation, centralized resettlement, and short-distance relocation for households to ensure fairness and justice while enhancing the well-being of relocated households. The views of households should be considered when formulating policies related to sustainability (Mairura et al., 2021). Early and effective communication of project details and the involvement of affected households in decision-making can help to safeguard their livelihoods (Nikuze et al., 2019). The purpose of planned relocation should focus not only on relocating groups away from hazardous areas but also on the social, economic, political, and institutional causes of vulnerability, which should be addressed (Fernando and Jayasinghe, 2023). In addition, effective community participation is conducive to resettlement (Jamshed et al., 2018; Jamshed et al., 2019). Therefore, after resettlement, we should also pay attention to the effective participation of the community. Although the results may vary among different survey sites, the studied area was very representative, and our findings are likely to apply to other less-developed regions worldwide. The constructed index system of the influencing factors of HLAC can serve as a specific supplement to micro-level research on disaster resettlement. It provides typical cases for studying households under different backgrounds. The research findings offer a basis for formulating targeted policy recommendations and are of important theoretical and practical significance.
Livelihood adaptive capacity is currently a priority topic in global development. Disaster resettlement is regarded as an opportunity to improve the livelihood adaptive capacity. However, resettlement projects can also have adverse effects, such as poverty, sudden changes in livelihood choices (Mohit et al., 2010), and disruption of social capital (Contreras et al., 2013). These factors may lead to dissatisfaction with relocated households (Aulia and Ismail, 2013). This study provides specific information on the link between the context of disaster resettlement and HLAC. Future research on HLAC, in the context of post-disaster relocation, should continue to examine its spatiotemporal changes, refine the HLAC indicator system, compare results across different research methodologies, and focus on adaptation strategies and outcomes among these populations. Through longitudinal studies, track and research the adaptation strategies of resettled populations to enhance the HLAC.
Data availability statement
The original contributions presented in the study are included in the article/Supplementary material, further inquiries can be directed to the corresponding author.
Author contributions
TZ: Writing – review & editing, Visualization, Project administration, Conceptualization. XW: Writing – review & editing, Writing – original draft, Visualization, Validation, Software, Methodology, Funding acquisition, Data curation. YC: Writing – original draft, Supervision, Software. WL: Writing – review & editing, Validation, Supervision, Resources, Methodology, Investigation, Funding acquisition, Data curation, Conceptualization.
Funding
The author(s) declare that financial support was received for the research, authorship, and/or publication of this article. This work was partially financed by the National Natural Science Foundation of China (No. 71803149; 72022014; 723B2019, and 72474173), the Ministry of Education Humanities and Social Science Research Youth Fund Project (No. 22XJC630007), the Natural Science Foundation of Shaanxi Province (No. 2023JCYB607 and No. 2024JC-YBQN-0758), and the Social Science Foundation of Shaanxi Province (No. 2023R290).
Acknowledgments
The authors are very grateful to the many people interviewed for their patience and assistance.
Conflict of interest
The authors declare that the research was conducted in the absence of any commercial or financial relationships that could be construed as a potential conflict of interest.
Publisher’s note
All claims expressed in this article are solely those of the authors and do not necessarily represent those of their affiliated organizations, or those of the publisher, the editors and the reviewers. Any product that may be evaluated in this article, or claim that may be made by its manufacturer, is not guaranteed or endorsed by the publisher.
Supplementary material
The Supplementary material for this article can be found online at: https://www.frontiersin.org/articles/10.3389/fsufs.2024.1412058/full#supplementary-material
References
Acosta, L., Klein, R. J. T., Reidsma, P., Metzgerf, M. J., Rounsevellf, M. D. A., Leemansg, R., et al. (2013). A spatially explicit scenario-driven model of adaptive capacity to global change in Europe. Glob. Environ. Change 23, 1211–1224. doi: 10.1016/j.gloenvcha.2013.03.008
Alam, G. M., Alam, K., and Mushtaq, S. (2016). Influence of institutional access and social capital on adaptation decision: empirical evidence from hazard-prone rural households in Bangladesh. Ecol. Econ. 130, 243–251. doi: 10.1016/j.ecolecon.2016.07.012
Asgary, A., Naeemi, P., Ganguli, N., Tofighi, M., Attarian, K., and Fioretto, T. (2024). Road to resettlement: understanding post-disaster relocation and resettlement challenges and complexities through a serious game. Int. J. Disaster Risk Sci. 15, 1–15. doi: 10.1007/s13753-024-00580-8
Aulia, D. N., and Ismail, A. M. (2013). Residential satisfaction of middle income population: Medan city. Procedia Soc. Behav. Sci. 105, 674–683. doi: 10.1016/j.sbspro.2013.11.070
Chambers, R., and Conway, G. R. (1991). Sustainable rural livelihoods: practical concepts for the 21st century. IDS Discussion Paper, no. 296.
Chen, Y., Tan, Y., and Luo, Y. (2017). Post-disaster resettlement and livelihood vulnerability in rural China. Disaster Prev Manag 26, 65–78. doi: 10.1108/DPM-07-2016-0130
Chen, J., Yin, S., Yang, X. J., and Gebhardt, H. (2018). Farmers’ livelihood adaptation to environmental change in an arid region: a case study of the Minqin oasis, northwestern China. Ecol. Indic. 93, 411–423. doi: 10.1016/j.ecolind.2018.05.017
Contreras, D., Blaschke, T., Kienberger, S., and Zeil, P. (2013). Spatial connectivity as a recovery process indicator: the L’Aquila earthquake. Technol. Forecast Soc. 80, 1782–1803. doi: 10.1016/j.techfore.2012.12.001
Engle, N. L. (2011). Adaptive capacity and its assessment. Glob. Environ. Change 21, 647–656. doi: 10.1016/j.gloenvcha.2011.01.019
Fernando, N., and Jayasinghe, N. (2023). Disaster-induced relocation of vulnerable households: evidence from planned relocation in Sri Lanka. Rebuilding Communities Displacement, 245–268. doi: 10.1007/978-3-031-21414-1_11
Galarza-Villamar, J. A., Leeuwis, C., Pila-Quinga, G. M., Cecchi, F., and Párraga-Lema, C. M. (2018). Local understanding of disaster risk and livelihood resilience: the case of rice smallholders and floods in Ecuador. Int. J. Disaster Risk Reduct. 31, 1107–1120. doi: 10.1016/j.ijdrr.2018.08.009
He, X., Huang, A., Yan, J., Zhou, H., Wu, Y., Yang, L. E., et al. (2023). Smallholders’ climate change adaptation strategies on the eastern Tibetan plateau. Nat. Hazards 118, 641–667. doi: 10.1007/s11069-023-06022-w
Jamshed, A., Rana, I. A., Khan, M. A., Agarwal, N., Ali, A., and Ostwal, M. (2018). Community participation framework for post-disaster resettlement and its practical application in Pakistan. Disaster Prev Manag 27, 604–622. doi: 10.1108/DPM-05-2018-0161
Jamshed, A., Rana, I. A., McMillan, J. M., and Birkmann, J. (2019). Building community resilience in post-disaster resettlement in Pakistan. Int. J. Disaster Resil. Built. Environ. 10, 301–315. doi: 10.1108/IJDRBE-06-2019-0039
Jones, L., Ludi, E., and Levine, S. (2010). Towards a characterisation of adaptive capacity: a framework for analysing adaptive capacity at the local level. Overs. Dev. Inst 2010:8.
Li, C., Guo, M. M., Li, S. Z., and Feldman, M. W. (2021). The impact of the anti-poverty relocation and settlement program on rural households’ well-being and ecosystem dependence: evidence from western China. Soc. Nat. Resour. 34, 40–59. doi: 10.1080/08941920.2020.1728455
Li, M., Huo, C., Peng, H., Qiu, Z., Shangguan, C., Chang, C., et al. (2017). Complementary livelihood capital as a means to enhance adaptive capacity: a case of the loess plateau, China. Glob Environ Change. 47, 143–152. doi: 10.1016/j.gloenvcha.2017.10.004
Li, C., Li, S., Feldman, M. W., Li, J., Zheng, H., and Daily, G. C. (2017). The impact on rural livelihoods and ecosystem services of a major relocation and settlement program: a case in Shaanxi, China. Ambio 47, 245–259. doi: 10.1007/s13280-017-0941-7
Li, C., Zheng, H., Li, S., Chen, X., Li, J., Zeng, W., et al. (2015). Impacts of conservation and human development policy across stake holders and scales. PNAS 112, 7396–7401. doi: 10.1073/pnas.1406486112
Liu, W., Cheng, Y., Li, J., and Feldman, M. (2022). Livelihood adaptive capacities and adaptation strategies of relocated households in rural China. Front. Sustain. Food Syst. 6:1067649. doi: 10.3389/fsufs.2022.1067649
Liu, W., Gao, J., Xu, J., and Li, C. (2023). Estimating livelihood vulnerability and its impact on adaptation strategies in the context of disaster avoidance resettlement in southern Shaanxi, China. Agriculture 13:1497. doi: 10.3390/agriculture13081497
Liu, W., Li, J., and Jie, X. (2020b). Impact of the ecological resettlement program in southern Shaanxi Province, China on households’ livelihood strategies. Forest Policy Econ. 120:102310. doi: 10.1016/j.forpol.2020.102310
Liu, W., Li, J., and Xu, J. (2020a). Effects of disaster-related resettlement on the livelihood resilience of rural households in China. Int. J. Disaster Risk Reduct. 49:101649. doi: 10.1016/j.ijdrr.2020.101649
Liu, W., and Wu, X. (2023). Poverty alleviation resettlement and household natural resources dependence: a case study from Ankang prefecture, China. Agriculture 13:1034. doi: 10.3390/agriculture13051034
Lo, K., and Wang, M. (2018). How voluntary is poverty alleviation resettlement in China. Habitat Int. 73, 34–42. doi: 10.1016/j.habitatint.2018.01.002
Lo, K., Xue, L., and Wang, M. (2016). Spatial restructuring through poverty alleviation resettlement in rural China. J. Rural. Stud. 47, 496–505. doi: 10.1016/j.jrurstud.2016.06.006
Mairura, F. S., Musafiri, C. M., Kiboi, M. N., Macharia, J. M., Ng'etich, O. K., Shisanya, C. A., et al. (2021). Determinants of farmers’ perceptions of climate variability, mitigation, and adaptation strategies in the central highlands of Kenya. Weather Clim. Extreme 34:100374. doi: 10.1016/j.wace.2021.100374
Maldonado, J. H. R., and Sanchez, D. P. M. (2014). Estimating the adaptive capacity of local communities at marine protected areas in Latin America: a practical approach. Ecol. Soc. 19, 1–16. doi: 10.5751/ES-05962-190116
Mekonen, A. A., and Berlie, A. B. (2021). Rural households’ livelihood vulnerability to climate variability and extremes: a livelihood zone-based approach in the northeastern highlands of Ethiopia. Ecol. Process. 10:1. doi: 10.1186/s13717-021-00313-5
Mohit, M. A., Ibrahim, M., and Rashid, Y. R. (2010). Assessment of residential satisfaction in newly designed public low-cost housing in Kuala Lumpur, Malaysia. Habitat. Int. 34, 18–27. doi: 10.1016/j.habitatint.2009.04.002
Nikuze, A., Sliuzas, R., Flacke, J., and van Maarseveen, M. (2019). Livelihood impacts of displacement and resettlement on informal households - a case study from Kigali, Rwanda. Habitat Int. 86, 38–47. doi: 10.1016/j.habitatint.2019.02.006
Nyamwanza, A. M. (2012). Livelihood resilience and adaptive capacity: a critical conceptual review: original research. JÀMBÁ 4, 1–6.
Ouya, F. O., Murage, A. W., Pittchar, J. O., Chidawanyika, F., Pickett, J. A., and Khan, Z. R. (2023). Impacts of climate-resilient push–pull technology on farmers’ income in selected counties in Kenya and Tanzania: propensity score matching approach. Agric. Food Secur. 12:15. doi: 10.1186/s40066-023-00418-4
Pagnani, T., Gotor, E., and Caracciolo, F. (2020). Adaptive strategies enhance smallholders’ livelihood resilience in Bihar, India. Food Secur. 13, 419–437. doi: 10.1007/s12571-020-01110-2
Peng, W., Robinson, B. E., Zheng, H., Li, C., Wang, F., and Li, R. (2022). The limits of livelihood diversification and sustainable household well-being, evidence from China. Environ. Dep. 43:100736. doi: 10.1016/j.envdev.2022.100736
Pormon, M. M. M., Yee, D. K. P., and Gerona, C. K. O. (2023). Households condition and satisfaction towards post-disaster resettlement: the case of typhoon Haiyan resettlement areas in Tacloban City. Int. J. Disaster Risk Reduct. 91:103681. doi: 10.1016/j.ijdrr.2023.103681
Rogers, S., Li, J., Lo, K., Guo, H., and Li, C. (2019). Moving millions to eliminate poverty: China’s rapidly evolving practice of poverty resettlement. Dev. Policy Rev. 38, 541–554. doi: 10.1111/dpr.12435
Salgueiro-Otero, D., Barnes, M. L., and Ojea, E. (2022). Transformation in times of climate change: what makes a fisher diversify livelihoods? Front. Mar. Sci. 1373:888288. doi: 10.3389/fmars.2022.888288
Sharp, K. (2003). Measuring destitution: Integrating qualitative and quantitative approaches in the analysis of survey data. Michigan: Institute of development studies.
Shi, X., Zhao, S., Lu, S., Wang, T., and Xu, X. (2017). The effect of farmers’ livelihood capital on non-agricultural income based on the regulatory effect of returning farmland to forests: a case study of Qingyuan Manchu Autonomous County in China. Small Scale For. 11, 1–25. doi: 10.1007/s11842-023-09554-y
Sina, D., Chang-Richards, A. Y., Wilkinson, S., and Potangaroa, R. (2019a). A conceptual framework for measuring livelihood resilience: relocation experience from Aceh, Indonesia. World Dep. 117, 253–265. doi: 10.1016/j.worlddev.2019.01.003
Sina, D., Chang-Richards, A. Y., Wilkinson, S., and Potangaroa, R. (2019b). What does the future hold for relocated communities post-disaster? Factors afecting livelihood resilience. Int. J. Disaster Risk Reduct. 34, 173–183. doi: 10.1016/j.ijdrr.2018.11.015
Siriwardhana, S. D., and Kulatunga, U. (2023). Evolution of post-disaster reconstruction policy framework in Sri Lanka: a longitudinal case study. Int. J. Disaster Risk Reduct. 85:103506. doi: 10.1016/j.ijdrr.2022.103506
Speranza, C. I., Wiesmann, U., and Rist, S. (2014). An indicator framework for assessing livelihood resilience in the context of social–ecological dynamics. Glob. Environ. Change. 28, 109–119. doi: 10.1016/j.gloenvcha.2014.06.005
Thulstrup, A. W. (2015). Livelihood resilience and adaptive capacity: tracing changes in household access to capital in Central Vietnam. World Dep. 74, 352–362. doi: 10.1016/j.worlddev.2015.05.019
Wang, W., Ren, Q., and Yu, J. (2018). Impact of the ecological resettlement program on participating decision and poverty reduction in southern Shaanxi, China. For Policy Econ. 95, 1–9. doi: 10.1016/j.forpol.2018.06.007
Wilmsen, B., and Webber, M. (2015). What can we learn from the practice of development-forced displacement and resettlement for organised resettlements in response to climate change? Geoforum 58, 76–85. doi: 10.1016/j.geoforum.2014.10.016
Wu, X., Nie, X., Chen, Z., Wang, H., Kang, Q., and Lei, W. (2023). How to improve the adaptive capacity of coastal zone farm households?——explanation based on complementary and alternative relationships of livelihood capital in a sustainable livelihood framework. Ocean Coasta Manag. 245:106853. doi: 10.1016/j.ocecoaman.2023.106853
Xu, D., Deng, X., Guo, S., and Liu, S. (2019). Sensitivity of livelihood strategy to livelihood capital: An empirical investigation using nationally representative survey data from rural China. Soc. Indic. Res. 144, 113–131. doi: 10.1007/s11205-018-2037-6
Xu, J., Liu, W., Li, J., Li, C., and Feldman, M. (2022). Disaster resettlement and adaptive capacity among rural households in China. Soc. Nat. Resour. 35, 245–259. doi: 10.1080/08941920.2022.2048329
Yang, X., Guo, S., Deng, X., Wang, W., and Xu, D. (2021). Study on livelihood vulnerability and adaptation strategies of farmers in areas threatened by different disaster types under climate change. Agriculture 11:1088. doi: 10.3390/agriculture11111088
Yang, X., Li, X., Lu, K., and Peng, Z. R. (2023). Integrating rural livelihood resilience and sustainability for post-disaster community relocation: a theoretical framework and empirical study. Nat. Hazards 116, 1775–1803. doi: 10.1007/s11069-022-05739-4
Yin, S., Yang, X. J., and Chen, J. (2019). Adaptive behavior of farmers’ livelihoods in the context of human-environment system changes. Habitat Int. 100:102185. doi: 10.1016/j.habitatint.2020.102185
Yuhan, Q., Xingmin, S., Xueping, L., and Jianwu, Y. (2021). Geographical indication agricultural products, livelihood capital, and resilience to meteorological disasters: evidence from kiwifruit farmers in China. Environ. Sci. Pollut. R. 46, 65832–65847. doi: 10.1007/s11356-021-15547-1
Keywords: disaster resettlement, relocated households, sustainable livelihoods, livelihood adaptive capacity, China
Citation: Zhao T, Wu X, Cheng Y and Liu W (2024) Does disaster resettlement reshape household livelihood adaptive capacity in rural China? Front. Sustain. Food Syst. 8:1412058. doi: 10.3389/fsufs.2024.1412058
Edited by:
Mirgissa S Kaba, Addis Ababa University, EthiopiaReviewed by:
Peng Jiquan, Jiangxi University of Finance and Economics, ChinaXin Deng, Sichuan Agricultural University, China
Copyright © 2024 Zhao, Wu, Cheng and Liu. This is an open-access article distributed under the terms of the Creative Commons Attribution License (CC BY). The use, distribution or reproduction in other forums is permitted, provided the original author(s) and the copyright owner(s) are credited and that the original publication in this journal is cited, in accordance with accepted academic practice. No use, distribution or reproduction is permitted which does not comply with these terms.
*Correspondence: Tiejun Zhao, enRqMjAwNDA3QDEyNi5jb20=